Click the Blue Text to Follow Us
Source | Advanced Manufacturing
1. Background and Significance
2. Overall Situation of Large Model and Its Industrial Application Development
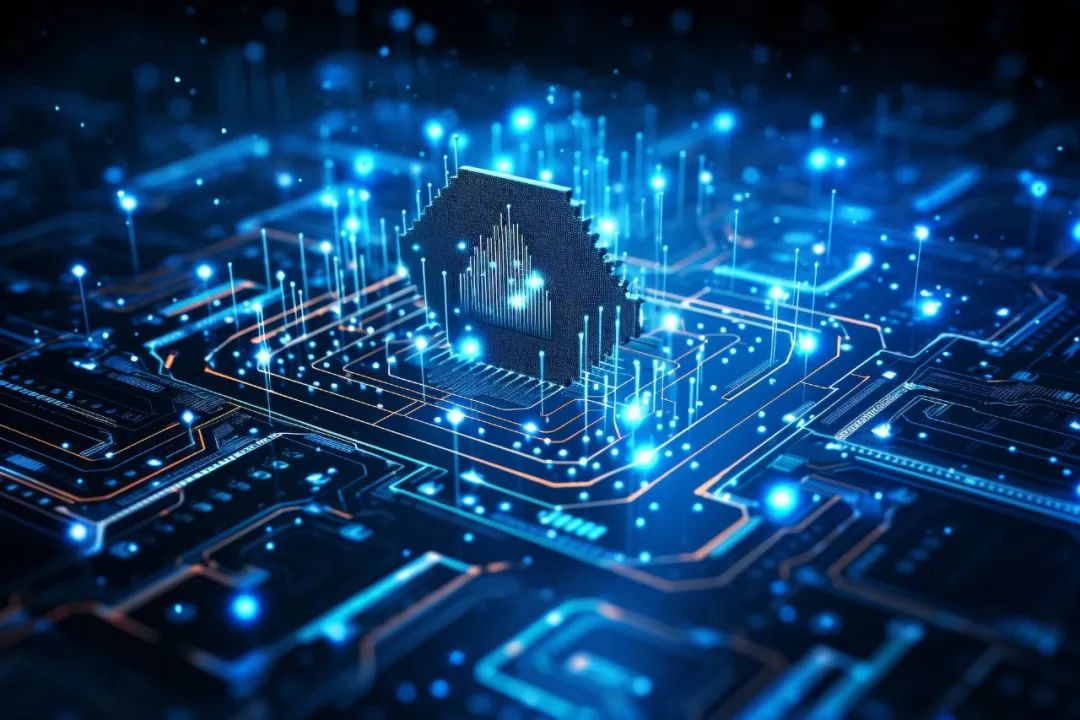
3. Seven Major Models of Large Model Industrial Applications
4. Suggestions for Future Development
END

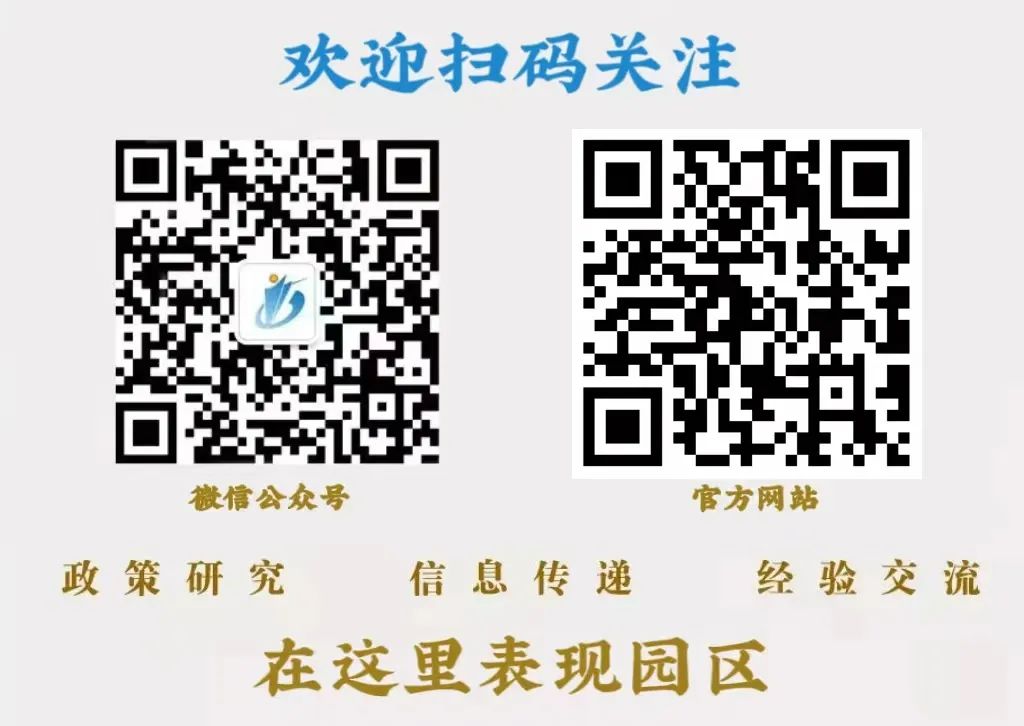