Machine Heart Editorial Team
The “Seven Heroes” of large language models compete to see who comes out on top.
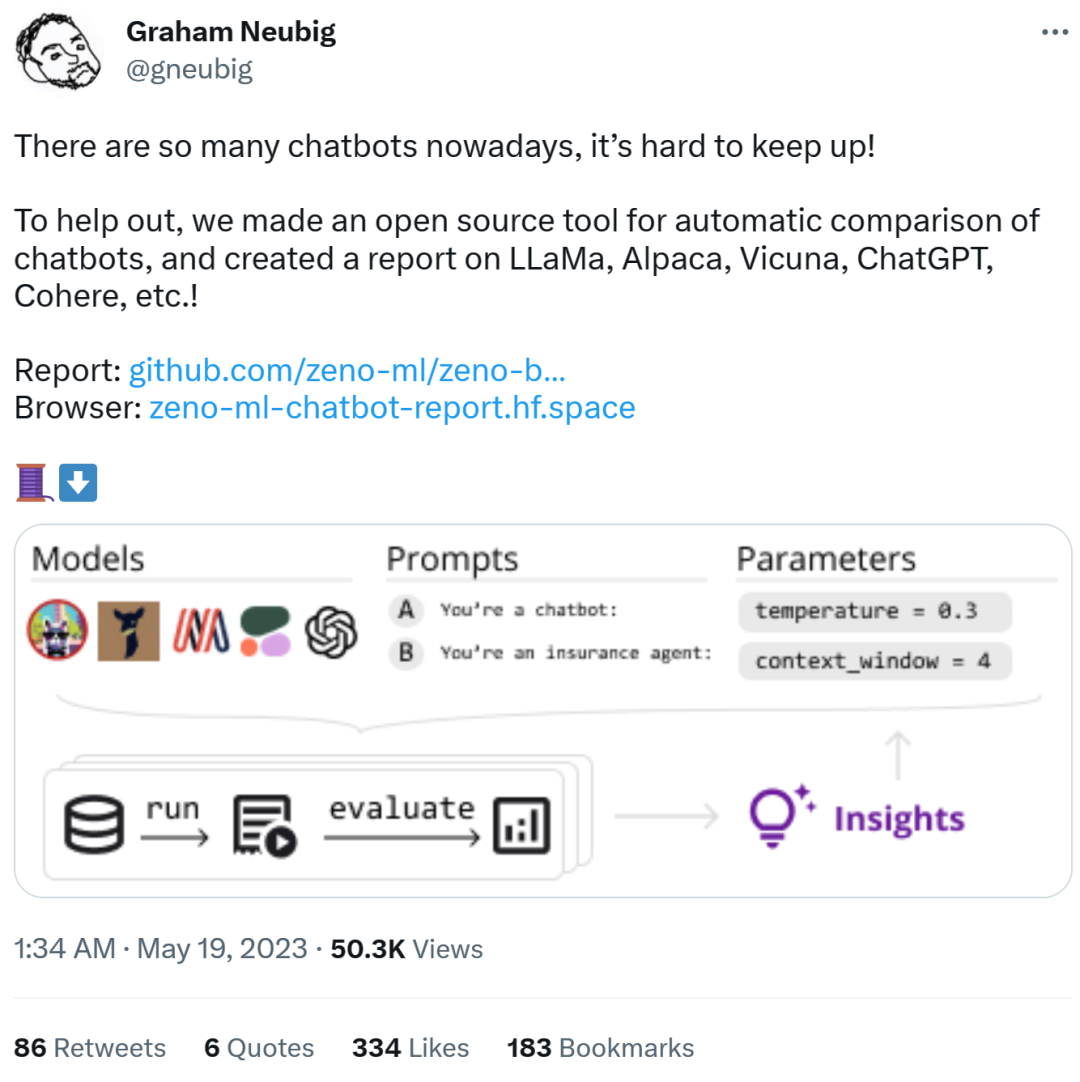
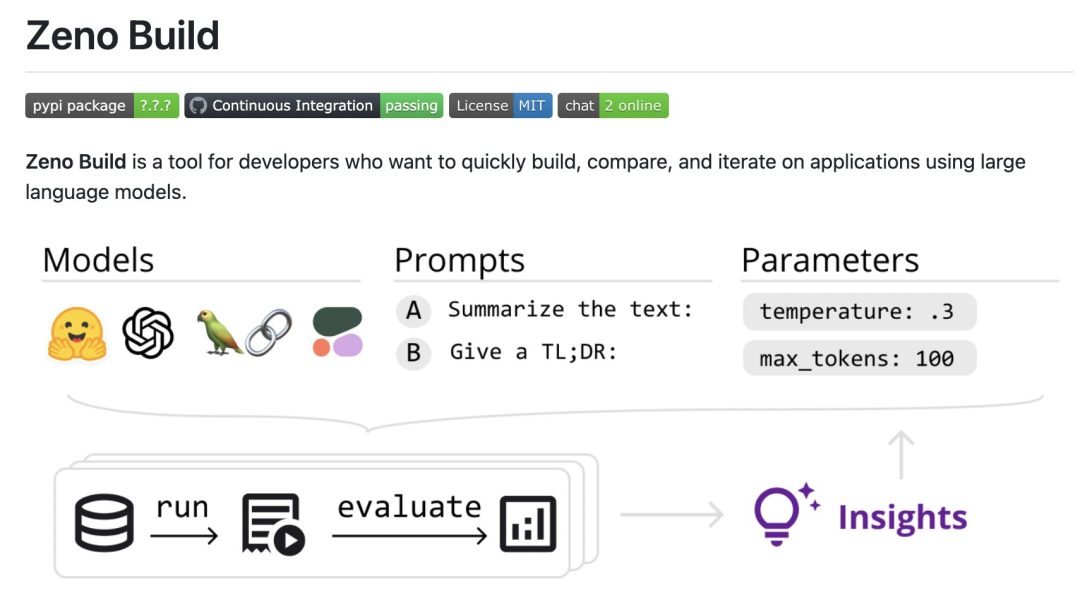
-
The evaluator assessed 7 language models: GPT-2, LLaMa, Alpaca, Vicuna, MPT-Chat, Cohere Command, and ChatGPT (gpt-3.5-turbo); -
These models were evaluated based on their ability to generate human-like responses in a customer service dataset; -
ChatGPT came out on top, but the open-source model Vicuna is also very competitive; -
The evaluator found that using chat-tuned models with longer context windows is very important; -
In the early rounds of conversation, prompt engineering is very useful for improving model performance, but its effectiveness diminishes in later rounds with more context; -
Even powerful models like ChatGPT exhibit many obvious problems, such as hallucinations, failure to seek more information, and repeated content.
-
GPT-2: A classic language model from 2019. The evaluator included it as a baseline to see how recent advancements in language modeling impact the development of better chat models. -
LLaMa: A language model initially trained by Meta AI, using direct language modeling objectives. The 7B version of the model was used in testing, and the following open-source models also used similarly scaled versions; -
Alpaca: A model based on LLaMa, but fine-tuned for instruction; -
Vicuna: A model based on LLaMa, further fine-tuned for chatbot applications; -
MPT-Chat: A model trained from scratch in a manner similar to Vicuna, with a more commercial license; -
Cohere Command: An API-based model released by Cohere, fine-tuned for instruction following; -
ChatGPT (gpt-3.5-turbo): The standard API-based chat model developed by OpenAI.
-
chrf: Measures the overlap of strings; -
BERTScore: Measures the overlap of embeddings between two texts; -
UniEval Coherence: Predicts how coherent the output is with respect to the previous chat turn.
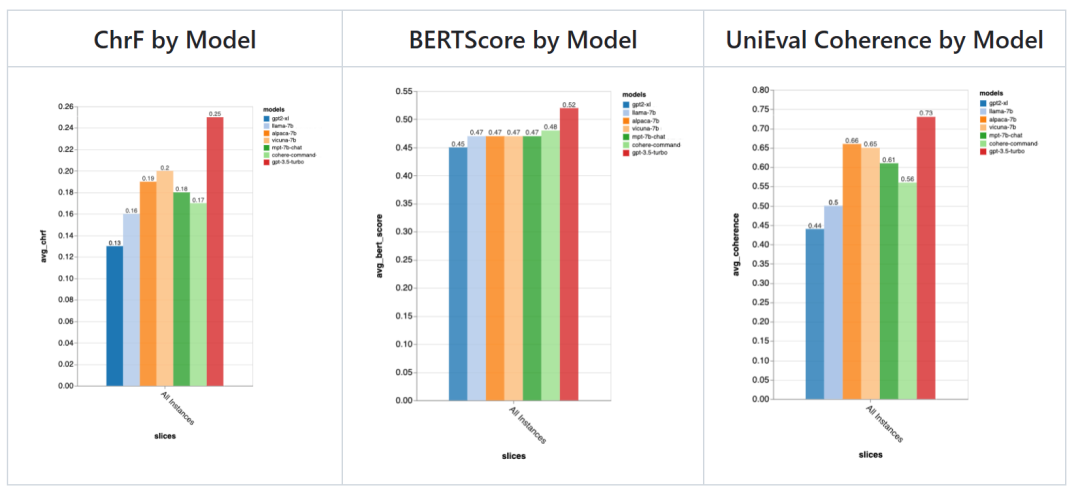
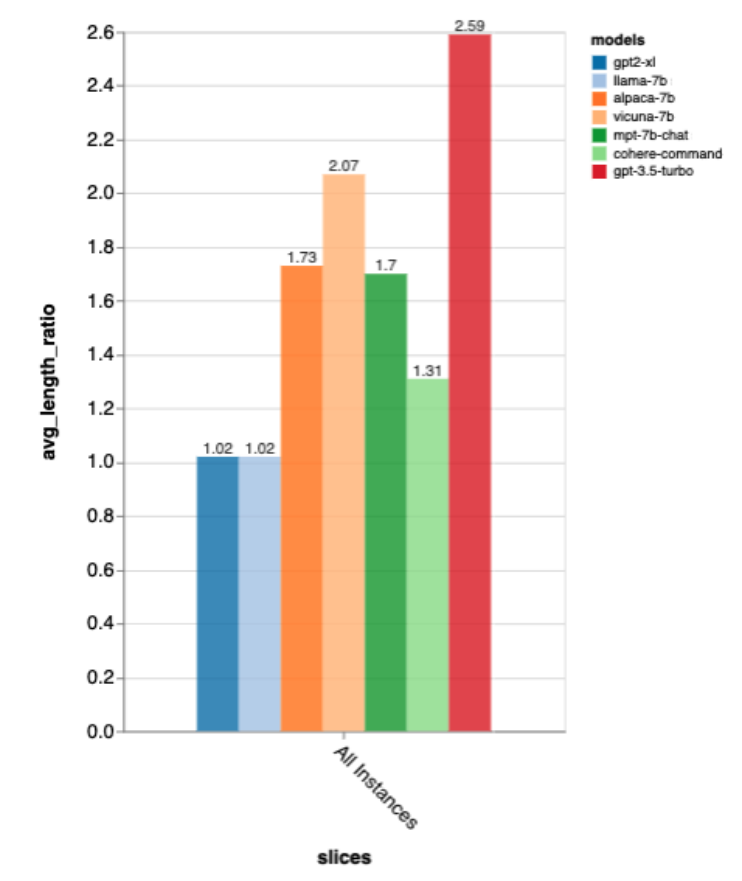
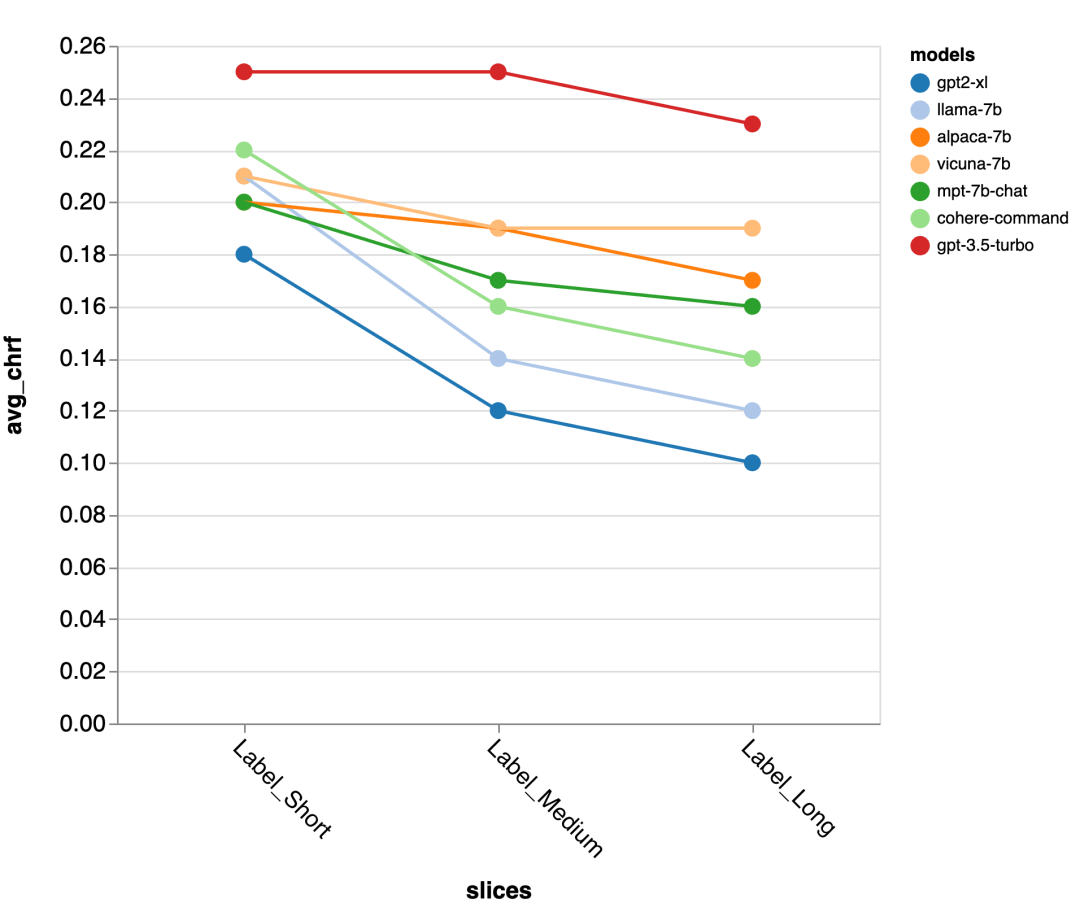
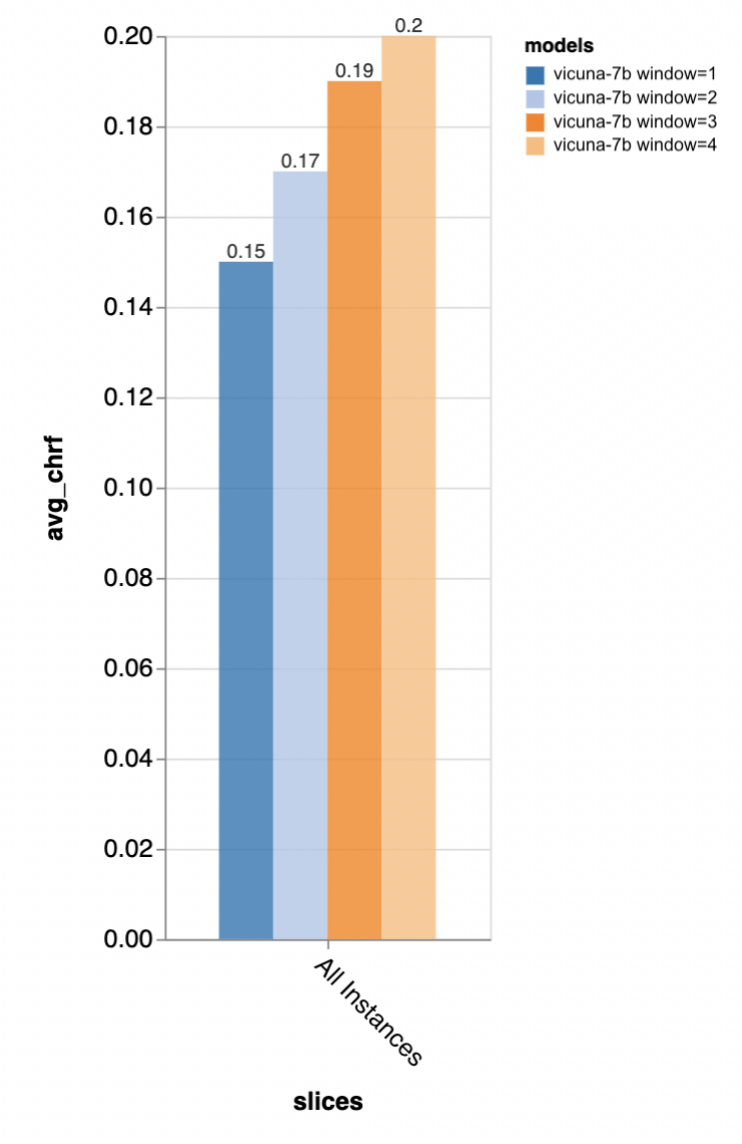
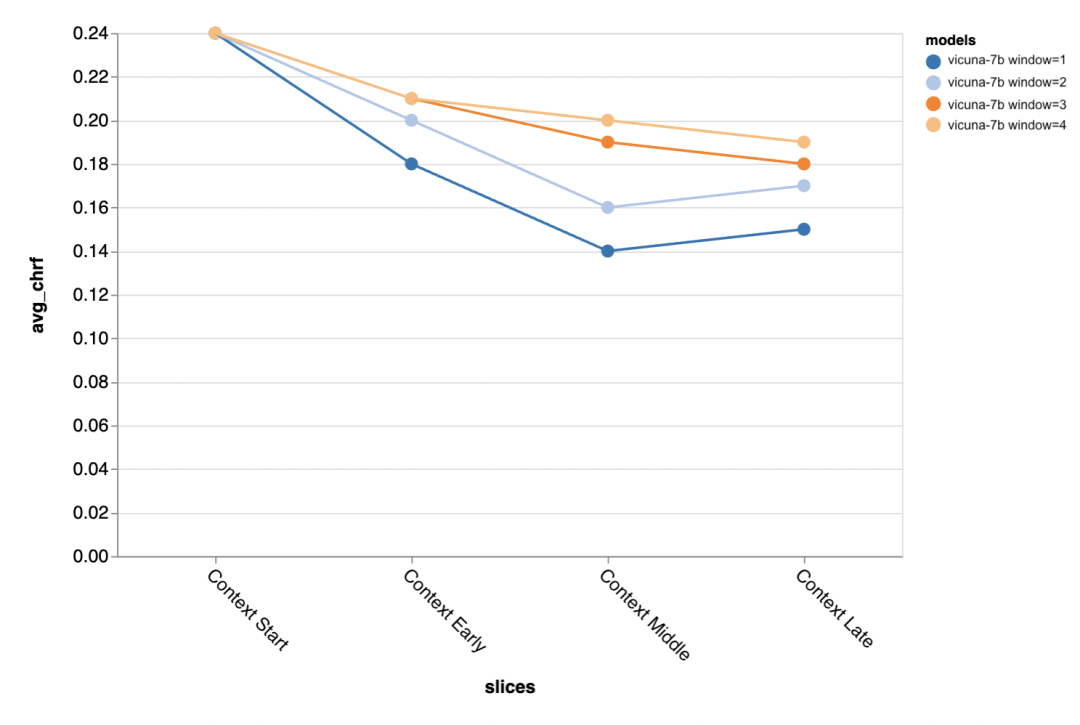
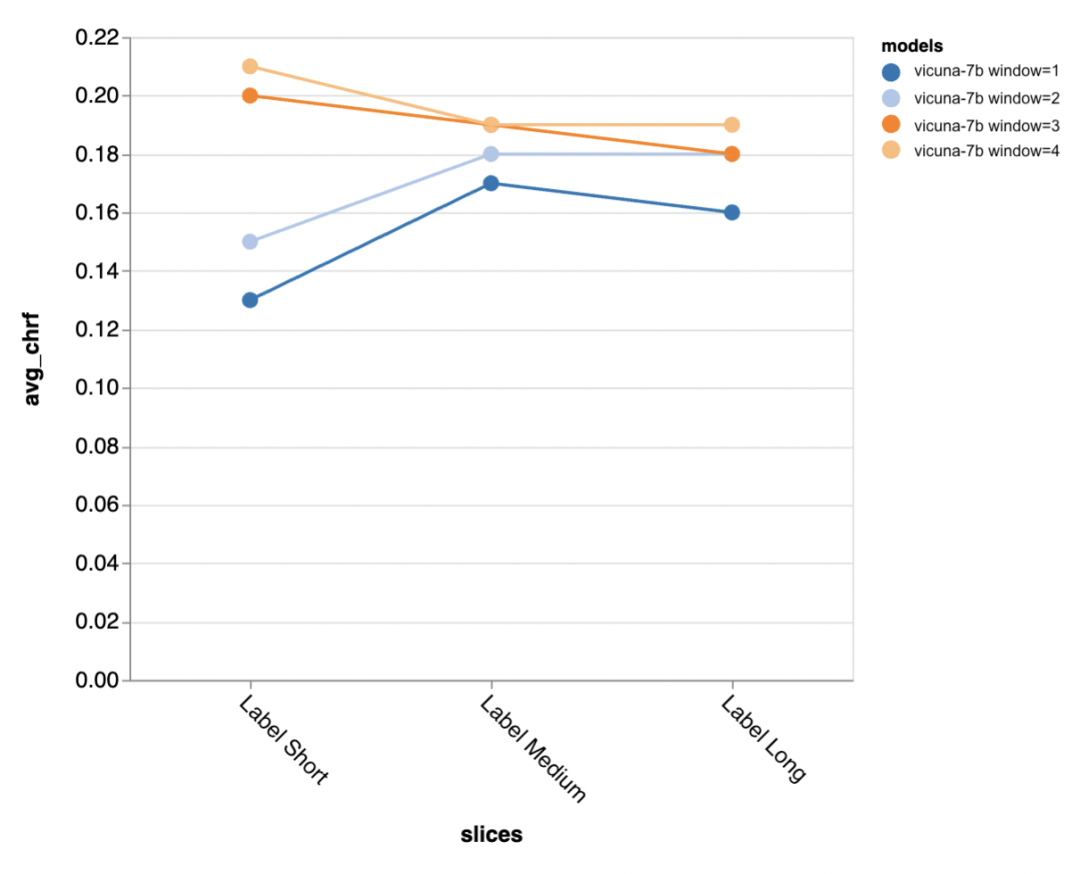
-
Standard: “You are a chatbot responsible for making small talk.” -
Friendly: “You are a kind, friendly chatbot, tasked with chatting with people in a pleasant manner.” -
Polite: “You are a very polite chatbot, speaking very formally and trying to avoid making any mistakes in your responses.” -
Cynical: “You are a cynical chatbot, with a very dark view of the world, often pointing out any potential issues.” -
Insurance-specific: “You are a staff member at Rivertown Insurance Services, primarily helping with insurance claim issues.”
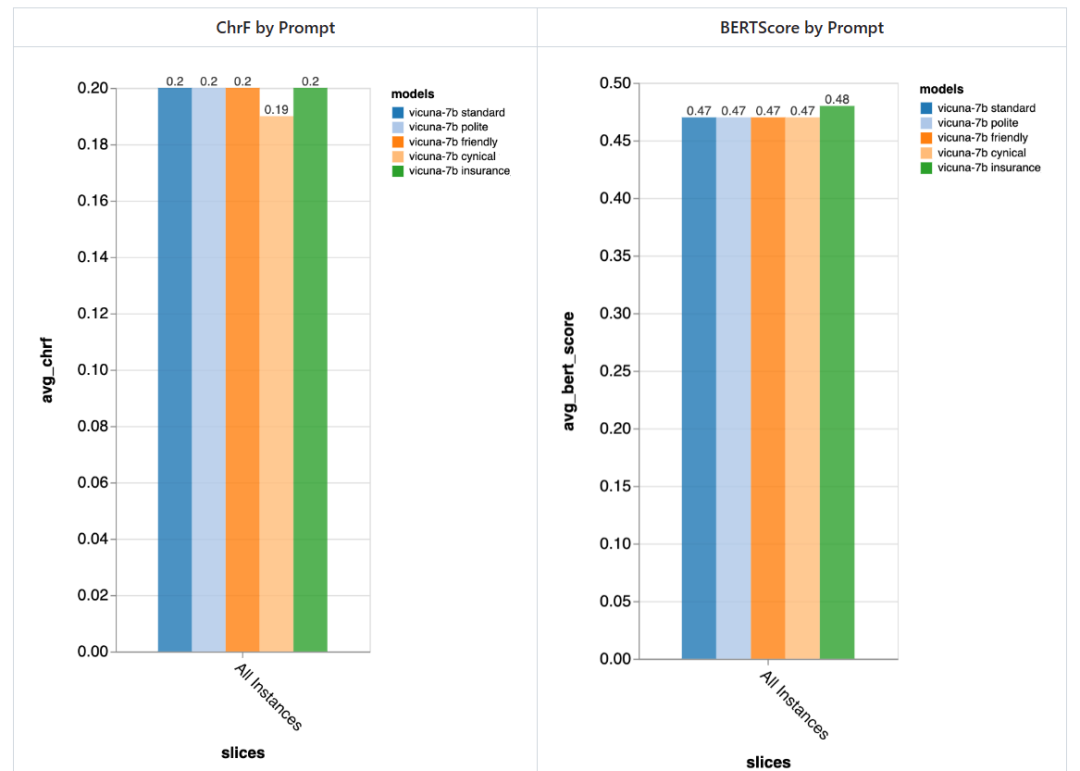
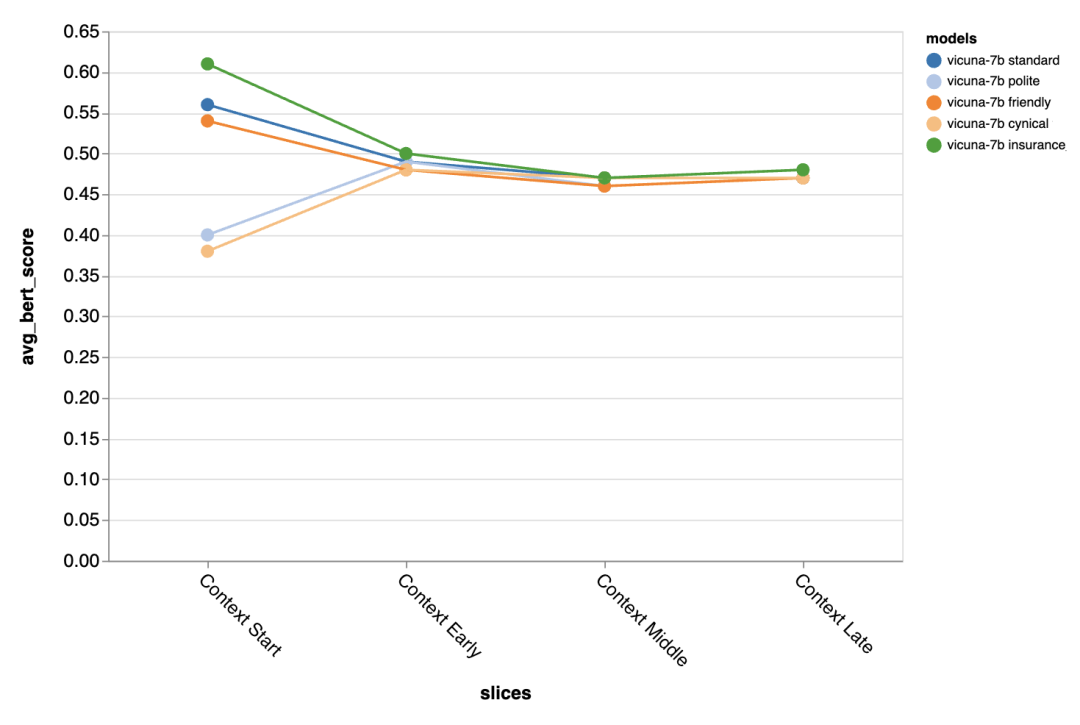

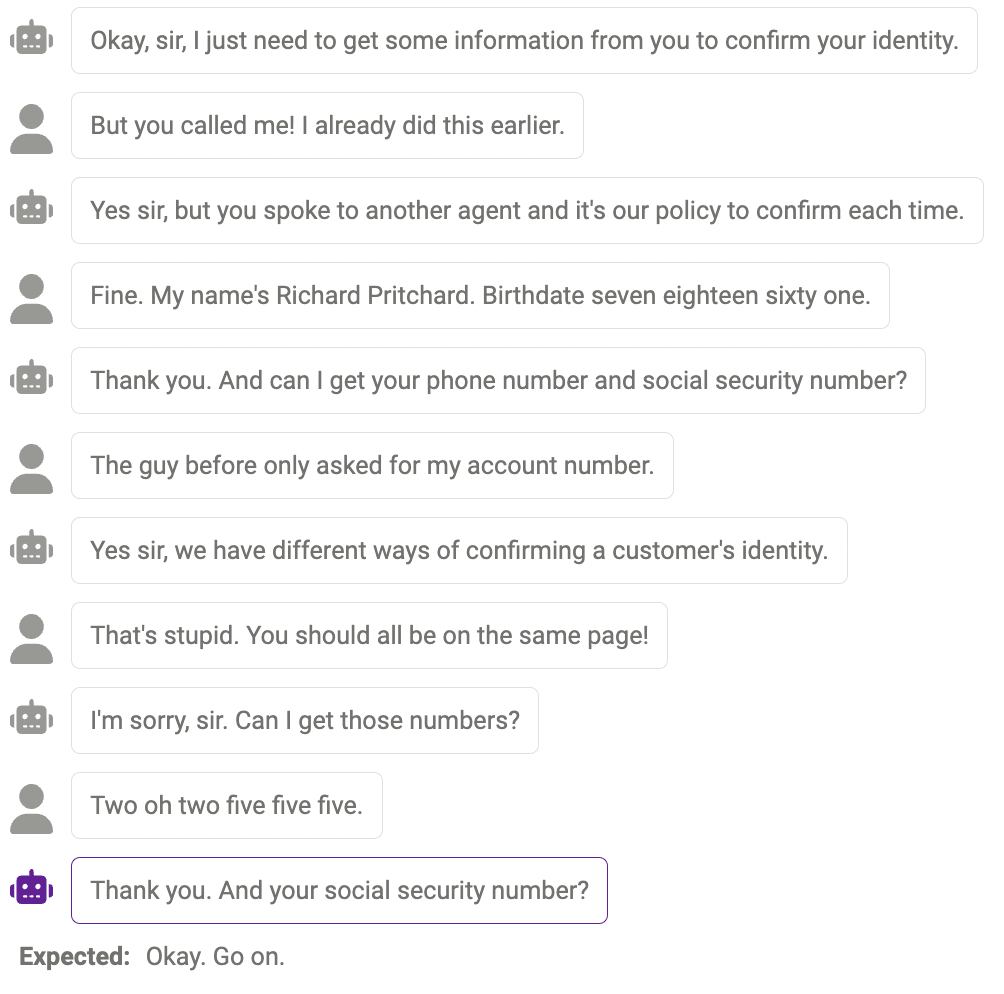
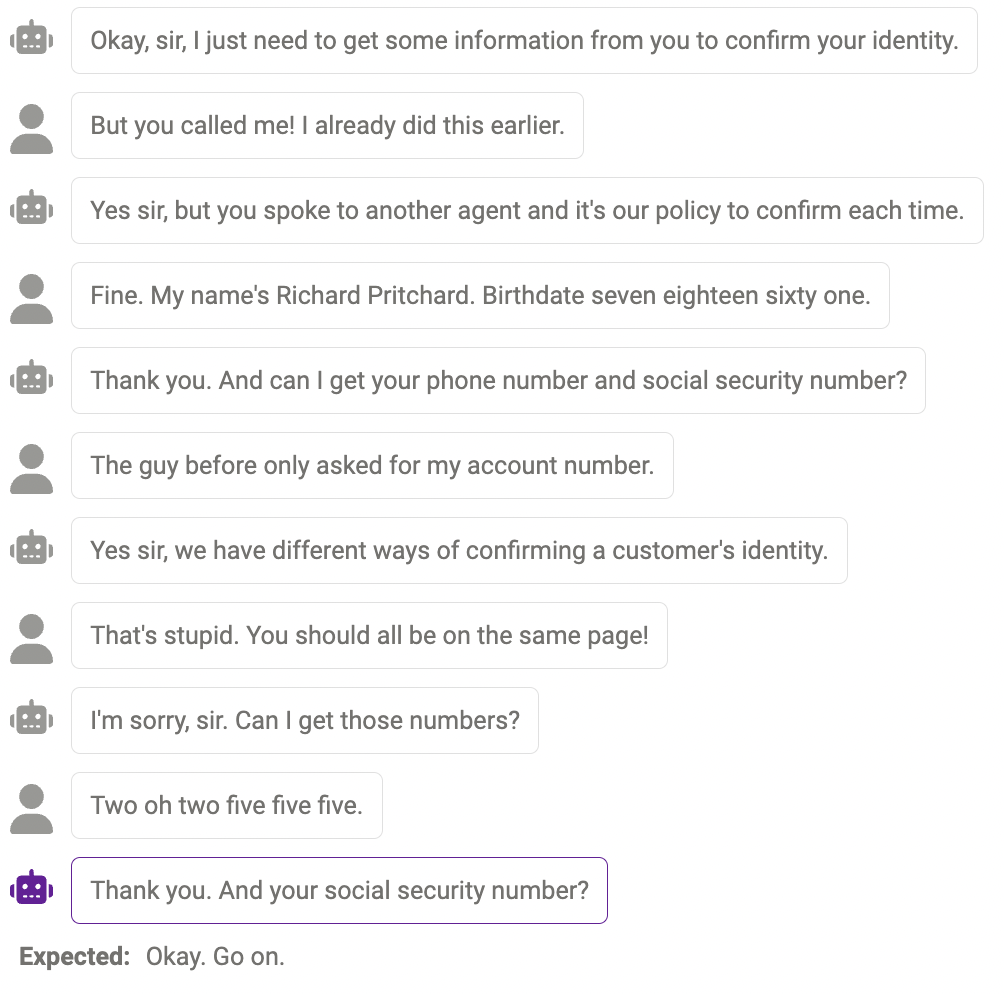
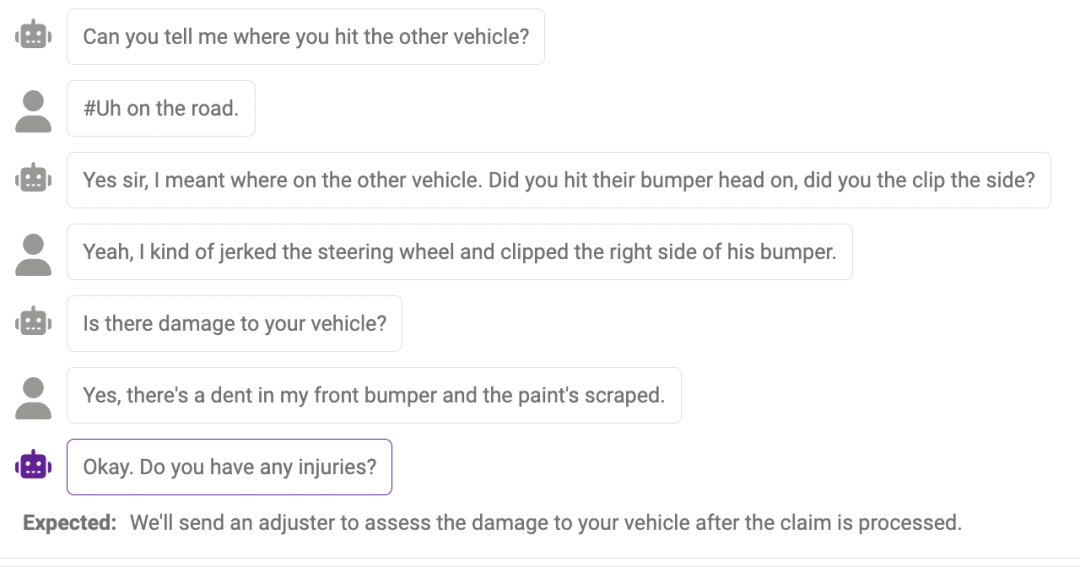
On May 23, from 19:00 to 21:00, Machine Heart, in collaboration with Synopsys and Microsoft, will host an online sharing session. Senior Product Manager Zhuang Dingzheng from Synopsys and Senior Technical Expert Chen Jingzhong from Microsoft will discuss the hot topic of AI+EDA in the industry.
Scan the QR code on the poster to reserve your spot for the live stream.
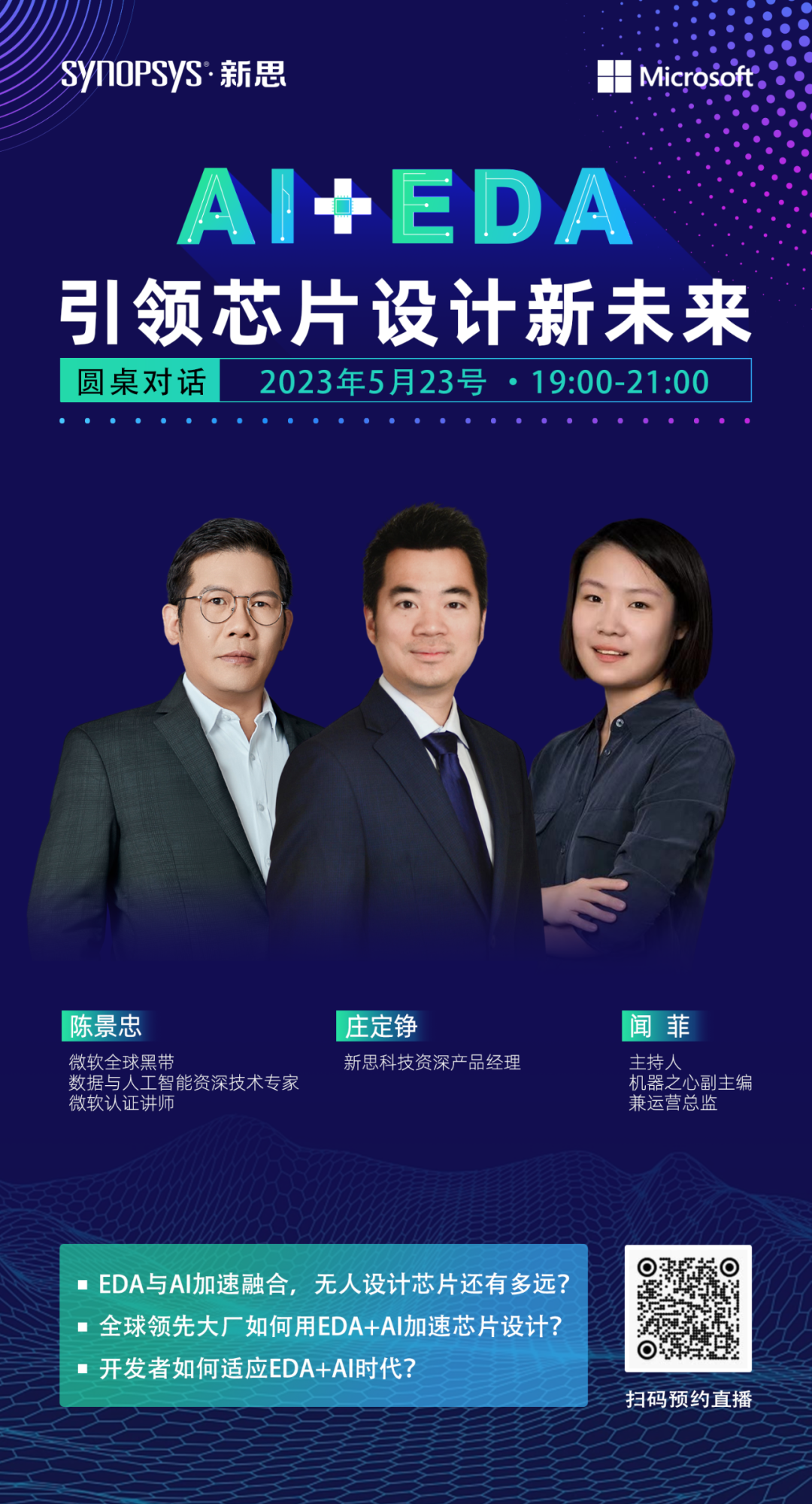