Author: Pulkit Sharma
Translation: Wang Weili
Proofreading: Ding Nanya
This article is about3400 words, recommended reading time is10 minutes.
This article introduces the process of building a deep learning model for image recognition. By stating the problem from an actual competition, introducing the model framework, and showcasing the solution code, it provides beginners with a foundational framework for solving image recognition problems.
Introduction
“Can a deep learning model be built in just a few minutes? Training takes hours, right? I don’t even have a good enough machine.” I have heard aspiring data scientists say this countless times, fearing to build deep learning models on their own machines.
In fact, you don’t have to work at Google or any other large tech company to train deep learning datasets. You can completely build your own neural network from scratch in just a few minutes without renting servers from Google. Students from Fast.ai designed a model for the ImageNet dataset in 18 minutes, and I will demonstrate a similar approach in this article.
Deep learning is a broad field, so we will narrow our focus to the image classification problem. Moreover, we will use a very simple deep learning architecture to achieve good accuracy.
You can use the Python code in this article as a basis for building an image classification model. Once you have a good understanding of these concepts, you can continue programming, participate in competitions, and climb the rankings.
If you are just starting to delve into deep learning and are fascinated by the field of computer vision (who isn’t?!), you should definitely check out the course on Computer Vision using Deep Learning. It provides a comprehensive introduction to this cool field and will lay the foundation for your future entry into this huge job market.
Course Link:
https://trainings.analyticsvidhya.com/courses/course-v1:AnalyticsVidhya+CVDL101+CVDL101_T1/about?utm_source=imageclassarticle&utm_medium=blog
Table of Contents
1. What is Image Classification and Its Use Cases
2. Setting Up Image Data Structure
3. Breakdown of the Model Building Process
4. Setting Problem Definition and Understanding Data
5. Steps to Build an Image Classification Model
6. Start Other Challenges
1. What is Image Classification and Its Use Cases
Observe the following image:
You should be able to recognize it immediately — it’s a luxury car. Step back and analyze how you reached this conclusion — you were shown an image, and then you categorized it as “car” (in this case). In simple terms, this process is image classification.
Often, images can have many categories. Manually checking and classifying images is a very tedious process, especially when the problem scales to 10,000 or even 1,000,000 images; this task becomes nearly impossible. So, how useful would it be if we could automate this process and quickly label image categories?
Self-driving cars are a great example of the real-world application of image classification. To achieve self-driving, we can build an image classification model to recognize various objects on the road, such as vehicles, people, and moving objects. We will see more applications in the following sections, many of which are present around us.
Now that we have grasped the topic, let’s delve into how to build an image classification model, what its prerequisites are, and how to implement it in Python.
2. Setting Up Image Data Structure
Our dataset needs a specific structure to solve the image classification problem. We will see this in several parts, but before moving forward, keep these suggestions in mind.
You should create two folders: one for the training set and another for the test set. The training set folder should contain a CSV file and an image folder:
-
The CSV file stores the names of all training images and their corresponding true labels.
-
The image folder stores all the training images.
The CSV file in the test set folder is different from the one in the training set folder; it only contains the names of the test images without their true labels. This is because we want to predict the images in the test set based on the training images.
If your dataset is not in this format, you need to convert it; otherwise, the prediction results may be incorrect.
3. Breakdown of the Model Building Process
Before we study the Python code, let’s first understand how image classification models are typically designed. The process can be divided into four parts. Each step takes a certain amount of time to execute:
Step One: Load and preprocess data — 30% time
Step Two: Define model architecture — 10% time
Step Three: Train model — 50% time
Step Four: Evaluate model performance — 10% time
Next, I will explain each of the above steps in more detail. This part is very important because not all models are built in the first step. You need to return after each iteration, fine-tune the steps, and run it again. Having a solid understanding of the fundamental concepts will greatly help accelerate the entire process.
-
Step One: Load and Preprocess Data
Data is crucial for deep learning models. If there are a large number of images in the training set, your image classification model will also have a greater chance of achieving better classification results. Additionally, depending on the framework used, the dimensions of the data may vary, affecting the results.
Therefore, for this critical data preprocessing step, I recommend browsing the following article for a better understanding of image data preprocessing:
Basics of Image Processing in Python
https://www.analyticsvidhya.com/blog/2014/12/image-processing-python-basics/)
But we are not fully at the data preprocessing step yet; to understand how our data performs on previously unseen datasets (before predicting the test set), we need to first split a portion of the training set into a validation set.
In short, we train the model on the training set and validate it on the validation set. If we are satisfied with the results on the validation set, we can use it to predict the data in the test set.
Time Required: Approximately 2-3 minutes.
-
Step Two: Build Model Framework
This is another important step in the process of building a deep learning model. During this process, several questions need to be considered:
-
How many convolutional layers are needed?
-
What is the activation function for each layer?
-
How many hidden units are there in each layer?
There are other questions as well. But these are basically the hyperparameters of the model, which play a crucial role in the prediction results.
How to determine the values of these hyperparameters? Good question! One method is to choose these values based on existing research. Another idea is to try these values continuously until the best ones are found, but this can be a very time-consuming process.
Time Required: Approximately 1 minute to define this framework.
-
Step Three: Train Model
For model training, we need:
-
Training images and their true labels.
-
Validation set images and their true labels. (We only use the validation set labels for model evaluation, not for training)
We also need to define the number of iterations (epochs). In the initial phase, we train for 10 epochs (you can change this).
Time Required: Approximately 5 minutes to learn the structure of the model.
-
Step Four: Evaluate Model Performance
Finally, we load the test data (images) and complete the preprocessing steps. Then we use the trained model to predict the categories of these images.
Time Required:1 minute
4. Setting Problem Definition and Understanding Data
We will attempt a very cool challenge to understand image classification. We need to build a model that can classify given images (shirts, pants, shoes, socks, etc.). This is actually a problem faced by many e-commerce retailers, making it a more interesting computer vision problem.
This challenge is called “Identify Apparel,” one of the practical problems we encountered on the data hack platform. You must register and download the dataset from the link above.
“Identify Apparel” Competition Link:
https://datahack.analyticsvidhya.com/contest/practice-problem-identify-the-apparels/)
Data Hack Platform:
https://datahack.analyticsvidhya.com/
There are a total of 70,000 images (28×28 dimensions), with 60,000 from the training set and 10,000 from the test set. The training images have already been labeled with clothing categories, totaling 10 categories. The test set has no labels. This competition is to identify the images in the test set.
We will build the model in Google Colab since it offers free GPU.
Google Colab:
https://colab.research.google.com/
5. Steps to Build an Image Classification Model
Now it’s time to showcase your Python skills; we have finally reached the execution phase!
The main steps are as follows:
Set up Google Colab
Import libraries
Import data preprocessing data (3 minutes)
Set up validation set
Define model structure (1 minute)
Train model (5 minutes)
Prediction (1 minute)
Below are detailed descriptions of the above steps.
-
Step 1: Set Up Google Colab
Since we will import data from a Google Drive link, we need to add a few lines of code in the Google Colab notebook. Create a new Python3 notebook and write the following code:
!pip install PyDrive
This step installs PyDrive. Next, import the required libraries:
import os
from pydrive.auth import GoogleAuth
from pydrive.drive import GoogleDrive
from google.colab import auth
from oauth2client.client import GoogleCredentials
Next, create a drive variable to access Google Drive:
auth.authenticate_user()
gauth = GoogleAuth()
gauth.credentials = GoogleCredentials.get_application_default()
drive = GoogleDrive(gauth)
You need to use the Google Drive file ID to download the dataset:
download = drive.CreateFile({'id': '1BZOv422XJvxFUnGh-0xVeSvgFgqVY45q'})
Replace the ID part with your folder’s ID. Next, download the folder and unzip it.
download.GetContentFile('train_LbELtWX.zip')
!unzip train_LbELtWX.zip
You need to run the above code every time you start the notebook.
-
Step 2: Import Required Libraries for the Model.
import keras
from keras.models import Sequential
from keras.layers import Dense, Dropout, Flatten
from keras.layers import Conv2D, MaxPooling2D
from keras.utils import to_categorical
from keras.preprocessing import image
import numpy as np
import pandas as pd
import matplotlib.pyplot as plt
from sklearn.model_selection import train_test_split
from keras.utils import to_categorical
from tqdm import tqdm
-
Step 3: Next is Data Import and Data Preprocessing.
train = pd.read_csv('train.csv')
Next, we will read the training set, store it as a list, and eventually convert it to a numpy array.
# We have grayscale images, so while loading the images we will keep grayscale=True, if you have RGB images, you should set grayscale as False
train_image = []
for i in tqdm(range(train.shape[0])):
img = image.load_img('train/'+train['id'][i].astype('str')+'.png', target_size=(28,28,1), grayscale=True)
img = image.img_to_array(img)
img = img/255
train_image.append(img)
X = np.array(train_image)
This is a multi-class problem (10 categories), so we need to one-hot encode the label variable.
y=train['label'].values
y = to_categorical(y)
-
Step 4: Split Validation Set from Training Set
X_train, X_test, y_train, y_test = train_test_split(X, y, random_state=42, test_size=0.2)
-
Step 5: Define Model Structure
We will establish a simple structure with 2 convolutional layers, one hidden layer, and one output layer.
model = Sequential()
model.add(Conv2D(32, kernel_size=(3, 3),activation='relu',input_shape=(28,28,1)))
model.add(Conv2D(64, (3, 3), activation='relu'))
model.add(MaxPooling2D(pool_size=(2, 2)))
model.add(Dropout(0.25))
model.add(Flatten())
model.add(Dense(128, activation='relu'))
model.add(Dropout(0.5))
model.add(Dense(10, activation='softmax'))
Next, compile the model.
model.compile(loss='categorical_crossentropy',optimizer='Adam',metrics=['accuracy'])
-
Step 6: Train the Model
In this step, we will train the training set data and validate it on the validation set.
model.fit(X_train, y_train, epochs=10, validation_data=(X_test, y_test))
-
Step 7: Prediction!
We will first follow the steps taken when processing the training dataset. Load the test images and predict the classification results using the model.predict_classes() function to predict their classes.
download = drive.CreateFile({'id': '1KuyWGFEpj7Fr2DgBsW8qsWvjqEzfoJBY'})
download.GetContentFile('test_ScVgIM0.zip')
!unzip test_ScVgIM0.zip
First, import the test set:
test = pd.read_csv('test.csv')
Next, read in the data and store the test set:
test_image = []
for i in tqdm(range(test.shape[0])):
img = image.load_img('test/'+test['id'][i].astype('str')+'.png', target_size=(28,28,1), grayscale=True)
img = image.img_to_array(img)
img = img/255
test_image.append(img)
test = np.array(test_image)
# making predictions
prediction = model.predict_classes(test)
You also need to create a submission folder to upload to the DataHack platform.
download = drive.CreateFile({'id': '1z4QXy7WravpSj-S4Cs9Fk8ZNaX-qh5HF'})
download.GetContentFile('sample_submission_I5njJSF.csv')
# creating submission file
sample = pd.read_csv('sample_submission_I5njJSF.csv')
sample['label'] = prediction
sample.to_csv('sample_cnn.csv', header=True, index=False)
Download the sample_cnn.csv file and upload it to the competition page to generate your ranking. This provides a foundational solution to help you start solving image classification problems.
You can try adjusting hyperparameters and regularization to improve model performance. You can also understand the details of tuning parameters by reading the article below.
A Comprehensive Tutorial to Learn Convolutional Neural Networks from Scratch
https://www.analyticsvidhya.com/blog/2018/12/guide-convolutional-neural-network-cnn/
6. Start a New Challenge
Let’s try testing on other datasets. In this part, we will solve the problem on Identify the Digits.
Identify the Digits Competition Link:
https://datahack.analyticsvidhya.com/contest/practice-problem-identify-the-digits/
Before you scroll down, try to solve this challenge yourself. You have gained the tools to solve the problem; you just need to use them. If you encounter difficulties, you can come back to check your process and results.
In this challenge, we need to recognize the digits in the given images. There are a total of 70,000 images, with 49,000 training images labeled and the remaining 21,000 test images unlabeled.
Are you ready? Great! Open a new Python3 notebook and run the code below:
# Setting up Colab
!pip install PyDrive
import os
from pydrive.auth import GoogleAuth
from pydrive.drive import GoogleDrive
from google.colab import auth
from oauth2client.client import GoogleCredentials
auth.authenticate_user()
gauth = GoogleAuth()
gauth.credentials = GoogleCredentials.get_application_default()
drive = GoogleDrive(gauth)
# Replace the id and filename in the below codes
download = drive.CreateFile({'id': '1ZCzHDAfwgLdQke_GNnHp_4OheRRtNPs-'})
download.GetContentFile('Train_UQcUa52.zip')
!unzip Train_UQcUa52.zip
# Importing libraries
import keras
from keras.models import Sequential
from keras.layers import Dense, Dropout, Flatten
from keras.layers import Conv2D, MaxPooling2D
from keras.utils import to_categorical
from keras.preprocessing import image
import numpy as np
import pandas as pd
import matplotlib.pyplot as plt
from sklearn.model_selection import train_test_split
from keras.utils import to_categorical
from tqdm import tqdm
train = pd.read_csv('train.csv')
# Reading the training images
train_image = []
for i in tqdm(range(train.shape[0])):
img = image.load_img('Images/train/'+train['filename'][i], target_size=(28,28,1), grayscale=True)
img = image.img_to_array(img)
img = img/255
train_image.append(img)
X = np.array(train_image)
# Creating the target variable
y=train['label'].values
y = to_categorical(y)
# Creating validation set
X_train, X_test, y_train, y_test = train_test_split(X, y, random_state=42, test_size=0.2)
# Define the model structure
model = Sequential()
model.add(Conv2D(32, kernel_size=(3, 3),activation='relu',input_shape=(28,28,1)))
model.add(Conv2D(64, (3, 3), activation='relu'))
model.add(MaxPooling2D(pool_size=(2, 2)))
model.add(Dropout(0.25))
model.add(Flatten())
model.add(Dense(128, activation='relu'))
model.add(Dropout(0.5))
model.add(Dense(10, activation='softmax'))
# Compile the model
model.compile(loss='categorical_crossentropy',optimizer='Adam',metrics=['accuracy'])
# Training the model
model.fit(X_train, y_train, epochs=10, validation_data=(X_test, y_test))
download = drive.CreateFile({'id': '1zHJR6yiI06ao-UAh_LXZQRIOzBO3sNDq'})
download.GetContentFile('Test_fCbTej3.csv')
test_file = pd.read_csv('Test_fCbTej3.csv')
test_image = []
for i in tqdm(range(test_file.shape[0])):
img = image.load_img('Images/test/'+test_file['filename'][i], target_size=(28,28,1), grayscale=True)
img = image.img_to_array(img)
img = img/255
test_image.append(img)
test = np.array(test_image)
prediction = model.predict_classes(test)
download = drive.CreateFile({'id': '1nRz5bD7ReGrdinpdFcHVIEyjqtPGPyHx'})
download.GetContentFile('Sample_Submission_lxuyBuB.csv')
sample = pd.read_csv('Sample_Submission_lxuyBuB.csv')
sample['filename'] = test_file['filename']
sample['label'] = prediction
sample.to_csv('sample.csv', header=True, index=False)
Submit this file on the practice problem page, and you will achieve a pretty good accuracy. This is a good start, but there is always room for improvement. Keep going and see if you can enhance our basic model.
Conclusion
Who says deep learning models need hours or days of training? My goal is to demonstrate that you can come up with a pretty decent deep learning model in double-quick time. You should accept similar challenges and try coding them from your terminal. Nothing beats learning through practice!
Top data scientists and analysts even prepare these codes before the hackathon starts. They use these codes to submit early before diving into detailed analysis. First, provide a baseline solution, then improve the model using different techniques.
Did you find this article useful? Please share your feedback in the comments section below.
Original Title:
Build your First Image Classification Model in just 10 Minutes!
Original Link:
https://www.analyticsvidhya.com/blog/2019/01/build-image-classification-model-10-minutes/
Edited by: Huang Jiyan
Translator’s Profile
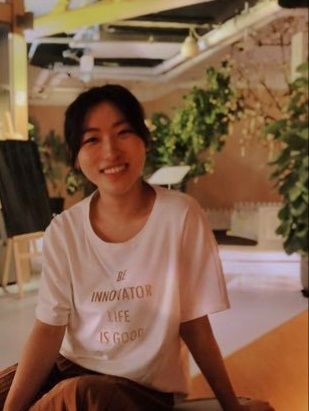
Wang Weili, job seeker, studying big data technology at the Hong Kong University of Science and Technology. I find data science challenging yet interesting, and I am still learning. I collaborate with data enthusiasts to tackle literature that one person cannot handle alone.
Recruitment Information for Translation Group
Job Content: Requires meticulous attention to detail to translate selected foreign articles into fluent Chinese. If you are an international student in data science/statistics/computer science or work in related fields, or if you are confident in your language skills, you are welcome to join the translation team.
What You Will Gain: Regular translation training to improve volunteers’ translation skills, enhance understanding of cutting-edge data science, and connect overseas friends with domestic technology applications. The THU Data Group’s industry-academia-research background offers good development opportunities for volunteers.
Other Benefits: You will have the opportunity to work with data scientists from well-known companies and students from prestigious universities such as Peking University, Tsinghua University, and others.
Click on “Read the Original” at the end to join the Data Group~
Reprint Notice
If you need to reprint, please indicate the author and source prominently at the beginning (Reprinted from: Data Group ID: datapi), and place a prominent QR code for Data Group at the end of the article. For articles with original identification, please send [Article Name – Authorized Public Account Name and ID] to the contact email to apply for whitelist authorization and edit as required.
After publication, please provide the link to the contact email (see below). Unauthorized reprints and adaptations will be pursued legally.
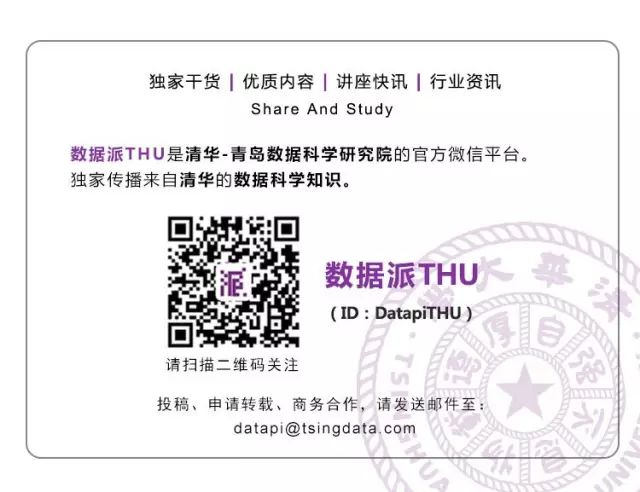
Click on “Read the Original” to embrace the organization