New quality productivity is driven by the deep application of new technologies, thereby constructing a new type of social production relationship and system. In the 2024 government work report, the development of new quality productivity ranks first among the government’s ten major tasks. As an important driving force for new quality productivity, the accelerated development of a new generation of artificial intelligence is of great significance for forming new quality productivity. As an important driving force for a new round of technological revolution and industrial transformation, artificial intelligence is a new technological science that studies and develops theories, methods, technologies, and application systems for simulating, extending, and enhancing human intelligence.
With OpenAI introducing reinforcement learning techniques based on human feedback, the model output of artificial intelligence has become consistent with human common sense, cognition, needs, values, and more. Since then, large language models (LLMs) have entered the public’s view. Large language models refer to pre-trained language models with a considerable scale (containing hundreds or thousands of billions of parameters). They learn from vast, multi-type scenario data, summarizing general capabilities across different scenarios, learning characteristics and patterns, and becoming a model library with strong generalization capabilities. LLMs have advantages such as naturalness, generalization ability, and efficiency. In today’s era of LLMs, AI-assisted decision-making and enterprise management have become an inevitable trend.
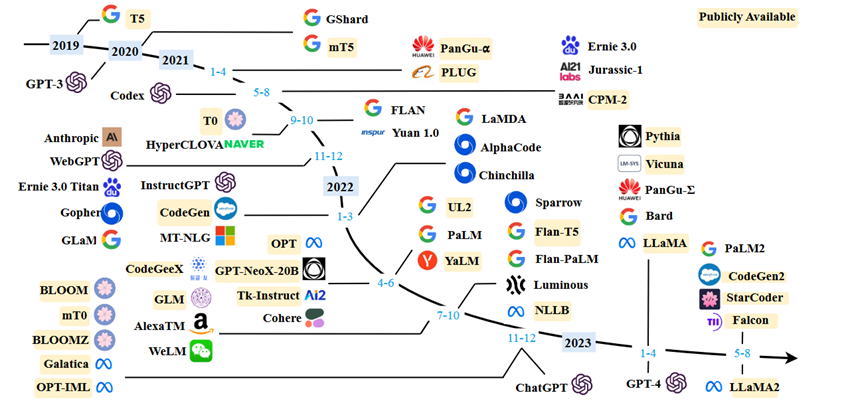
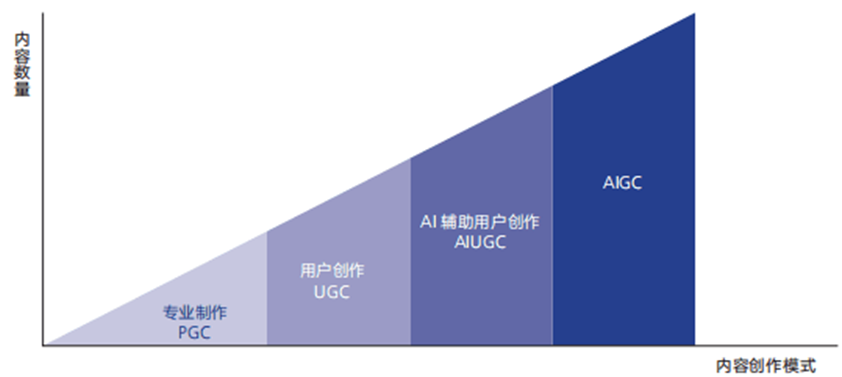
Currently, the AIGC industry in China has developed two main types of business formats.
The first is aimed at C-end users, providing products that can be categorized by modality into text generation, image generation, audio-video generation, and other diverse content forms.
The second is aimed at B-end enterprise clients, providing specialized services based on specific fields.
Currently, the most populated tracks include gaming, media/film, e-commerce, and advertising marketing, all heavy content tracks. In the future, AIGC is expected to continue to extend the industrial chain and deepen its commercialization scenarios, profoundly transforming various industries.
Focusing on the financial industry, the application of AIGC is divided into front office, middle office, and back office, covering marketing, investment research projects, product design, risk control compliance, customer service, and operational management, all supported by underlying artificial intelligence technology. The front office marketing and middle office departments have widespread applications, with the front office marketing department utilizing AIGC for intelligent writing and recommendations. The middle office can utilize AIGC to create risk control and customer service platforms, with commercial products already being quite mature. The front office investment research involves more complex modeling and due diligence work, but currently, generative AI products are primarily open-source large models, with low commercialization levels, and AIGC mainly undertakes auxiliary work in the short term.
Figure 4: Application Scenarios of AIGC in the Financial Industry
Source: “2023 AIGC Scenario Application Outlook Research Report”, iResearch Consulting
Meanwhile, another key term in this article, ESG, refers to Environmental (Environment), Social (Social), and Governance (Governance) factors. The ESG concept was first clearly proposed in the UN Global Compact initiative in 2004, representing a series of investment philosophies and corporate evaluation standards measuring corporate environmental, social, and governance performance rather than financial performance. As expectations for sustainability and social responsibility from enterprises continue to rise, ESG has become a key framework for assessing and defining these efforts. AIGC, with its cutting-edge advances in data processing and pattern recognition, provides innovative pathways for addressing ESG challenges. From the strong future application potential of AIGC in the ESG field, it not only provides enterprises with a tool but also represents a disruptive innovation to traditional corporate philosophy.
Figure 5: Application Scenarios of AIGC in Corporate Governance
Source: iHR Integrated HR System
The core technology of artificial intelligence applications in the ESG field is Retrieval-Augmented Generation (RAG), which provides external knowledge sources for large models, enabling them to generate accurate and contextually relevant answers while reducing model hallucinations. This can be decomposed into three main processes: Indexing – Retrieval – Generation.
Indexing: After document cleansing, documents are converted into a unified plain text format and segmented into more manageable chunks based on language model context limitations. These chunks are encoded into vector representations using embedding models and stored in a vector database.
Retrieval: Using the same embedding model, queries are converted into vector representations, and cosine similarity calculates similarity scores between the query vector and chunk vectors in the indexed corpus, prioritizing the retrieval of the top K chunks most relevant to the query to expand context.
Generation: The user’s query and selected documents are synthesized into a coherent prompt using a template, and the large model generates a response based on this prompt.
From the three dimensions of ESG, in the Environmental (E) dimension, climate science large models have emerged that combine reports from the Intergovernmental Panel on Climate Change (IPCC). They fundamentally solve issues of large model hallucinations and outdated information, creating an expert system in the climate field called Chat Climate. The three types of Chat Climate include:
Hybrid Chat Climate: Provides answers based on given information using its own knowledge.
Chat Climate: The chatbot provides answers solely based on IPCC AR6.
GPT-4: The prompt provides no additional information or instructions on how to provide answers, representing baseline behavior.
At the same time, the Kaiwu large language model launched by Xu Ming’s team at Tsinghua University’s School of Environment on March 17, 2023, is the world’s first large language model in the environmental field that combines existing human knowledge in sustainable development.
Figure 6: Technical Principles of Chat Climate
Source: Vaghefi S A, Stammbach D, Muccione V, et al. ChatClimate: Grounding conversational AI in climate science[J]. Communications Earth & Environment, 2023, 4(1): 480.
In the Social (S) dimension, in April 2024, Huazhong University of Science and Technology explored the role of AI Agents in supply chain management, such as optimizing communication, automating processes, and enhancing decision support systems, helping enterprises better understand market demands, risk factors, and supply chain bottlenecks. It emphasized three core components: model infrastructure, lifecycle management, and downstream application ecosystem:
Infrastructure: This is the foundational layer of the supply chain, containing the necessary datasets and toolchains that support the efficient training, optimization, and deployment of LLMs.
Model Lifecycle: The entire lifecycle of LLMs, from development training to deployment and maintenance. It encompasses a series of processes that LLMs undergo, including the development and application using training datasets, iterative cycles of testing and evaluation, and the ongoing updates and maintenance needed to ensure the model remains effective and safe over time.
Downstream Application Ecosystem: Applications and services leveraging LLM capabilities. This includes domain-specific models (DSM) that adapt general LLM capabilities to specific needs.
Figure 7: Role of AI Agent in Supply Chain Management
Source: Wang S, Zhao Y, Hou X, et al. Large Language Model Supply Chain: A Research Agenda[J]. arxiv preprint arxiv, 2024: 2404.12736.
In the Corporate Governance (G) dimension, the iHR integrated HR system shown in Figure 5 is a successful application result. Additionally, in a broader governance context, in March 2024, South China University of Technology proposed the AlphaFin framework, which combines traditional research datasets, real-time financial data, and handwritten thought chain (CoT) data to train large language models. The AlphaFin dataset benchmarks a method known as Stock-Chain for effectively handling financial analysis tasks, significantly improving the accuracy of stock predictions and the interpretability of results, enabling artificial intelligence to participate in corporate investment decision-making.
Stage 1 Stock Trend Prediction: Demonstrates the annualized return rate and accuracy of the stock prediction system in the actual market.
• Problem Definition: For a set of companies C and related knowledge documents D, the prediction system forecasts stock trends.
• Knowledge Processing: Relevant documents are retrieved for each company, combining prompt templates and document content to form inputs.
• Stock Prediction Fine-Tuning: The stock prediction system (StockGPT) is trained using financial report data from the AlphaFin dataset.
• Prediction and Post-Processing: StockGPT predicts whether stocks will rise or fall based on inputs and extracts specific prediction results from responses.
Stage 2 Financial Q&A: Through user evaluations and machine evaluations, the model’s effectiveness and superiority in handling actual financial Q&A tasks have been demonstrated.
• Vector Database Construction: A vector database is constructed using key knowledge from documents for rapid retrieval.
• Knowledge Retrieval: Relevant knowledge documents are retrieved from the vector database through user queries to assist in generating responses.
• LLMs Fine-Tuning: Further training on the AlphaFin dataset enhances the model’s financial Q&A capabilities based on the first stage.
• Response Generation: Responses are generated based on retrieved knowledge documents, dialogue history, and user queries.
Figure 8: Benchmark Financial Analysis Using Retrieval-Augmented Stock-Chain Framework
Source: Li X, Li Z, Shi C, et al. AlphaFin: Benchmarking Financial Analysis with Retrieval-Augmented Stock-Chain Framework[J]. arXiv preprint arxiv, 2024: 2403.12582.
Finally, looking at ESG as a whole, in November 2023, the project “Research on ESG Text Analysis Tools for Evaluating Corporate Carbon Disclosure Quality Based on Large Language Models,” led by Li Nan’s team at Tsinghua University’s School of Environment, won the SMP (Social Media Processing) – Zhizhu AI Large Model Interdisciplinary Fund, proving that artificial intelligence can indeed participate in relevant ESG practice activities.
In this project, the carbon footprint characterization of corporate product life cycles involves numerous supply chain entities, and data collection and disclosure present challenges, resulting in ESG reports becoming a “black box.” The resulting loss of trust affects consumers, investors, and regulators’ evaluations of companies. Therefore, transparent and detailed carbon disclosure is fundamental to strengthening corporate environmental responsibility, enhancing social recognition, and improving the ESG ecosystem.
To encourage companies to actively disclose carbon emissions and enhance the trust of rating agencies and the public in corporate ESG reports, this project will focus on carbon emissions, systematically constructing a multi-dimensional analysis model for the quality of corporate ESG report carbon disclosures, and providing intelligent analysis tools based on large language models to achieve intelligent analysis of the quality of corporate ESG report carbon disclosures, promoting the deep integration of environmental science, data science, and artificial intelligence.
Figure 9: Benchmark Financial Analysis Using Retrieval-Augmented Stock-Chain Framework
Source: Tsinghua School of Environment Official Website
In the next article, we will delve into the energy crisis faced by AI, and how clean energy can provide sustainable solutions for AI development, and further analyze the potential and challenges of AI technology in the ESG field.
Original Statement
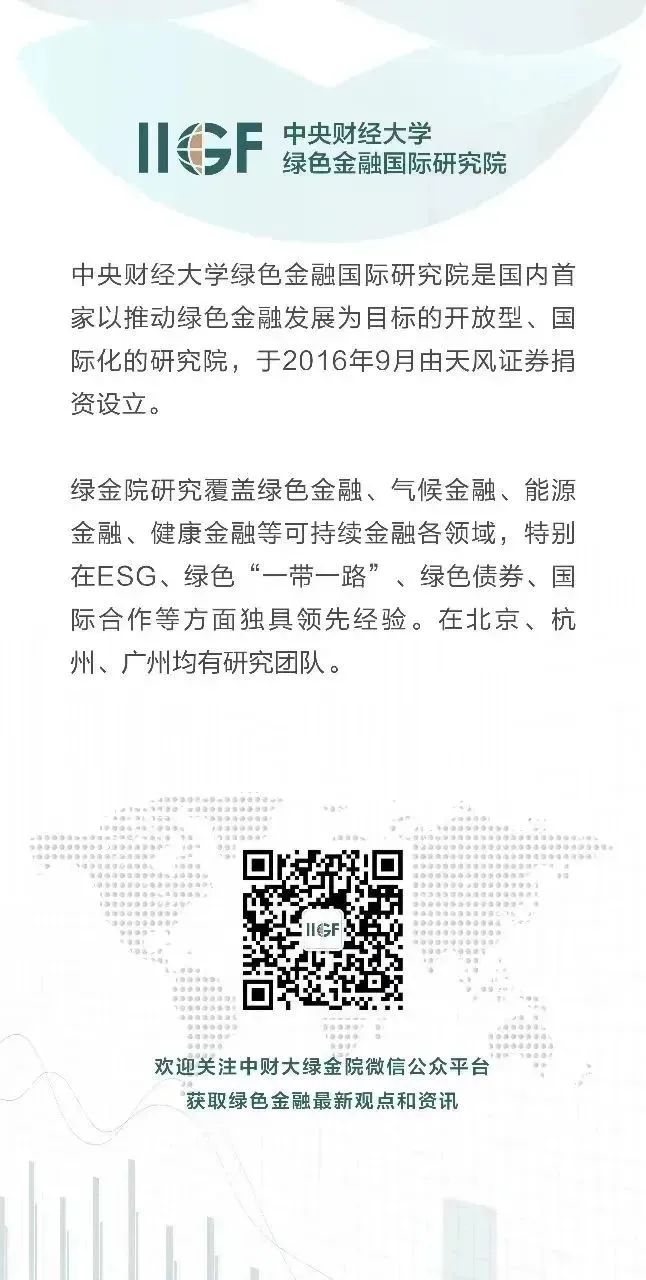