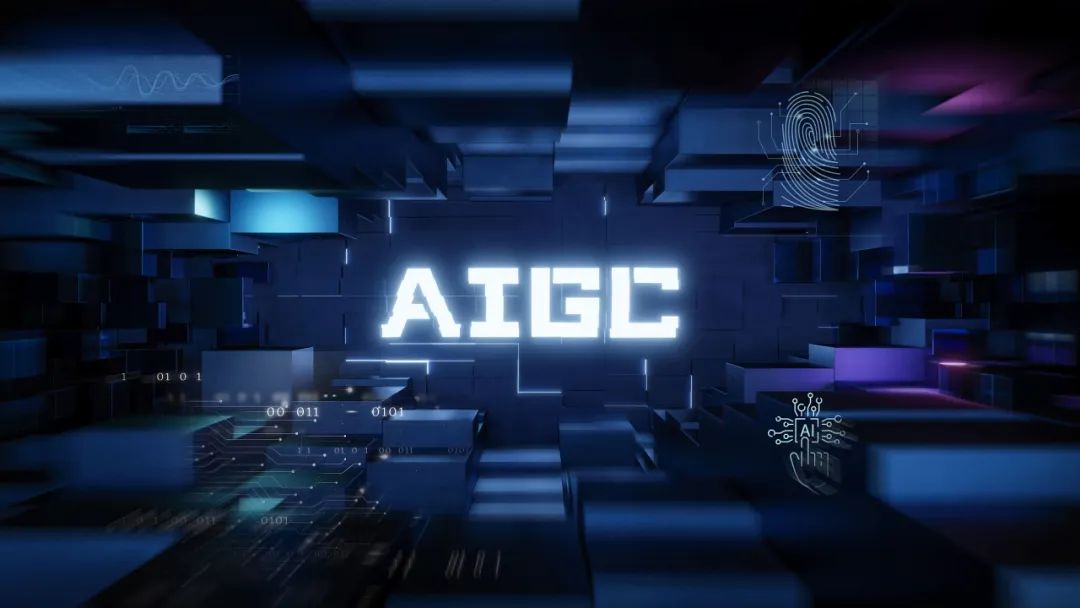
In the context of information and intelligence, AI technology, with its powerful computing and learning capabilities, has brought revolutionary changes to the broadcasting and television and online audiovisual industries. From content creation to dissemination channels, the application of AI technology is gradually penetrating every aspect of the broadcasting and television industry, greatly enhancing production efficiency and enriching user experience.
The application of AIGC in the broadcasting and television and online audiovisual fields presents significant characteristics of a new productive force driven by innovation. Production methods, online dissemination capabilities, interactive effects, and monitoring and regulation methods will undergo systematic changes, giving rise to new labor materials, new labor objects, and new laborers.
Transformation of the Three Elements of Productivity Under AIGC
Productivity is the fundamental driving force for social change, and production materials are an objective measure of the level of productivity development, as well as a material symbol that distinguishes economic eras. According to the “AIGC Economic Impact Report” released by McKinsey in 2023, it is predicted that with the integration of AIGC and industry applications, labor productivity could achieve an annual growth of 0.5% to 3.4% by 2040.
During the iterative upgrades of the internet from Web 1.0, Web 2.0, Web 3.0 to the Metaverse era, complementary content production methods have emerged, and mainstream media have also undergone their own transformations and transitions alongside the changes in the internet, evolving from a traditional broadcasting and television dissemination system to an integrated dissemination system. Content production methods have also experienced a development process from PGC to UGC, and then to AIGC.
The combination of “big data + big models + big computing power” has built the “new foundation” of the new productive force for the development of broadcasting and television and online audiovisual, which will become the content production infrastructure in the Metaverse era. There will be significant changes in technology innovation, industry structure, human resources, and resource utilization in the broadcasting and television and online audiovisual industry.
(1) Data Becomes the New Labor Object
Data, as a new type of production factor, has new characteristics such as replicability, scalability, non-consumability, and marginal costs approaching zero. Compared with traditional broadcasting and television production methods, it has significantly improved production costs, content stability, content richness, and audience experience, providing broader opportunities for high-quality development in broadcasting and television and online audiovisual.
In traditional content production processes, the labor object is mainly the specific material processed by creators through intelligence and skills. The application of AIGC technology has transformed the labor object in the broadcasting and television audiovisual industry from a single manually created material to diversified data resources and intelligent generation processes, making it more data-driven, virtualized, and dynamically flexible.
(2) Large Models Become the New Labor Material
The learning process of AIGC is essentially the training process of models, which involves adjusting variables and optimizing parameters. According to academic experience, the learning ability of deep neural networks is positively correlated with the scale of model parameters, and the learning ability of AIGC depends on the scale of parameters and the amount of data required for training.
Thus, the main changes in production infrastructure have the following characteristics: First, the demand for high-performance computing resources has increased. AIGC technology relies on powerful computing support, and hardware such as data centers, cloud computing platforms, and GPU servers will become indispensable labor materials to efficiently process large-scale data and run complex AI algorithms. Second, the replacement of intelligent creation tools is accelerating. AIGC content generation software, intelligent editing systems, automatic editing software, etc., will become new labor materials that can assist or directly participate in various stages of content creation, editing, and synthesis, with a faster pace of technological iteration. Third, the integration of new technologies is broader. The application of AIGC in fields such as 3D modeling and virtual reality means that the technologies and materials needed for immersive audiovisual experiences have also become important labor materials. Technologies such as natural language processing, computer vision, speech recognition, and synthesis constitute important components of AIGC and are core labor materials in the production process.
(3) New Laborers Emerge
The greatest advantage of AIGC is its logical reasoning ability, which breaks through the linear thinking framework to achieve nonlinear reasoning, describing complex logical relationships through induction, deduction, and analysis.
New laborers have the following obvious characteristics: First, skill structures undergo transformation, and laborers need to master new skills and knowledge, including understanding the basic principles of AIGC technology, familiarizing themselves with the use of AIGC creation tools, and how to effectively collaborate with AIGC, such as training, optimizing, and preliminary reviewing AIGC-generated content. Second, job functions change, and the role of content creators may shift to AIGC content strategists or AIGC content supervisors, responsible for guiding and supervising the AIGC creation process to ensure that the output content meets creative goals, ideological standards, and regulatory requirements. Third, ethical and legal literacy requirements increase, as the content created by AIGC is richer and more diverse, involving copyright, originality, privacy, etc., which complicates issues that laborers need to be more ethically aware and legally literate to address potential intellectual property and ethical challenges. Fourth, interdisciplinary collaboration increases, as the application of AIGC technology promotes cross-disciplinary and cross-professional collaboration, such as working closely with data scientists and engineering teams to develop customized large models to meet specific content production needs.
In summary, the efficient allocation of production factors realizes the leap in productivity, forming the embryonic form of a new productive force in broadcasting and television and online audiovisual, reflecting the quality, efficiency, and driving changes brought about by AIGC in the broadcasting and television network audiovisual industry from macro, meso, and micro levels.
Current Status and Issues of AIGC in Broadcasting and Television
(1) Current Status of AIGC Application in Broadcasting and Television
1. Mainstream Video Generation Large Models
Currently, there are three main categories of video generation models domestically and internationally: diffusion models, transformer models, and the DiT (Diffusion Transformer) model that combines the two. Applications related to generated videos can also be divided into three categories: text-to-video models, image-to-video models, and video optimization models.
From the above, it can be seen that the DiT model is currently the main model for text-to-video applications. According to documents released by OpenAI, Sora’s training has a similar underlying algorithmic logic to other large models, but it leverages ChatGPT’s natural language understanding capabilities, emerging with an understanding of basic physical rules, thereby achieving precise presentation of user needs. According to its published paper, Sora has advantages in image deconstruction training and multiple algorithm combinations.
On one hand, in terms of image structure, the OpenAI team decomposes images or clips into “patches” during the training process. These patches are like words or letters in text, allowing AI to learn to understand and process video data by learning these “patches”. This complex image can be transformed into simple patches, each being a “patch”. This method enables large models to process and generate videos more effectively and provides the foundation for training the DiT path that combines diffusion and transformer models.
On the other hand, Sora’s construction uses a combination of multiple high-quality algorithms. Before Sora’s release, the image generation field had formed two mainstream algorithms: Diffusion and Transformer. The DiT architecture used by Sora integrates both. By adding noise through the Diffusion process, the image is disturbed, and then the diffusion algorithm randomly denoises to generate a clear image. The Transformer, as a deep learning algorithm, uses an Encoder-Decoder architecture and introduces self-attention and multi-head attention mechanisms, learning to understand and create images through pre-training on “patches”. If the Transformer architecture is a versatile interpreter, then the Diffusion architecture is a creative artist. Sora combines these two technologies to not only create a variety of images and videos but also to create based on text.
If the emergence of large language models represented by ChatGPT marks the beginning of machines “understanding human language”, then video generation models represented by Sora signify that artificial intelligence has begun to “depict the world”. In the future, with technological advancements, more human-like “senses”, such as smell and touch, will be endowed to artificial intelligence.
2. Three Main Modes of AIGC Content Production
(1) AI Generate (Large Model) Production Mode
This approach generates content directly through user input commands, supplemented by text, images, videos, etc., by large AI models. The generation process remains a “black box”, and once humans complete the algorithm and model training, the inference process of the video large model escapes the control of creators, resulting in high unpredictability of the generated results.
The large model production method requires a significant investment of training data and computing resources based on a specific general large model, with high initial construction costs, and requires cooperation and support in scientific research, demand applications, security development, and industrial ecology. In 2023, the Central Radio and Television Station, in collaboration with the Shanghai Artificial Intelligence Laboratory, released the “CCTV Listening Media Large Model”, with “Qianqiu Shisong” being the first series of animations produced under this model, highly reproducing the character shapes, scenes, and props in Chinese ancient poetry.
Based on the “CCTV Listening Media Large Model” text-to-image and controllable image generation technology, prompts are used to generate visual content, and style reference images, sketches, etc., can also be used to refine the generated content, creating the desired character images, scenes, props, etc. For example, when generating scenes, one can select a reference image and provide prompts such as “Chinese style, Tang dynasty, autumn and winter roads” to generate the corresponding AI scene.
In terms of dynamic effects, the “CCTV Listening Media Large Model” uses text-to-video and image-to-video functions. After selecting the main character, inputting dynamic effect prompts can yield animation effects. For example, selecting the character of an ancient scholar and inputting “Tang dynasty, a scholar holding an ancient zither” can generate the corresponding animation; inputting image materials and using image-driven video can create 4-8 second animation videos. Additionally, based on the thematic consistency module, the generated animations can achieve a “multi-camera” effect, maintaining a coherent storyline.
In post-production, voice and music models are combined to generate rhythm-matched and melody-matched voiceovers and soundtracks, supplemented by manual editing to produce the final piece.
(2) AI Workflow Production Mode
This mode of video production is akin to an “AI combo”, where the creator is central, and the creative capabilities at various stages are enhanced by different large models. It is currently the most widely used production mode in the industry. Based on the functional differentiation of foundational models, this type of video creation can be divided into several stages:
Compared to the large model production method, the workflow production method has lower initial construction costs, requiring only the integration of relevant model interfaces to leverage artificial intelligence in traditional content production processes for digital synthesis and virtual-real integration effects. Many mainstream media outlets have adopted this “one-stop AIGC workstation” content production method to enhance production efficiency. The “Smart Media Cube” of Shanghai Broadcasting Network, the “AIGC HUB” of Mango Super Media, and the “Zhizhu AI Smart Application Platform” of Chengdu Broadcasting Network are all similar out-of-the-box AIGC toolsets that meet some needs in mainstream media content creation.
Following “Qianqiu Shisong”, the Central Radio and Television Station produced and aired the AI full-process micro-drama “Chinese Mythology” using the workflow production method, achieving that all aspects of art, storyboarding, video, voiceover, and music were completed by AI. The “Chinese Mythology” AI toolset integrates four important functional applications: “text-to-script”, “text-to-image”, “image-to-video”, and “text-to-audio”, with the “text-to-script” function based on the GPT-4 language model, the “text-to-image” function based on the Midjourney painting model, the “image-to-video” function based on Runway and Pika video generation models, and the “text-to-audio” function based on the Suno music model.
To ensure the unification of mythical scenes and characters with Eastern aesthetic roles and the coherence of the storyline, creators repeatedly modified prompts to generate mythological characters and scenes that align with public perception and possess Eastern charm, with the prompt in the “text-to-image” stage playing a crucial role. To present the story comprehensively to the audience, more emphasis was placed on character presentation, logical coherence, and emotional expression in the content production.
In the “image-to-video” stage, to maximize the character’s “acting skills” and explore the continuity of character actions and emotional expressions, the production team attempted various parameter combinations to overcome the synchronization issues between the subject’s motion amplitude and image stability. For instance, in the series “Filling the Sea” from “Chinese Mythology”, to animate the “bird feathers gently moving” scene for 2 seconds, creators repeatedly adjusted parameter combinations to achieve the desired effect, with the voiceover and music for the series also completed by AI, demonstrating a degree of creative capability.
(3) AI Agent Production Mode
Intelligent agents are capable of directly facing target tasks, possessing autonomous memory, reasoning, planning, and execution capabilities, generating video content directly from human instructions without human intervention during the process. In this regard, intelligent agents are similar to the generation process of large models. The core difference is that the generation process of intelligent agents is no longer a “black box”, and human factors have a greater influence on creation. As a model that controls foundational models, intelligent agents possess long-term memory and can decompose work tasks based on user habits, local data, and professional datasets after receiving user instructions, finding the best way to execute the instructions through adaptation with various foundational models.
The production method of intelligent agents may be a trend for future AIGC applications, with the core of the intelligent agent production method being enhanced autonomy, allowing AI to independently complete a work node or reduce human intervention at a certain work node. Currently, the content production method of intelligent agents is still in the exploratory stage, and from the perspectives of construction costs, generation effects, and output efficiency, this content production method is worth the attention of content production organizations.
(2) Issues in AIGC Applications
1. Insufficient Model Maturity
From the perspective of generation effects, text-to-image models generally have poor understanding of quantity and negative instructions, exhibiting issues such as weak authenticity, insufficient texture details, and poor consistency in generated images. The common shortcomings of multimodal large models currently include:
(1) Realism: Although AIGC technology has made significant progress in content generation, the generated video content still cannot match the realism of the real world. This includes the physical properties of objects, lighting effects, texture details, etc., which are difficult to achieve the complexity and diversity of the real world.
(2) Diversity of Scenes and Elements: AIGC models have limitations in generating diverse scenes and elements. If the types of scenes and elements in the training dataset are limited, the content generated by the model will appear monotonous and repetitive, lacking the richness and variability found in the real world.
(3) Coherence and Logic: Video content needs to maintain coherence and logic over time. AIGC models may encounter difficulties in maintaining content coherence when generating long videos or complex scenes, resulting in unnatural or illogical jumps in the generated videos.
(4) Character Interaction and Dynamic Behavior: In scenes involving multiple characters or dynamic interactions, AIGC models may struggle to accurately simulate the complex interactions and behaviors between characters. This includes actions, expressions, dialogues, etc., which may not achieve the naturalness and fluency present in the real world.
2. Insufficient Computing Power
Compared to text and static images, video content has higher data density and complexity, requiring processing of a large amount of pixel information for each frame. For long videos, this not only means a linear increase in data volume but also involves processing the temporal relationships between consecutive frames, which places high demands on computing resources. Especially when using HD or ultra-HD resolutions, processing a single frame image requires substantial computing power, let alone synthesizing coherent dynamic videos.
Insufficient computing power is one of the main limiting factors for AIGC in generating long videos. First, due to limited computing resources, the time cost of generating long videos is extremely high, often requiring hours or even days, making it difficult to meet the needs for rapid creation and instant feedback. Second, to complete tasks within limited computing power, it may be necessary to reduce model complexity or video quality, resulting in missing details and decreased smoothness in the generated content. Third, high computing power demands hinder the speed of algorithm exploration and iteration, obstructing the development and application of new technologies and models, and affecting content diversity and creative expression.
At the technical level, every link in the video generation process, from in-frame rendering to inter-frame prediction, is a highly computationally intensive task; larger and more complex models can generate higher quality content, but their demand for computing power grows exponentially. Although accelerators like GPUs improve parallel processing capabilities, in large-scale long video generation scenarios, data transmission and synchronization issues become bottlenecks; long video processing requires storing large amounts of intermediate data in memory, and existing hardware configurations often struggle to meet the needs of such large-scale data processing.
3. Immature Evaluation Index System for Large Models
The evaluation index system for large models is crucial in the application process of models, directly influencing model selection, optimization, deployment, and effect evaluation.
Researchers from Tsinghua University’s School of Journalism and Communication have conducted a series of studies on the comprehensive performance of large language models, developing the “Meta Test” model evaluation system, which provides five primary indicators: usability, availability, credibility, substitutability, and plasticity, along with 26 sub-indicators, evaluating the physical properties, generation effects, safety, perception abilities, and interference resistance of the models, using a combination of objective and subjective evaluation methods, with objective data being obtainable.
China University of Communication has conducted research on the subjective evaluation system for text-to-video models, employing subjective evaluation methods that directly use viewers’ visual perceptions as subjective evaluation results, evaluating model generation quality based on four primary indicators: text-image consistency, realism, video quality, and aesthetic quality, with 26 secondary indicators; this subjective evaluation system highly depends on the evaluators’ professional recognition abilities and data analysis, requiring planning and implementation by professional institutions.
Currently, the evaluation work for large models does not have an industry-recognized evaluation index system, particularly for visual large models, where research by evaluation institutions is still in its infancy, lacking more granular indicators, and the datasets and evaluation strategies used for assessment are still in exploratory stages, with no systematic evaluation plans in place.
4. Intellectual Property Disputes in Content Generation
AIGC is essentially an application of machine learning, and during the model training phase, it is unavoidable to use an encyclopedic volume of massive datasets for training. However, there is currently no conclusion on the copyright ownership of the generated content post-training. The current industry views on copyright issues related to AIGC mainly fall into two categories. One viewpoint argues that content is generated from a material library and originates from the material library, requiring copyright payments to the relevant material authors. However, for AI projects in broadcasting and television and online audiovisual, the AI’s material learning library is vast, making it impractical to obtain authorization for all training sets. Additionally, AIGC is essentially a process of machine re-creation, akin to a director creating new works after watching hundreds of thousands of films, inevitably influenced by the works viewed, yet requiring payment of copyright to all authors of the films watched is unreasonable. The other viewpoint holds that the process of AIGC generating content is entirely random and innovative, with no copyright issues, and the copyright belongs to the users or platforms of AIGC, with specific regulations set by the platforms.
In addressing copyright issues, platforms may adopt the following three approaches: First, if the generated work is created by the author using AIGC tools, the copyright belongs entirely to the author; second, if the generated work is produced by the platform’s AIGC tools, the copyright belongs to the platform, but the author can use it freely for non-commercial purposes, while only paying users have the right to use it freely for commercial purposes; third, if the generated work is derived from public works data training, its intellectual property should not be owned by any institution or individual, and any works generated by anyone can be used freely by others in any legally compliant manner.
Research institutions generally believe that at the current stage, where AIGC’s content production level has not reached a high level, there is no need to consider the copyright issues of works. The most critical task now is to find ways to address the issues of insufficient computing power and outdated algorithms. Content production organizations approach the copyright issues of AIGC works cautiously, believing that AIGC-generated works are also the fruits of human labor and are labor products directly influenced by prompt engineers on production materials, and copyright should be adequately protected.
Prospects for AIGC Industry Applications
Despite the challenges and difficulties currently faced by AIGC technology, it is one of the important trends in current and future technological development. The emergence of AIGC technology will trigger changes in the broadcasting and television and online audiovisual dissemination systems. When productivity undergoes qualitative changes, it will inevitably lead to a reconstruction of production relationships, forming new production relationships that are compatible with AIGC. The broadcasting and television and online audiovisual industry will usher in systematic reforms in construction, operation, and management, which will be an important measure for further deepening reforms in the broadcasting and television and online audiovisual industry.
Accelerating the formation of new productive forces in broadcasting and television has become a common goal and consensus across the industry, forming a new dissemination system based on data, which may be one of the trends for high-quality development in broadcasting and television. With an intelligent production platform based on “data + models”, optimizing and enriching content supply; with an intelligent dissemination platform based on “computing-network integration”, expanding the dissemination effects of mainstream media and network dissemination capabilities; with an intelligent interactive platform based on “virtual + reality”, expanding new cultural consumption scenarios; and with an intelligent regulatory platform based on “model-to-model”, promoting the systematic process of ensuring safe broadcasting.
(1) “Data + Models” in Content Production
The traditional content production methods of mainstream media have been limited by three factors: cost, technology, and resources, leading to a slow development of content output volume and production efficiency. The introduction of AIGC will bring higher production rates and more diverse content output to mainstream media content production. Although AIGC is dominated by algorithmic models, human content creators still play a crucial role and cannot be completely replaced in the short term. The optimization of algorithmic models and the judgment of values are the core of this new content production method. In the near future, the human-machine collaborative content production method will be the best model for mainstream media to achieve high-quality content production.
This new production method will introduce new content production factors, no longer limited to real sounds and images; virtual scenes, digital humans, and AI voices will become important components of content production factors. By leveraging the natural language processing capabilities, visual processing capabilities, and semantic understanding capabilities of large models, cross-modal and cross-platform content matching can be achieved, further enriching content production. Mainstream media content production will move towards the direction of “data + models”, further enhancing the attractiveness, dissemination power, and influence of broadcasting and television and online audiovisual.
(2) “Computing-Network Integration” in Intelligent Dissemination
According to the “China Computing Power Development Index White Paper (2022)” published by the China Academy of Information and Communications Technology, as of the end of 2021, the total global data output reached 67ZB, and China’s total data output reached 6.6ZB, with the global total computing power scale reaching 616EFLOPS. By 2030, global data is expected to reach YB levels. In the future, AIGC will improve the efficiency of content production, giving rise to rich interactive application forms. The new broadcasting and television network will inevitably carry more content and a wider variety of business forms, meeting the needs for cross-screen, cross-network, and cross-terminal dissemination of programs in the AIGC era. The new broadcasting and television network will bear enormous data computing and network security needs, requiring unified management and integrated routing scheduling of computing power and network resources.
The intelligent dissemination platform based on “computing-network integration” will become a solid infrastructure supporting the development of artificial intelligence. Content production and network operations will no longer be separate business segments, but rather an organic whole within an efficient, reliable, secure, and user-friendly network ecological environment.
In the AIGC application era, the computing environment and resource configuration required for training and running large-scale machine learning models, especially deep learning models, have extremely high demands for computing power, data management, storage, bandwidth, and network performance, necessitating stronger support for various AI applications.
On the basis of the intelligent dissemination platform for “computing-network integration”, mainstream media will construct a differentiated and characteristic business system, achieving a management goal that combines “grasping connotation” and “grasping dissemination”. On one hand, it will highlight the characteristics of mainstream media, making good use of broadcasting and television content resources; on the other hand, it will enhance the coverage, reach, and influence of mainstream media, meeting the growing cultural life needs of the people, bringing richer viewing experiences into the dissemination channels of mainstream media, and strengthening the integration of the entire process, all channels, all screens, and all services.
(3) “Virtual + Reality” in Intelligent Interaction
Under the influence of AIGC, content is diversifying. The use of large models not only broadens the creative modes of broadcasting and television and online audiovisual practitioners but also lowers the threshold for users to use and participate in creation. The dissemination network is no longer just a channel for program transmission but will systematically resolve the allocation of computing power and network resources, providing possibilities for mainstream media to enrich their business models. The interaction space between mainstream media and audiences will also expand from the real space to the virtual space, ushering in a new transformation in the interaction methods between mainstream media and audiences. More content-rich works will be integrated into the work and life of the people through cross-interaction methods, meeting the information needs, cultural needs, and entertainment needs of the people.
In the future, AIGC will bring profound changes to blockchain, Web 3.0, and the Metaverse, accelerating the formation of parallel worlds with broad digital twin forms and physical forms. In the Metaverse space, through VR/AR/MR/XR and the new content modality of “script killing”, new ideas will be generated, stimulating the enthusiasm of the people to love, inherit, and promote excellent traditional Chinese culture. By utilizing multimodal sensory perception, data visualization tools, immersive audiovisual technologies, integrating enhanced analytical tools, geographic location data, sensory stimuli, automatic speech recognition, and behavioral algorithms, a connection between real life and the Metaverse space can be achieved, forming a cross-interaction platform between content production large models and audiences, innovating new categories and services of mainstream media culture, and creating a new space for cultural consumption.
(4) “Model-to-Model” Intelligent Regulation
The new video generation large models involve massive training datasets, featuring high intelligence, fast generation speed, and strong creativity. Due to their ability to simulate dynamic visual effects and capture interactive patterns consistent with daily life experiences, the generated video content may involve various complex scenes and plots, especially when used by individual users, raising issues such as copyright, privacy, security, false content, and undesirable values, which are exacerbated by the rapid generation speed and large quantity of AIGC.
The “model-to-model” intelligent regulation approach is an industry management idea that involves using algorithms to manage algorithms and models to supervise models. Specifically, it involves using a set of algorithms or models to monitor and evaluate the behaviors and characteristics of another set of algorithms or models to ensure the transparency, fairness, and security of algorithms and models, safeguarding the legality and compliance of video content generated by large models, while better controlling the potential risks of artificial intelligence systems and promoting their positive development.
AIGC integrates data, algorithms, and computing power into the operation of the entire industry, and large models are likely to become a public infrastructure in society in the future, participating in the entire process of content production and dissemination. The attributes of ideology, public service, and technological industry determine the basic positioning of large models in broadcasting and television. The training datasets of large models are the fundamental guarantee for their effective operation, and the intelligent network infrastructure based on “computing-network integration” is the foundation for the operation of large models in broadcasting and television. Building a broadcasting and television large model that aligns with the core values of socialism with Chinese characteristics is a common vision for the industry and a choice for its development.
This article was published in the “Audiovisual World” 2024 Issue 4
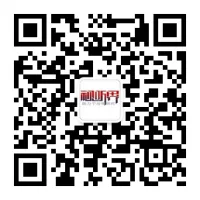
Audiovisual World
Long press to recognize the QR code to follow us
WeChat: jsbc-shitingjie
National Press and Publication Administration recognized academic journal
Top Ten Academic Journals in National Broadcasting and Film
Postal issue number 28-320
Annual price 108 yuan
Phone: 025-83287966
Submission: [email protected]