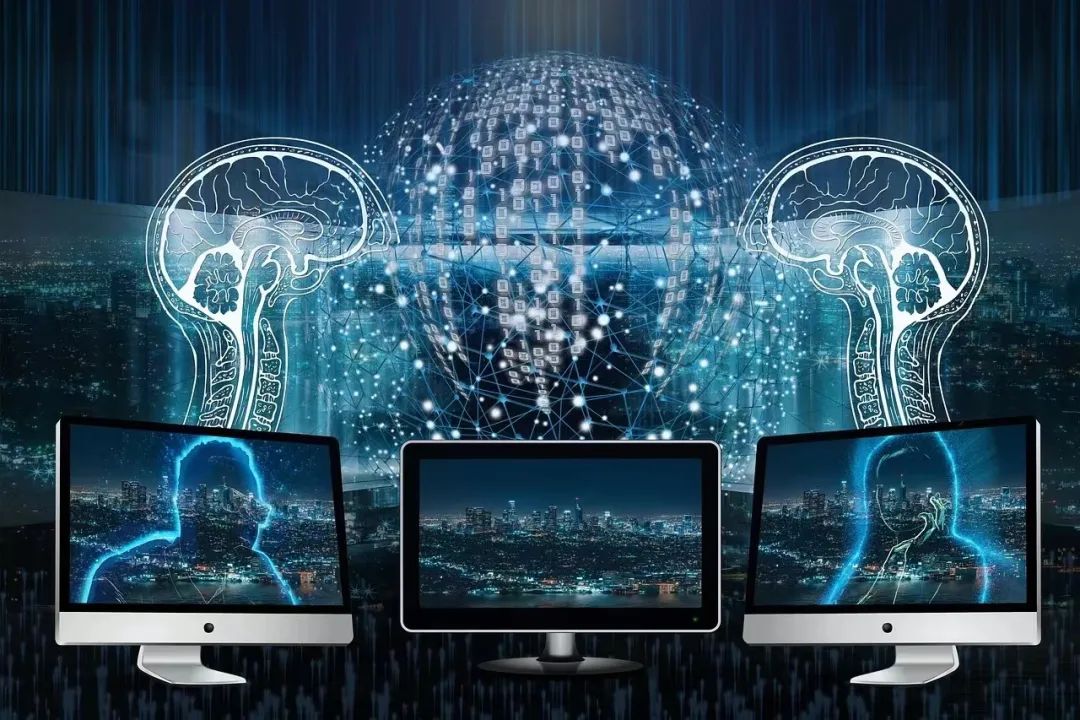
● ● ●
Artificial Intelligence
(AI)
tools are changing the way scientific research is conducted. AlphaFold has essentially solved the problem of protein structure prediction; DeepMD has significantly improved the efficiency and accuracy of molecular simulations; and emerging large language models, such as ChatGPT, are also expanding the boundaries of scientific research.
In a roundtable discussion organized by the National Science Review (NSR), five experts from China and the United States discussed the concept, development, bottlenecks, and opportunities of “AI for Science” (AI4S), sharing their understanding of the relationship between AI and Science.
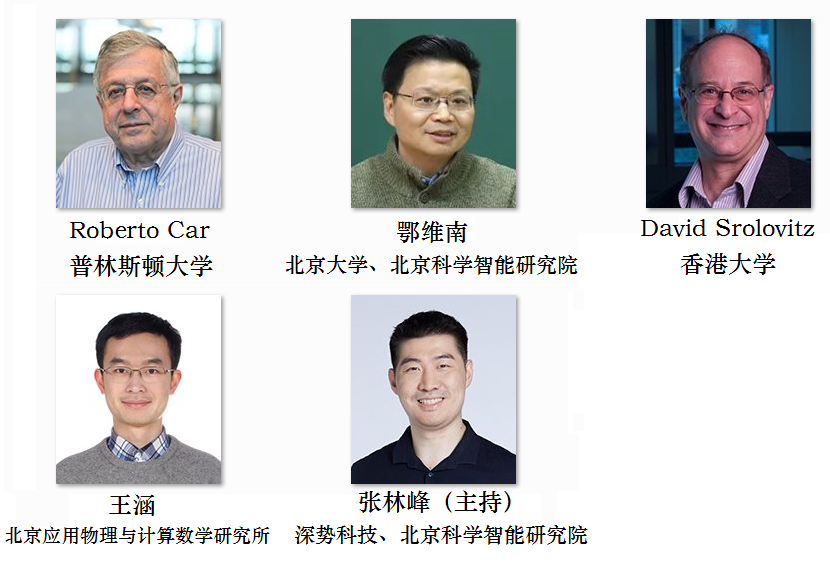
Emerging AI4S Tools
Zhang Linfeng: Let’s start with a fundamental question: What is “AI for Science”?
He Weinan: AI for Science is a new research paradigm where we use AI tools to enhance our ability to conduct scientific research, similar to how we use computers to assist scientific research. Specifically, AI-based algorithms can greatly improve the efficiency and accuracy of first-principles modeling. AI can also improve our experimental methods by providing new experimental designs, more accurate and efficient experimental characterization algorithms, or even new experimental devices. Furthermore, the workflow and open-source, collaborative spirit in the AI field inspire scientific research.
Roberto Car:: In my view, AI provides a set of tools that can facilitate scientific discovery, represented by machine learning and deep neural networks. To achieve this, specific tools need to be developed. I would like to cite three examples from my research area.
First, AI can bridge the gap between quantum mechanics and classical coarse-grained models. In this field, AI has significantly improved the accuracy and time/space range of molecular coarse-grained models used for molecular simulations. This enhancement may seem like a quantitative change, but in these fields, quantitative changes can lead to qualitative changes. As Philip Anderson pointed out: more is different. Such tools have already led to new discoveries.
The second example is that AI can design new materials and molecules with specific properties. I am not directly engaged in this research area, but I know many people are working on it, and AI can leverage vast amounts of data—from experiments, theories, and simulations—to predict which materials or molecules may be better suited for certain purposes.
The third example is that AI can be used to analyze experimental data, for instance, by enhancing the signal-to-noise ratio of software to improve probe selectivity.
Wang Han: Besides the examples mentioned by Roberto, AI tools are also changing the way we handle scientific data. In particular, large language models can efficiently extract knowledge and key points from scientific data and literature.
Moreover, AI is also transforming the development of scientific software, enabling automatic code generation, software bug detection, and providing suggestions for improving code efficiency. All these AI tools significantly enhance the efficiency of scientific research.
David Srolovitz: AI will indeed disrupt our traditional way of reading literature. In the past, when we wanted to thoroughly read and understand the literature in a field, we would have a graduate student read those papers and draft a review article. But in the future, we will be able to have large language models do that. The volume of literature is so vast that I believe humans cannot digest so much information.
New Opportunities Brought by Large Language Models
Zhang Linfeng: Besides reading papers, what new possibilities will large language models bring to AI4S?
David Srolovitz: I have recently been “playing” with these models. We tried a case reported by others: letting a large model predict whether a material is glass, amorphous, or crystalline. We provided it with 15 examples and then asked it to classify another 10 materials, and it provided answers in seconds with an accuracy of 70%. This is interesting, and what’s more interesting is that when you ask the model, “Why did you give these results?”, it will provide a rationale. Although the reasoning it provides may not be reasonable, it can indeed answer the question.
Regarding the further development of these models, I think prompt engineering is an intriguing direction that can help us better guide the models to do more astonishing things.
Wang Han: Generative model technology is very helpful for scientific research. For example, many scientific problems require sampling from high-dimensional probability distributions, which is a generative problem. Successful examples have emerged in this area, where generative tools like diffusion models and generative adversarial networks (GANs) can generate samples of high-dimensional distributions.
Another example is that conditional diffusion models can be used to design molecules with specific properties under given conditions. This opens up new possibilities for solving molecular and materials design problems.
Zhang Linfeng:
A great starting point for large models to assist scientific research is their ability to read images. For example, large models can be used to read electron microscope images and generate structures from those images.
Currently, we are trying to develop new tools to enhance large language models’ ability to understand scientific literature. In particular, in literature, molecules are represented in various forms such as text, molecular formulas, and images, and the current models do not combine this information well for thorough understanding.
He Weinan: Large models may provide suggestions for new problems and ideas, and help integrate different disciplines.
AI and Science: Answers and Understanding
David Srolovitz: I would like to discuss the relationship between artificial intelligence and science from a somewhat philosophical perspective. I once thought that the methods of artificial intelligence were anti-scientific because they often aim to obtain “answers” rather than “understanding.” However, the mission of science is precisely to gain “understanding.”
However, I have changed my mind. The reason is that I have begun to realize that when we can obtain many credible answers, these answers can provide valuable hints to guide the development of science. This is similar to simulations. As a theoretical researcher, I always viewed simulations as a method to peek at answers before theories mature.
Over time, I have also realized that some things can only be achieved with vast amounts of data. Massive data allows us to identify and understand things in ways that were previously unimaginable. As I just mentioned, if you ask large language models to explain how they arrive at certain answers, they can provide explanations. However, clearly, the explanations they provide are not yet satisfactory for scientists like us. There is still a long way to go in explainable AI.
In any case, AI is changing the way we do science, and I believe we are at the early stages of a new scientific paradigm.
Wang Han: I believe that a major weakness of current large language models is that they cannot reason logically like humans. This may be why they struggle to explain the answers they provide.
David Srolovitz: That’s right. But do you think that reasoning like humans could yield better answers? I’m not so sure about that.
Roberto Car: I agree that new data from AI tools can provide new insights, but once you have those data, human scientists are still needed to decide what analyses to perform next. This is something that AI cannot do.
AI is indeed creating a new paradigm for scientific research, but that does not mean traditional research concepts will be replaced or discarded. On the contrary, traditional theoretical research needs to be strengthened to better validate the robustness of machine learning models. These models allow us to extrapolate model predictions to environments broader than those in which the training occurred. However, it is often difficult to validate these predictions within strict mathematical boundaries.
For example, as I mentioned, in simulations, AI bridges the gap between quantum mechanical calculations and molecular simulations. But in large-scale systems, if rare events that are bound to occur are not accounted for in the training data, AI tools may fail. That is to say, when we need to analyze problems using fundamental tools of physical intuition and physical thinking, it will promote the development of new theoretical models—these models will ultimately be represented by new differential equations and will better describe the dynamics of complex systems than existing models.
He Weinan: AI often gives the impression of being black magic, but I think the reality may not be so. Currently, AI is still very much like an engineering experimental field. But I believe that over time, this situation will change, and people will begin to find some guiding principles. In fact, there has already been much progress in this direction, although for some reason, the larger AI community seems not to be aware of these achievements. So I believe that AI will also become a relatively scientific discipline.
AI is indeed better at obtaining answers rather than understanding. But this is not necessarily the case. One example is that knowledge graphs can be used to understand the relationships between different molecules. I am not sure if this has already been achieved, but it is certainly a beneficial direction. We created a knowledge graph on economics that helps reveal how different economic parameters are interrelated. I believe such attempts are very helpful and enlightening in science.
Is AI Creative?
Roberto Car: In my impression, AI models can perform routine analyses but find it hard to do anything that requires creativity. But I could be wrong, or in the near future, I might be wrong.
He Weinan: I believe AI can create, but it is too early to discuss the details now.
David Srolovitz: Current generative models can create artworks, and I feel they perform very well in this regard. Is this creativity? I’m not sure. Speaking of which, I have always thought that interatomic potential is a scientific art. 15 or 20 years from now, scientific research may no longer follow the ways we are familiar with.
Wang Han: My understanding of art-generating models is that the images they generate are more or less combinations of existing artistic styles from the training data, rather than creating entirely new artistic styles. However, in reality, most human artworks are also combinations of existing artistic styles and works, and AI can creatively combine them.
David Srolovitz: Scientific research is also like this; I believe most research work is about recombining existing things in new ways.
Building an Open Environment for AI4S
Zhang Linfeng: Who should be the main drivers of AI4S, scientists or tech companies? How should stakeholders collaborate?
He Weinan:
I hope the scientific community can lead the AI4S revolution. Tech companies need time to grasp the necessary scientific background knowledge. More importantly, the scientific community has always been the main force in scientific research. In the future, AI methods will be integrated into scientific research in all aspects, and if we lose our leadership position, we will find ourselves in a very difficult situation, much like what has happened with large language models.
David Srolovitz: Clearly, no single research team can independently create competitive large language models. As scientists, we should not attempt to write our own versions. What we should do is learn how to harness, train, and design them to do what we want to do. Just as no research team would attempt to build its own hadron collider. They are tools that scientists can utilize.
He Weinan: Ultimately, this is a question of input and output. Currently, although commercial opportunities have emerged, AI4S is largely still a direction of scientific research. This presents a great opportunity for funding agencies. The National Natural Science Foundation of China has funded major research programs supporting AI4S.
Wang Han: I believe that the interests of companies may not always align with those of scientists. If it does not generate profit, companies will not develop tools for scientists. This may be the main divergence between the scientific community and companies. This divergence could be bridged through government investment, but I am not sure if that will be enough.
David Srolovitz: Now, scientific researchers, including those in military technology, are learning how to use commercial software and technologies to solve scientific problems they care about. Although these technologies are not developed for them, they can learn how to utilize them. So I believe if some AI tools are not designed for scientists, the challenge we face will be learning how to use them to do what we want to do as scientists.
Zhang Linfeng: Developing AI4S tools, such as the DeePMD-kit we are developing, relies on the collaborative efforts of many community partners. During the development process, the challenges and bottlenecks we face will constantly shift.
Initially, the main challenge was model design and software development. Subsequently, to meet the needs of different users, we need technical talents who are both proficient in software operation and understand scientific issues. After that, infrastructure such as high-performance computing and cloud computing became new bottlenecks. Currently, based on the accumulation of a large amount of data, we have the possibility to develop large atomic models, but we are also facing new bottlenecks in model and software development again.
In this process, we are also committed to developing an open-source community called DeepModeling and building a stable user platform for these tools, named the Bohr Space Station. We hope this interface can be as intuitive and easy to use as personal computers or smartphones, allowing different users to freely explore and solve their respective problems.
Roberto Car: I’m not sure if we need to create an interface that integrates all AI4S tools, but some degree of integration is certainly beneficial and will emerge. This requires more interaction between different sub-communities, including simulation researchers, materials designers, experimental researchers, and so on.
David Srolovitz: One fact is that scientists are not good at developing interfaces or standardizing toolsets. Scientists are better than companies at posing good questions, which is crucial for science itself.
Roberto Car: We need both companies and scientists. We need an open environment where information can be easily exchanged, everyone can view data, and new questions can be freely posed. If we can maintain this environment, there will be significant progress. But unfortunately, creating such an environment faces many difficulties.
David Srolovitz: Yes. Openness is essential. However, it is disappointing that whenever a technology appears that could change the economy and society, different countries try to develop it into their unique advantage. I am very optimistic about AI technology and what it can contribute to science, but this “exclusivity” may impede technological development more severely than anything else. However, it reminds me of the famous line from the movie “Jurassic Park”: “Life finds a way.” I believe we will eventually break through this situation; the question is how long it will take.
Challenges and Opportunities
Zhang Linfeng:
Thank you all for the discussion. As a summary, please provide what you think is a bottleneck currently facing AI4S, and one of your suggestions.
Roberto Car: One bottleneck for AI4S in the field of molecular simulations is its inability to handle electron transfer phenomena well. Electron transfer is essential for various chemical reactions, but since we cannot yet capture precise electron coordinates, it remains challenging to simulate this phenomenon. To solve this problem, we need not only the development of AI technologies but also new modeling methods that transcend the current scientific paradigms based on fundamental physical laws (such as the Born-Oppenheimer approximation and density functional theory).
One suggestion I have for AI4S is what we have already discussed: we need to make every effort to maintain an open research environment and operate according to scientific laws and methods.
David Srolovitz: Looking ahead, I am genuinely interested in seeing more developments in “explainable AI” to understand the reasoning behind AI predictions. There is a lot of work to be done in this area, and I am optimistic about it. I also believe that these advancements may benefit science more than they benefit computer science and AI technology.
Wang Han: For me, the next significant opportunity will be large atomic models. The ultimate goal is to establish a universal model of the periodic table, but this goal may not be achieved in the foreseeable future. However, large atomic models, as pre-trained models for atomic simulations, will be achieved in the near future.
The bottleneck for large atomic models is the dataset. The broader the range of data in the training set, the stronger the model’s generalization ability. However, unlike large language models, which can obtain language materials from various sources, the training data required for large atomic models is very limited and expensive, such as fine crystal structures and DFT calculation results.
He Weinan: The biggest bottleneck for AI4S currently is the lack of good data. One of my suggestions is to use new AI-based models to generate high-quality data. To achieve this, we also need to further improve simulation capabilities. For instance, although AI-based algorithms have significantly improved the accuracy of ab initio calculations and molecular dynamics simulations, we are still quite far from being able to use these new tools to simulate real systems of interest; the time scales that can be simulated remain limited, and modeling defects is still quite expensive.
*This article’s original English version “A panel discussion on AI for science: the opportunities, challenges and reflections” was published in the National Science Review (NSR) Forum: https://doi.org/10.1093/nsr/nwae119