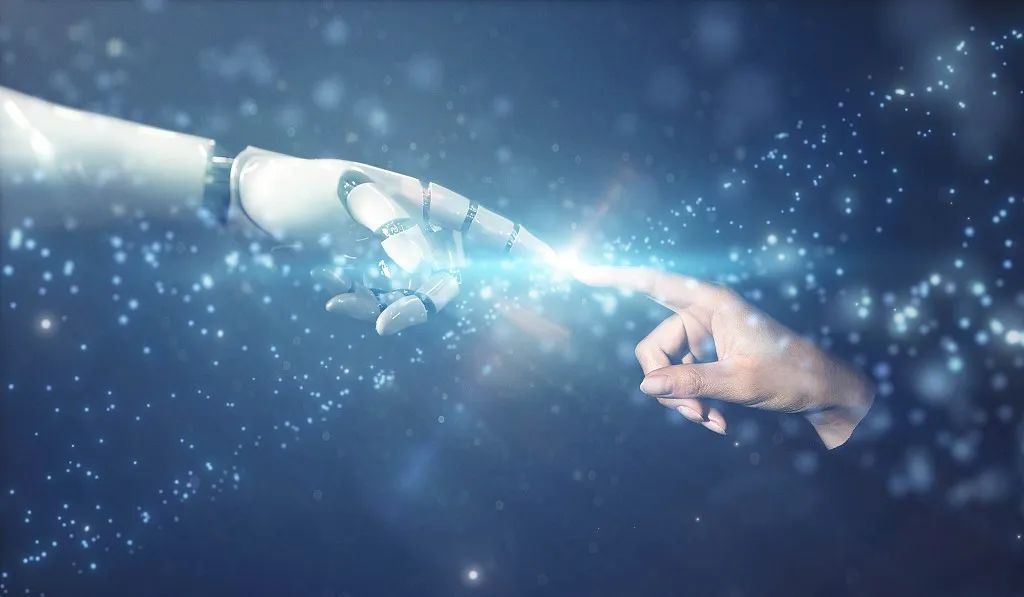



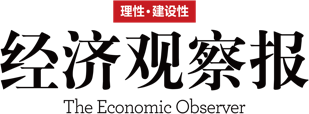

Introduction
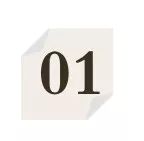
Proteins play a very important role in the process of life. On one hand, they are the builders of organisms; from a single cell to various organs, all need to be constructed by proteins. On the other hand, they are also important participants in many life activities, whether it is for substance transport within organisms, catalyzing various biochemical processes, or resisting external invasions, all rely on the involvement of proteins.
Currently, there are over 200 million known proteins, each with a unique three-dimensional structure, and their functional differences are determined by these different structures. For example, people often try to supplement collagen for moisturizing and skin care needs; the secret lies in the structure of this type of protein, which is similar to a twisted rope, thus possessing strong toughness, allowing it to transmit tension between cartilage, ligaments, bones, and skin. Similarly, the antibody proteins in our immune system roughly exhibit a Y-shaped structure, forming a unique hook shape, which allows them to attach to viruses and bacteria, detecting, marking, and eliminating pathogenic microorganisms. Because of the relationship between protein structure and function, the exploration of protein structure has become a focus of study for biologists since the mid-20th century.
In 1961, Christian Anfinsen, a researcher at the National Institutes of Health in the United States, published a paper introducing an experiment he conducted: in the experiment, he denatured the bovine pancreatic ribonuclease protein molecule with denaturing agents, reducing disulfide bonds to thiols, thereby destroying the original folded structure of the protein, leading to a loss of enzyme activity. Then, he left the beaker containing the experimental sample exposed to air overnight. To his surprise, after a night of exposure, most of the enzyme activity was restored, and the damaged protein structure refolded back to its original form. How strange is that? It’s roughly equivalent to taking a flower made of iron wire and straightening it with pliers, only to find that after some time, the straightened wire has turned back into a flower!
Why does this happen? Anfinsen proposed a hypothesis: this may indicate that the arrangement of amino acids in the protein polypeptide chain, known as the primary structure of the protein, determines its final three-dimensional structure—once the primary structure is determined, the polypeptide chain will obey the laws of thermodynamics and automatically fold into a state of energy minimization. In subsequent biological research, Anfinsen’s above conjecture was summarized as the “Anfinsen Rule.” In 1972, Anfinsen won the Nobel Prize in Chemistry for this important rule.
For researchers, the Anfinsen Rule points to an important research direction: the “protein folding problem”: since the three-dimensional structure of proteins depends on their primary structure, theoretically, people can predict their three-dimensional structure based on the energy optimization laws between molecules using the primary structure of the proteins. Since the function of proteins largely depends on their structure, if people can fully understand the three-dimensional structure of proteins, they can search for or even create the proteins they need based on this understanding. Clearly, the imaginative space brought by this is enormous.
However, as the saying goes, “ideals are abundant, reality is thin.” Although the potential value of the “protein folding problem” seems enormous, it is very difficult to predict protein folding through its structure due to the vast number of amino acids that make up the protein polypeptide chain. Therefore, the path pointed out by the Anfinsen Rule seemed bright, but for a long time, it became a road less traveled.
In contrast, biologists seem to prefer to explore protein structures through direct observation. From early X-ray diffraction methods to the more recent cryo-electron microscopy methods, with the continuous development of experimental tools, people’s ability to explore protein structures through experiments has gradually improved. However, despite this, compared to the vast number of protein types, people’s efforts to explore protein structures through experiments can only be described as a drop in the bucket.
In 2018, a turning point occurred. At the 13th Global Protein Structure Prediction Competition (CASP) held in November of that year, DeepMind’s AI program AlphaFold successfully predicted the structures of 25 out of 43 proteins, winning first place among 98 participants. In contrast, the second-place contestant only accurately predicted the structure of 3 proteins. Moreover, it is worth mentioning that in predicting the structures of certain proteins, AlphaFold’s conclusions were even more accurate than those obtained through X-ray diffraction and cryo-electron microscopy methods.
How did AlphaFold achieve such outstanding results? In fact, its method is quite simple: it learns from a large amount of protein sequence and structure data, looking for interactions between amino acid molecules and the evolutionary relationships between protein fragments, and then predicts the structure of proteins based on the identified patterns.
After its initial success, AlphaFold continuously drew inspiration from the latest developments in biology, physics, and machine learning to upgrade its algorithms, significantly improving its predictive capabilities. On July 28, 2022, DeepMind published a news article titled “AlphaFold Reveals the Structure of the Protein Universe” on its official website, announcing that AlphaFold had predicted the structures of almost all known proteins. Subsequently, all predicted protein structures were made available online for researchers to download and use. According to many researchers who downloaded the data, the accuracy of these data was very high.
Although there will still be a need to verify the prediction data provided by AlphaFold for a period of time in the future, it can be said that the “protein folding problem” that has troubled people for more than half a century has basically been solved.
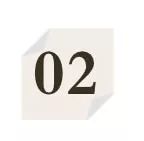
There is no doubt that AlphaFold’s success in cracking the “protein folding problem” has made a significant contribution to the development of biology. However, this event has an even more important significance: it proves that AI can play a crucial, even decisive role in the field of scientific research. Thus, “AI-driven scientific research” (AIforscience, sometimes abbreviated as AI4S) has become a prominent field of AI research.
The development of science is a process of continuous conjecture and verification. In scientific research, researchers first need to propose a hypothesis, then design experiments based on this hypothesis, collect data, and test the hypothesis through experimentation. In this process, researchers need to perform a large amount of calculations, simulations, and proofs. AI has significant applications in almost every step.
(1) Proposing Research Questions
Proposing a good question is the first step in conducting good research; only if the proposed research question is important can subsequent research be meaningful. Traditionally, scientific questions mainly come from two sources: one is proposing certain conjectures based on observations of phenomena and data; for example, the famous Kepler’s laws of planetary motion were proposed by Kepler after organizing a large amount of data left by the astronomer Tycho Brahe, and then established through theoretical research. The second source is through reviewing existing literature, seeing where previous research has shortcomings, and then proposing one’s own questions as breakthroughs. After using AI as a tool, researchers using both of the above methods to find questions can significantly improve their efficiency.
First, let’s look at proposing questions through observation. In the past, proposing questions through observation required a very high intuition from researchers. For example, Kepler’s first law (the law of ellipses) states that “the orbit of a planet around the sun is an ellipse, with the sun at one focus of the ellipse” is relatively intuitive, and one can propose this hypothesis based on observations of recorded data. However, the second law (the law of areas), which states that “the line segment joining a planet and the sun sweeps out equal areas during equal time intervals,” is not so intuitive; even the most careful person may only discover this rule with the inspiration of a flash of insight. As for the third law (the harmonic law), which states that “the square of the orbital period (T) of a planet around the sun is proportional to the cube of the semi-major axis (a) of its orbit,” it is an even less intuitive phenomenon, and only a very talented researcher might propose such a hypothesis.
After applying AI, people can relatively easily propose relevant research questions once they have sufficient observational data. For example, if people have a large amount of data on planet orbits and suspect that the time it takes for a planet to orbit the sun may be related to the length of a certain axis of its elliptical orbit, they can let AI try to establish a functional relationship between these variables. Through this method, Kepler’s third law could potentially be proposed relatively easily.
Next, let’s look at proposing questions through literature review. In the past, the number of people engaged in scientific research was relatively small, and the quantity of research was also relatively low; thus, a researcher could at least master the relevant literature in their field if they were willing to put in the effort. However, with the development of science, the number of people engaged in research has continuously increased, and various research results have emerged, making it increasingly difficult for a researcher to fully understand the progress in their research field, let alone keep up with developments in other fields to inspire their own research.
With the application of AI tools, the above problems can be alleviated to a large extent. For example, researchers can now use AI models like ChatGPT to organize existing literature and write summaries. Thus, they can significantly reduce the energy spent on searching and reading literature, gaining a better understanding of existing research progress at a lower cost, and based on this, propose new research questions.
(2) Data Collection
Once relevant research questions are proposed, researchers need to design experiments and collect relevant data to prepare for further research. In this process, the potential applications of AI are also very broad.
This role first manifests in data selection. In experiments, not all data is usable. Many data may be generated after being disturbed, and if these data are not removed, subsequent research results may be severely interfered with. Currently, deep learning has become the primary method for this task in many experiments.
After collecting data, annotating the data is also a daunting task. For example, in biology, labeling the functions and structures of new molecules is very important for subsequent research, but this task is not easy. Although new sequencing technologies continue to emerge, less than 1% of sequenced proteins have been annotated for biological function. Currently, to improve data labeling efficiency, researchers are trying to train AI to learn from manually annotated results, thereby training surrogate models to help label new data. Existing results show that this approach can effectively improve labeling efficiency.
In addition, AI now plays a very important role in data generation. This aspect is most evident in the field of AI research. In the past decade, the main development of artificial intelligence has come from the field of machine learning, which is known for its strong dependence on data. In practice, the collection and organization of data not only incur high costs and quality control difficulties but may also lead to issues such as invasion of personal privacy and threats to data security. To address these issues, some scholars suggest using synthetic data as a supplement to real data for machine learning.
Compared to real data, synthetic data has several advantages: on one hand, in terms of training effectiveness, models trained with synthetic data do not perform worse than those trained with real data; in some cases, their performance may even be higher. During the formation of real data, unnecessary noise information may be mixed in, which can affect its quality, while synthetic data does not have this problem. A joint study by MIT, Boston University, and IBM found that models trained with synthetic data performed better than those trained with real data in recognizing human behavior. On the other hand, the cost of synthetic data is much lower than that of real data. Additionally, since synthetic data is generated rather than collected, using them for research can also avoid many legal and ethical risks.
More and more AI researchers are beginning to replace real data with synthetic data as materials for machine learning, and their contributions to AI technology development are becoming increasingly significant. For this reason, the MIT Technology Review ranked synthetic data technology as one of the top ten breakthrough technologies in the world in 2022.
(3) Scientific Computation and Simulation
During scientific research, a large amount of computation and simulation work is usually required. For example, if scientists discover a certain star’s motion pattern, how can they prove that their discovery is correct? The most intuitive method is to calculate the position of this star at a future point in time based on the discovered pattern and then compare it. From this perspective, accurate computation and simulation are key to validating theories.
However, computation is not an easy task. For example, theoretically, the relative motion relationships between major celestial bodies can be derived from the law of universal gravitation. After discovering the three laws of motion, Newton famously claimed that he had mastered the ultimate secret of the universe. However, the reality is not so simple. Taking the “three-body problem,” which is well-known due to Liu Cixin’s novel, as an example. On the surface, the “three-body” system seems very simple, consisting of only three entangled stars and one planet in between; simulating its trajectory does not seem difficult. However, once we try to use Newtonian mechanics to derive its position, we find that the resulting system of differential equations actually constitutes a chaotic system, making its motion trajectory very difficult to determine; a slight disturbance can lead to significant deviations. For this reason, in the “Three-Body” novel, even the three-body people, who are technologically advanced compared to Earth, cannot create an accurate calendar for ten thousand years.
In reality, there are far more complex problems than the “three-body system.” When studying these problems, people have to face the challenge of “dimensional explosion.”
For instance, predicting the trajectory of typhoons is a task that requires a very high amount of computation. Traditionally, people mainly rely on dynamic system models for prediction. This method constructs a large number of differential equations based on the physical laws of fluid dynamics and thermodynamics to simulate atmospheric motion and subsequently predict the direction of typhoons. Clearly, this dynamic system is very complex, requiring a large amount of computation for predictions and is very easily influenced by external disturbances. For this reason, even with the use of the most advanced supercomputers, predictions often go awry. In recent years, people have adjusted their prediction approach and started using AI models to predict typhoons, leading to a surge of related AI models. These models abandon the traditional physical model for predictions and instead use machine learning methods, significantly reducing computational burdens while effectively improving prediction accuracy. For example, the “WindWu” model can run on a single GPU computer and generate high-precision global forecast results for the next ten days in just 30 seconds. During the recent prediction of typhoon “Doksuri,” the trajectory error predicted by the “WindWu” model was far less than that of traditional models, thus making a significant contribution to combating the typhoon.
(4) Assisting Proofs
In some disciplines (such as mathematics), theoretical proofs of propositions are required during the research process. For a long time, people have tried to use computers to help them complete this challenging task. Their basic idea is: first, formalize a mathematical proposition, and then use a computer to provide a proof for the formalized proposition.
In reality, many mathematical propositions are expressed in natural language. For example, the famous “four-color problem” is to prove that “any map can be colored with four colors such that no two adjacent regions share the same color.” For a computer, this natural language is difficult to understand, and thus it cannot help people solve proof problems in natural language. Fortunately, mathematicians have established a formal axiomatic system for most branches of mathematics after long-term efforts. With the help of this axiomatic system, propositions expressed in natural language can be formulated as formal propositions composed of a series of logical judgments. Through specific encoding methods, computers can recognize these formal propositions, thus assisting people in proving them.
Taking the proof of the “four-color problem” as an example: historically, several versions of proof have existed for this famous problem. Although computers have been used as aids in each version of proof, the initial proofs were primarily derived by humans, with computers mainly providing computational support. In 2005, Georges Gonthier, a senior researcher at the University of Cambridge, provided a new generation of proof for the “four-color problem.” Unlike previous generations of proofs, Gonthier first transformed the problem into a series of formal propositions and then used an interactive assistant software called Coq to prove them. Since Coq completed a large number of the most complex proofs during the proof process, this process can be considered a machine proof in a certain sense.
It should be noted that although proof assistant software, including Coq, can help people complete many proof tasks, its automation is very low. Most of the time, human researchers still need to act as guides, helping convert natural propositions into formal propositions.
With the development of AI, people have begun to attempt using AI to solve this problem. For example, in 2022, a team of researchers from Google, Stanford University, and other institutions published a paper introducing the use of OpenAI Codex’s neural network for automatic formalization, demonstrating the feasibility of automatically translating non-formalized statements into formal statements using large language models. This year, the team proposed a complete set of AI-assisted proof methods called “Draft, Sketch, and Prove” (DSP), which suggests using large language models to first convert natural language propositions into formal propositions composed of a series of logical reasoning steps, and then using interactive theorem provers to prove these propositions. Of course, there are a series of intermediate conjectures between these steps. Therefore, at the end of the proof, intermediate conjectures also need to be proven through automatic verifiers. This way, the above work can be combined to form a complete formal proof.
(5) Assisting Writing
For scientific work, AI also makes an important contribution: assisting in writing. For many people, after completing research and obtaining relevant conclusions, writing them into a paper seems to be an easy task. However, the reality may not be so. In practice, many researchers are very enthusiastic about conducting experiments and running data but are quite resistant to writing papers, even considering spending time on wording as a waste of time. With the rise of generative AI represented by ChatGPT, these researchers have been saved. Now, after completing their research, they can directly give the relevant conclusions to ChatGPT and receive a well-structured paper in return. Clearly, this greatly reduces their workload and improves their efficiency.
Another Often Overlooked Contribution
It should be noted that in addition to the direct contributions mentioned above, AI has another impact that is often overlooked: reconstructing the relationship between industry, academia, and research, and promoting enterprises’ enthusiasm for investing in basic research. For our country, which faces being “choked” in certain fields by the West, this point may be particularly important.
According to the “China R&D Expenditure Report 2022,” the basic research expenditure in our country in 2022 was 195.1 billion yuan, with a basic research investment intensity of 6.3%. Although the intensity of investment in basic research in our country has been continuously increasing compared to history, it is still very low compared to foreign countries.
If we analyze basic research investment by implementing institutions, we will find that universities account for the highest proportion, making up 49.4% of total investment. The second highest is research and development institutions, accounting for 39.1%, while enterprises only account for 6.5% as implementing institutions. In contrast, in the United States, the proportion of basic research funding executed by enterprises is 32.4%, and in Japan, it is 47.07%. It is well known that funding for universities and research institutions mainly comes from government allocations, while research funding for enterprises mainly comes from their own investments. Therefore, these figures indicate that enterprises in our country are far less willing to invest in basic research compared to those in the US and Japan.
What is the reason for this situation? One important reason is that the cycle of basic research is too long, the risks are high, and the conversion rate is low, leading enterprises, which aim for profit maximization, to consider engaging in basic research as unprofitable. In developed countries, due to the establishment of a relatively complete ecosystem of industry-academia-research symbiosis, similar risks can be better shared among enterprises, governments, and research institutions, thus enterprises are more actively investing in basic research. In our country, however, the isolation between industry, academia, and research is still relatively high, making it difficult to have a similar risk-sharing mechanism.
Clearly, to solve the above problems, the fundamental solution lies in cultivating a healthy innovation ecosystem and promoting the integration of industry, academia, and research. However, this is a long-term process that cannot be achieved overnight. Nevertheless, even under conditions where the innovation ecosystem has not effectively improved, the application of AI can significantly enhance enterprises’ enthusiasm for investing in basic research. As analyzed earlier, with the assistance of AI, the cycle of basic research can be greatly shortened, and efficiency can be significantly improved. From an economic perspective, this actually increases the expected returns of basic research while reducing its failure risks. Therefore, basic research, which was originally considered unprofitable, could become a profitable business, leading to increased enthusiasm for investment from enterprises. In this way, the problem of insufficient investment in basic research can be effectively alleviated.
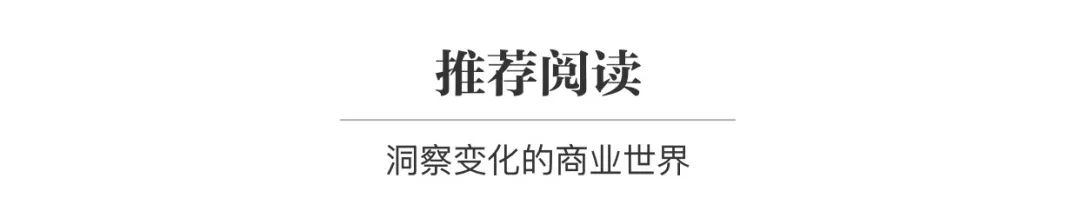
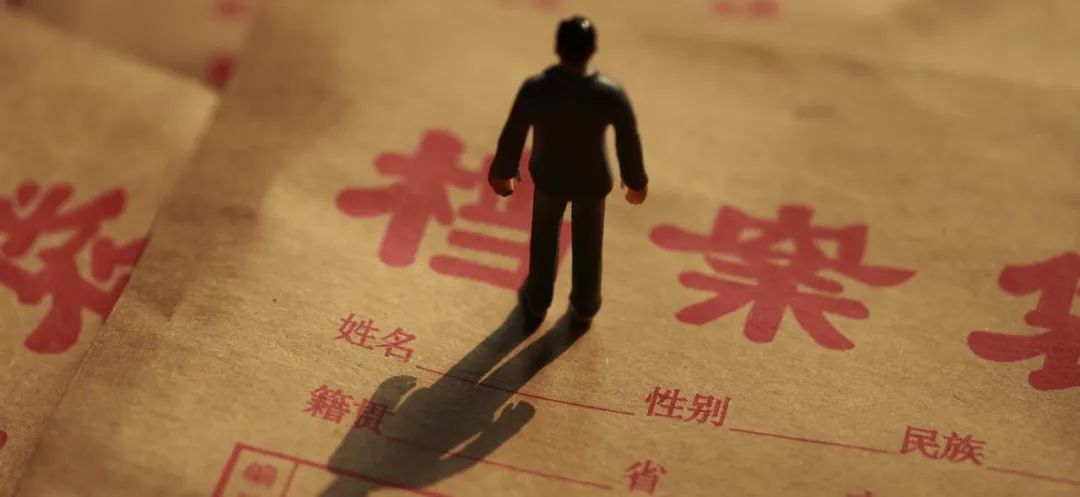
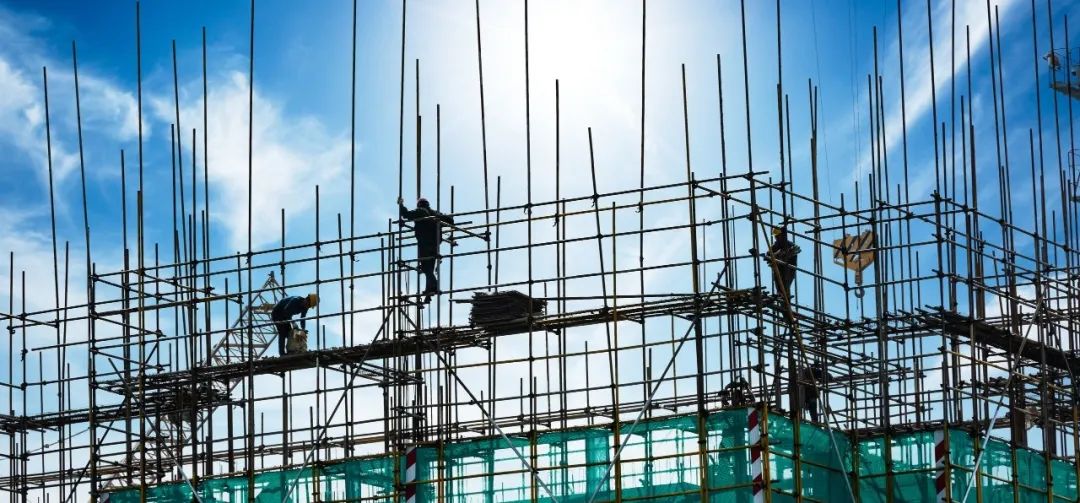
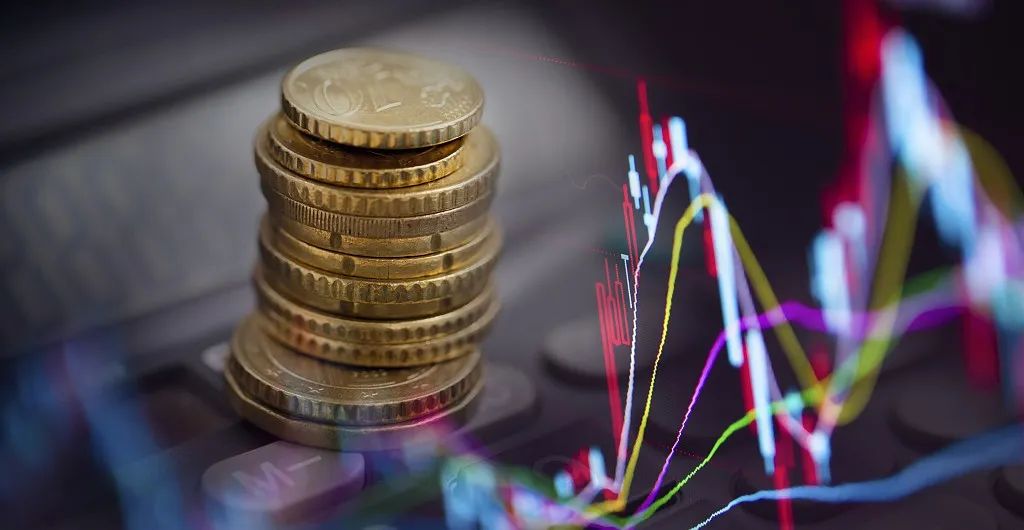
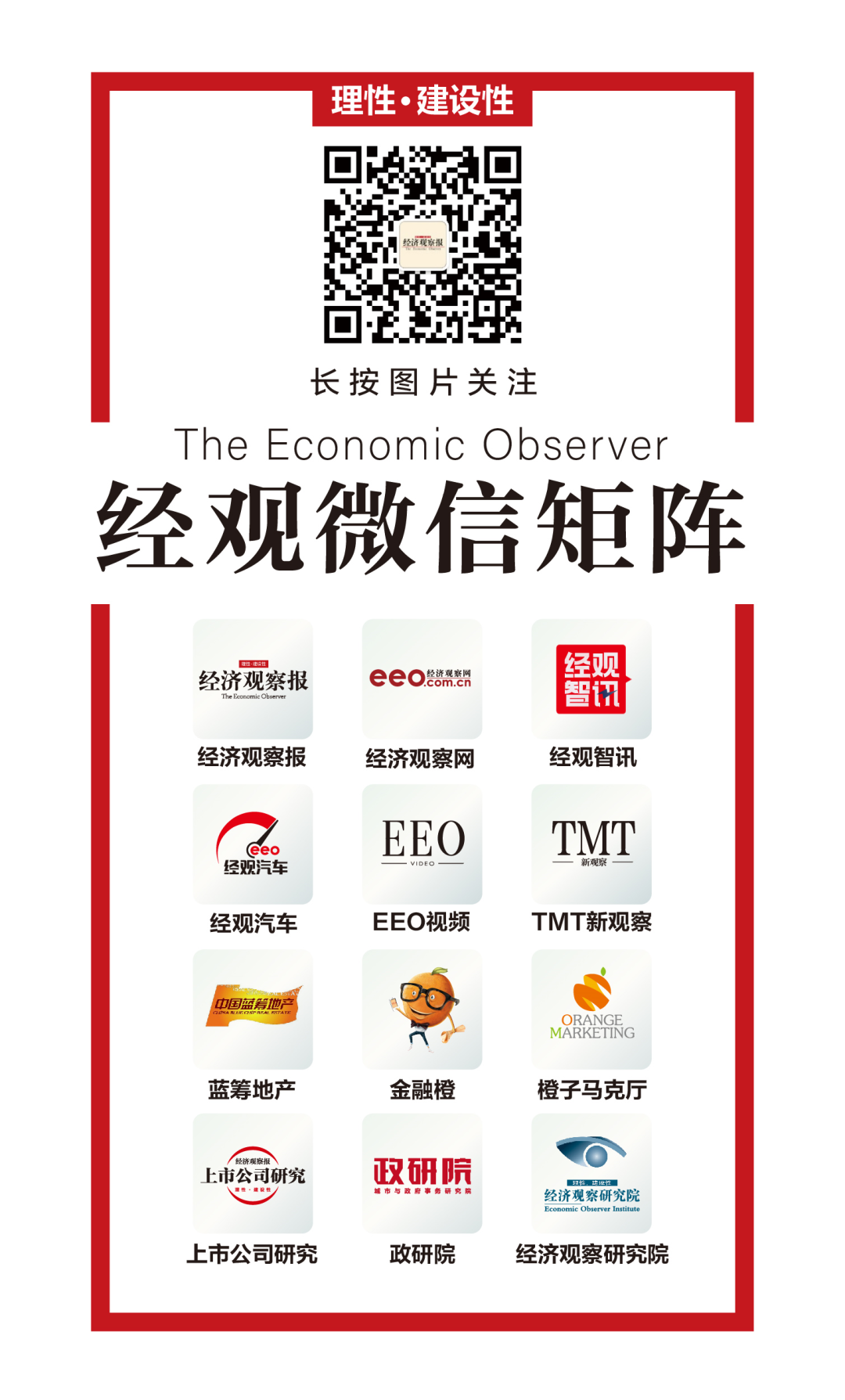
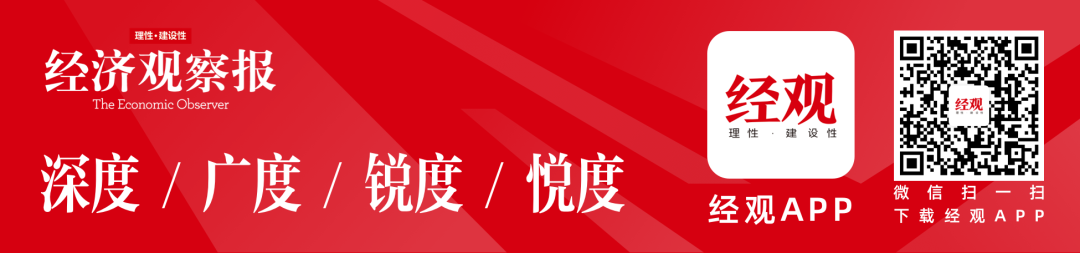