1
Background of AI Large Model Training
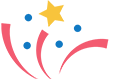
With the rapid development of technology, Artificial Intelligence (AI) has become an important driving force leading a new round of technological revolution and industrial transformation. Large Language Models (LLMs), as a revolutionary breakthrough in the AI field, are reshaping our understanding of intelligent interaction, knowledge management, content creation, and even the entire digital world at an unprecedented speed. In recent years, the continuous emergence of large models such as the GPT series and Sora not only demonstrates the immense potential of AI in natural language processing but also indicates that AI technology is about to enter a new era that is more complex, nuanced, and widely applicable.
In the context of AI becoming a global focus, the 2024 Chinese government work report has proposed the launch of the “AI +” initiative for the first time, and more favorable policies for AI and “AI +” are expected to follow. At the national level, promoting the “AI +” initiative will unleash countless opportunities.
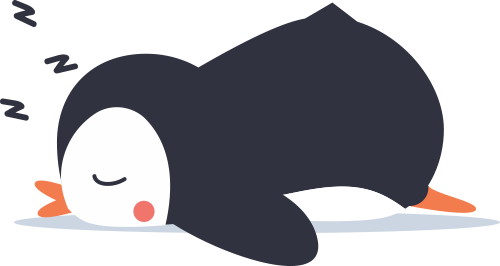
2
Introduction to AI Large Model Course
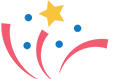
The AI Large Model Full-Stack Engineer Training Camp aims to help students systematically learn the design, development, optimization, and deployment of AI large models. Through a curriculum closely aligned with industry frontiers and a practical teaching model, students will deeply learn knowledge related to large models, acquire the ability to build and deploy large-scale AI applications, and gain the professional skills to stand out in the fiercely competitive AI industry, becoming pioneers and leaders in advancing future intelligent technology and opening a new chapter in their personal career.
3
Value of AI Large Model Training
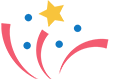
Master large model theoretical knowledge |
Understand self-attention mechanism, Transformer model, BERT model |
Master the principles and practical applications of GPT1, GPT2, GPT3, and ChatGPT |
Understand the technology stack and prompt engineering of LLM applications |
Understand domestic large model ChatGLM |
Understand the technical advantages of Sora large model |
Master language understanding and subtitle generation and its applications |
Master image generation and practical operations |
Understand application scenarios and potential analysis |
Engage in practical projects of large model enterprise commercial applications |
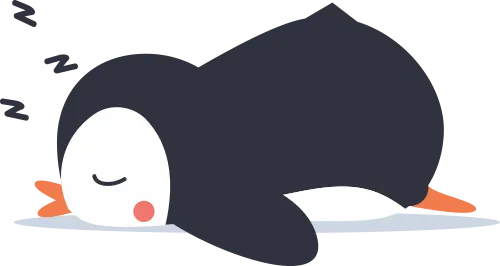
4
Training Methods for AI Large Model
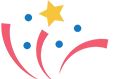
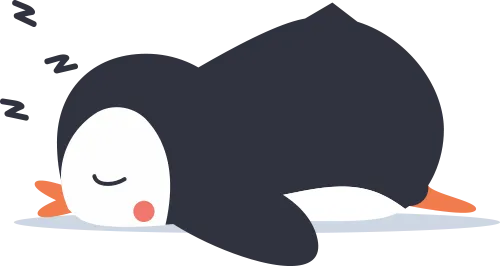
5
Features of AI Large Model Training
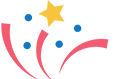
[Theoretical Lectures] PPT + Textbooks + Reference Materials
[Hands-on Practice] Scenarios + Cases + Simulation Environment
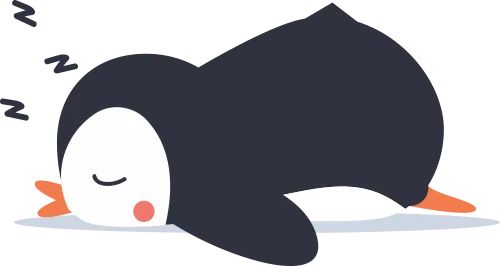
6
Training Location and Time for AI Large Model
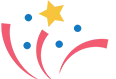
▶ Nanjing July 29-31
▶ Guangzhou September 22-24
▶ Beijing November 24-26
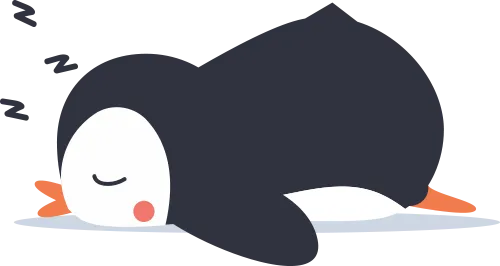
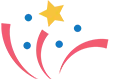
Target Audience for AI Large Model Training
▶ People working in the field of artificial intelligence
If you are currently working in related fields such as artificial intelligence, machine learning, or data analysis, or if you want to enter these fields, learning AI large model development will greatly help your career development.
▶ Software engineers and architects
These professionals can improve team R&D efficiency by learning AI large model development courses, understand how large models affect software architecture, and master new development paradigms based on large models.
▶ People with a strong interest in artificial intelligence
Practitioners who are deeply interested in artificial intelligence, machine learning, etc., want to gain in-depth knowledge and master relevant skills, and have a certain software development foundation.
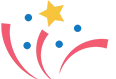
Advantages of Zhongpei IT Academy – AI Large Model Training
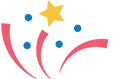
Zhongpei IT Academy – AI Large Model Course Outline
Training Topic |
Training Outline |
Day 1 |
|
Preparatory Knowledge Section 1: Large Model Theoretical Knowledge |
Introduction to Large Models: Origins and Development The GPT Model Family: From Beginning to Present Introduction to Large Models – Comparison of GPT_ChatGPT Practical Application of Large Models – Explanation of Two Learning Paths for Large Models The Three Core Technologies of Large Models: Model, Fine-tuning, and Development Framework OpenAI GPT Series Online Large Model Technology Ecosystem Introduction to OpenAI Text Models A, B, C, D Introduction to OpenAI Speech Model Whisper and Image Model DALL·E Introduction to the Strongest Embedding Model text-embedding-ada Global Open Source Large Model Performance Evaluation Rankings Introduction to Chinese Large Model Ecosystem and GLM 130B Model Introduction to ChatGLM Model and Deployment Threshold ChatGLM Open Source Ecosystem: Fine-tuning, Multi-modal, WebUI, etc. |
Preparatory Knowledge Section 2: Self-attention Mechanism, Transformer Model, BERT Model |
Basic Concepts of RNN-LSTM-GRU Encoder, Decoder Detailed Explanation of Self-attention Mechanism Transformer Mask Multi-Head Attention Positional Encoding Task-specific Input Transformation Unsupervised Pre-training, Supervised Fine-tuning Understanding BERT’s Approach Network Layer Design for BERT’s Downstream Tasks Training of BERT Inference of BERT Model in HuggingFace Contextual Learning Code and Case Practice: Code Implementation of Basic Q&A System Code Implementation of Deep Reading Comprehension Code Implementation of Paragraph Relevance |
Section 1: Principles and Practical Applications of GPT1, GPT2, GPT3, ChatGPT |
Supervised Fine-tuning (SFT) Model Instruction Learning and Prompt Learning Simple Prompts, Few-shot Prompts, User-based Prompts Instruction Fine-tuning Detailed Explanation of RLLHF Technology (Learning from Human Feedback) Training Reward Model (RM) Using Aggregated Q&A Data Reinforcement Learning Fine-tuning, PPO, InstructGPT Following User Intent Using Reinforcement Learning Scheme Instruct Learning vs. Prompt Learning ChatGPT Adds Chat Attributes New Paradigms for AI Systems Technical Relationships Between GPT1-GPT2-GPT3-InstructGPT-ChatGPT Code and Case Practice: Create Your Personal Chat Assistant Using ChatGPT Demonstrate Prompt Techniques, Translator, JavaScript Console, Excel Sheet Custom ChatGPT Web Application |
Section 2: Embedding Model Practical Applications |
Positioning of Embedding Technology in the Wave of Large Model Technology Introduction to Embedding Technology From One-hot to Embedding Embedding Text Measurement and Similarity Calculation OpenAI Embedding Model and Open Source Embedding Framework Introduction to Two Generations of OpenAI Embedding Models Detailed Method for Calling text-embedding-ada-002 Model Detailed Parameters and Optimization Strategies for text-embedding-ada-002 Model Feature Encoding Using Embedding Visualization and Result Analysis of Embedding Results [Practice] Complete Supervised Prediction Using Embedding Feature Encoding [Practice] Cold Start of Recommendation System Using Embedding [Practice] Zero-shot Classification and Text Search Using Embedding Fine-tuning and Optimization of Embedding Model Structure Optimizing Embedding Results Using CNN [Enterprise-level Practice] Efficient Matching of Massive Texts Using Embedding |
Section 3: LLM Application Technology Stack and Prompt Engineering |
Design Patterns: Contextual Learning Data Preprocessing/Embedding Prompt Building/Retrieval Prompt Execution/Inferences Data Preprocessing/Embedding Open Source Systems such as Weaviate, Vespa, and Qdrant Local Vector Management Libraries such as Chroma and Faiss OLTP Extensions such as pgvector Prompt Building/Retrieval Prompt Execution/Inferences Emerging Large Language Model (LLM) Technology Stack Data Preprocessing Pipeline Embedding Endpoint + Vector Store LLM Endpoints LLM Programming Framework Main Functions and Modules of LangChain Prompts: Includes Prompt Management, Prompt Optimization, and Prompt Serialization LLMs: Includes General Interfaces for All LLMs and Common LLM Tools Document Loaders: Includes Standard Interfaces for Loading Documents and Integration with Various Text Data Sources Utils: Language Models Interacting with Other Knowledge or Computational Sources Python REPLs, Embeddings, Search Engines, etc. Common Tools Provided by LangChain Indexes: Language Models Combined with Custom Text Data Agents: Action Execution, Observing Results, Standard Interfaces for LangChain Agents, Available Agents, End-to-End Agent Examples Chat: Chat Models Processing Messages Code and Case Practice: Using LLMs Designing and Using Prompts |
Day 2 |
|
Section 4: Using LangChain |
General Ideas and Methods for Building Vertical Domain Large Models (1) Large Model + Knowledge Base (2) PEFT (Parameter-Efficient Fine-Tuning) (3) Full Fine-Tuning (4) Customization Starting from Pre-training Introduction to LangChain Learning LangChain Modules – LLMs and Prompts LangChain Chains Module LangChain Agents Module LangChain Callback Module Embedding Custom Knowledge Base Handling Knowledge Conflicts Methods for Vectorized Calculations Document Loader Module Design of Vector Database Q&A Research and Analysis of LangChain Competitors Dust.tt/Semantic-kernel/Fixie.ai/Cognosis/GPT-Index Introduction to LlamaIndex LlamaIndex Index Hands-on Implementation of Knowledge Q&A System Code and Case Practice: Hands-on Implementation of Knowledge Q&A Robot LangChain Text Summarization PDF Text Reading Q&A |
Section 5: Domestic Large Model ChatGLM |
Introduction to the New Generation GLM-4 Model Usage of Zhizhu AI Mass Open Platform 03GLM Online Large Model Ecosystem Introduction to CharGLM, CogView, Embedding Models Usage of GLM Online Knowledge Base and Model Billing Instructions How to Obtain GLM Model API Key and Account Management Calling GLM Model SDK and Three Running Methods Detailed Explanation of GLM4 Calling Function Parameters GLM4 Message Format and Identity Setting Method GLM4 Tools External Tool Calling Method GLM4 Function Calling Function Packaging and GLM4 Accessing Online Knowledge Base Retrieval Process GLM4 Accessing Internet web_search Method [Practice] Building Automatic Data Analysis Agent Based on GLM4 [Practice] Natural Language Programming Practice Based on GLM4 [Practice] User Intent Recognition Based on GLM4 Function Call [Practice] Long Text Reading and Optimization Based on GLM4 |
Section 6: Technical Advantages of Sora Large Model |
What is Sora Sora Video Generation Capability Unique Features of Sora Technology Unified Visual Data Representation Video Compression Network Diffusion Transformer Model Video Compression and Latent Space |
Section 7: Language Understanding and Subtitle Generation and Its Applications |
Using Images and Videos as Prompts Animated DALL·E Images Extended Generated Videos Video-to-Video Editing Connecting Videos Subtitle Generation Re-Subtitle Technology Applications of GPT Technology |
Day 3 |
|
Section 8: Image Generation and Practical Applications |
Emerging Simulation Functions Long-term Continuity and Object Permanence Consistency of Roles and Objects Coherence of Video Content Interacting with the World Simple Impact Behavior Simulation Simulating the Digital World |
Section 9: Application Scenarios and Potential Analysis |
Film and Entertainment Industry Game Development Education and Training Advertising and Marketing Scientific Research and Simulation Generating Data Case Study of Graduate Job Classification Prompt Functions Function Calling Application of Prompt Engineering in Models AI Chat Social Applications CallAnnie NewBing AI-Assisted Article Creation Swift AI Writing ChibiAI AI Office Intelligent Assistant GrammaAI Creative Work in the AI Art Field |
Section 10: Practical Explanation of Large Model Enterprise Commercial Projects |
Using Large Models to Implement Recommendation Systems (Commercial Case) Using Large Models to Implement Online Car Sales Systems Enterprise Natural Language SQL Generation (Used in Internal Systems) |
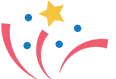
Instructor Team of Zhongpei IT Academy – AI Large Model
Java web, Senior Architect, Langchain Developer, 11 years of IT development experience, 5 years of IT architecture and management experience. Proficient in the design and technical development of large distributed Internet application architectures. Particularly knowledgeable about large-scale distributed architecture, microservices architecture, cloud computing and containerization technology, integrated development and operations, application system security and architecture design, massive data processing, and big data, especially with rich architecture and implementation experience in high-concurrency systems. Skilled in Java, software architecture, microservices, software engineering, and R&D team management, currently serving as a big data architect for a listed group company, primarily providing security services for the country and abroad.
Led the company’s AI large model development project, utilizing AI to implement the company’s intelligent SQL project, and developing promotion systems and sales management systems using AI.
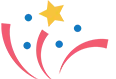
Registration Method for Zhongpei IT Academy – AI Large Model