Follow our public account to discover the beauty of CV technology
This article shares the ACL 2024 paper PreFLMR: Scaling Up Fine-Grained Late-Interaction Multi-modal Retrievers
, open-sourced by the Cambridge University team, empowering multi-modal large model RAG applications, and is the first pre-trained general multi-modal late-interaction knowledge retriever.
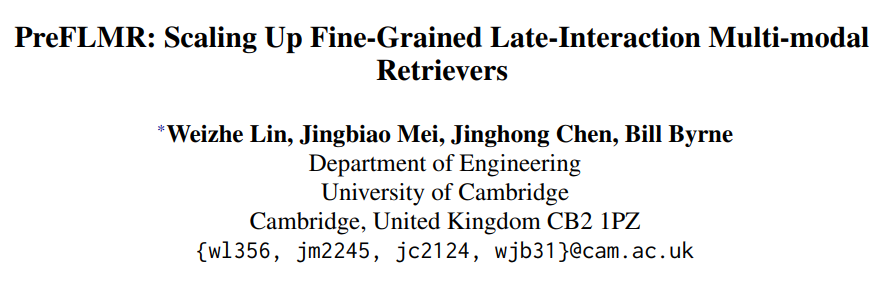
-
Paper link: https://arxiv.org/abs/2402.08327 -
Project homepage: https://preflmr.github.io/
Introduction
The PreFLMR model is a general pre-trained multi-modal knowledge retriever that can be used to build multi-modal RAG applications. The model is based on the Fine-grained Late-interaction Multi-modal Retriever (FLMR) published at NeurIPS 2023, with model improvements and large-scale pre-training on M2KR. Currently, training data, pre-trained weights, fine-tuning code, etc., have all been open-sourced. This model has been successfully implemented in enterprise-level RAG applications. The author team will participate in ACL 2024 from August 10 to 17 and welcomes academic exchanges and business collaborations.
Background
Despite the strong general image-text understanding capabilities of multi-modal large models (such as GPT4-Vision, Gemini, etc.), they still perform inadequately when answering questions that require specialized knowledge. Even GPT4-Vision cannot answer knowledge-intensive questions (as shown in Figure 1), which has become a bottleneck for many enterprise-level applications.
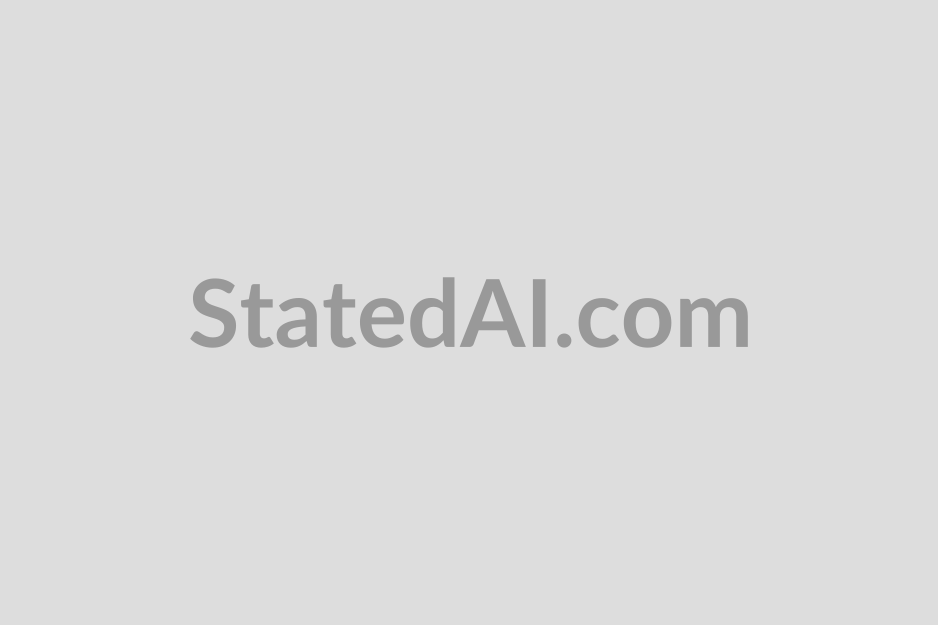
To address this issue, Retrieval-Augmented Generation (RAG) provides a simple and effective solution for making multi-modal large models become