Introduction

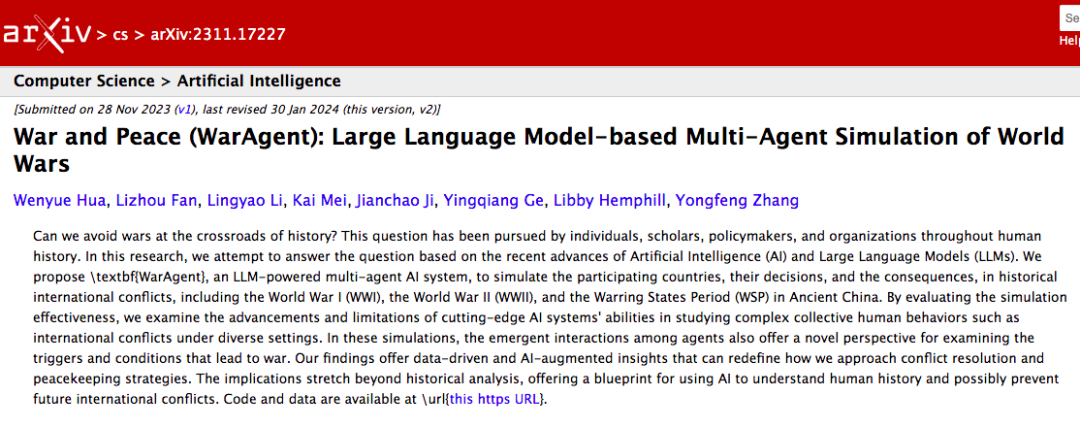
Paper Title:
War and Peace (WarAgent): Large Language Model-based Multi-Agent Simulation of World Wars
Paper Address::
https://arxiv.org/abs/2311.17227
1. Introduction
2. Related Background Work
3. WarAgents Simulation Setup
4. WarAgent Architecture
5. Agent Interaction Design
6. Experimental Design
7. Simulation Effect Evaluation
8. Assessment of War Triggers
9. Discussion of War Inevitability
10. Discussion and Outlook
1. Introduction
1. Introduction
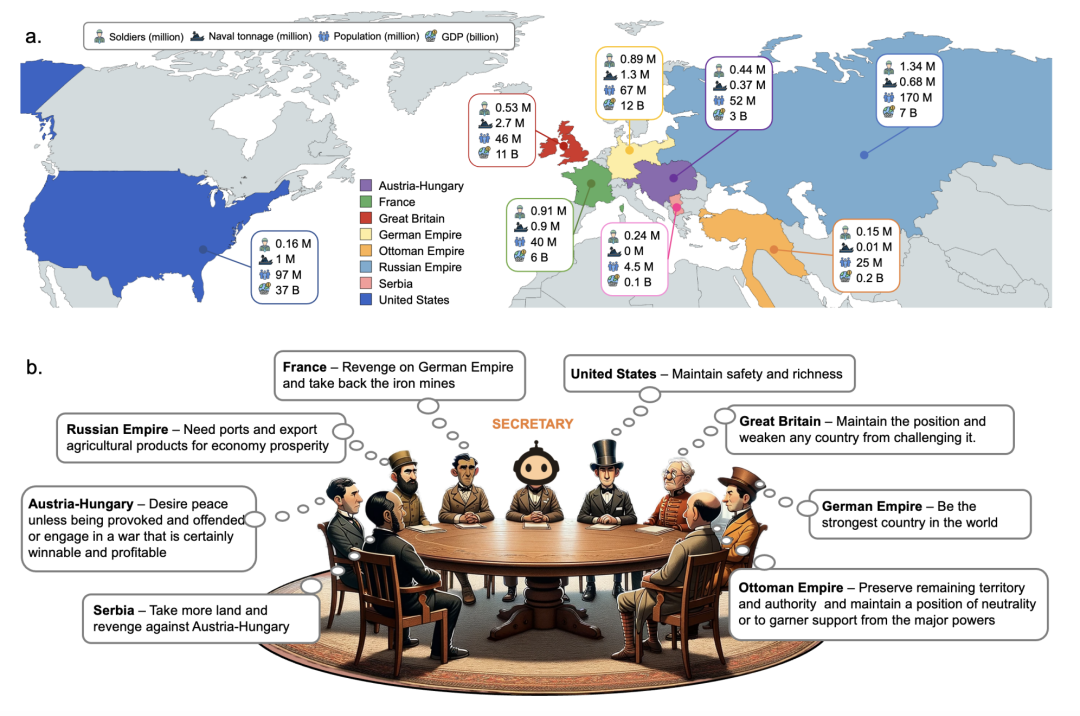
2. Related Background Work
2. Related Background Work
3. WarAgents Simulation Setup
3. WarAgents Simulation Setup
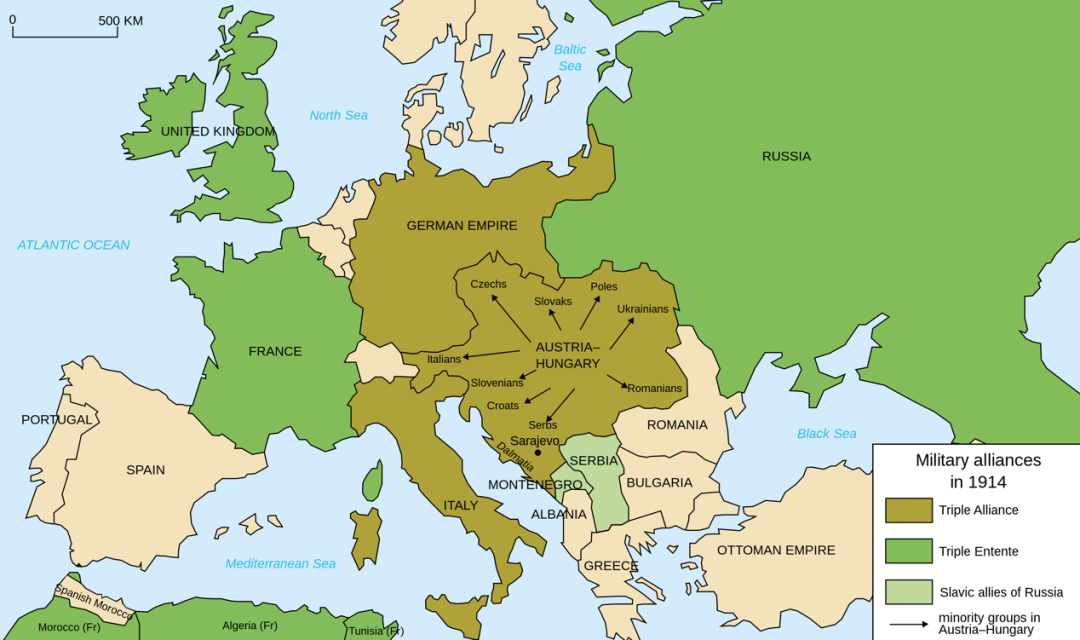
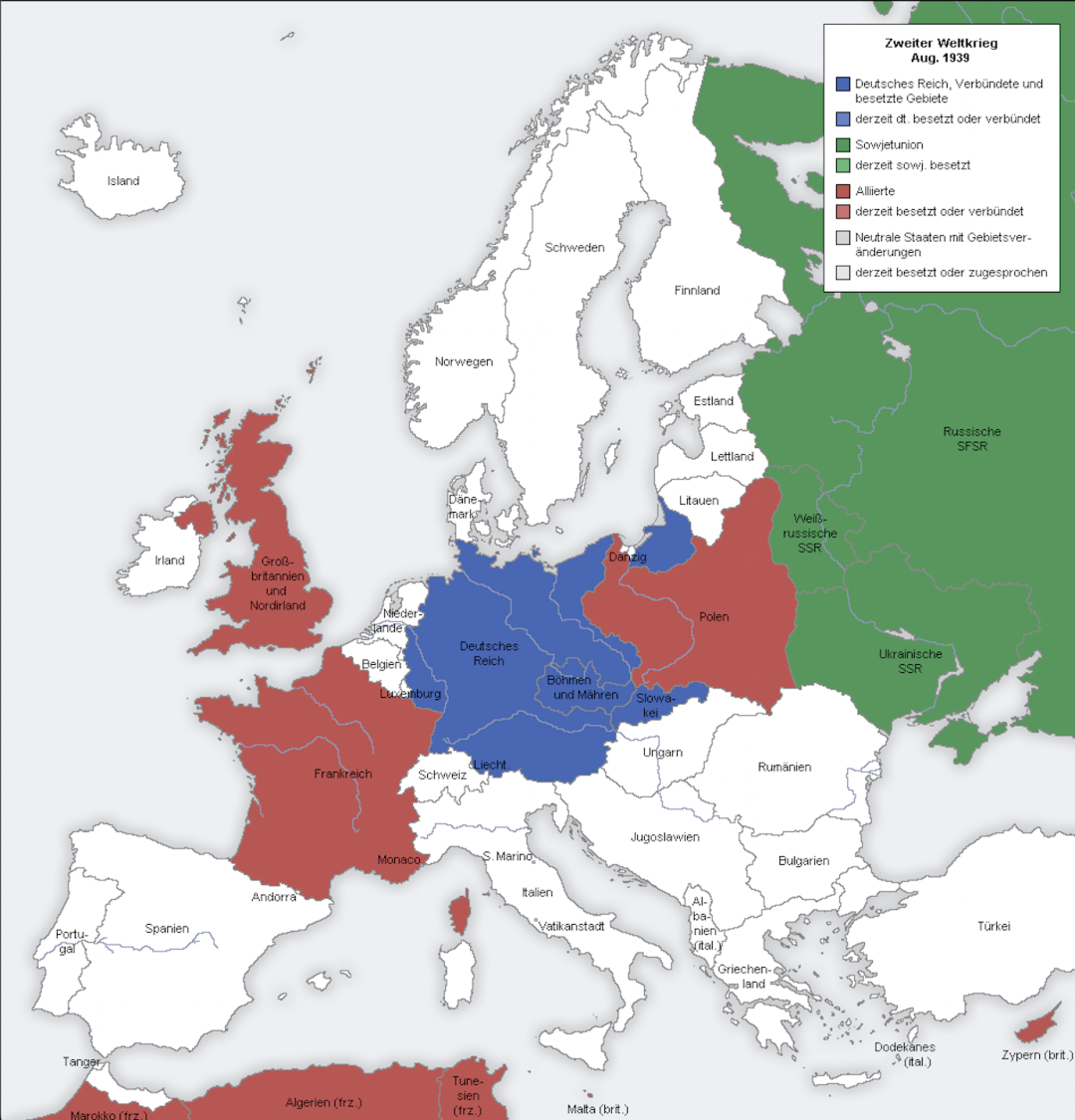
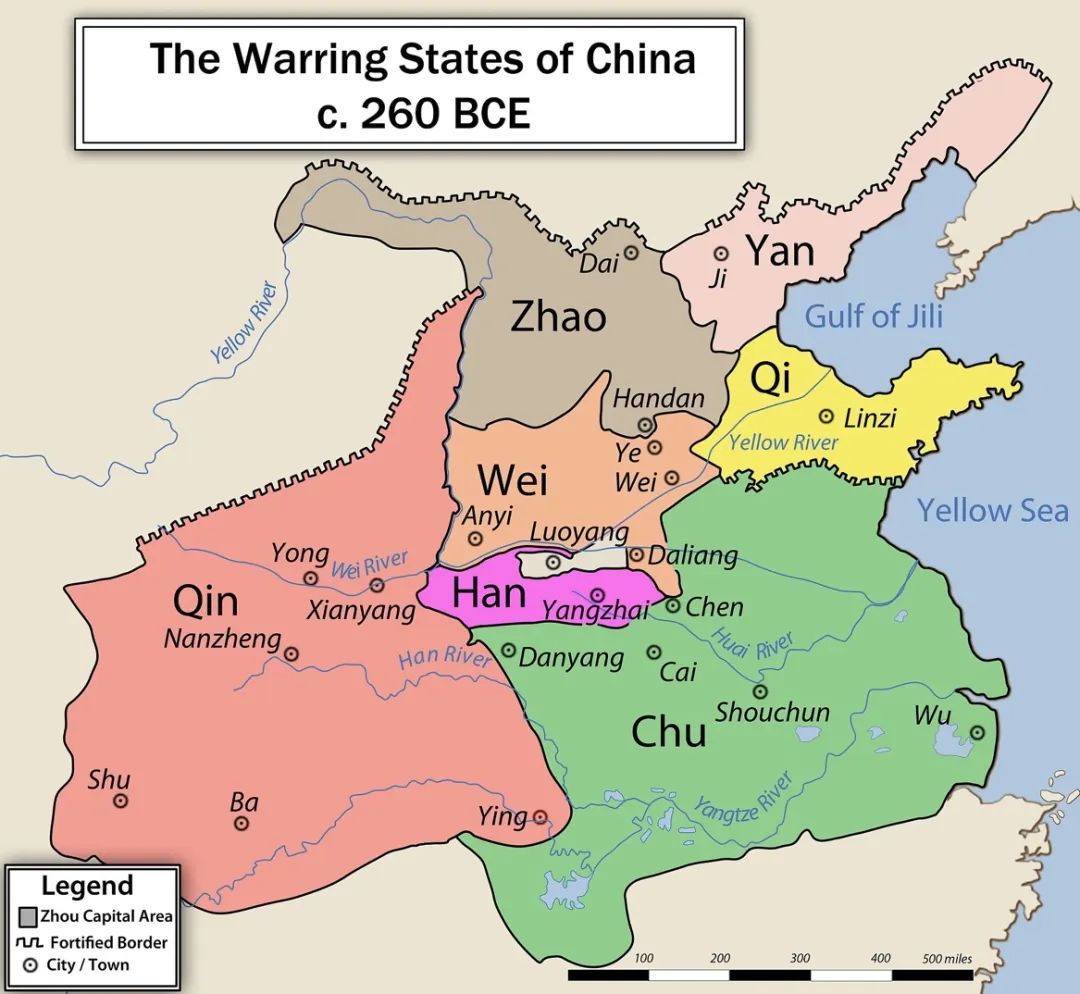
## Overview of Britain # British Leadership (1) Britain is a constitutional monarchy with significant democratic institutions, characterized by pragmatism and calmness in governance. # British Military Capability (1) Active military size: 530,000 soldiers. (2) Navy tonnage: 2.7 million, the world’s most powerful navy, with total tonnage exceeding that of the second and third strongest navies combined. # British Resources (1) Location: An island located west of France, Germany, Austria-Hungary, and Russia, with numerous colonies. (2) Population: 46 million. (3) Gross Domestic Product: 110 billion, accounting for 13.6% of the global total. (4) Geography: Characterized by rolling hills, green fields, and rugged coastlines, influenced by a maritime climate that keeps it moist year-round. (5) Climate: Mild maritime climate, often cloudy, rainy, and cool. # British Historical Background (1) Britain is one of the most influential countries in the world, with the most colonies. # Key Policies (1) As the most powerful country at the time, Britain’s goal was to maintain its leading position and weaken any potential challengers, such as Germany. (2) Britain established a policy: whenever Germany built a battleship, Britain would build two. # Public Morale |
4. WarAgent Architecture
4. WarAgent Architecture
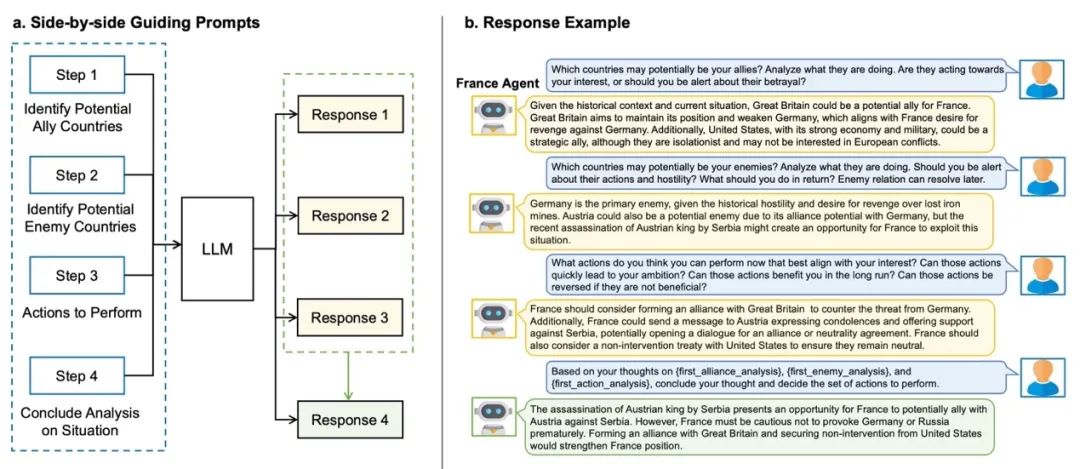
Figure 5 Guiding Prompts. (a) Multi-step guiding prompt design diagram. (b) Example of interaction between the French agent and the customized GPT-4 model.
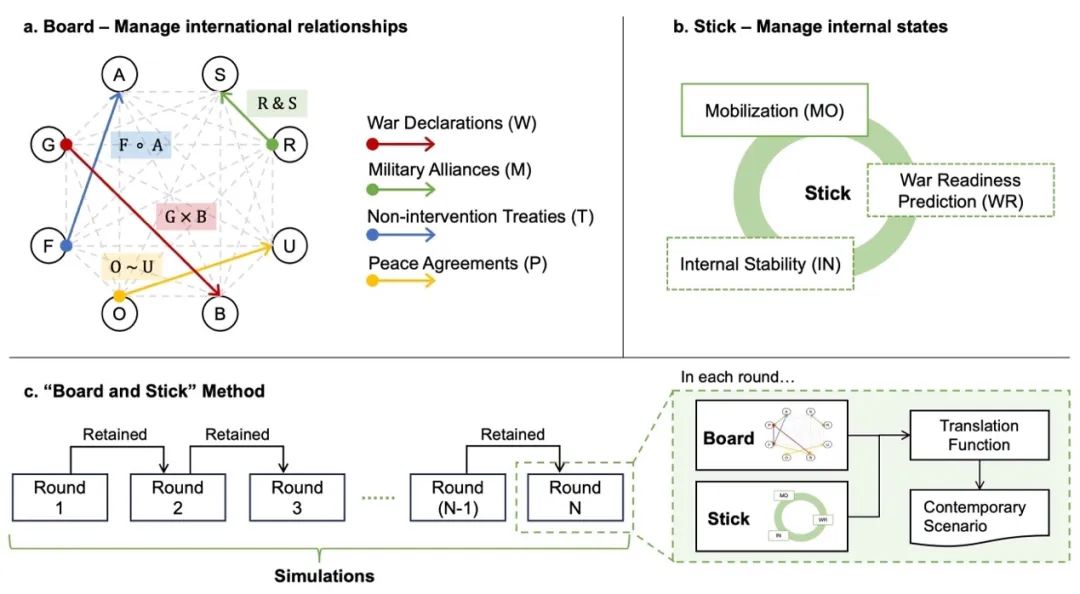
Figure 6 Bulletin Board and Notepad Design. (a) Bulletin board design. (b) Notepad design (internal stability and war readiness prediction will be discussed in future work). (c) Bulletin board and notepad methods during experiments.
5. Agent Interaction Design
5. Agent Interaction Design
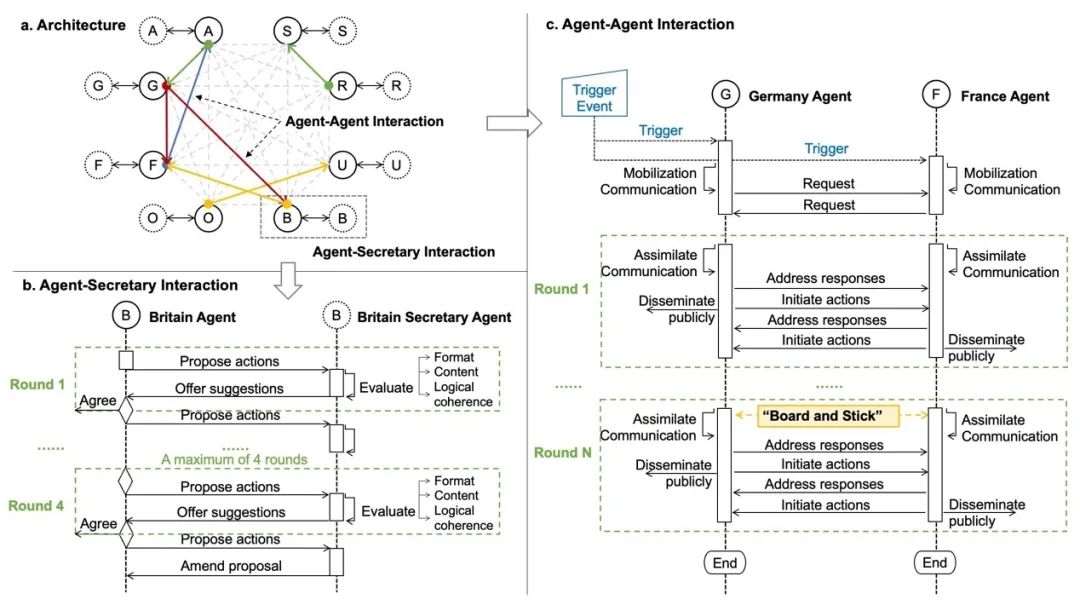
Figure 7 Agent Interaction Design. (a) WarAgent architecture. (b) Interaction between national agents and secretary agents. (c) Interaction between national agents.
## Trigger: Serbia sends assassins to kill Archduke Franz Ferdinand of Austria-Hungary. # British Response to the Triggering Event: To France: Britain decides to propose a military alliance to France. To Russia: Britain decides to propose a military alliance to Russia. |
# Collected Requests to Britain: From France: France chooses to send a message to Britain stating: Given the recent developments and our mutual interests in countering German aggression, we propose a dialogue to explore potential cooperation opportunities. From the Ottoman Empire: The Ottoman Empire chooses to propose a non-interference treaty to Britain. # Britain’s Responses to the Requests: To France: Britain chooses to send a message to France stating: We welcome the dialogue to explore potential cooperation against a common threat. To the Ottoman Empire: Britain chooses to accept the non-interference treaty proposed by the Ottoman Empire. |
|
6. Experimental Design
6. Experimental Design
|
|
|
|
|
|
|
|
|
|
|
|
7. Simulation Effect Evaluation
7. Simulation Effect Evaluation
France # Round 1 United States # Round 1 To Austria-Hungary: The United States proposes a non-interference treaty with Austria-Hungary. |
|
8. Assessment of War Triggers
8. Assessment of War Triggers
|
|
|
9. Discussion of War Inevitability
9. Discussion of War Inevitability
10. Discussion and Outlook
10. Discussion and Outlook
This week’s live broadcast of the Large Language Model and Multi-Agent System Reading Group:
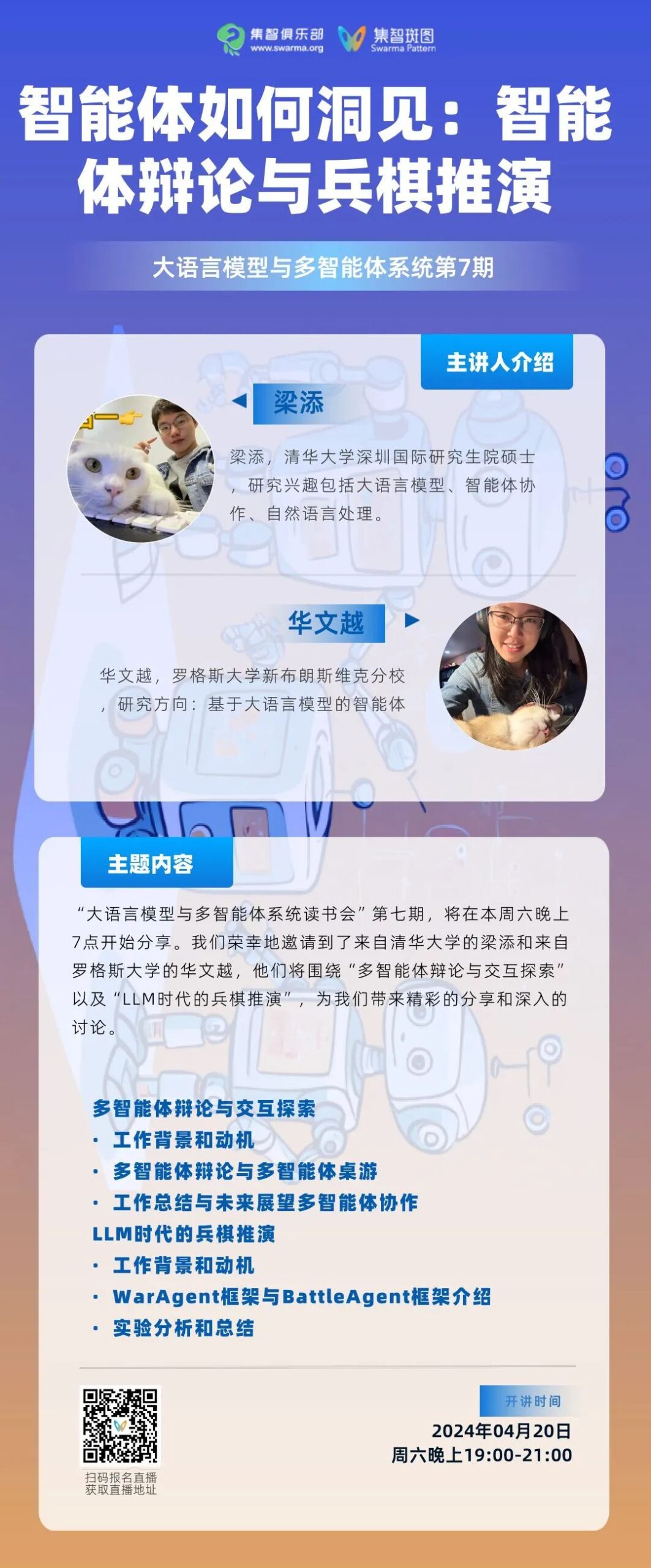
Large Language Model and Multi-Agent System Reading Group
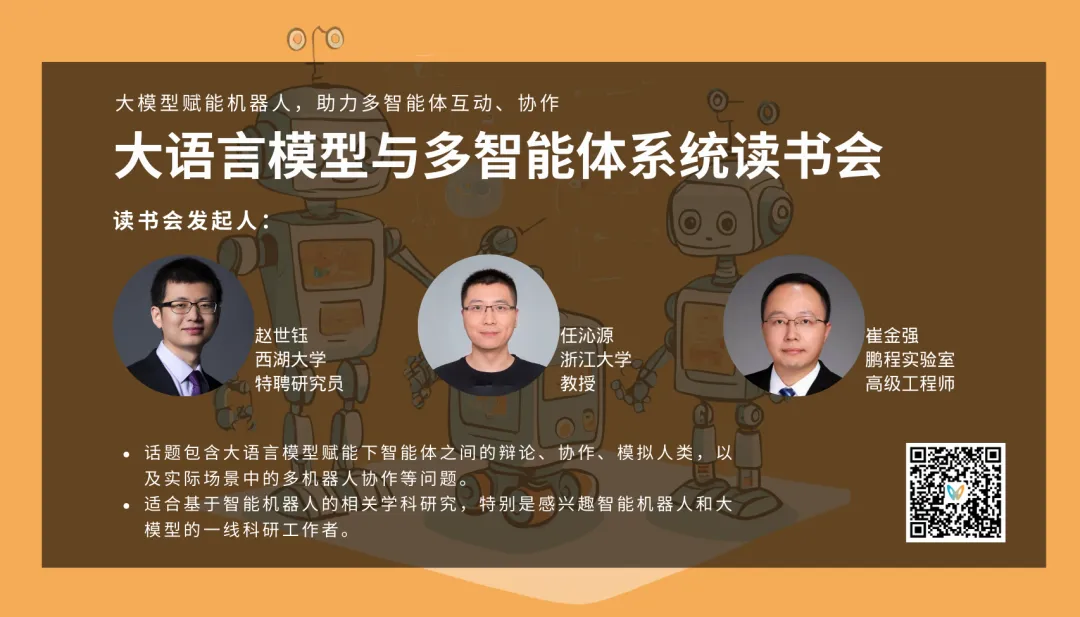
Recommended Reading