Author: Sienna
Reviewed by: Los
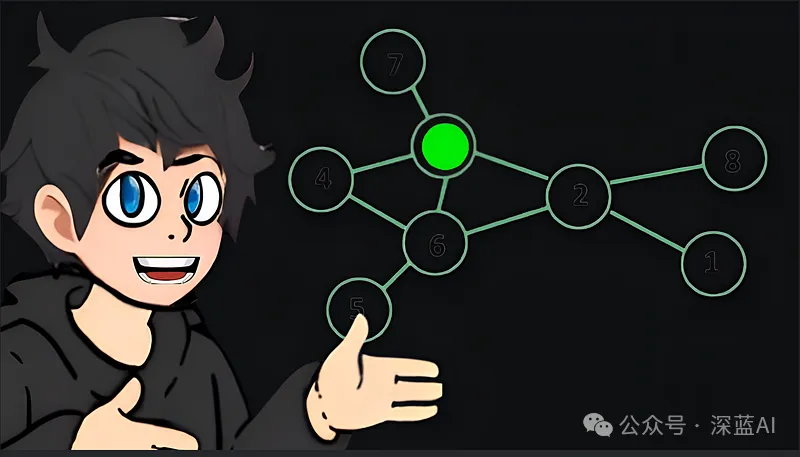

This article will delve into the highly regarded open-source LLM project on GitHub, providing a detailed guide on how to utilize the Graph RAG (Retriever-Augmented Generator with Graph) technology, in conjunction with the Langchain framework and the capabilities of GPT-4 (or similar LLM models), to build a local chatbot that can provide accurate and coherent information. The article not only offers comprehensive step-by-step instructions and code examples but also covers the entire process from installing Python libraries, configuring the Neo4j graph database, to using the OpenAI API for natural language processing and extracting structured information. Additionally, we will explore how Graph RAG efficiently manages complex information relationships through graph database technology, significantly enhancing the accuracy of search and response. Finally, we will demonstrate the application of GraphCypherQAChain in the knowledge graph through practical queries and compare the advantages of Graph RAG with vector databases.
■2.1 Definition and Advantages
Graph RAGs, or graph-represented retrieval-augmented generators, are an innovative information retrieval and generation technology. It utilizes graph databases to construct a network of associations between information, allowing even abstract or complex queries to quickly find relevant and accurate answers based on the graphical structure. Compared to traditional vector RAGs, Graph RAG can understand the contextual relationships between information more deeply, thus providing more human-like responses.
■2.2 Working Principle
The working mechanism of Graph RAG combines the efficient querying capabilities of graph databases with the semantic understanding capabilities of RAG. In Graph RAG, each piece of information is treated as a node in the graph, while relationships between information are represented by edges. This structure not only intuitively presents the inherent connections of the data but also allows the system to flexibly traverse the graph based on query needs to find the most relevant nodes and paths.
■2.3 Example Analysis
For example, when using Graph RAG to handle the abstract query “What are Sakura’s characteristics?” from “Naruto,” the system quickly locates the “Sakura” node based on existing graph database information and retrieves related information along the edges connected to it (such as “long hair,” “light green eyes,” etc.). Thus, even if the original data does not directly mention the word “characteristics,” Graph RAG can infer an appropriate answer through the graphical structure, achieving semantic accuracy.
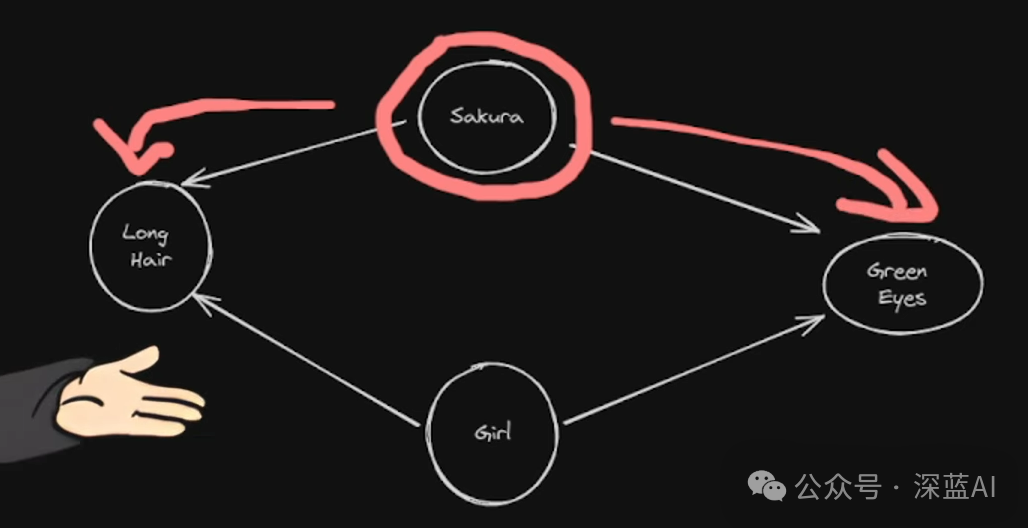
Graph RAG focuses on leveraging graph databases to model and query complex relationships between entities. It effectively represents and stores the associations between information by constructing a graphical structure of nodes and edges. This structure not only supports graph-based operations, such as traversing relationships, finding paths, and identifying patterns, but also exhibits powerful capabilities when dealing with data rich in context and relationships. Graph RAG is particularly suitable for scenarios that require a deep understanding of relationships between information, complex reasoning, or generating structured answers.
Vector databases, on the other hand, focus on efficiently executing similarity searches and nearest neighbor queries. They utilize vectorization techniques to convert unstructured data (such as text, images, etc.) into vectors in high-dimensional space, allowing for fast retrieval of the most similar vectors to a given query vector through specialized indexing techniques and algorithms. Vector databases excel in handling large datasets, performing rapid similarity matching, or recommendation systems. However, they may fall short when dealing with tasks that require a deep understanding of semantic relationships and complex reasoning.
Building a Graph RAG system in Python typically requires integrating multiple libraries and frameworks, such as LangChain, Neo4j, and OpenAI. Below is a simplified example demonstrating how to use these tools to construct a basic Graph RAG system.
First, ensure that the necessary Python libraries, including LangChain and its dependencies, are installed. Then, create an instance of the Neo4j graph database and configure the relevant connection information. Next, use the OpenAI API to extract structured information from natural language and convert it into nodes and edges in the graph database.
import os
from langchain.chat_models import ChatOpenAI
from langchain_community.graphs import Neo4jGraph
from neo4j import GraphDatabase
# Set OpenAI API key
os.environ["OPENAI_API_KEY"] = 'Your_API_Key'
# Create an instance of the OpenAI chat model
model = ChatOpenAI()
# Configure Neo4j database connection
url = "bolt://localhost:7687"
username = "neo4j"
password = "your_password"
driver = GraphDatabase.driver(url, auth=(username, password))
graph = Neo4jGraph(driver=driver)
print(graph)
OpenAI’s functions demonstrate its exceptional ability to extract structured information from natural language text. The core idea behind this functionality is to guide the LLM to generate a well-structured JSON object filled with detailed data obtained from processing the text. To represent and construct the knowledge graph, several core data structure classes have been meticulously designed within the project, each carrying different attributes: Property class (for defining specific key-value pairs), Node class (representing entities in the knowledge graph), Relationship class (defining connections between nodes), and KnowledgeGraph class (serving as a container for the entire knowledge graph).
# Import necessary libraries and base classes
from langchain_community.graphs.graph_document import (
Node as BaseNode, # Import BaseNode class and rename it to Node to avoid conflict with the Node class below
Relationship as BaseRelationship,
GraphDocument,
)
from langchain.schema import Document
from typing import List, Dict, Any, Optional
from langchain_core.pydantic_v1 import BaseModel, Field
# Define Property class for storing key-value pairs
class Property(BaseModel):
"""Represents a single property, containing key and value"""
key: str = Field(..., description="The key of the property")
value: str = Field(..., description="The value of the property")
# Define Node class, inheriting from BaseNode and adding a list of properties
class Node(BaseNode): # If BaseNode already has enough properties, this inheritance may not be necessary or may need to be adjusted based on actual situation
"""Represents a node in the knowledge graph, can contain multiple properties"""
properties: Optional[List[Property]] = Field(
None, description="List of properties for the node"
)
# Define Relationship class, inheriting from BaseRelationship and adding a list of properties
class Relationship(BaseRelationship):
"""Represents relationships between nodes in the knowledge graph, can contain multiple properties"""
properties: Optional[List[Property]] = Field(
None, description="List of properties for the relationship"
)
# Define KnowledgeGraph class, as a container for the entire knowledge graph
class KnowledgeGraph(BaseModel):
"""Used to generate a knowledge graph containing entities and relationships"""
nodes: List[Node] = Field(
..., description="List of all nodes in the knowledge graph"
)
rels: List[Relationship] = Field(
..., description="List of all relationships in the knowledge graph"
)
To overcome API limitations, property values need to be reconstructed as a list of Property class objects, abandoning traditional dictionary structures. Given the API's restriction of only passing a single object, a class named KnowledgeGraph is designed to merge nodes and relationships into a whole, achieving efficient data encapsulation.
←Swipe left and right to view the complete code→
Next, we define a series of utility functions to optimize and simplify the operations of nodes and relationships in the knowledge graph.
●format_property_key:This function is responsible for formatting the property key, ensuring that the key naming conforms to specific style requirements.
●props_to_dict:This function converts the list of properties into dictionary format for subsequent processing and storage.
●map_to_base_node:This function maps custom node objects to the base class BaseNode, ensuring consistency and compatibility of node data.
●map_to_base_relationship:Similar to map_to_base_node, this function is responsible for mapping custom relationship objects to the base class BaseRelationship.
def format_property_key(s: str) -> str:
"""
Format the property key, making the first letter lowercase and capitalizing the first letter of other words.
Args:
s (str): The original property key string.
Returns:
str: The formatted property key string.
"""
words = s.split()
if not words:
return s
first_word = words[0].lower()
capitalized_words = [word.capitalize() for word in words[1:]]
return "".join([first_word] + capitalized_words)
def props_to_dict(props) -> dict:
"""
Convert the list of properties into a dictionary.
Args:
props (List[Property]): List of properties.
Returns:
dict: Property dictionary.
"""
properties = {}
if not props:
return properties
for p in props:
properties[format_property_key(p.key)] = p.value
return properties
def map_to_base_node(node: Node) -> BaseNode:
"""
Map custom nodes to the base node class.
Args:
node (Node): Custom node object.
Returns:
BaseNode: Mapped base node object.
"""
properties = props_to_dict(node.properties) if node.properties else {}
# Generate name property for Cypher statements
properties["name"] = node.id.title()
return BaseNode(
id=node.id.title(),
type=node.type.capitalize(),
properties=properties
)
def map_to_base_relationship(rel: Relationship) -> BaseRelationship:
"""
Map custom relationships to the base relationship class.
Args:
rel (Relationship): Custom relationship object.
Returns:
BaseRelationship: Mapped base relationship object.
"""
source = map_to_base_node(rel.source)
target = map_to_base_node(rel.target)
properties = props_to_dict(rel.properties) if rel.properties else {}
return BaseRelationship(
source=source,
target=target,
type=rel.type,
properties=properties
)
Define a function named get_extraction_chain, which aims to build a chain specifically for accurately extracting knowledge graphs from text data. This function provides two flexible optional parameters: allowed_nodes and allowed_rels, which serve to specify the allowed node labels and relationship types, respectively.
By explicitly defining these parameters, we can significantly enhance the accuracy and specificity of information extraction, effectively reducing ambiguity and vagueness as described in the Sakura example. Although the prompt construction may be complex, we will attempt to embed disambiguation logic, but in certain or specific scenarios, directly specifying allowed_nodes and allowed_rels will become a key step in optimizing extraction results.
Ultimately, the get_extraction_chain function will call the create_structured_output_chain method, utilizing these customized parameters and preset prompts, combined with the powerful capabilities of LLM, to construct an efficient and accurate knowledge graph extraction chain. This design ensures both functional flexibility and the accuracy and practicality of the results.
# Import necessary modules and functions
from langchain.chains.openai_functions import create_structured_output_chain # Import function to create structured output chain from langchain library
from langchain_core.prompts import ChatPromptTemplate # Import class for generating chat prompt templates from langchain_core library
# Assume we have an instance of a large language model, represented by model here
llm = model
# Define a function to get the knowledge graph extraction chain
def get_extraction_chain(
allowed_nodes: Optional[List[str]] = None, # Optional parameter to specify allowed node labels, default is None
allowed_rels: Optional[List[str]] = None # Optional parameter to specify allowed relationship types, default is None
):
# Use ChatPromptTemplate.from_messages method to construct a prompt template
# This template provides detailed instructions to GPT-4 or other LLMs on how to construct the knowledge graph
prompt = ChatPromptTemplate.from_messages(
[
(
"system",
f"""# Knowledge Graph Instructions for GPT-4
## 1. Overview
You are a top-tier algorithm designed for extracting information in structured formats to build a knowledge graph.
- **Nodes** represent entities and concepts. They're akin to Wikipedia nodes.
- The aim is to achieve simplicity and clarity in the knowledge graph, making it accessible for a vast audience.
## 2. Labeling Nodes
- **Consistency**: Ensure you use basic or elementary types for node labels.
- For example, when you identify an entity representing a person, always label it as **"person"**. Avoid using more specific terms like "mathematician" or "scientist".
- **Node IDs**: Never utilize integers as node IDs. Node IDs should be names or human-readable identifiers found in the text.
{'- **Allowed Node Labels:**' + ", ".join(allowed_nodes) if allowed_nodes else ""}
{'- **Allowed Relationship Types**:' + ", ".join(allowed_rels) if allowed_rels else ""}
## 3. Handling Numerical Data and Dates
- Numerical data, like age or other related information, should be incorporated as attributes or properties of the respective nodes.
- **No Separate Nodes for Dates/Numbers**: Do not create separate nodes for dates or numerical values. Always attach them as attributes or properties of nodes.
- **Property Format**: Properties must be in a key-value format.
- **Quotation Marks**: Never use escaped single or double quotes within property values.
- **Naming Convention**: Use camelCase for property keys, e.g., `birthDate`.
## 4. Coreference Resolution
- **Maintain Entity Consistency**: When extracting entities, it's vital to ensure consistency.
If an entity, such as "John Doe", is mentioned multiple times in the text but is referred to by different names or pronouns (e.g., "Joe", "he"),
always use the most complete identifier for that entity throughout the knowledge graph. In this example, use "John Doe" as the entity ID.
Remember, the knowledge graph should be coherent and easily understandable, so maintaining consistency in entity references is crucial.
## 5. Strict Compliance
Adhere to the rules strictly. Non-compliance will result in termination.
"""),
("human", "Use the given format to extract information from the following input: {input}"),
("human", "Tip: Make sure to answer in the correct format"),
]
)
return create_structured_output_chain(KnowledgeGraph, llm, prompt, verbose=False)
def extract_and_store_graph(
document: Document,
nodes: Optional[List[str]] = None,
rels: Optional[List[str]] = None
) -> None:
"""
Extract and store graph structure information from the document into the Neo4j database.
Args:
document (Document): Document object to be processed.
nodes (Optional[List[str]]): Optional list of node types to limit the types of nodes extracted.
rels (Optional[List[str]]): Optional list of relationship types to limit the types of relationships extracted.
Raises:
TypeError: If document is not an instance of Document.
"""
if not isinstance(document, Document):
raise TypeError(f"Expected document to be an instance of Document, got {type(document)}")
# Use custom extraction chain to obtain graph data
extract_chain = get_extraction_chain(nodes, rels)
extracted_data = extract_chain.invoke(document.page_content)['function']
# Construct graph document object
graph_document = GraphDocument(
nodes=[map_to_base_node(node) for node in extracted_data.nodes],
relationships=[map_to_base_relationship(rel) for rel in extracted_data.rels],
source=document
)
# Print graph document information
print(graph_document)
# Store graph document information in the Neo4j database
graph.add_graph_documents([graph_document], True)
←Swipe left and right to view the complete code→
Using larger chunk size values can include as much context as possible for each sentence. This helps improve coreference resolution. It is important to remember that the coreference step only works when entities and their references appear in the same chunk; otherwise, the LLM does not have enough information to link the two.
# Use larger chunk sizes and overlap to enhance contextual understanding
from langchain_community.document_loaders import WebBaseLoader
from langchain_text_splitters import TokenTextSplitter
raw_documents = WebBaseLoader("https://blog.langchain.dev/what-is-an-agent/").load()
text_splitter = TokenTextSplitter(chunk_size=2048, chunk_overlap=24)
# Process only the first raw document as an example
documents = text_splitter.split_documents(raw_documents[:1])
For each document in the list, call the extract_and_store_graph function to extract the knowledge graph and store it in the Neo4j database. Additionally, the tqdm library can be used to display processing progress.
# Iterate through the document list, extract and store the knowledge graph
from tqdm import tqdm
for i, d in tqdm(enumerate(documents), total=len(documents)):
print(f"Processing chunk {i+1}: {d}")
extract_and_store_graph(d)
print("Graph stored successfully.")
# Use GraphCypherQAChain to browse information in the knowledge graph, similar to SQL queries in relational databases
# (This part of the code assumes GraphCypherQAChain has been correctly defined and is available)
# For example: Query specific nodes and their relationships
# query_chain = GraphCypherQAChain(...)
# result = query_chain.run("MATCH (n:Person)-[r]-(m) RETURN n, r, m")
# print(result)
←Swipe left and right to view the complete code→
Finally, we can leverage the GraphCypherQAChain class to explore and browse the rich information in the knowledge graph by carefully constructing Cypher query statements, paralleling the data retrieval in relational databases using SQL language, showcasing the commonalities across different fields of technology.
# Query the knowledge graph in RAG applications
from langchain.chains import GraphCypherQAChain
# Refresh the graph database schema to ensure the latest state
graph.refresh_schema()
print(graph.schema)
# Initialize GraphCypherQAChain, integrating large language models to enhance Cypher query capabilities
cypher_chain = GraphCypherQAChain.from_llm(
graph=graph,
cypher_llm=model, # LLM used to generate and optimize Cypher queries
qa_llm=model, # LLM used to answer query results
validate_cypher=True, # Validate relationship directions to ensure query accuracy
# return_intermediate_steps=True, # Optional, return intermediate steps of the query
verbose=True # Output detailed logs for debugging
)
Despite attempts at various query methods, uncertainties still arise in the process of converting natural language questions into Cypher query statements.
For example, using the following natural language query to explore the knowledge graph:
cypher_chain.invoke({"query": "what is Ai Agent?"})
Query results as follows ⬇️
Although the result does not directly show a node named “musings,” it indicates that the current system may have limitations in handling certain types of queries. We hope to optimize the query strategy or improve the data model to enable the system to handle such complex queries more intelligently. Currently, one of the most recognized effective strategies is to first identify the approximate range of relevant information using vector search, and then precisely retrieve specific details. However, the current example being processed is not entirely ideal, which somewhat reduces the urgency to find alternative solutions.
In summary, the journey of exploring knowledge graphs is both enjoyable and accompanied by numerous technical challenges. Although there are still many unknown areas to explore, the integration of knowledge graphs with RAG (retrieval-augmented generation) technology will unlock infinite possibilities. Looking ahead, Graph RAG is expected to become the global standard in this field, leading us into a new era of more intelligent and efficient information processing.
References:
https://github.com/tomasonjo/blogs/blob/master/llm/openaifunctionconstructinggraph.ipynb
https://github.com/tomasonjo/blogs/blob/master/llm/openaifunctionconstructinggraph.ipynb
https://www.linkedin.com/pulse/graph-databases-vs-vector-constantin-a-alexander/
https://pub.towardsai.net/langchain-graph-rag-gpt-4o-python-project-easy-ai-chat-for-your-website-46a46e24f161zqa
Tencent strikes again with a “king bomb”: Pre-training joint reinforcement learning gives quadruped robots a “lively” buff
2024-08-15
ACL2024 Best Paper Released! The diffusion model for deciphering oracle bone inscriptions is on the list
2024-08-15

【Deep Blue AI】‘s original content is created with great effort by the author team. We hope everyone abides by the original rules and cherishes the authors’ hard work. For reprinting, please message the backstage for authorization, and be sure to indicate the source from【Deep Blue AI】 WeChat official account, otherwise, legal action will be taken ⚠️⚠️
*Click like + save and recommend this article*