This article will cover the essence of GNN、GNNprinciples、GNNapplications in three aspects, allowing you to understand Graph Neural Networks (GNN) in one article.
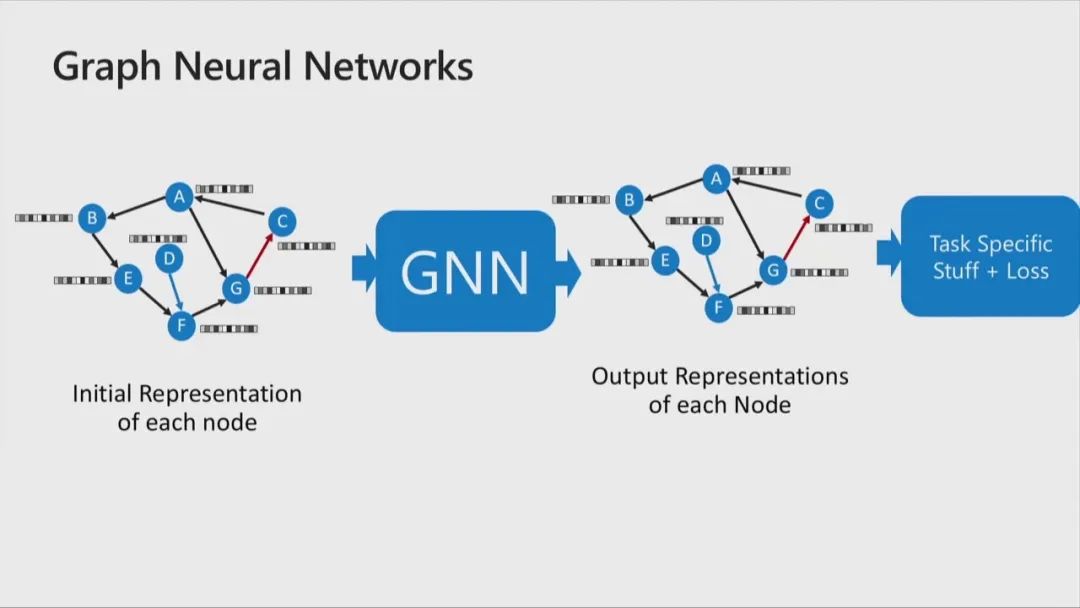
Graph Neural Network (GNN)
1. The Essence of GNNEssence of GNN
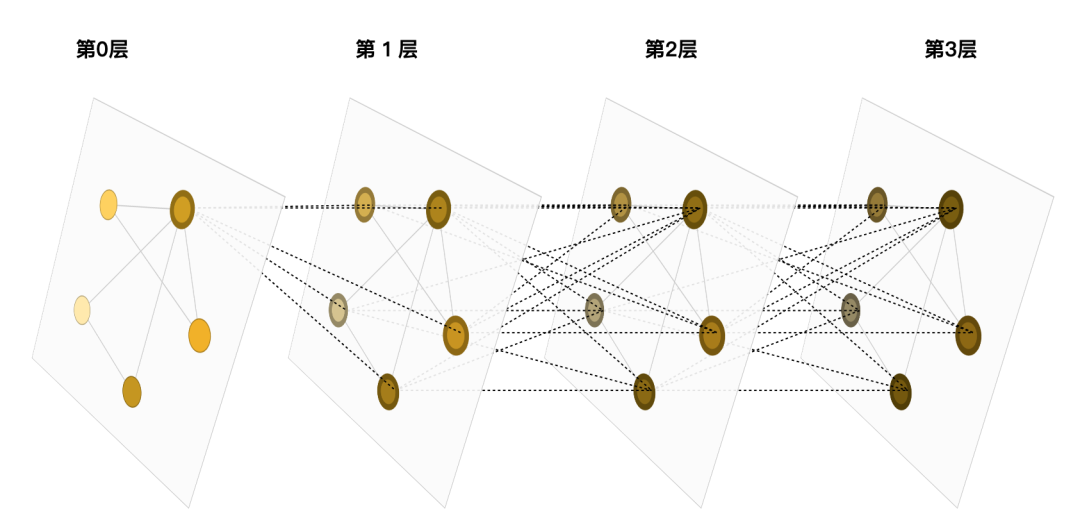
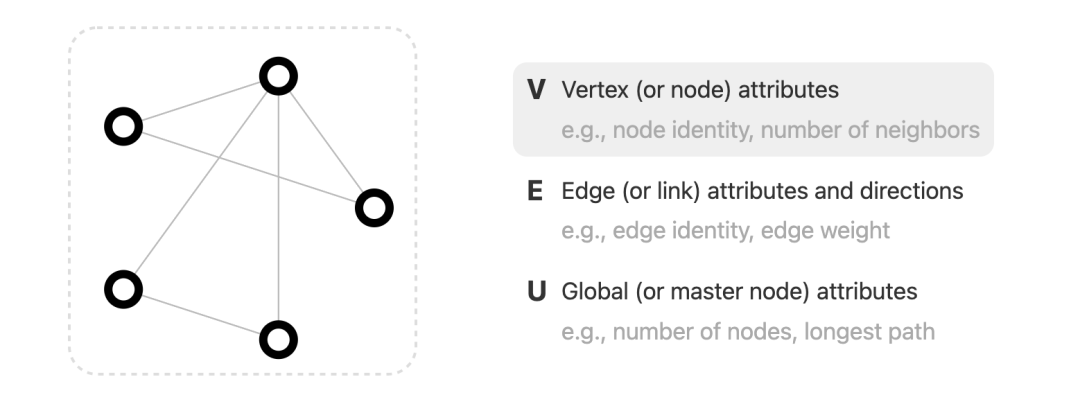
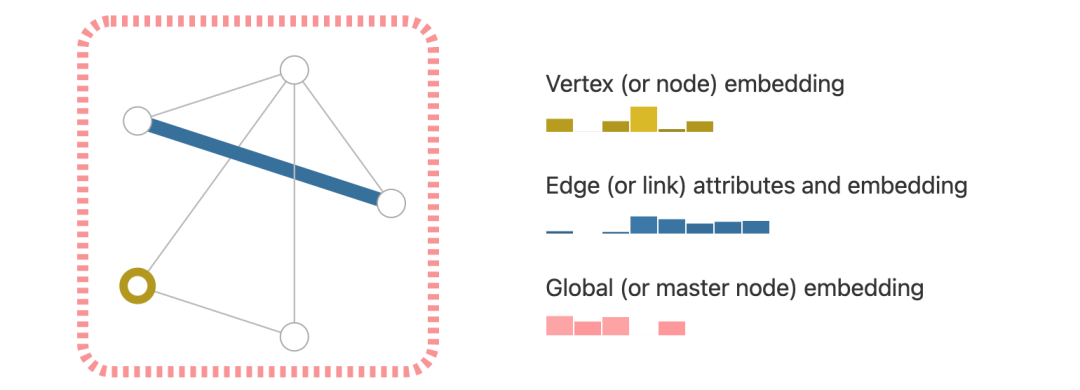
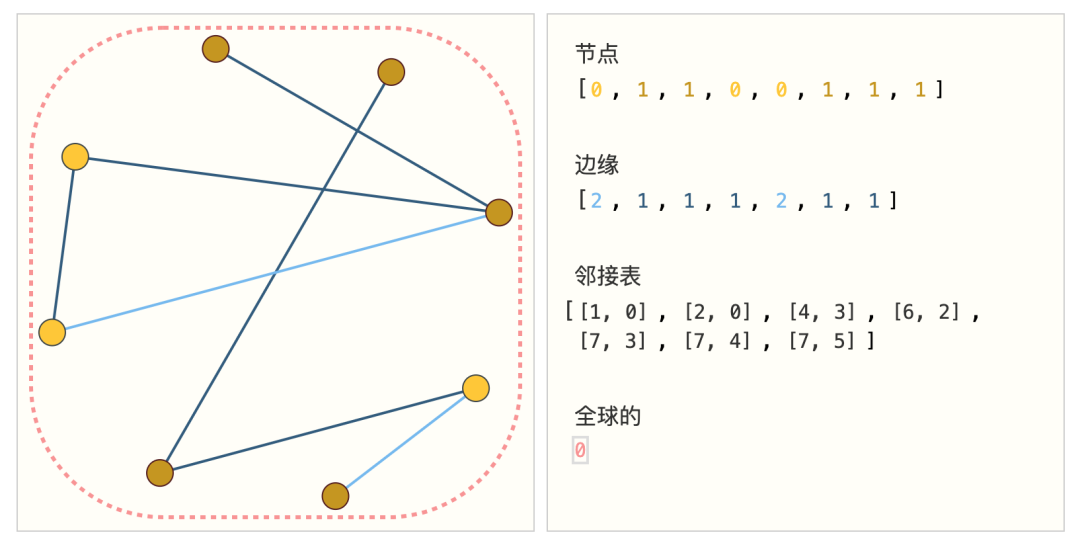
The adjacency matrix is a matrix representation of a graph.It uses a two-dimensional array to represent the relationships between the vertices in the graph. The rows and columns of the matrix correspond to the vertices in the graph, and the elements in the matrix represent the connection relationships between the vertices.
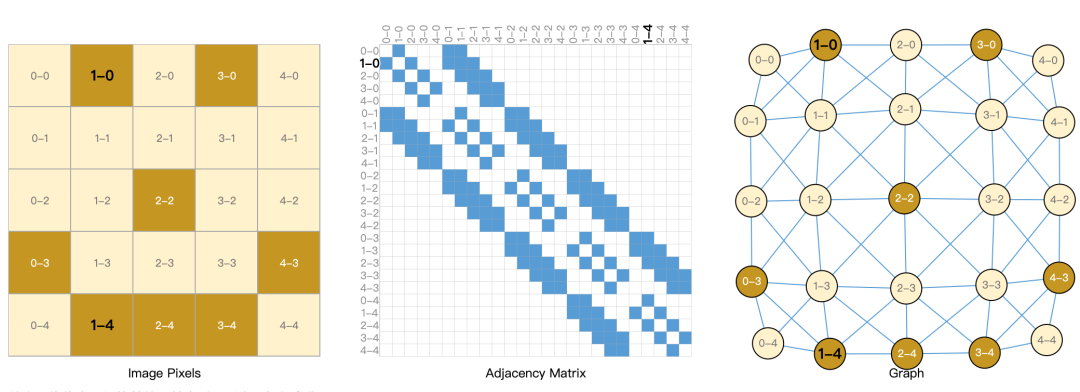
2. Principles of GNN
Architecture of GNN:GNN consists of three main functions,node function, edge function, and global function.These functions work together on the components of the graph (nodes, edges, and global context) to produce new embeddings or outputs.
-
Node function: Responsible for updating the node’s embedding by aggregating messages from neighboring nodes. The node function can be any differentiable function, such as a multilayer perceptron (MLP) or a recurrent neural network (RNN).
-
Edge function: Defines how to pass messages from edges to adjacent nodes, calculating messages based on the characteristics of the edge and the features of the two endpoints. The edge function can also be any differentiable function, such as a multilayer perceptron (MLP) or a recurrent neural network (RNN).
-
Global function: Generates a representation of the entire graph based on the information from all nodes and edges, used for graph-level prediction tasks. The global function can be achieved by aggregating the representations of all nodes or applying some form of pooling operation.
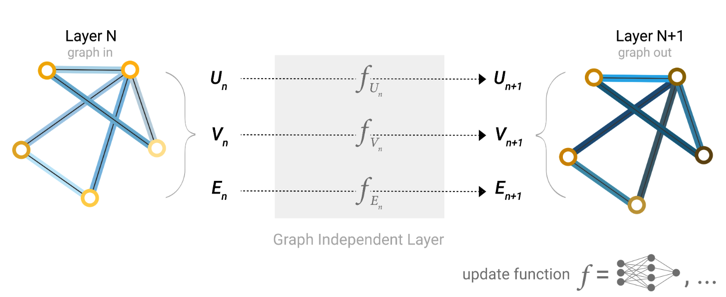
Architecture of GNN
Working principle of GNN:In GNN layers, using pooling and message passing mechanisms, we can build more complex GNN models that can capture the connectivity and structural information of the graph to make more accurate predictions.
Message passing mechanism typically includes the following three key steps:
-
Message Generation: For each node in the graph, we first need to collect information (i.e., “messages”) from all its neighboring nodes. This is usually done by passing the embeddings (or features) of neighboring nodes through a function
<span>g</span>
(such as a linear transformation or a neural network) to generate messages. These messages can contain information about the features of neighboring nodes and their relationships with the central node. -
Message Aggregation: Next, we need to aggregate all the messages received by the central node. This is typically done through an aggregation function (such as summation, average, max pooling, etc.). The purpose of the aggregation function is to combine multiple messages into a single vector that will represent the neighborhood information of the central node.
-
Node Update: Finally, we use the aggregated messages to update the embedding of the central node. This is usually achieved by passing the aggregated messages through an update function (such as a neural network). The role of the update function is to combine the current embedding of the central node with neighborhood information to produce a new, richer embedding.
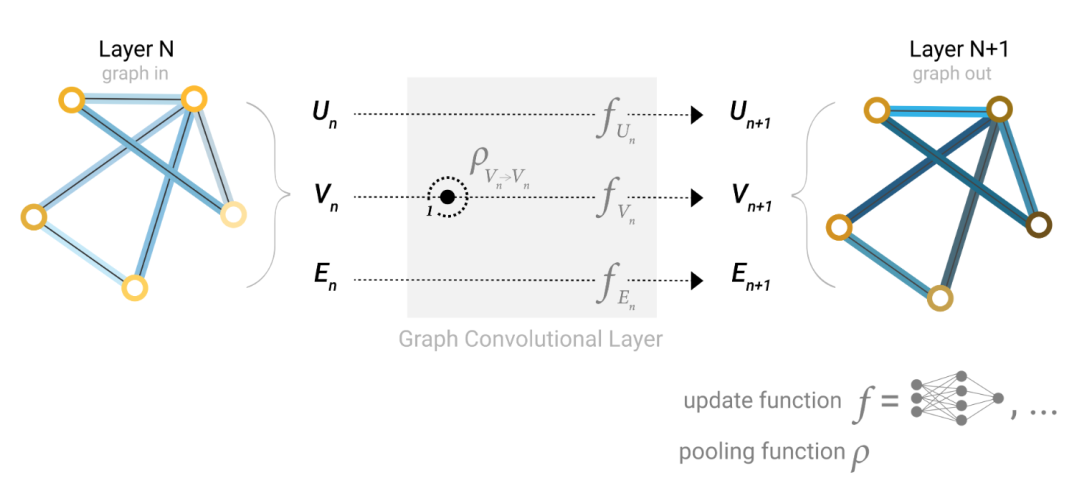
Working principle of GNN
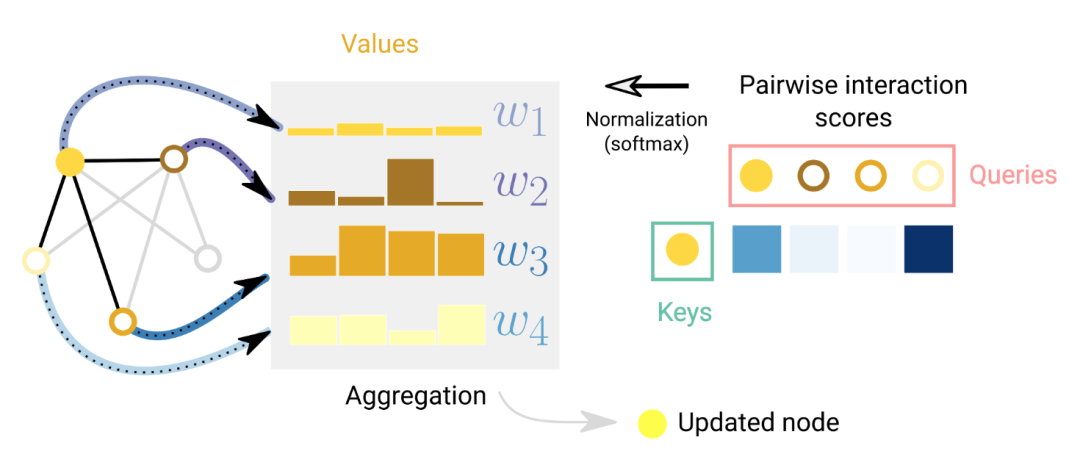
3. Applications of GNN
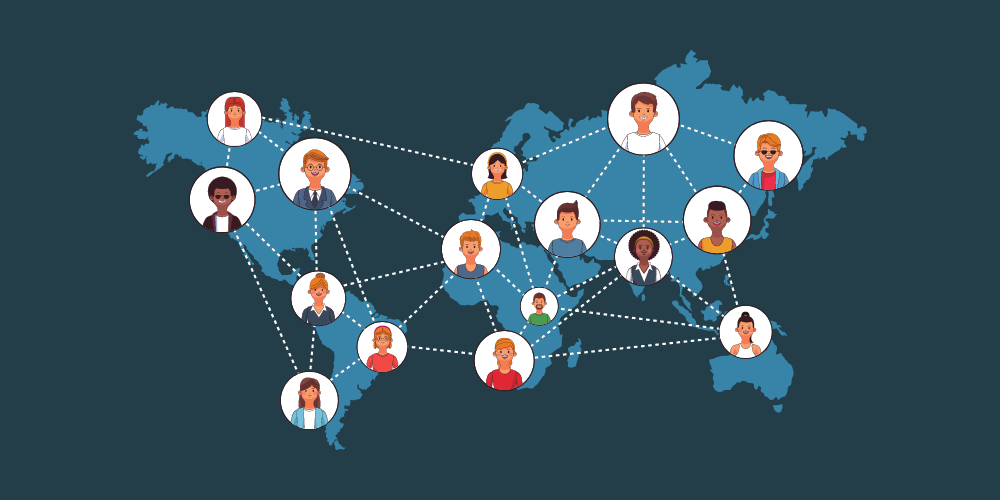
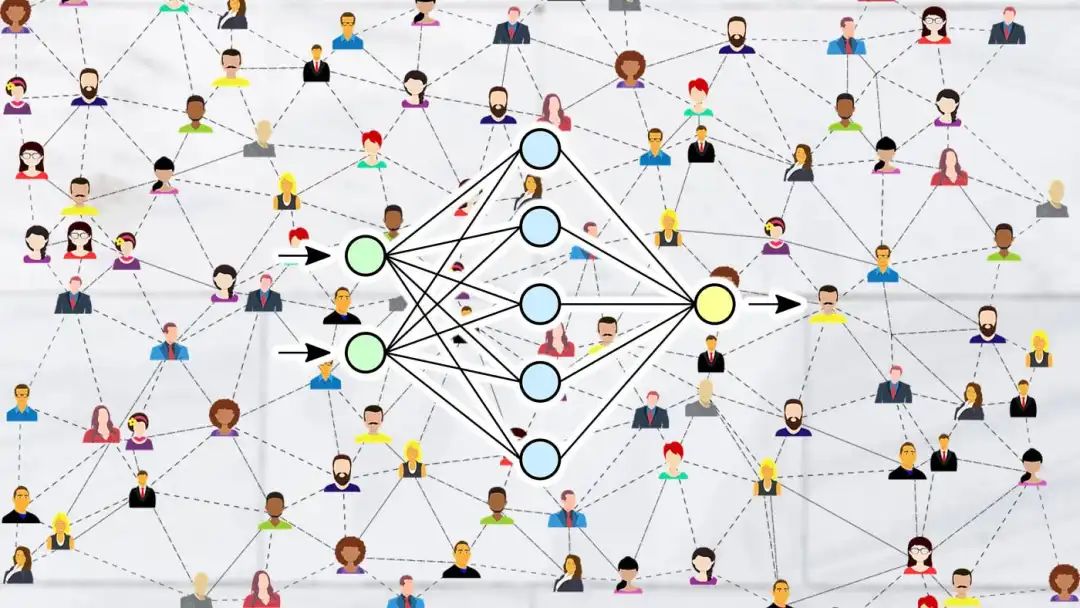
The following are four main applications of GNN in social network analysis:
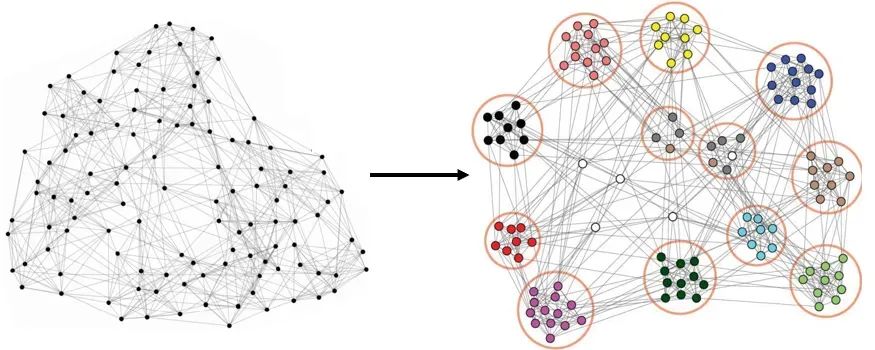
Link Prediction:In social networks, link prediction can be used for friend recommendations and predicting user behavior. By analyzing and modeling the graph structure, GNN can improve the accuracy and effectiveness of link prediction.
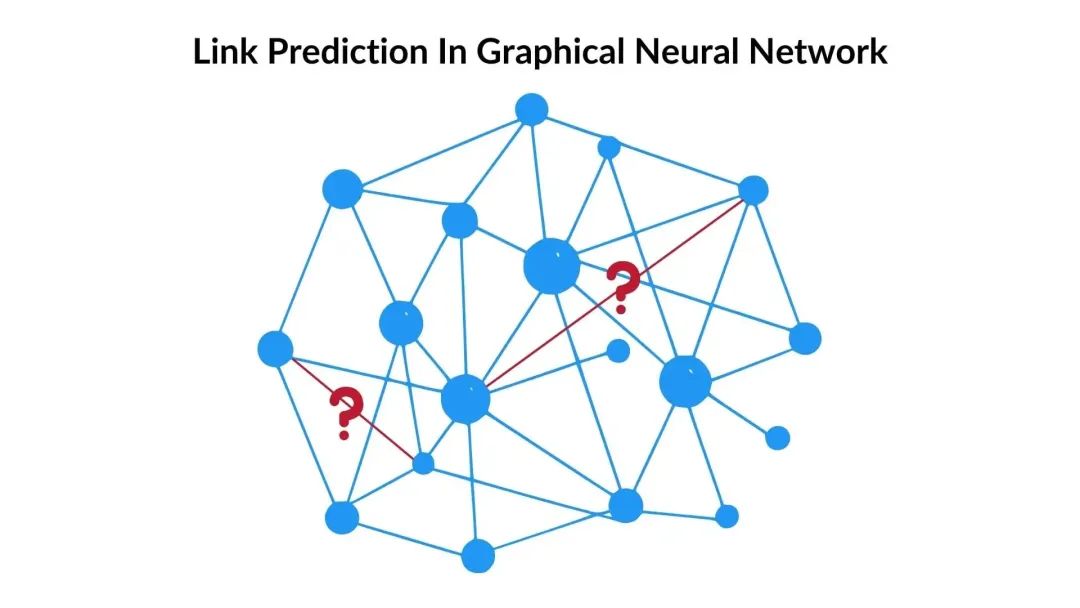
Node Classification:GNN can obtain the representation of nodes by iteratively updating the feature vectors of the nodes. Then, a linear classifier or other machine learning models can be applied to predict the categories of unlabeled nodes for classification and analysis in social networks.
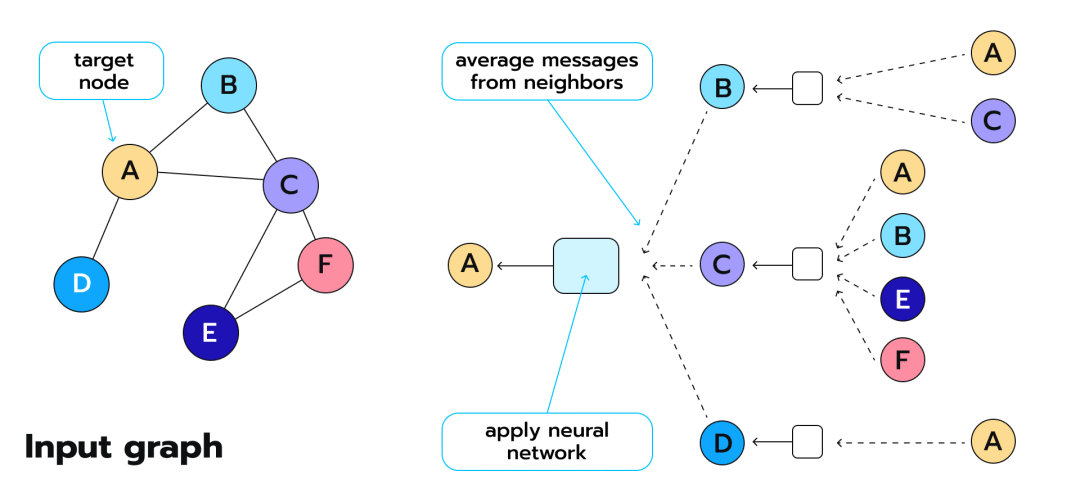
Personalized Recommendations:GNN can mine user relationships to recommend content that better meets their needs and interests. By learning and analyzing social relationships between users, GNN can provide more accurate recommendation services.
Personalized Recommendations