Translated by Machine Heart
Editor: Rome Rome
Stable Diffusion has learned the skill of “changing the sky and replacing the sun”.
Every creative process begins with “imitation” and ends with “creation”. For AI, this learning process is the same.
Recently, the open-source version of the Stable Diffusion text-to-image diffusion model has led to widespread applications of image generation technology. However, how to avoid pornographic or stylistic infringement remains a challenge. The authors proposed the Erased Stable Diffusion (ESD) method, which effectively addresses these issues.
Text-to-image generation models have attracted significant attention due to their excellent image generation quality and seemingly infinite generation potential. Such generative models are trained on large-scale internet datasets, allowing them to learn a wide range of concepts. However, some concepts generated by the model are undesirable, such as copyrighted content and pornographic material.
How can we avoid the model outputting these contents? In a recent paper, authors from Northeastern University and MIT proposed a method to selectively remove individual concepts from the weights of the text-conditioned model after pre-training.
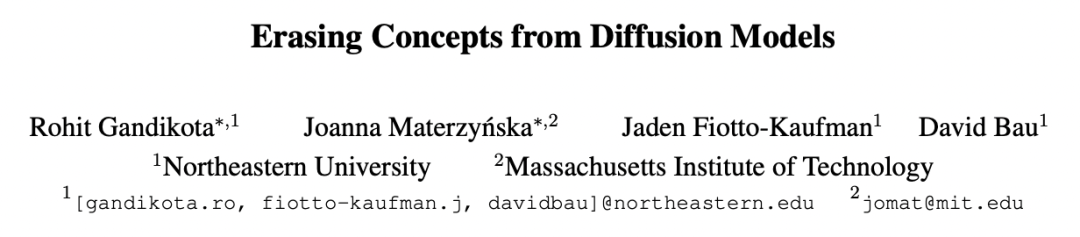
Paper link: https://arxiv.org/pdf/2303.07345v1.pdf
Previous methods focused on dataset filtering, post-generation filtering, or inference guidance, while the method proposed in this paper does not require retraining, which is incredible for large models. Inference-based methods can review or effectively guide the model’s output to avoid generating unwanted concepts. In contrast, the method in this paper directly removes concepts from the model’s parameters, allowing for safe allocation of its weights.
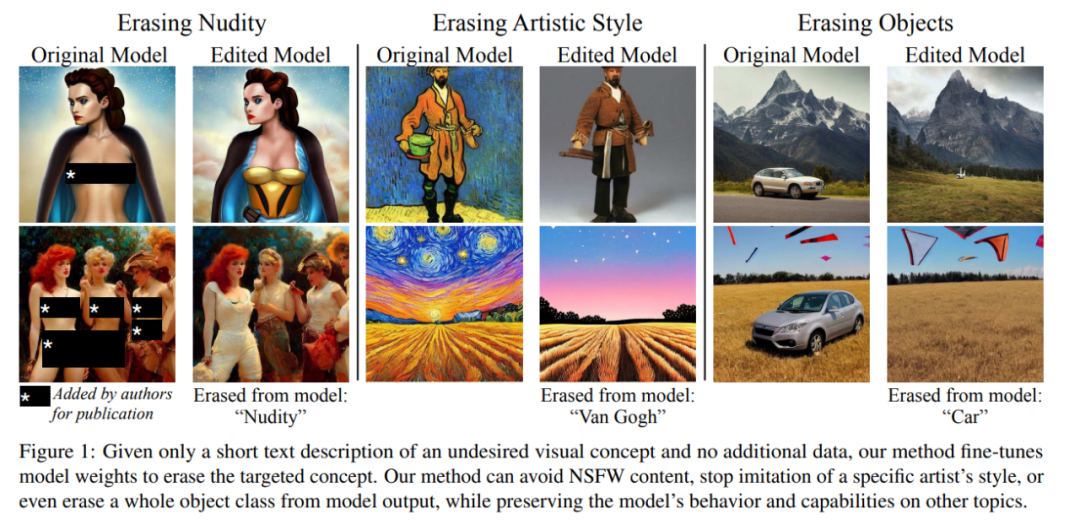
Safety Issues in Image Generation
The open-source Stable Diffusion text-to-image diffusion model has led to widespread applications of image generation technology, but it has also brought some issues.
To restrict the generation of unsafe images, the first version of Stable Diffusion included an NSFW filter to review images when the filter was triggered. However, since both the code and model weights are publicly available, the filter can easily be disabled.
To prevent the generation of sensitive content, the subsequent Stable Diffusion 2.0 model trained on filtered data to remove obviously problematic images, with experiments conducted on the LAION dataset of 5 billion images, consuming 150,000 GPU hours.
This high cost of the entire process makes it extremely challenging to establish a causal relationship between changes in data and emergent capabilities. Some researchers have reported that removing obviously problematic images and other subjects from the training data may negatively affect output quality.
Despite the authors’ efforts, content related to pornography remains prevalent in the model’s output: when the authors evaluated the image generation results using 4,703 prompts from the Inappropriate Image Prompts (I2P) benchmark, they found that the currently popular SD1.4 model generated 796 images of exposed body parts, while the newly restricted SD2.0 model produced 417 similar images.
Another issue is that the works imitated by text-to-image models may be copyrighted. AI-generated artworks not only match the quality of human-generated art but can also faithfully replicate the artistic styles of real artists. Users of Stable Diffusion and other large text-to-image synthesis systems have found that prompts such as “art in the style of [artist]” can imitate specific artists’ styles, potentially resulting in infringing works. Previously, concerns from multiple artists led to a legal lawsuit against the creators of Stable Diffusion, where artists accused Stable Diffusion of infringing their works. To protect artists, some recent efforts have attempted to apply adversarial interference to artworks before online publication to prevent the model from imitating them. However, this approach does not remove the artistic styles that the model has already learned from the pre-trained model.
Therefore, to address safety and copyright infringement issues, the authors of this paper proposed a method to “erase concepts” from the text-to-image model, namely Erased Stable Diffusion (ESD), which can achieve erasure by fine-tuning model parameters without the need for additional training data.
Compared to training set review methods, the proposed method is faster and does not require retraining the entire system from scratch. Additionally, ESD can be applied to existing models without modifying input images. Unlike post-generation filtering or simple blacklist methods, “erasure” is not easily circumvented, even if users have access to the parameters.
The goal of the ESD method is to erase concepts from the text-to-image diffusion model using its own knowledge, without requiring additional data. Therefore, ESD chooses to fine-tune the pre-trained model rather than training a model from scratch. This method focuses on Stable Diffusion (SD), which consists of three sub-networks: a text encoder T, a diffusion model (U-Net) θ, and a decoder model D.
ESD removes specific styles or concepts by editing the weights θ of the pre-trained diffusion U-Net model. ESD is inspired by classifier-free guidance methods and score-based synthesis. Specifically, it uses the principles of classifier-free guidance to train the diffusion model, erasing the model’s scores from specific concepts c that need to be eliminated, such as the term “Van Gogh”. It utilizes the pre-trained model’s understanding of concepts while allowing it to learn to remove the quality distribution of the fine-tuned output from that concept.
The score-based formula of the diffusion model aims to learn the conditional model’s scores
.Using Bayes’ rule and

This can be interpreted as having a gradient from the classifier pθ(c|xt) of unconditional scores. To control the effect of conditionality, the authors introduce a guidance factor η for the classifier’s gradient.

The authors aim to negate concept c by reversing
‘s behavior, thus using a negative version of the guidance to train θ. Additionally, inspired by classifier-free guidance, the authors convert the RHS of equation 5 from the classifier to conditional diffusion.

Based on the Tweedie formula and reparameterization techniques, the gradient of the log probability score can be represented as a score function scaled by time-varying parameters. The modified score function shifts the data distribution to maximize the log probability score.

The objective function in equation 7 fine-tunes the parameters θ to simulate negative guidance noise. Thus, after fine-tuning, the conditional predictions of the edited model will move away from the erased concepts.
The following diagram illustrates the training process. Utilizing the model’s knowledge of concepts to synthesize training samples eliminates the need for data collection. The training uses several instances of diffusion models, where one set of parameters is frozen (
), while another set of parameters (θ) is trained to erase concepts.Using θ to sample partially denoised images xt under condition c, the frozen model
is subjected to two rounds of inference, once under condition c and once without any condition. Finally,the two prediction results are linearly combined to offset the prediction noise associated with the concept and adjust the new model towards the new target.
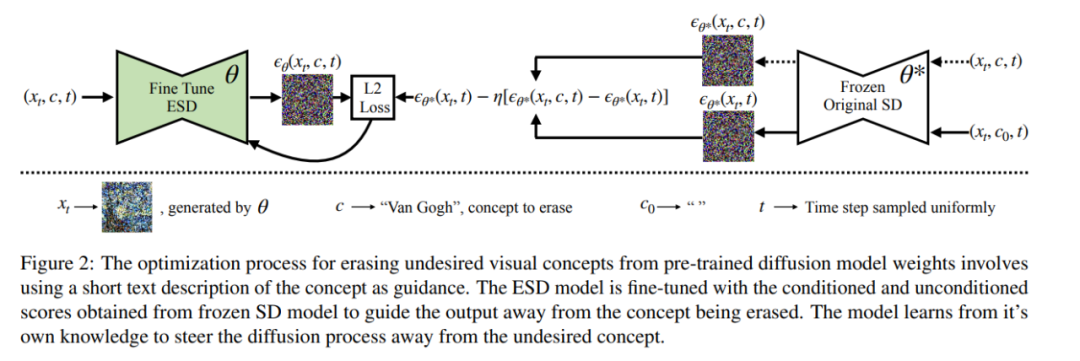
To analyze the artistic imitation among contemporary practicing artists, the authors selected 5 modern artists and artworks for examination, namely: Kelly McKernan, Thomas Kinkade, Tyler Edlin, Kilian Eng, and the “Ajin: Demi-Human” series. These authors or works have reportedly been imitated by the Stable Diffusion model. Although the authors did not observe the model directly copying certain specific original artworks, it is undeniable that the model must have captured these artistic styles.
Figure 5 shows relevant qualitative results, and the authors also conducted user studies (Figure 6) to measure human perception of the effectiveness of art style removal. The final experimental results validate this observation, indicating that the model has removed the styles of specific artists while preserving the content and structure of the prompt (Figure 5), with minimal interference from other artistic styles.
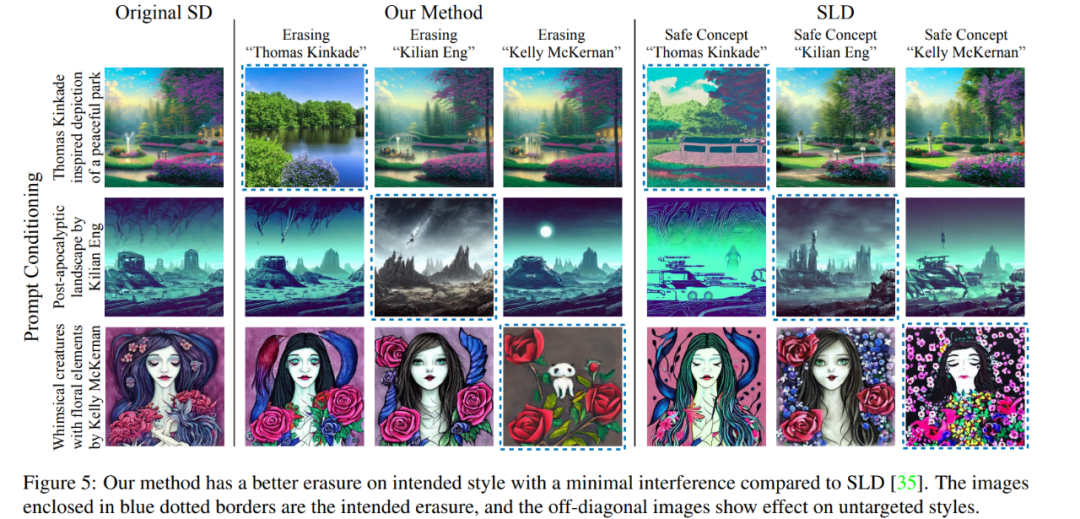
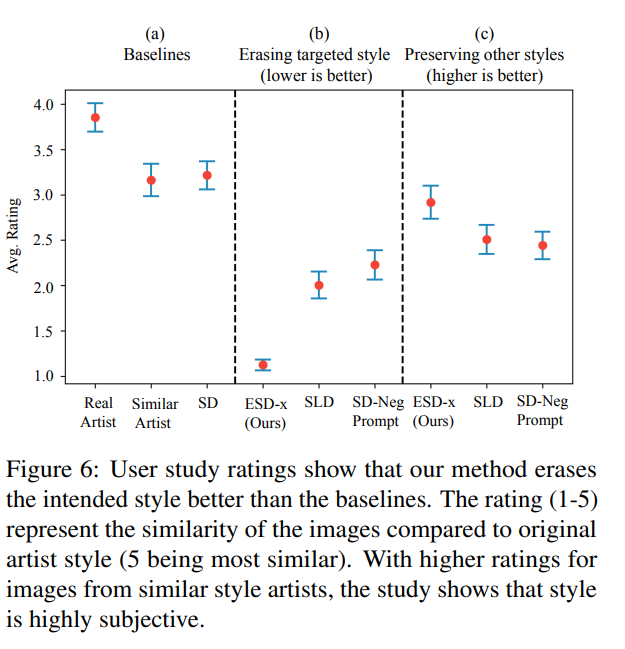
In Figure 7, the percentage change of nude classification samples compared to Stable Diffusion v1.4 is shown. The authors studied the effectiveness of ESD using inference methods (SLD) and filtered retraining methods (SD V2.0). For all models, 4,703 images were generated using I2P prompts. They used the Nudenet detector to classify images into various nude categories. The conclusion is that at a weak erasure ratio η=1, the ESD method is significantly more effective in erasing nudity across all categories.
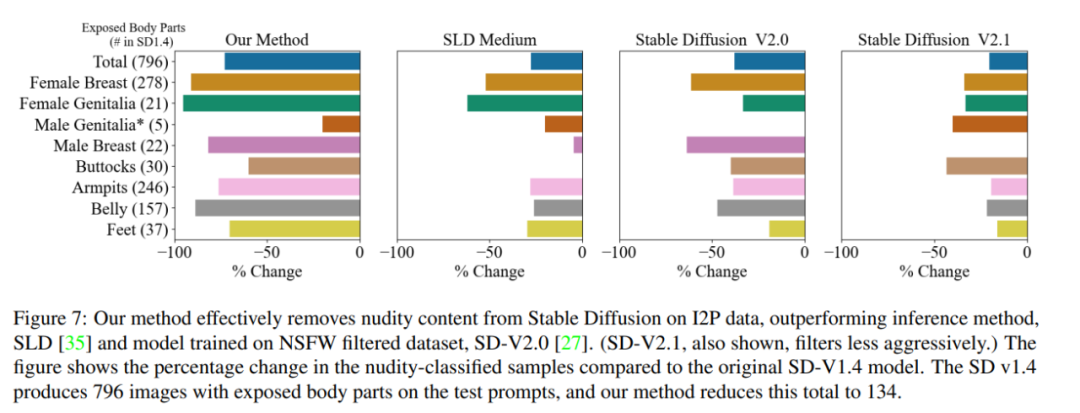
Table 2 compares the classification accuracy of the original Stable Diffusion model and the ESD-u model when erasing target classes during training, while also showing classification accuracy when generating the remaining nine classes. The results indicate that ESD can effectively remove target classes in most cases, although some categories (like churches) are more challenging to remove. However, the classification accuracy of the categories that were not removed remains high, even though there may be some interference in certain cases, such as removing “French horn” causing noticeable distortion to other categories. The authors provide visual effect images after object removal in the supplementary materials.
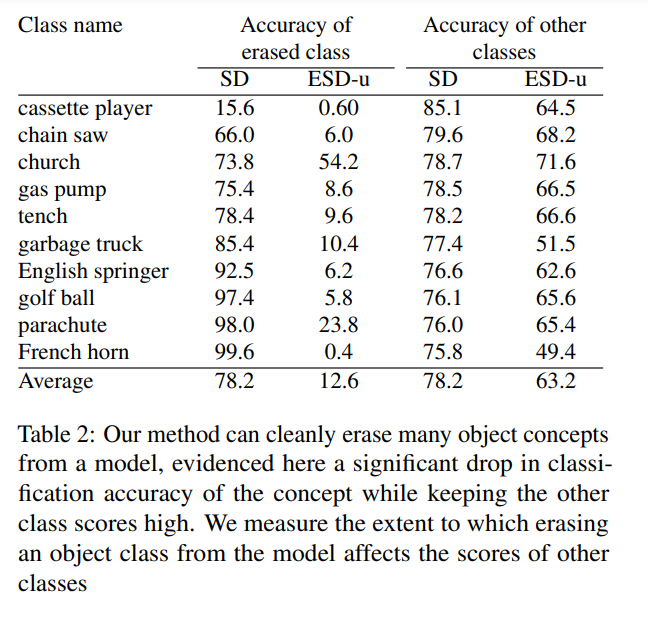
For more research details, please refer to the original paper.
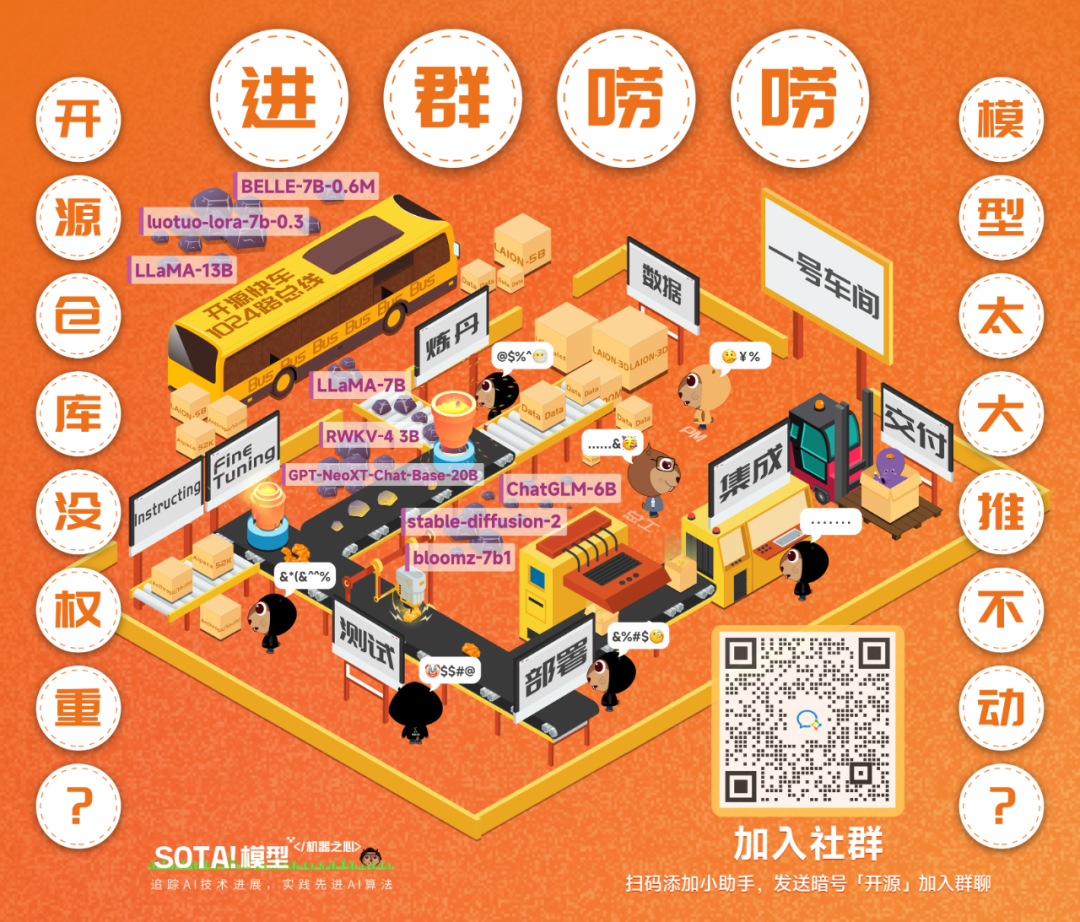
© THE END
Please contact this public account for authorization
Submissions or inquiries: [email protected]