Please click “Yueying Technology“, “Brain Talk“, and choose to follow us.Yueying Technology focuses on online/offline training and data processing services for technologies such as EEG/ERP, fMRI, sMRI, DTI, fNIRS, and machine learning, hoping that professional services can provide support and help to colleagues. In addition, we have establisheda WeChat group and QQ group for neuroscience literature services, with dedicated personnel to help download literature for free, and colleagues are welcome to join. If you have any questions, you can add WeChat ID15560177218 for consultation.
Even with significant advancements in information technology, predicting the initial compatibility of heterosexual individuals based on self-reported characteristics and preferences has not been successful. To overcome the limitations of self-report measurements and predictions of compatibility, we used functional connectivity from resting-state functional magnetic resonance imaging (fMRI) data, which carries rich individual-specific information sufficient to predict psychological constructs and activation patterns in social cognitive tasks. A few days after collecting data from resting-state fMRI, participants engaged in a speed dating experiment where they interacted with all other opposite-sex participants for 3 minutes each. Our machine learning algorithms successfully predicted whether pairs were compatible in the experiment, using the (dis)similarity of functional connectivity obtained before the experiment. The similarities and differences in functional connectivity between individuals and these multivariate relationships contribute to predictions, indicating the importance of complementarity (observed dissimilarity) and the similarity between individuals and potential partners in the initial attraction phase. The results suggest that the salience network, marginal regions, and cerebellum are particularly important for compatibility. This study emphasizes the utility of neural information in predicting complex phenomena in social contexts, which cannot be predicted solely by behavioral measurements.
Finding a suitable romantic partner is challenging, which is why the marriage and dating service industry has a strong economic market. To help people find their soulmates, some matching services utilize algorithms designed to predict their potential suitable partners based on self-reported data before users begin to communicate. However, the performance of such algorithms is currently very limited. Among these algorithms, following social relationship research, similarity and complementarity of personality are considered important for compatibility.People prefer to date those with similar personality types and complementary attachment insecurities (anxious or avoidant).They also prefer partners whose interpersonal styles complement each other (dominant or submissive) for dyadic interactions. Therefore, this psychological construct can help predict individuals’ compatibility.
However, a recent study showed negative results in predicting compatibility. Participants first completed over 100 psychological questionnaires. Subsequently, they participated in a speed dating event where they had 4 minutes of conversation with each opposite-sex participant, known as speed dating. After each speed date, participants filled out three items regarding their romantic desire for potential partners. The results indicated that even with over 100 psychological constructs, machine learning algorithms could not predict the degree of desire individuals had for each other. This may be due to the limited time in the context of speed dating where individual behaviors do not necessarily trigger actions consistent with self-reported psychological constructs that reflect general behavioral tendencies. Another study also indicated that perceived similarity in dyadic interactions, rather than similarity based on self-report measures collected before meeting, is crucial for predicting initial attraction. There is also a discrepancy between subjective preferences for specific qualities in a partner before meeting and the corresponding qualities of the chosen partner. Therefore, to predict compatibility at the beginning of a relationship, predictive variables need to reflect the behavioral tendencies exhibited by both individuals in dyadic interactions.
Over the past two decades, intensive research has been conducted on spontaneous brain activity in the absence of tasks due to the valuable information it carries and the simplicity of data collection. By scanning resting-state fMRI for no more than 10 minutes, correlation patterns across regions can be obtained (i.e., functional connectivity) and functional organization (i.e., large-scale brain networks) corresponding to activation patterns induced by various tasks can be identified. Resting-state functional connectivity can predict various psychological constructs representing general behavioral and thought tendencies (e.g., Big Five personality traits) and can also predict activation patterns in various social cognitive tasks requiring the abilities necessary for dyadic interactions (e.g., emotional processing, language, and social cognition). Research has also found that the (dis)similarity in functional connectivity profiles between two individuals represents the (dis)similarity of psychological constructs or behavioral tendencies. Furthermore, recent studies have successfully predicted proximity in real social networks based on the similarity of resting-state functional connectivity, suggesting that functional connectivity may capture potential interpersonal similarities between friends, which are often not fully captured by demographic or personality measurement methods. Based on these findings, it is likely that the (dis)similarity of functional connectivity profiles between an individual and a potential romantic partner corresponds to the (dis)similarity of behavioral tendencies exhibited in dyadic interactions. This would allow us to predict the outcome of such interactions, namely compatibility at the beginning of a relationship.
In this study, we used a speed dating paradigm and functional magnetic resonance imaging (fMRI) to demonstrate that compatibility in male-female relationships can be predicted through collected individual functional connectivity profiles. This study utilized functional connectivity similarity indices and machine learning algorithms to predict compatibility. The comparable index used by Hyon et al. (2020) is the absolute value of resting-state fMRI functional connectivity before the meeting.
We hypothesize that compatibility can be successfully predicted through the (dis)similarity of functional connectivity profiles. Considering that compatibility depends not only on similarity but also on the complementarity of characteristics, such as dominance/submission and attachment anxiety/avoidance, the feature values contributing to compatibility classification can be both positive (indicating similarity) and negative (indicating dissimilarity or complementarity). This feature contributed by classification will allow us to explore the neural basis of compatibility.
As far as we know, this current research is the first to use functional connectivity similarity indices as feature values in neuroimaging studies (although similar methods have been used in social psychology questionnaire data), we first evaluated the utility of the index using publicly available data from the Human Connectome Project (HCP). In this index evaluation, we attempted to classify paired data collected from a given individual (self-self) and different individuals (self-other) at different time points using the same protocol. We then confirmed that the index represents pairing-specific information, enabling us to distinguish pairs with different attributes. While traditional resting-state fMRI studies only use low-frequency (<0.1 Hz) data, recent research has revealed the importance of frequency-related information and the connection between high-frequency data and complex information processing. Therefore, we classified the information using data from four different frequency bands. The effective frequency bands for data classification were also marked during the index evaluation using the HCP dataset.
The subjects came from the test-retest dataset of HCP. The data were preprocessed using DPARSF.
2.1.2 Decomposing Data into Multiple Frequency Bands
To ensure that the identification and compatibility classification used the same frequency bands, this program used data for compatibility classification, as described in the “Decomposing Signals into Multiple Frequency Bands” section of compatibility classification. Four frequency bands (0.109-0.199, 0.055-0.109, 0.027-0.055, and 0.014-0.027 Hz) were defined similarly to previous studies for subsequent analysis; these bands are referred to as F1, F2, F3, and F4, respectively.
2.1.3 Personality Identification
The personality of the two groups of data depends on whether they come from the same person. A self-self pair contains two groups of collected data from one individual at different time points (e.g., subject A at time 1 and subject A at time 2). A self-other pair contains two groups of data collected from two different individuals at different time points (e.g., subject A at time 1 and subject B at time 2). Therefore, we obtained 44 pairs of self-self pairs and 44 pairs of self-other pairs.
Considering that self-self pair data is considered more similar than self-other pairs data, if the feature values indeed represent individual-specific information, then the machine learning algorithm should be able to distinguish self-self pairs from self-other pairs. Moreover, the feature values used for classification (to be explained in the next section) should have positive coefficients, as a high probability of being a self-self pair should be related to high similarity in connectivity aspects (rather than differences). Therefore, we confirmed that the program can correctly classify pairs based on individual-specific information, assessing which frequency bands contain particularly rich individual information and may predict compatibility.
2.1.4 Features of Paired Data
Appropriate feature values are needed to construct machine learning classifiers and classify personality labels. Features that may represent the consistency of two groups of data are calculated through functional connectivity, namely the Pearson correlation of regional average time series data. Regions of interest (ROIs) are defined by automated anatomical labeling (AAL), which divides the brain into 116 regions of interest. Each data point yields 6670 functional connectivity vectors. The absolute difference vector of the two vectors is then obtained as a set of feature values for each frequency band pair. Below, we refer to these feature values as functional connectivity comparisons.
2.1.5 Similarity of Connectivity Patterns
In addition to preparing feature values, we also compared the similarity (Pearson correlation of functional connectivity vectors) within pairs between the two groups (i.e., self-self pairs vs. self-other pairs) as an initial analysis.
2.1.6 Personality Classification from Functional Connectivity Comparisons
We classified personality based on the comparisons of functional connectivity vectors. In terms of classification, we used a machine learning algorithm called Sparse Logistic Regression with Elastic Net Regularization (SLR-EN) in Python’s scikit-learn package. Elastic Net is a regularization method that allows for feature selection (i.e., different vectors of functional connectivity) and prevents overfitting of the classifier, which contains a large number of parameters compared to the number of participants. Therefore, the combination of Elastic Net and logistic regression allows the extraction of a set of functional connections whose similarities distinguish personality from numerous parameters in a more generalized manner, rather than assessing the importance of each parameter through independent application of logistic regression.
2.2 Compatibility Classification Based on Functional Connectivity Comparisons
2.2.1 Participants and Experimental Design
The participants for compatibility classification included 42 healthy young volunteers with no history of neurological disorders. Participants underwent a 4-day experiment, including three phases: 1) resting-state fMRI testing before speed dating tasks/rest, 2) three speed dating events, and 3) resting-state fMRI testing after speed dating. The experimental paradigm is shown in Figure 1.
Figure 1 Experimental Design
2.2.2 Image Acquisition and Preprocessing
BOLD imaging and T1 structural imaging were collected. Preprocessing was consistent with the previous section.
2.2.3 Signal Decomposition
The wavelet transform method was applied to extract regional average time series data from the 116 ROIs defined by AAL. This step decomposes the signal into seven waveforms, with frequency bands varying according to waveforms, ROIs, and subjects. Thus, each subject has 116 minima at the lower frequency limit for each frequency band. The median of these minima is set as the lower limit for each frequency band, and the upper limit for each frequency band is set as the lower limit of the following frequency bands. Frequency bands below 0.010 Hz were discarded due to low information value, and the analysis subsequently used data from four frequency bands: 0.109-0.199, 0.055-0.109, 0.027-0.055, and 0.014-0.027 Hz; these bands are referred to as F1, F2, F3, and F4, respectively.
2.2.4 Definition of Compatibility
Compatibility between two individuals is defined by their impressions of each opposite-sex participant. If both individuals choose each other as potential partners they want to talk to again, they are marked as “compatible”. Otherwise, a pair is marked as incompatible. Based on this definition, there are 158 compatible pairs and 282 incompatible pairs.
2.2.5 Feature Values of Paired Data
Defined as in the previous section.
2.2.6 Similarity of Connectivity Patterns
We compared the similarities between compatible and incompatible groups, following the procedures described in the similarity of connectivity patterns section for personality classification.
2.2.7 Compatibility Classification from Functional Connectivity Comparisons
In the main analysis, we classified compatibility after speed dating based on the comparisons of functional connectivity vectors. This was completed using the methods described in the personality classification section from functional connectivity comparisons, with additional oversampling.
3 Results
3.1 Personality Classification Results with the HCP Dataset
As shown in Figure 2A, the functional connectivity pattern similarity of self-self pairs is significantly higher than that of self-other pairs across all frequency bands. This indicates that functional connectivity represents individual-specific patterns.
The negative coefficients of functional connectivity comparisons in personality classification should be the result of overfitting. Therefore, we used a binomial test for each frequency band to examine whether the number of positive coefficients was significantly greater than the number of negative coefficients. Among the top 1% of coefficients contributing to personality classification (6670/100 = 67) (Figure 3), most coefficients in F1 (53 out of 67) and F2 (52 out of 67) were positive. These results confirm that the functional connectivity comparisons in these frequency bands reflect the differences in connectivity patterns between self-self pairs and self-other pairs. In contrast, F3 did not show significant differences (33 out of 67), indicating that significant classification in this frequency band may not be achieved through individual-specific information but rather through overfitting. Regarding the proportion of connectivity related to classification in brain networks, the study found that the functional connectivity comparisons of the cerebellum were important for personality classification across all significant frequency bands (Figure 4).
Figure 3 The top 100 feature values, i.e., the absolute values of functional connectivity differences across frequency bands, contribute to classification
Figure 4 The proportion of connectivity contributed by classification in brain networks
In summary, the index evaluation confirmed that functional connectivity comparisons in paired data, especially in higher frequency bands (F1 and F2), represent pairing-specific information, allowing for differentiation of pairs with different attributes. The results also indicate that the information represented by lower frequencies (i.e., F3 and F4) is unreliable.
3.2 Compatibility Classification Results
The results of compatibility classification differ significantly from those of personality classification. First, as shown in Figure 5A, there is no significant difference in overall functional connectivity pattern similarity between compatible and incompatible pairs. This indicates that compatibility in male-female relationships is not necessarily represented by the similarity of functional connectivity patterns.
Secondly, and most importantly, significant accuracy for compatibility classification was achieved for F1 (above chance level 5.47%±2.10%) and F2 (above chance level 4.95%±2.28%) as shown in Figure 5B. This means that the functional connectivity comparisons in these frequency bands contain specific information capable of predicting whether a given pair is compatible.
Unlike personality classification, compatibility classification is supported by considerably large negative coefficients for features (Figure 6A, B).
Figure 5 Compatibility Classification Results
Figure 6 Significant features contributing to compatibility classification
The contribution of ROIs to classification is represented by the number of functional connectivity comparisons belonging to the ROI. Figures 6C, E show that the contributing ROIs tend to belong to specific networks (rather than visual and sensorimotor networks) and exhibit lateralization differences (cerebellar comparisons).
Regarding the proportional contributions of each network combination (Figures 6D, F), the cerebellum exhibited a significant overall positive contribution in F1, while the significant overall contribution from the salience network in F2.
No significant results were found regarding the total comparisons of lateralization within networks (Figures 6C, E).
4 Discussion
In this study, we attempted to predict compatibility in male-female relationships after speed dating using resting-state fMRI data. The index evaluation results from the HCP test-retest dataset (personality classification) indicate that compatibility in relationships can be classified by comparing functional connectivity in high-frequency bands (i.e., F1 and F2). The study found that the overall functional connectivity profile similarity of compatible pairs was not higher than that of incompatible pairs, and compatibility classification supports significant differences in functional connectivity and personality classification. This may reflect the fact that compatibility depends on both similarity and complementarity between individuals and potential partners. To our knowledge, this is the first study elucidating the neural basis of compatibility and emphasizing the potential of resting-state fMRI in predicting interpersonal outcomes.
Our study raises four valuable future directions. First, to enhance our understanding of the neural basis of compatibility and interpersonal attraction or mating behaviors, we should examine the missing links between resting-state functional connectivity similarity, brain-brain coupling during dyadic interactions, and perceived similarity. Secondly, utilizing task-based functional connectivity can improve the performance of compatibility predictions. Thirdly, this study emphasizes the importance of frequency-specific functions in social relationships. Fourthly, the prediction of dyadic psychological constructs based on similarity from resting-state functional connectivity is beneficial.
In summary, this study demonstrates that the initial compatibility of heterosexual individuals, which cannot be predicted by self-reported psychological constructs, can be predicted through functional connectivity from resting-state fMRI data. The contributions of similarities and differences in functional connectivity between individuals and these multivariate relationships indicate the importance of complementarity and the similarity between individuals and potential partners. Our research underscores the utility of neuroimaging in examining complex phenomena in social contexts.
References: Brain Knows Who Is on the Same Wavelength: Resting-State Connectivity Can Predict Compatibility of a Female–Male Relationship
EEG Training Courses
The 7th EEG and Machine Learning Practical Course (Python Version, Online Class, 2022.7.23-26)
The 22nd Practical Course on EEG Data Analysis Techniques (Online Live, 2022.8.10-14)
MRI Training Courses
The 6th Practical Course on Multimodal MRI Brain Network Analysis Techniques (Online Live, 2022.6.25-28)
The 3rd Practical Course on Brain Imaging Machine Learning (Online Live, 2022.7.16-19)
The 11th Basic Course on MRI Data Analysis Techniques (Online Live, 2022.8.15-18)
The 7th Practical Course on Multimodal MRI Brain Network Analysis Techniques (Online Live, 2022.8.25-28)
The 1st Practical Course on Task-Based fMRI Data Analysis Techniques (Online Live, 2022.8.18-25, Limited Spots Available)
fNIRS Training Courses
The 2nd Practical Course on Near-Infrared Brain Function Imaging Data Analysis (Online Class, 2022.7.8-12)
EEG/MRI/fNIRS Data Processing
Top Journal New Method Reproduction Service—Central Brain Network Model
Top Journal New Method Reproduction Service—Gradient of Time Scale in the Brain
Never Forget the Original Intention, Yueying Technology Helps Clients Publish High-Level SCI Papers One
Never Forget the Original Intention, Yueying Technology Helps Clients Publish High-Level SCI Papers Two
Never Forget the Original Intention, Yueying Technology Helps Clients Publish High-Level SCI Papers Three
Equipment Sales/Rental
Smarting: The First Truly Portable and Mobile EEG Device
MRI and EEG Equipment Sharing Services
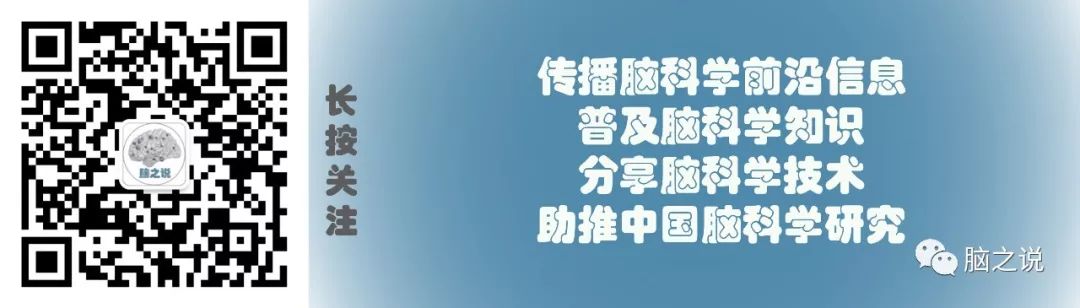
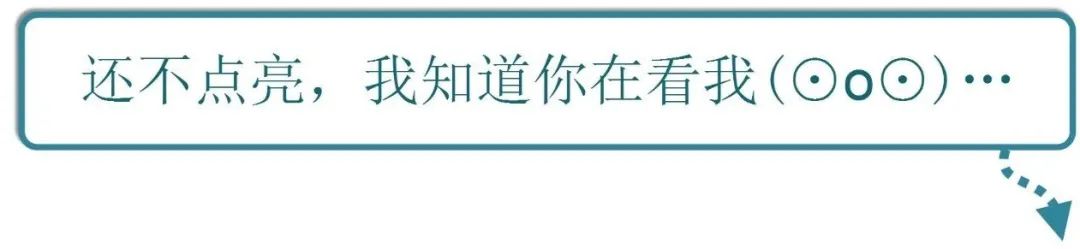