Click Blue Words · Follow Us
Professor Wu Xijun and others from the School of Electrical Engineering at Yanshan University utilized two-dimensional correlation spectroscopy (2D-COS) based on Raman spectroscopy combined with various deep learning techniques to propose a fast, non-invasive, and low-cost spectral strategy for quantifying honey adulteration, achieving quantitative analysis of cheap multifloral honey in Manuka honey. The related research results were published on July 25, 2023, in the international academic journal Food Control (IF= 6) under the title “Adulteration quantification of cheap honey in high-quality Manuka honey by two-dimensional correlation spectroscopy combined with deep learning.” Associate Professor Wu Xijun is the first author of the paper.
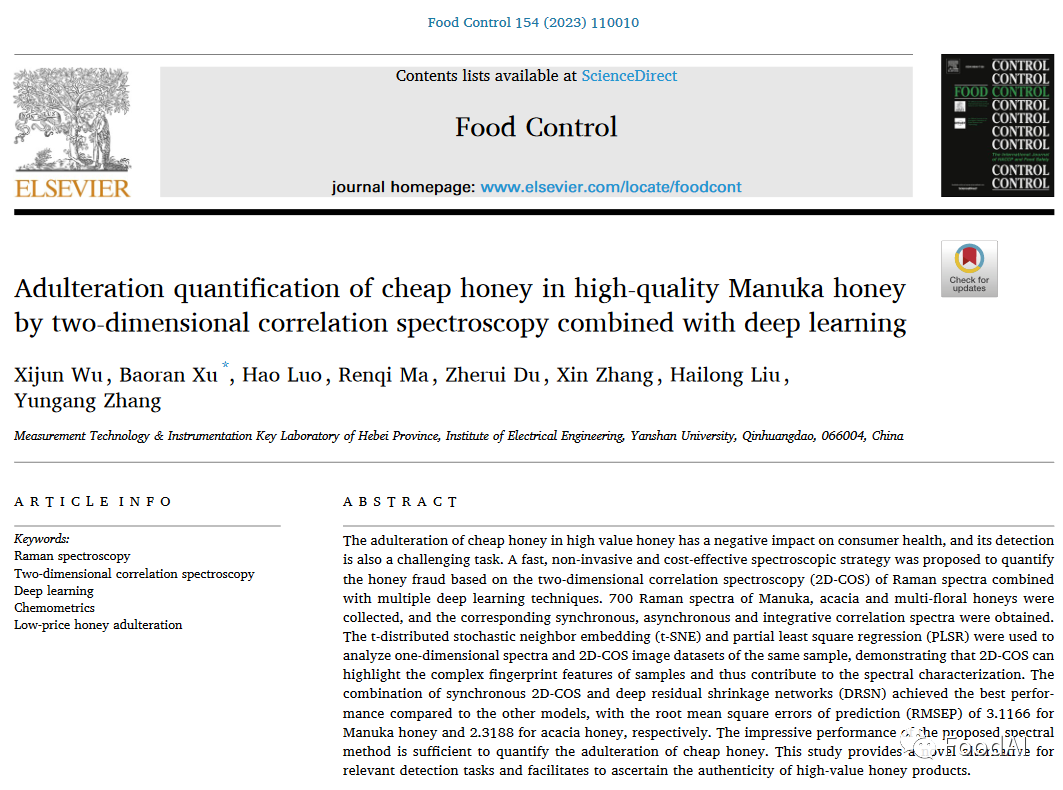
Summary of Results
Manuka honey is a type of increasingly popular and well-known monofloral honey worldwide, and its high selling price and growing demand have prompted food fraud in the market. To date, commonly used modern instrumental analysis methods such as high-performance liquid chromatography (HPLC), gas chromatography (GC), stable carbon isotope ratio analysis (SCIRA), mass spectrometry (MS), nuclear magnetic resonance (NMR), etc., have achieved excellent results in honey identification and adulteration detection. However, factors such as the complex and time-consuming sample pretreatment, the need for specialized personnel, and the high detection costs limit the large-scale application of these techniques. In contrast, spectroscopic methods, especially Raman spectroscopy, as a non-targeted analysis method, have advantages such as being green, fast, non-invasive, and economical, making them suitable for rapid detection of adulterated honey. This study proposed a method based on Raman spectroscopy and two-dimensional correlation spectroscopy (2D-COS) combined with various deep learning techniques to quantify honey adulteration. A total of 700 Raman spectra of Manuka honey, acacia honey, and multifloral honey were collected, obtaining the corresponding synchronous, asynchronous, and integrated correlation spectra. The t-distributed stochastic neighbor embedding (t-SNE) and partial least squares regression (PLSR) were employed to analyze the one-dimensional spectra and 2D-COS image datasets of the same sample, demonstrating that 2D-COS can highlight the complex fingerprint features of the samples, thereby aiding spectral characterization. Finally, a Deep Residual Shrinkage Network (DRSN) model was constructed for honey adulteration identification and compared with the performance of various other deep learning algorithms to fully exploit the data potential and obtain the most suitable model for honey adulteration identification.
Research Highlights
1. Combination of two-dimensional correlation spectroscopy (2D-COS) based on Raman spectroscopy with various deep learning techniques.
2. Obtained and compared synchronous, asynchronous, and comprehensive correlation spectra of the samples.
3. Utilized Deep Residual Shrinkage Network (DRSN) to overcome gradient vanishing and explosion in the network.
4. The proposed method can quantify the adulteration of cheap honey in Manuka and acacia honey.
Visual Appreciation
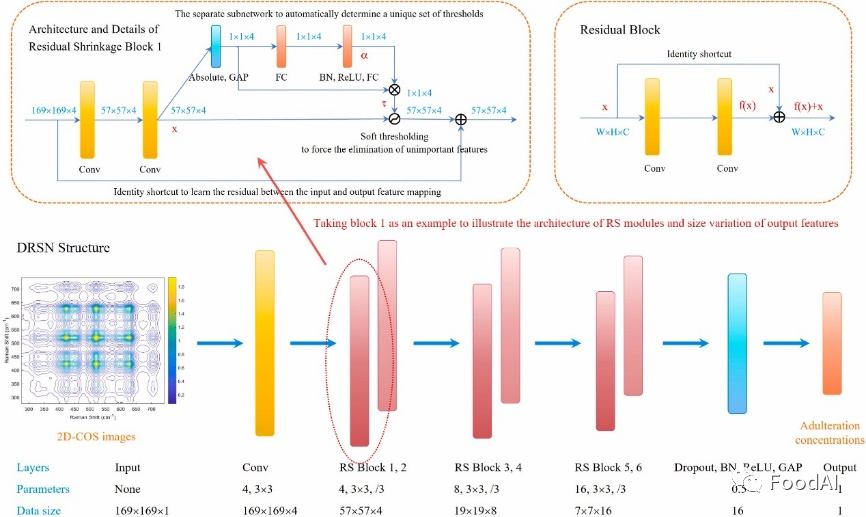
Figure 1. Architecture and parameter schematic diagram of the DRSN model for quantifying adulteration.
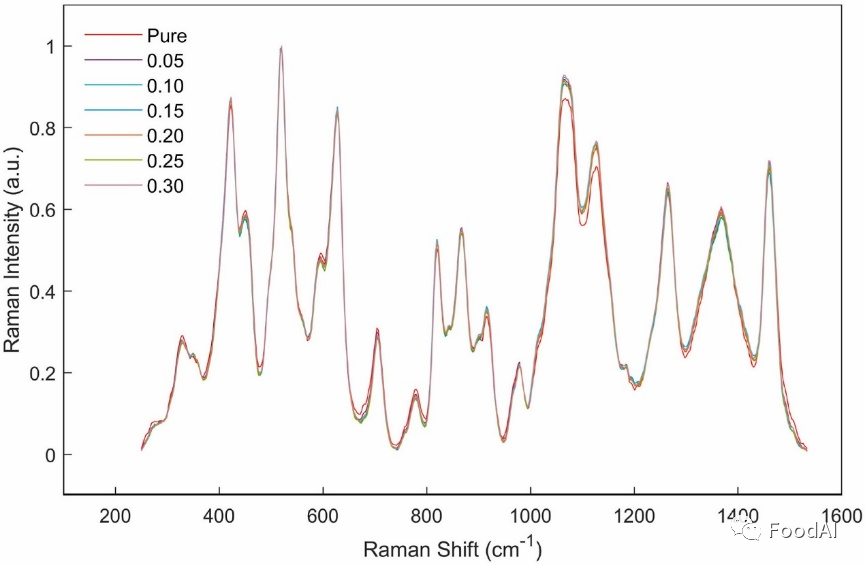
Figure 2. Pre-processed Raman spectra of Manuka honey adulterated with different concentrations of multifloral honey.
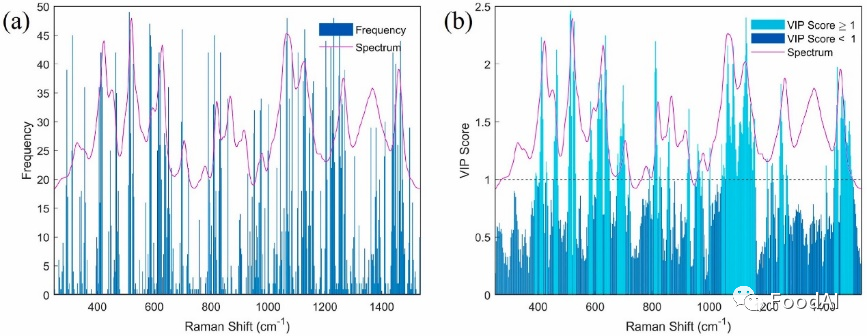
Figure 3. (a) Frequency diagram of key wavenumbers selected in multiple runs; (b) Indicative diagram of VIP scores for each wavenumber.
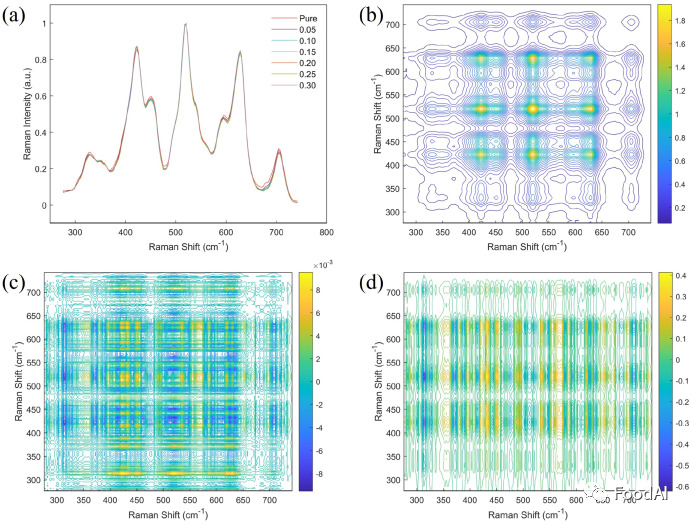
Figure 4. Fingerprint spectral bands (a) of specific honey samples and their corresponding synchronous (b), asynchronous (c), and integral (d) correlation spectra.
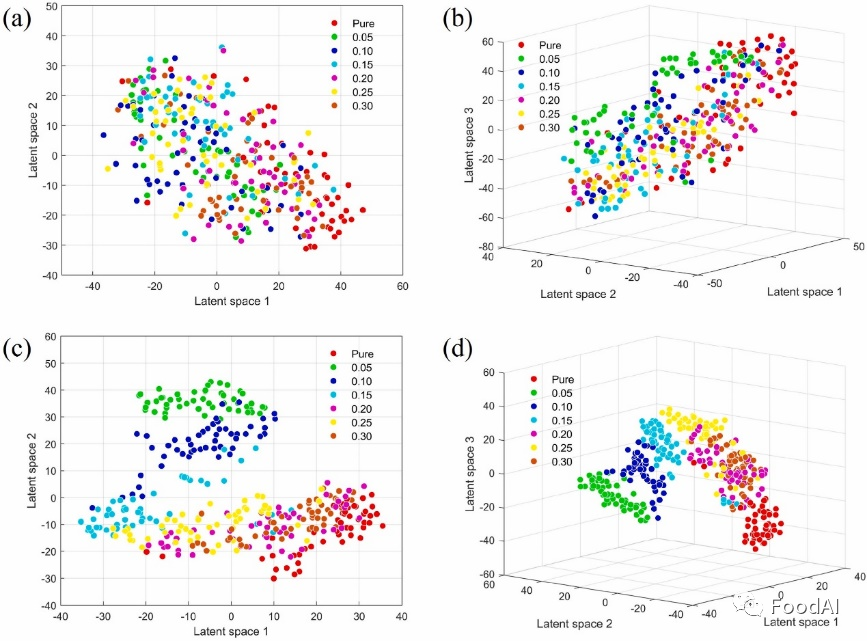
Figure 5. Visualization results of Raman spectra (a, b) and synchronous correlation spectra (c, d) datasets of Manuka honey adulterated with multifloral honey based on t-SNE in two-dimensional and three-dimensional latent spaces.
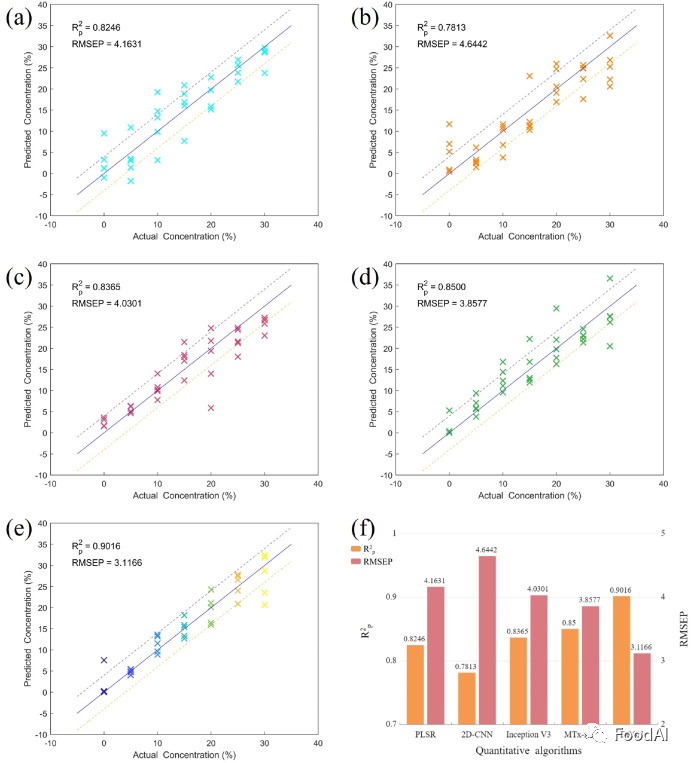
Figure 6. Quantitative results of PLSR (a), 2D-CNN (b), InceptionV3 (c), MTx-Net (d), and DRSN (e) models for detecting low-value honey adulteration in Manuka honey, as well as a comparison of quantitative performance of different algorithms (f). The solid line represents the fitting curve where predicted values equal actual values, and the dashed line represents the fitting line with an error of ±4%.
# Research Conclusion #
This study proposes a green, non-destructive, and cost-effective spectral analysis strategy based on the combination of synchronous correlation spectroscopy of Raman spectra and various deep learning architectures to quantify the adulteration of cheap honey in Manuka and acacia honey. A total of 700 Raman spectra were collected, and key fingerprint spectral bands were determined through CARS and VIP. Synchronous, asynchronous, and comprehensive correlation spectra were calculated and obtained. The t-SNE and PLSR algorithms were used to analyze the one-dimensional spectra and 2D-COS images, and the results indicate that synchronous spectra can highlight the abstract and complex fingerprint features of the samples, aiding spectral characterization and analysis. The visualization results based on t-SNE also provide an overall visualization scheme for the spectral dataset. Among various algorithms, the prediction results of DRSN significantly outperformed other quantitative algorithms such as PLSR, 2D-CNN, InceptionV3, and MTx-Net. Overall, the method combining Raman synchronous spectra with DRSN achieved excellent performance in detecting adulteration in Manuka and acacia honey. This spectral method is sufficient to detect cheap honey adulteration in high-quality honey, and this strategy can also be applied to more complex food matrices for difficult and challenging detection tasks.
Original Link
https://doi.org/10.1016/j.foodcont.2023.110010
Colloids, Emulsions, and Delivery Systems Polysaccharides, Fibers, Oligosaccharides, etc. Lipids
Food Proteins, Peptides, and Amino Acids Polyphenolic Compounds Starch
Food Packaging and Shelf Life Food Safety and Quality Control Gels
Food Nutrition and Human Health Food Testing and Analysis Tea Alcohol Bee Products
Food-Related Synthesis Artificial Meat Food Flavor Prebiotics, Probiotics, and Synbiotics
3D and 4D Printing Food Allergies Food Sensory Science and Analysis
Flavonoids Agricultural Products Storage and Processing
Meat and Meat Products Eggs and Egg Products Aquatic Products Dairy Products
Beans and Bean Products Fruits, Vegetables, and Related Products Rice and Rice Products Mushrooms
Inflammatory Bowel Disease Diabetes Liver Disease Neurological Diseases
ThankYouForYourAttention ForMoreInformationVisitOurWebsite
Thisarticleisforacademicexchangeandinformationdisseminationneedstobeapprovedbytheauthorsofthisarticleandthecopyrightbelongstotheauthorsandshouldnotbereproducedorpublishedwithoutpermissionfromtheauthorsofthisarticleisstrictlyprohibited.