2021 Issue 4
Public Sentiment Analysis of Meteorological Disasters Based on LSTM-BLS
Jia Luo1 Lehao Wang2 Shanshan Tu1 Ge Song1 Ying Han2
1Hubei Provincial Public Meteorological Service Center, Wuhan, 430074
2Nanjing University of Information Science and Technology, School of Automation, Nanjing, 210044

Abstract:In recent years, Long Short-Term Memory (LSTM) networks have shown certain advantages in text sentiment analysis, but LSTM has issues with incomplete semantics and low accuracy when extracting features. Researchers often introduce Convolutional Neural Networks (CNN) to compensate for these shortcomings, yet the syntactic dependency between words is still not considered. This paper proposes an LSTM-BLS text sentiment analysis model by integrating the Broad Learning System (BLS) based on incremental learning algorithms with LSTM, and analyzes public sentiment tendencies during sudden meteorological disasters using the cliff-like cooling event of 2020 as an example. The results show that compared to the baseline models K-means and Support Vector Machine (SVM), the accuracy of the LSTM-BLS model improves by 17.23 and 13.46 percentage points, respectively; compared to existing deep models LSTM and CNN-LSTM, the accuracy of this model improves by 7.13 and 4.17 percentage points, respectively.
Keywords:Meteorological disasters; Broad Learning System; Long Short-Term Memory; Sentiment analysis;

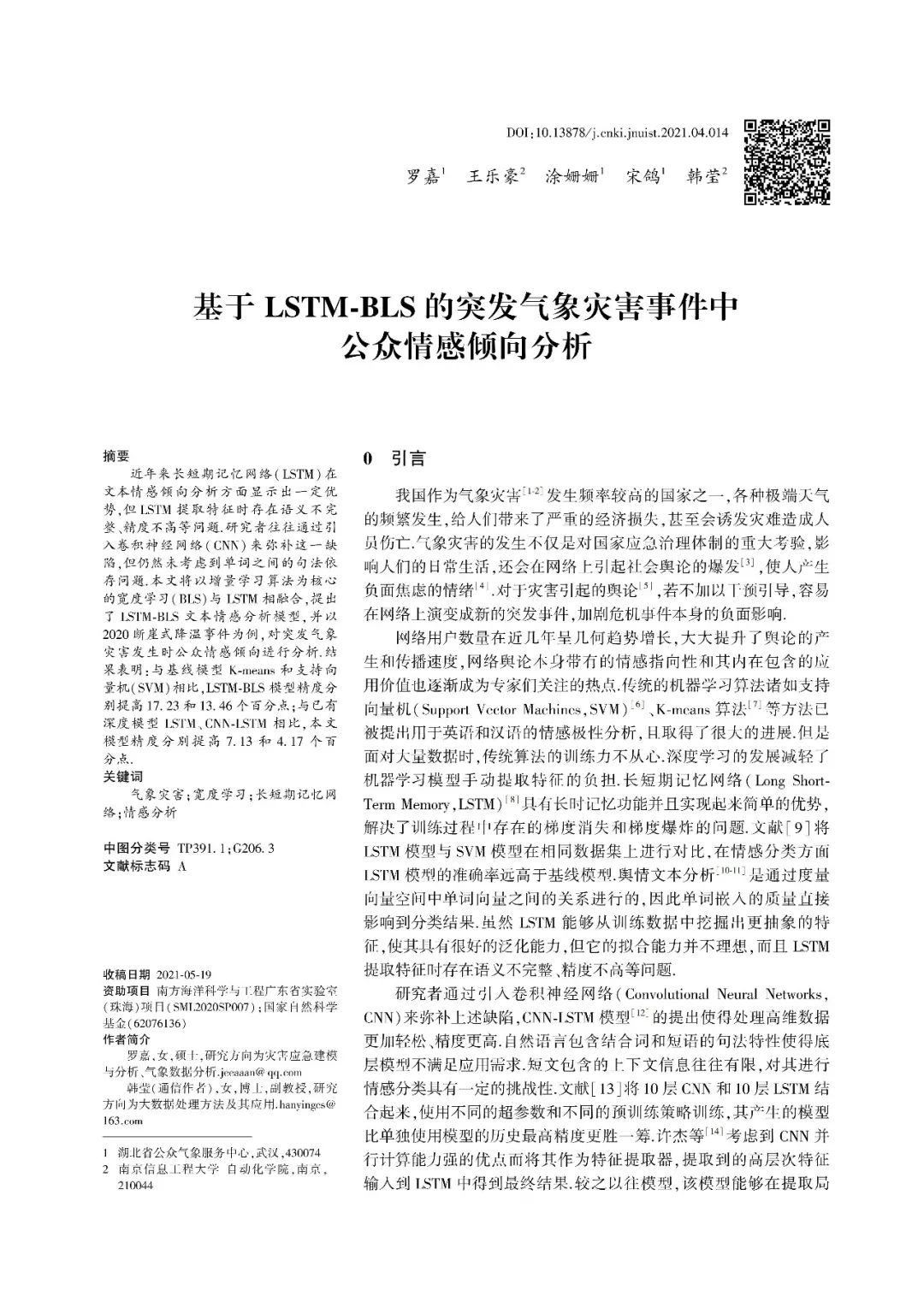
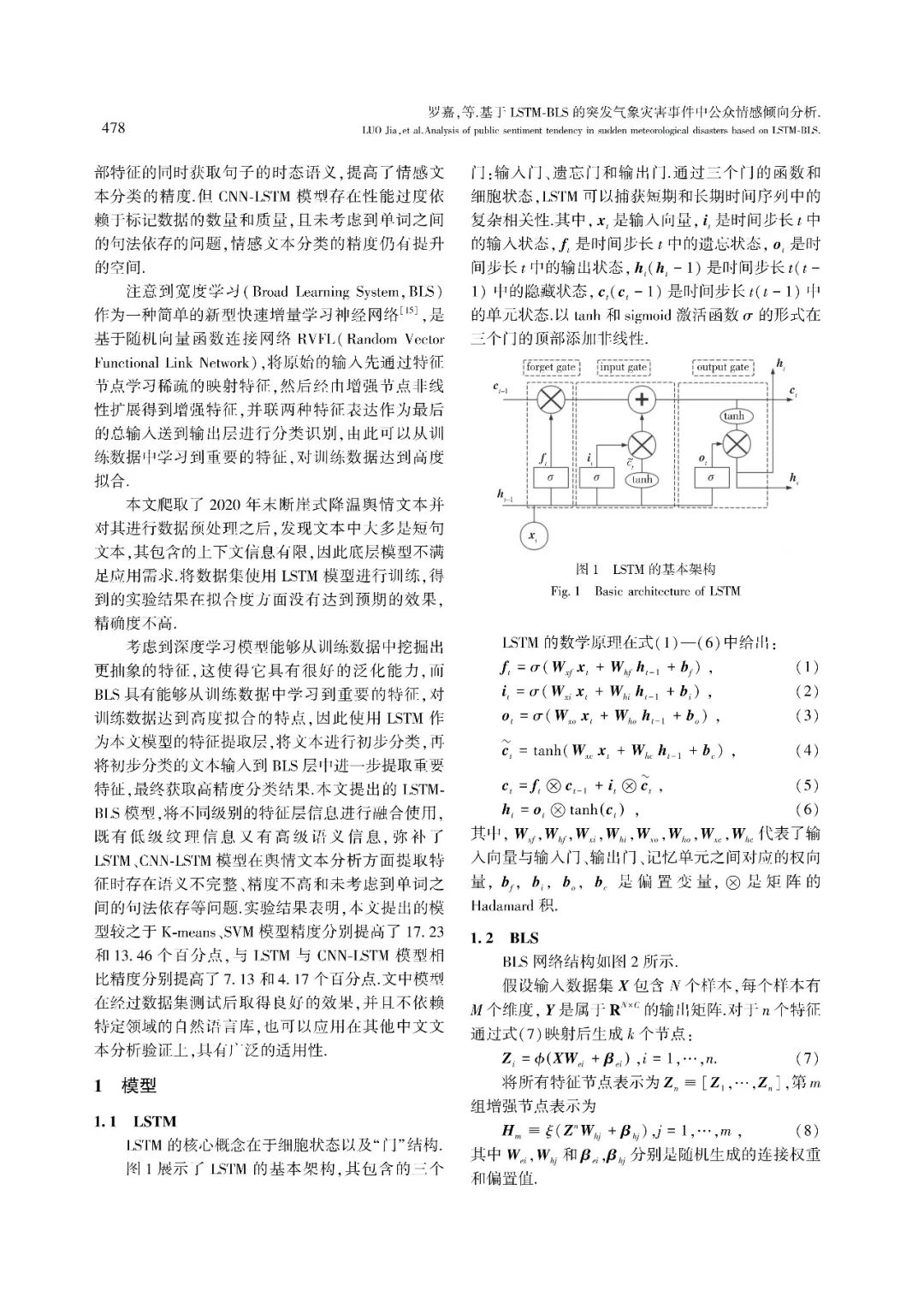
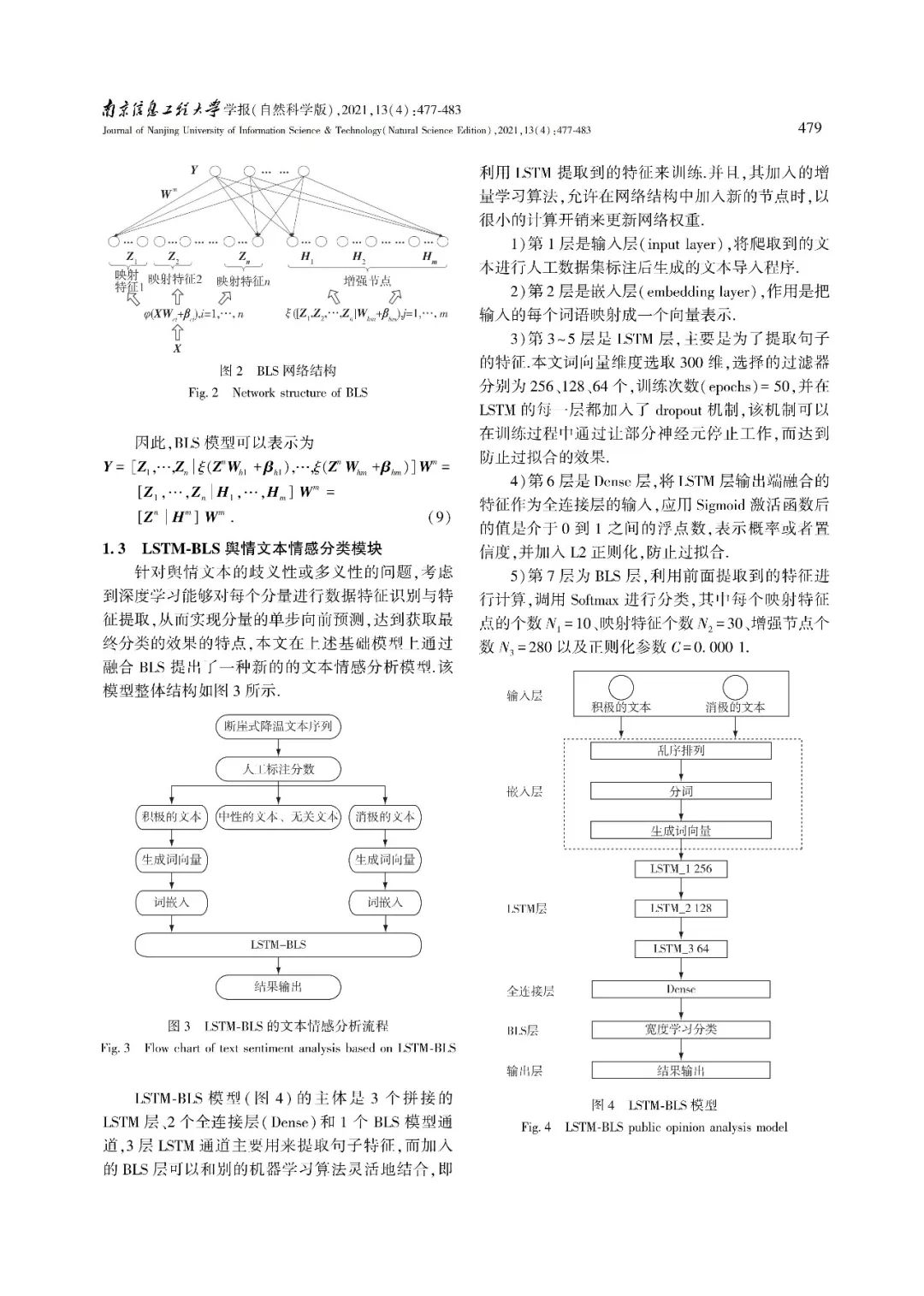
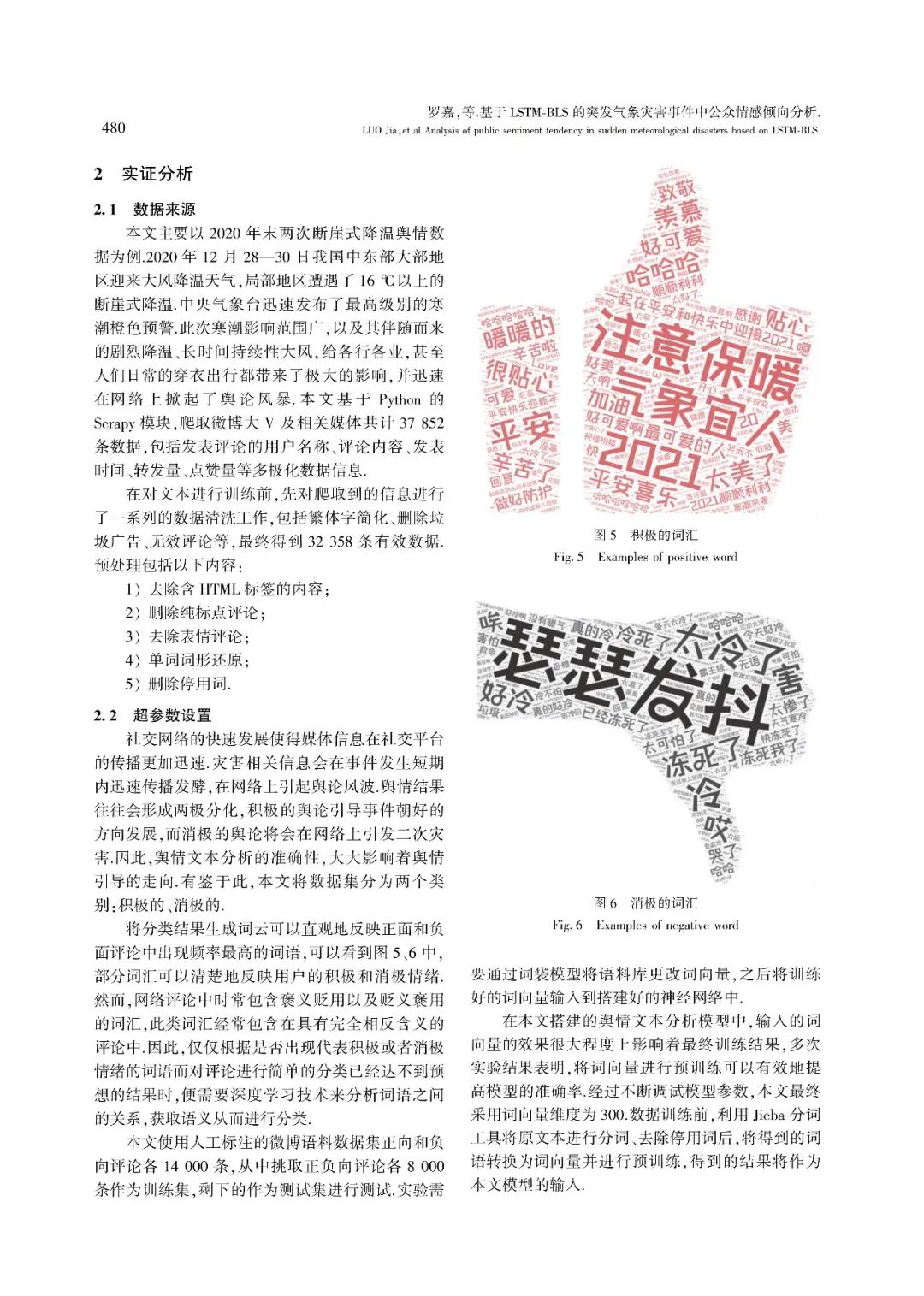
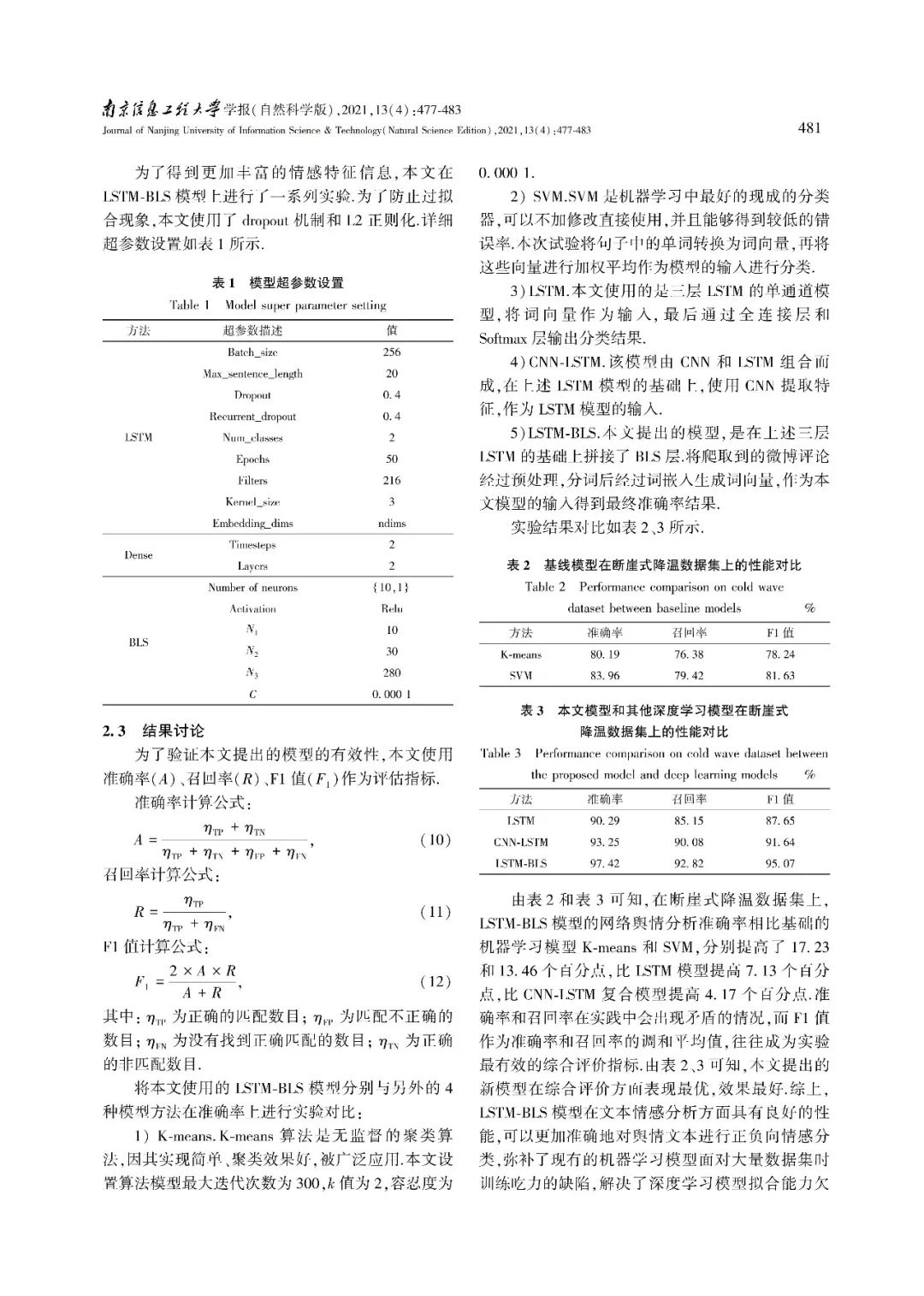
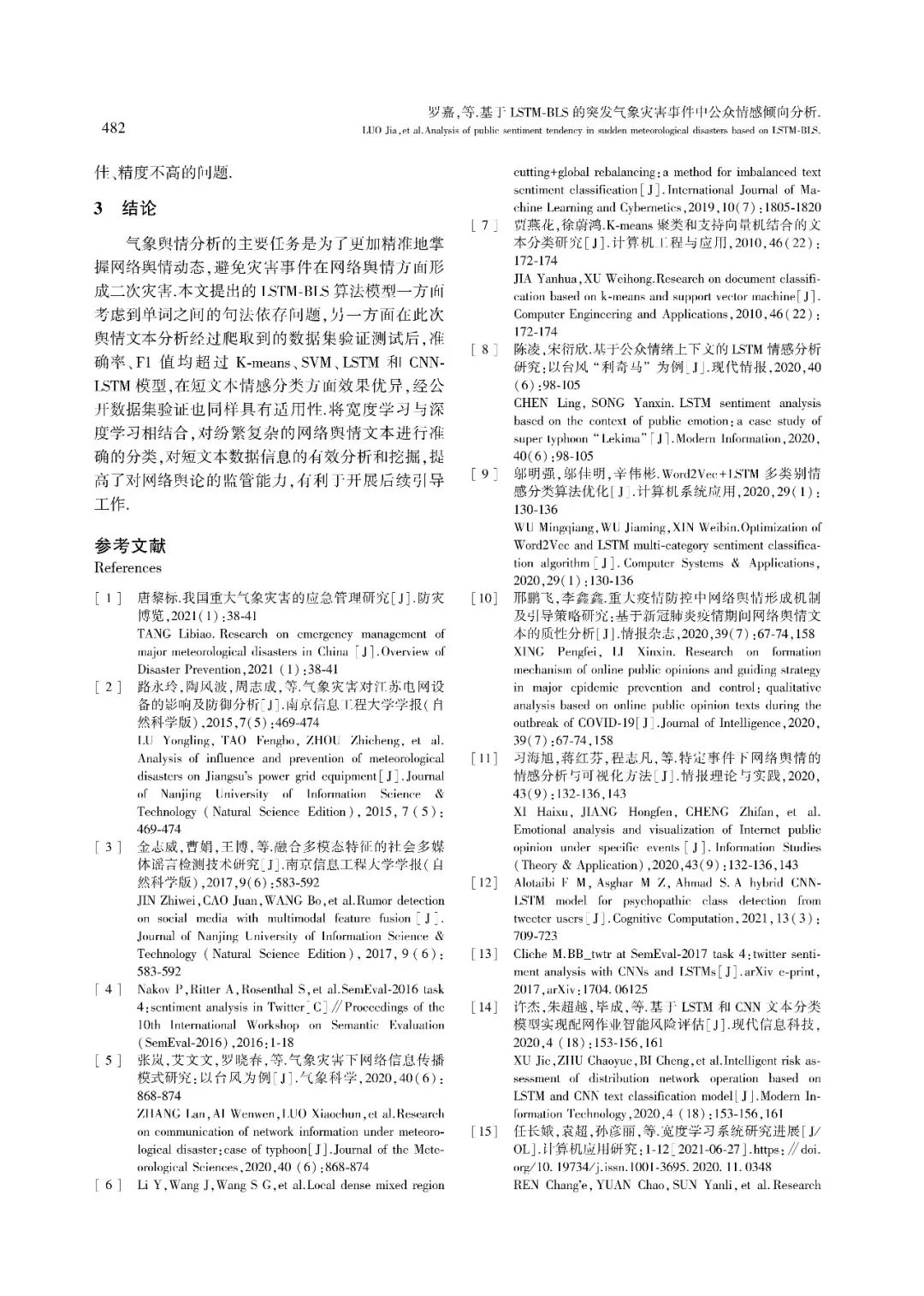
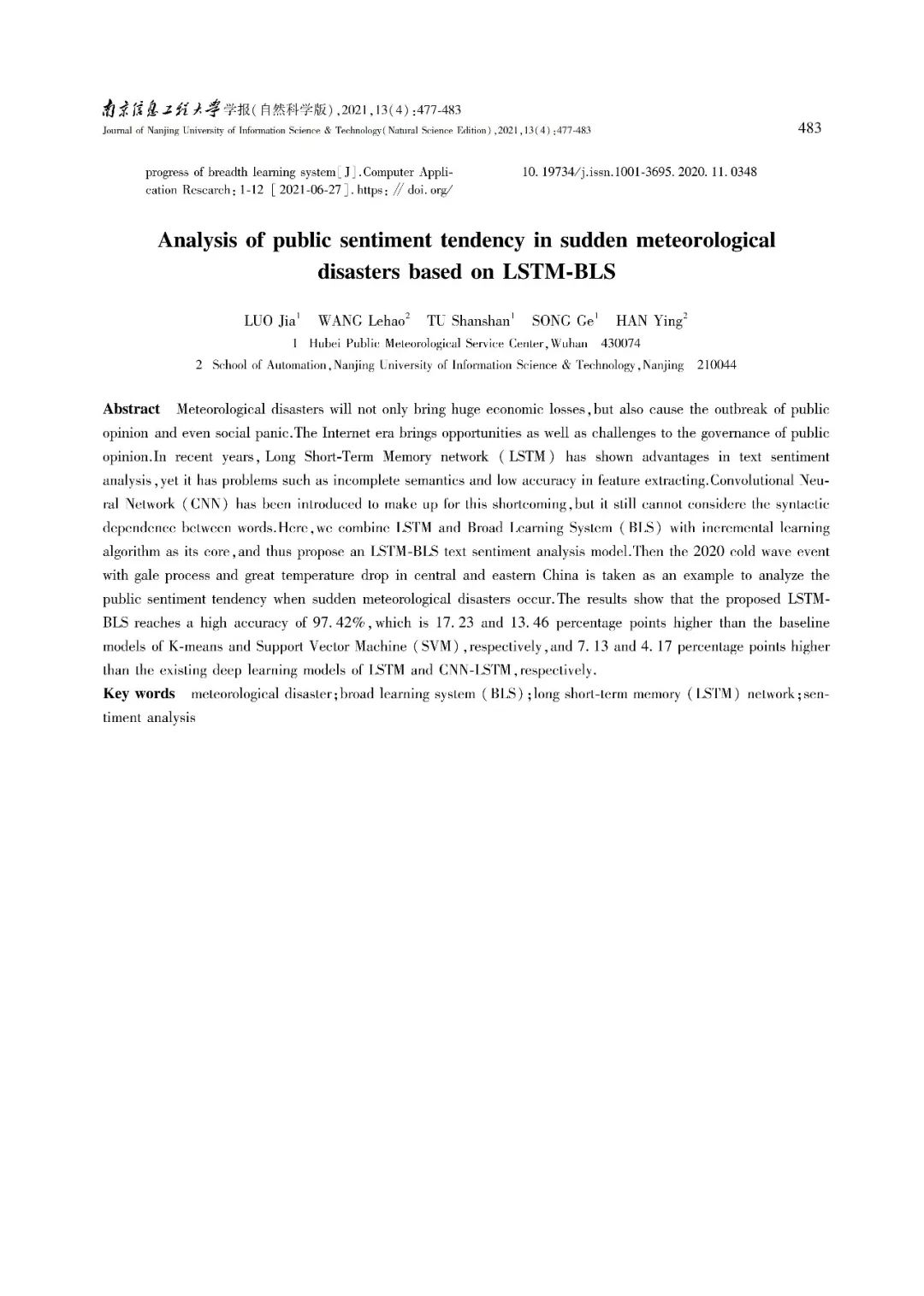
(Swipe left to read the full text)
This issue’s journal link: 【Table of Contents】 Overview of the 2021 Fourth Issue of the Journal of Nanjing University of Information Science and Technology
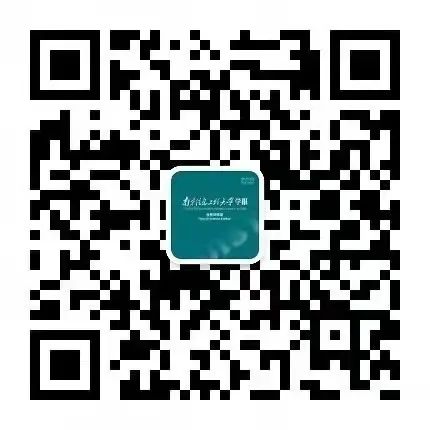
Journal of Nanjing University of Information Science and Technology
Tracking Technological Frontiers, Focusing on Discipline Directions
Both Theory and Application
Upholding Academic Standards, Creating High-Quality Journals
Read the Original Text
Learn More