01
Introduction
2022 was a breakout year for AIGC (AI-Generated Content), gaining popularity among consumers and attracting significant attention from the investment community, as well as being pursued by the technology and industry sectors. On September 23, Sequoia Capital published an article titled “Generative AI: A Creative New World,” suggesting that AIGC represents the beginning of a new paradigm shift.
In October 2022, Stability AI secured approximately $100 million in funding, achieving a valuation of $1 billion and entering the unicorn club. In December 2022, OpenAI’s large language generation model ChatGPT went viral, capable of handling high-emotional intelligence conversations, generating code, and conceptualizing scripts and novels, elevating human-machine dialogue to new heights. Major tech companies worldwide are actively embracing AIGC, continuously launching related technologies, platforms, and applications.
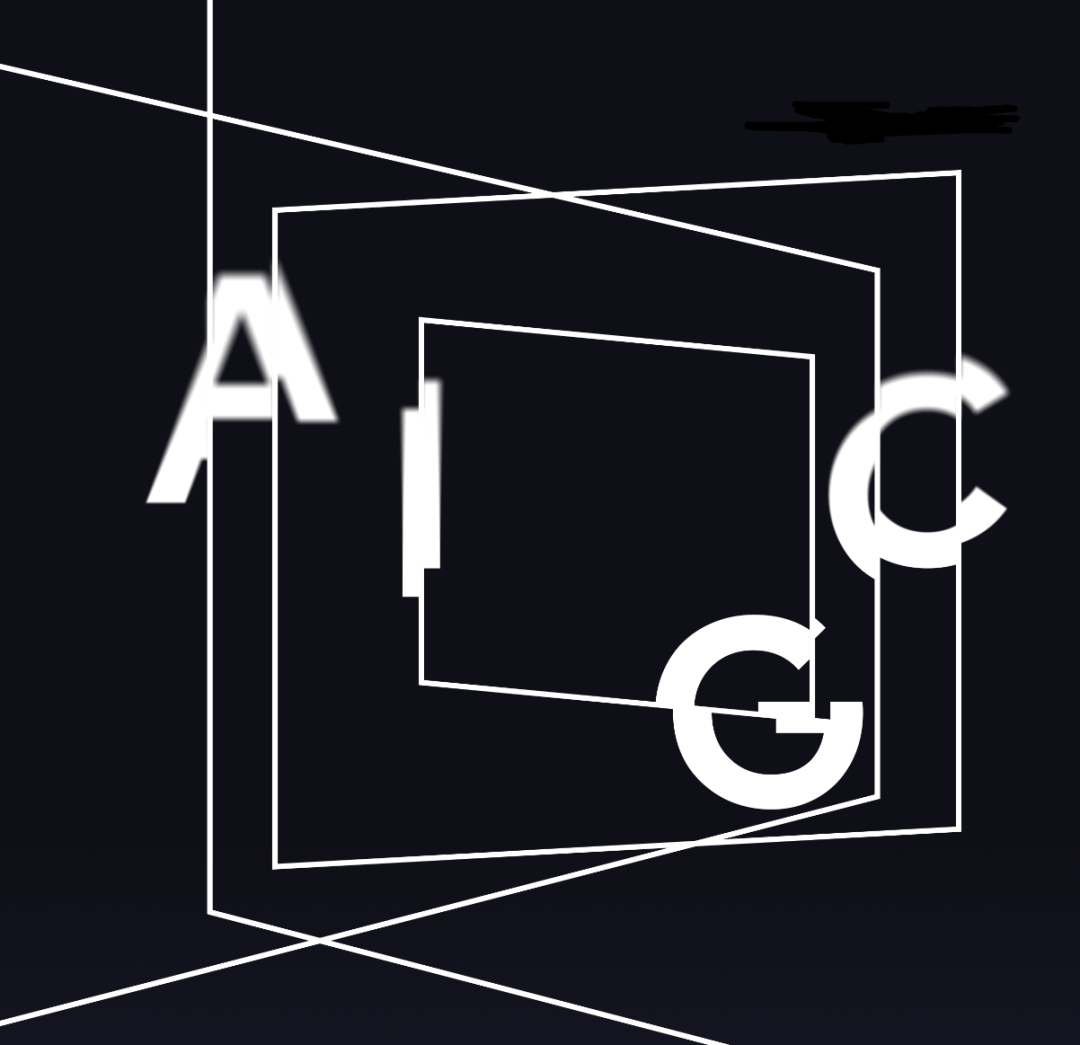
02
What is AIGC?
Literally speaking, AIGC is proposed in contrast to past PCG and UGC. Thus, the narrow definition of AIGC refers to the production method of automatically generating content using AI.
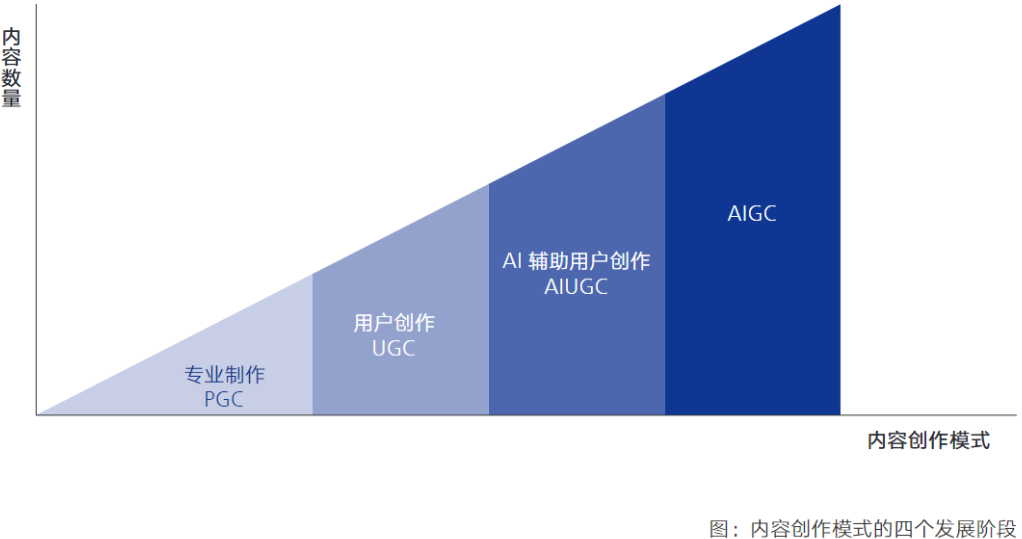
However, AIGC already represents a new trend in AI technology development. Traditionally, artificial intelligence has focused on analytical capabilities, discovering patterns and rules from a set of data for various applications, such as the widely used personalized recommendation algorithms. Now, artificial intelligence is generating new things rather than just analyzing existing ones, achieving a leap from perceiving and understanding the world to generating and creating it. Therefore, in this sense, the broad definition of AIGC can be seen as AI technology that possesses generative and creative capabilities like humans, namely generative AI, which can independently generate new text, images, music, videos, 3D interactive content (such as virtual avatars, virtual items, virtual environments), and other forms of content and data based on training data and generative algorithm models, as well as initiate scientific discoveries and create new values and meanings.
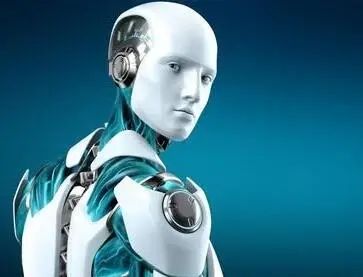
Therefore, AIGC has accelerated its emergence as a new domain in the field of AI, ushering in the next era of artificial intelligence. Gartner listed generative AI as one of the five most influential technologies of 2022. MIT Technology Review also included AI synthetic data as one of the ten breakthrough technologies of 2022, even calling generative AI the most promising advancement in the field of AI in the past decade. In the future, AIGC models that combine large models and multimodal models are expected to become a new technological platform.
03
AIGC Industry Ecosystem Architecture
Currently, the embryonic form of the AIGC industry ecosystem has emerged, presenting a three-tier architecture.
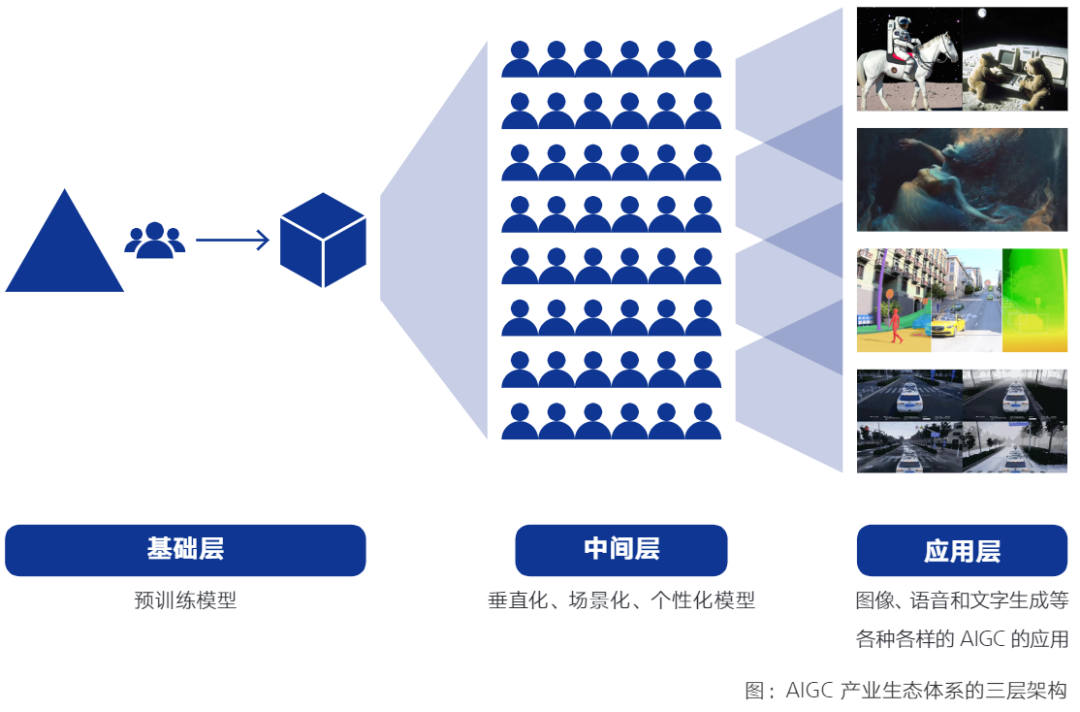
Basic Layer
The first layer is the upstream basic layer, which is the AIGC technology infrastructure layer built on pre-trained models. Due to the high costs and technical investments associated with pre-trained models, there is a high entry barrier. For example, the cost of training the GPT-3 model launched in 2020 is estimated by Alchemy API founder Elliot Turner to be close to $12 million. Therefore, currently, the main institutions entering the pre-trained model space are leading technology companies and research institutions.
Currently in the AIGC field, the infrastructure companies in the United States (positioned in the upstream ecological niche) include OpenAI and Stability.ai, etc. With the support of the basic layer’s technology, downstream industries can develop rapidly, forming the current AIGC commercial flow in the United States.
Middle Layer
The second layer is the middle layer, which consists of vertical, scenario-based, and personalized models and application tools. The pre-trained large model serves as the infrastructure, upon which smaller models can be quickly extracted and generated for specific scenarios and customization, achieving industrial assembly line deployment in different industries, vertical fields, and functional scenarios, while also possessing on-demand use and efficient economic advantages.
Since the open-sourcing of Stable Diffusion, many secondary developments based on open-source models have become popular, such as the well-known Novel-AI for generating two-dimensional art styles, as well as various character generators of different styles.
Application Layer
The third layer is the application layer, which provides content generation services for end users, including text, images, audio, and video. In the application layer, the emphasis is on meeting user needs, seamlessly connecting AIGC models with user demands to achieve industrial implementation. For example, with the open-sourcing of Stable Diffusion, it not only provides the program but also the already trained models, allowing subsequent entrepreneurs to better utilize this open-source tool. With the computing power threshold of consumer-grade graphics cards, it plays a crucial role in enriching the content ecosystem and promoting the wider adoption of AIGC among end users.
Now, tools that are close to end users are becoming increasingly rich and diverse, including web applications, locally installed programs, mobile mini-programs, group chat bots, and even content consumption services that customize and generate images using AIGC tools.
04
AIGC Applications — Content Production
Content production, especially creative work, has always been regarded as a human exclusive and a manifestation of intelligence. Now, AI is stepping into the digital content production field, achieving “human-like” performance in writing, painting, composing, and more, showcasing extraordinary creative potential based on big data learning. This will shape a new paradigm of human-machine collaboration in digital content production, allowing content creators and more ordinary people to transcend the limitations of “skills” and “effectiveness” to unleash their content creativity.
In terms of scope
AIGC is gradually deeply integrated into the production of text, music, images, video, and 3D across various media forms, serving as news writers, academic paper and novel authors, music composers and arrangers, diverse style artists, short and long video editors and post-processing engineers, 3D modelers, and other diverse assistant roles, completing the creation, editing, and style transfer of specified thematic content under human guidance.
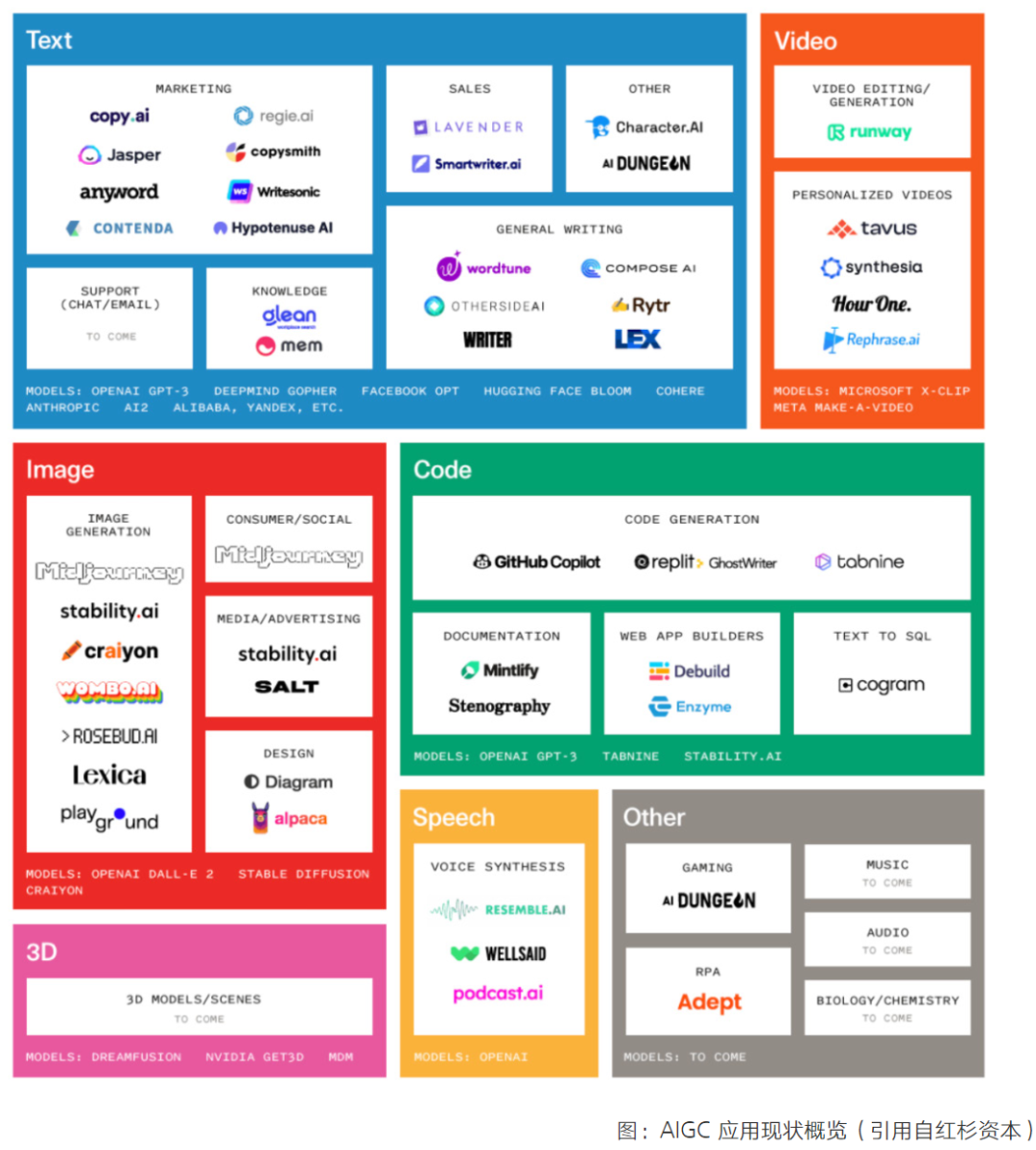
In terms of effectiveness
AIGC has initially shown satisfactory results in generating text, speech, and images based on natural language, particularly in knowledge-based short articles, illustrations, and highly stylized image creations, with creative results comparable to those of moderately experienced creators; in the realm of video and 3D, which have higher complexity, it is still in the exploratory stage but is growing rapidly. Although AIGC still has much room for improvement in handling extreme cases, detail control, and product accuracy, its potential is promising.
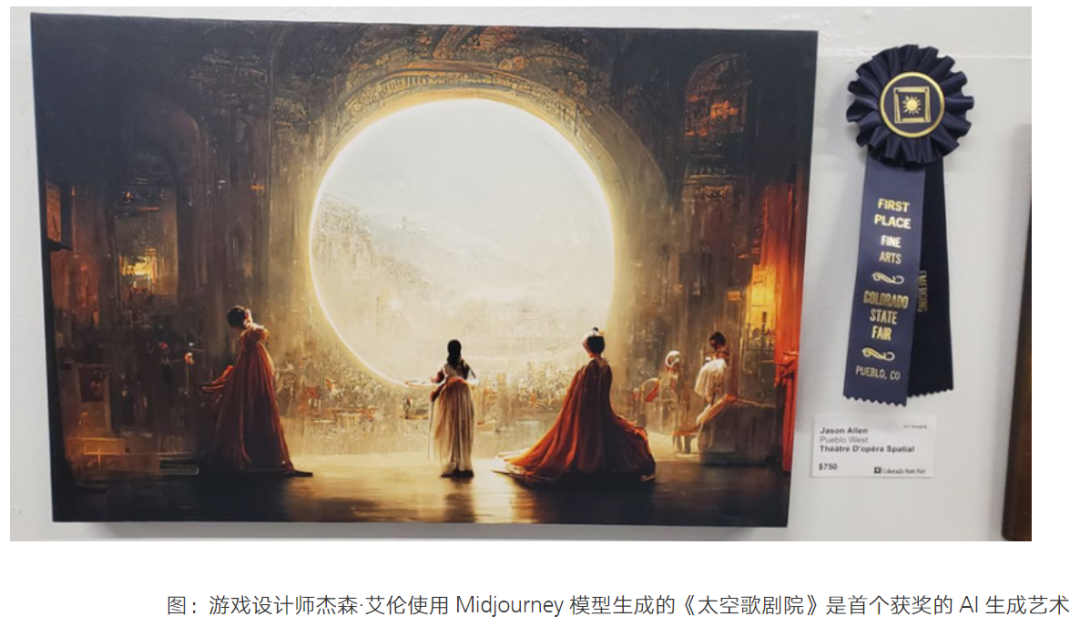
In terms of methods
The multimodal processing of AIGC is a hot topic. Andrew Ng believes that multimodality is the most important trend in AI in 2021, with AI models making progress in discovering relationships between text and images, such as OPEN AI’s CLIP matching images and text, Dall.E generating images corresponding to input text; DeepMind’s Perceiver IO can classify text, images, videos, and point clouds. Typical applications include text-to-speech conversion, text-to-image generation, and broadly speaking, AI translation and image stylization can also be seen as mapping between two different “modalities”.
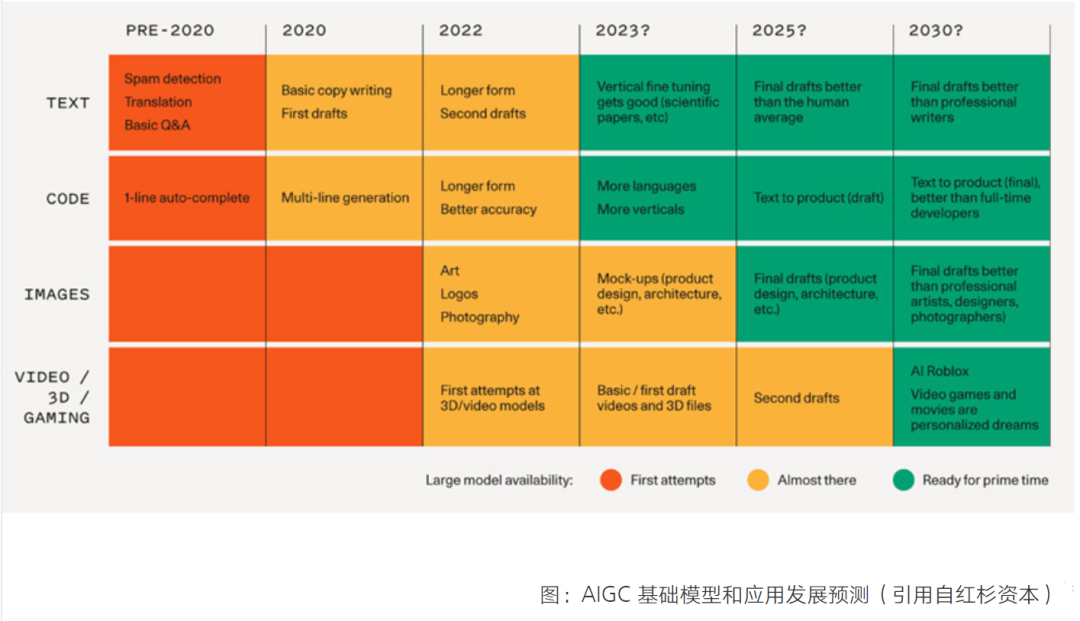
05
Advantages of AIGC
In the field of content consumption, AIGC has restructured the entire application ecosystem. AIGC has the following advantageous features in content generation:
(1) Automatic content generation: Large language and image AI models can be used to automatically generate content, such as articles, blogs, or social media posts. This can be a valuable time-saving tool for businesses and professionals who regularly create content.
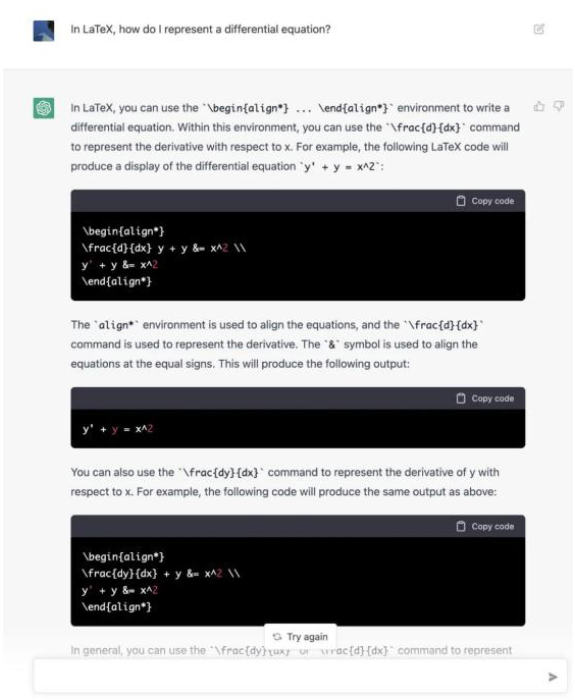
(2) Improved content quality: The content generated by AIGC may be of higher quality than that created by humans, as AI models can learn from vast amounts of data and recognize patterns that humans may miss. This can result in more accurate and informative content.
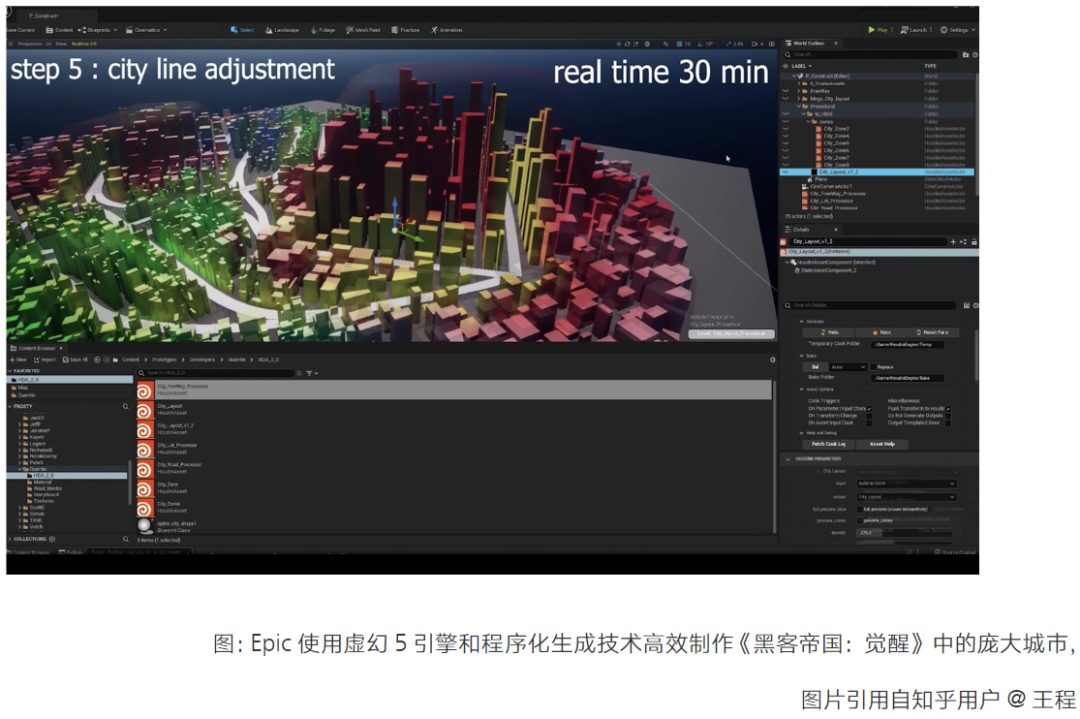
(3) Increased content diversity: AIGC models can generate various types of content, including text, images, audio, video, and 3D content. This can help businesses and professionals create more diverse and engaging content, attracting a broader audience.
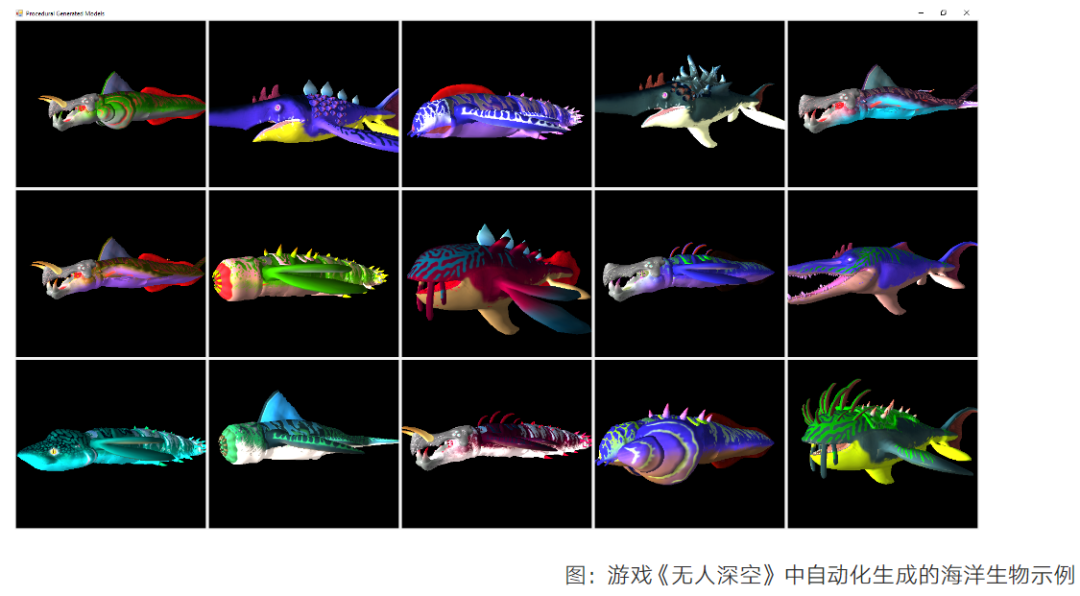
(4) Lower content production costs and barriers: Based on AIGC, the cost of content production is significantly reduced, and efficiency is greatly improved, allowing for the creation of unique content with independent perspectives at a fraction of the cost and at hundreds to thousands of times the production speed. Moreover, AIGC also lowers the threshold for users to produce content, enabling even those with no drawing skills to create high-quality works using AIGC tools.
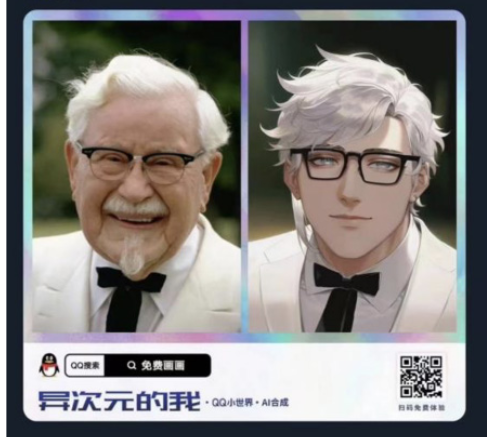

(5) Personalized content generation: AI models can generate personalized content based on individual user preferences. This can help businesses and professionals create content that is more likely to interest their target audience, thus increasing the chances of being read or shared. For example, the Novel-AI tool based on Stable Diffusion can cater to the preferences and content needs of niche two-dimensional communities, to some extent promoting the development of niche cultures.
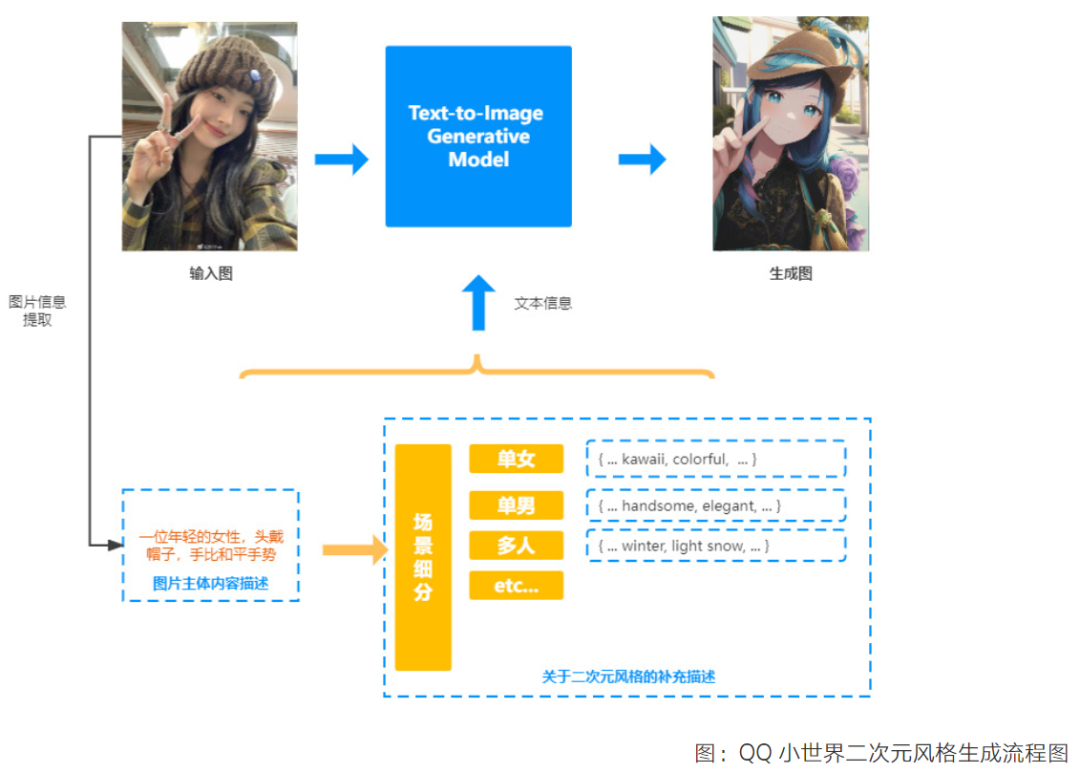
06
Future Development Trends of AIGC
3D Internet
One important direction for the next generation of internet technology upgrades and evolution is moving from “online” to “presence”, stepping into the 3D internet era, where AIGC will become the cornerstone for creating an integrated virtual and physical world. People will build simulated worlds in virtual spaces, overlaying virtual enhancements in the real world, achieving a true sense of presence. With breakthroughs in various interaction, simulation, and transmission technologies such as XR, game engines, and cloud gaming, information transmission is getting closer to lossless, and the ability for digital simulation to be indistinguishable from reality will take human interaction and experiences to a new stage.
At this stage, AIGC’s breakthroughs in the 3D field mainly involve transforming images and existing objects through AI intervention. Advances in computer vision technology are paving new paths for 3D: we can infer the shapes of objects in 3D space from images, restoring the original depth information of each pixel as much as possible. Using devices like LiDAR to scan the physical world allows for the rapid acquisition of a large number of 3D models.
Currently, AIGC in the 3D model field is still in the exploratory stage. From the basic principles and models used, it is still based on diffusion models, processing along the text-image-3D path. Google and NVIDIA are leading in this field, having released their own text-to-3D AI models in 2022. However, in terms of generation quality, there is still a distance from the average quality of manually created 3D content, and the generation speed is also unsatisfactory.
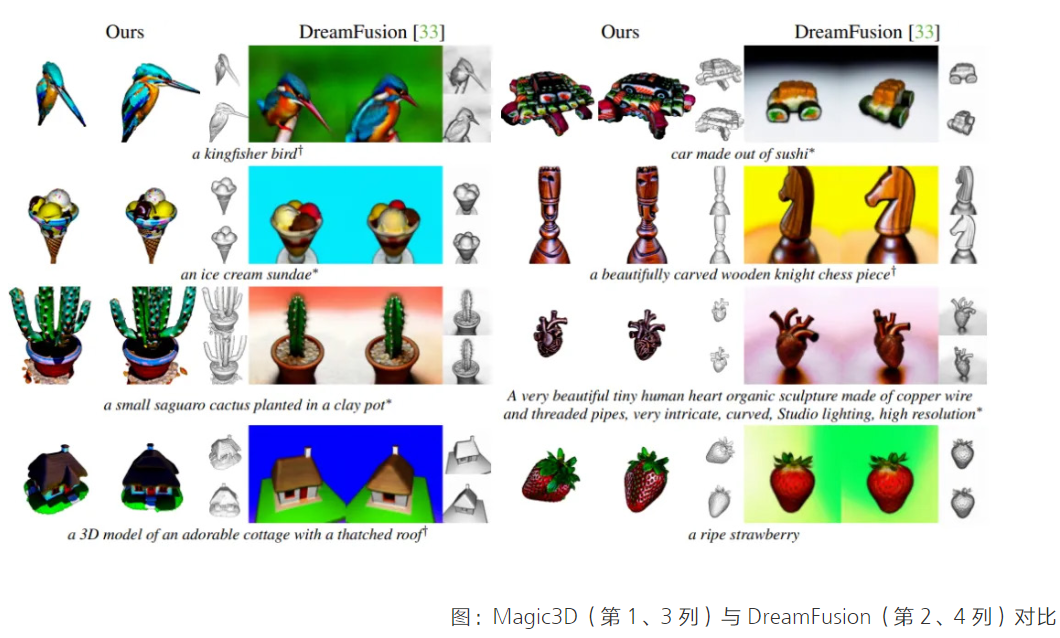
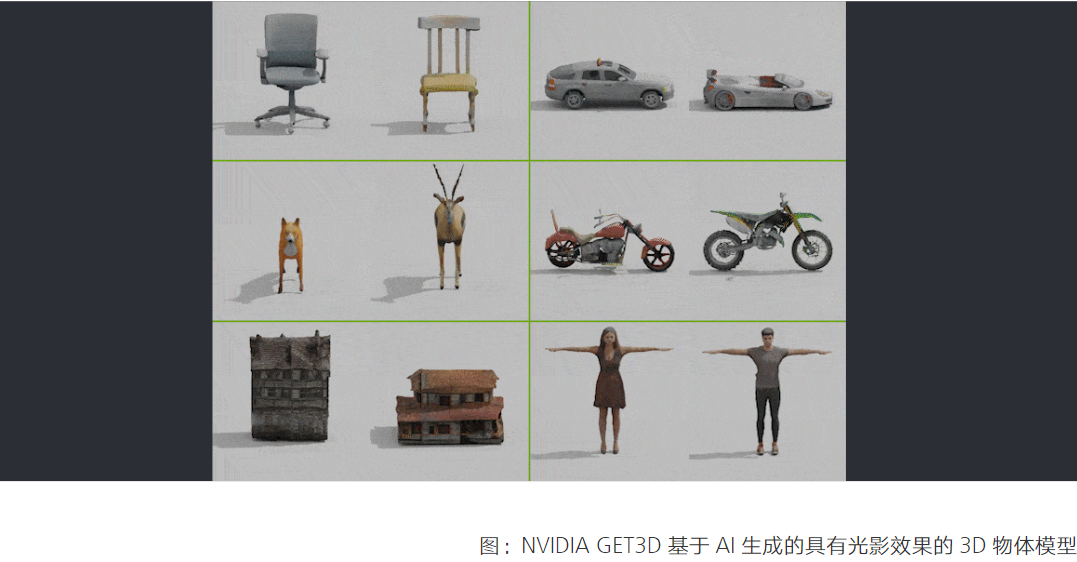
Digital Humans
Digital humans and virtual robots are digital agents, representing a new form of interaction. There are already many applications, including NPC virtual characters in the metaverse and users’ virtual avatars, which can be divided into human-driven and AI-driven types. Here, we mainly discuss AI-driven digital humans.
First, AIGC greatly enhances the efficiency of creating digital humans. Users can upload photos/videos to generate realistic digital humans with a shorter generation process, lower costs, and customization features. 3D digital human modeling based on AIGC has initially achieved productization, with precision reaching next-generation game character levels. Its advantage lies in the ability to open program interfaces for various applications, thus having significant potential and broad scope, especially in C-end applications, where users can generate 3D digital human facial models simply by uploading images.
The future direction is to improve accuracy and optimize modeling effects through algorithmic enhancements, such as offset correction and shadow restoration. In generating and creating user avatars, the AI engine can analyze users’ 2D images or 3D scans to create highly realistic simulations, while incorporating facial expressions, emotions, hairstyles, and age characteristics to make users’ virtual images more vibrant.
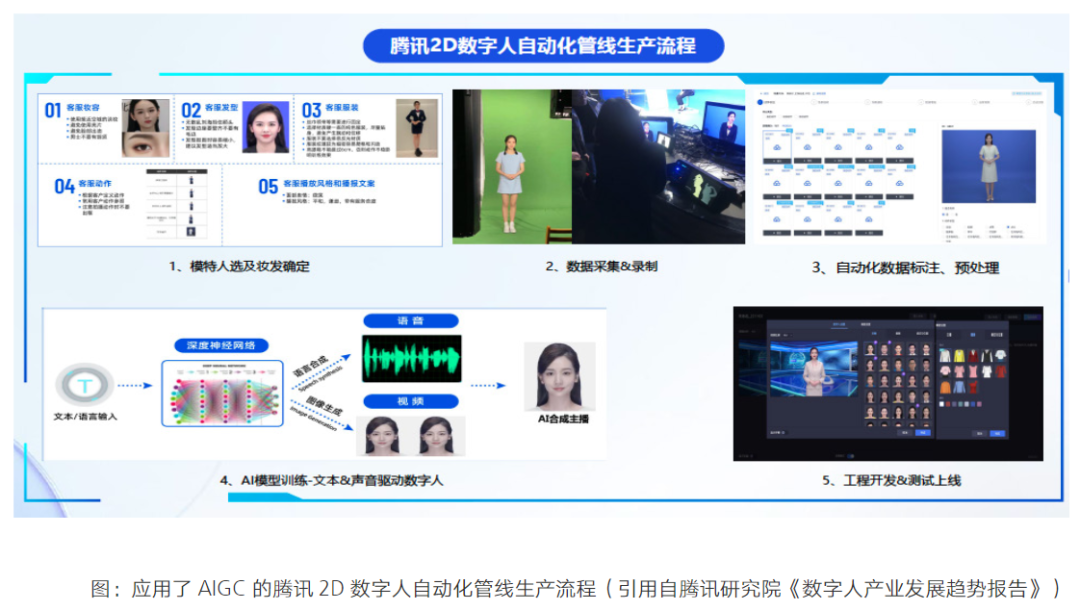
Metaverse
One of the most exciting features of the metaverse is its potential for scalability, which will greatly expand human living space in the virtual world. However, the question arises: who will build this vast world? How will it be filled with content? Creating high-fidelity 3D models or virtual humans typically requires many computer scientists and digital artists. Faced with the massive digital content required for this space, relying solely on human design and development is insufficient, and AIGC will be the new solution for content generation in the metaverse.
First, AIGC provides the core infrastructure technology for building immersive metaverse environments, becoming a productivity tool for the metaverse. Secondly, during the development of the metaverse, games serve as important carriers of the virtual world, requiring a large amount of digital-native scenes. AIGC can significantly enhance the efficiency of developing digital-native content and reduce game development costs. Specifically, storylines, characters, avatars, props, scenes, voiceovers, actions, special effects, and main programs can all be generated through AIGC in the future. Moreover, AIGC can free developers’ productivity, aiding them in writing code so they can spend less time coding and more time expressing requirements, ultimately leading to a significant increase in productivity and the speed of building metaverse environments.
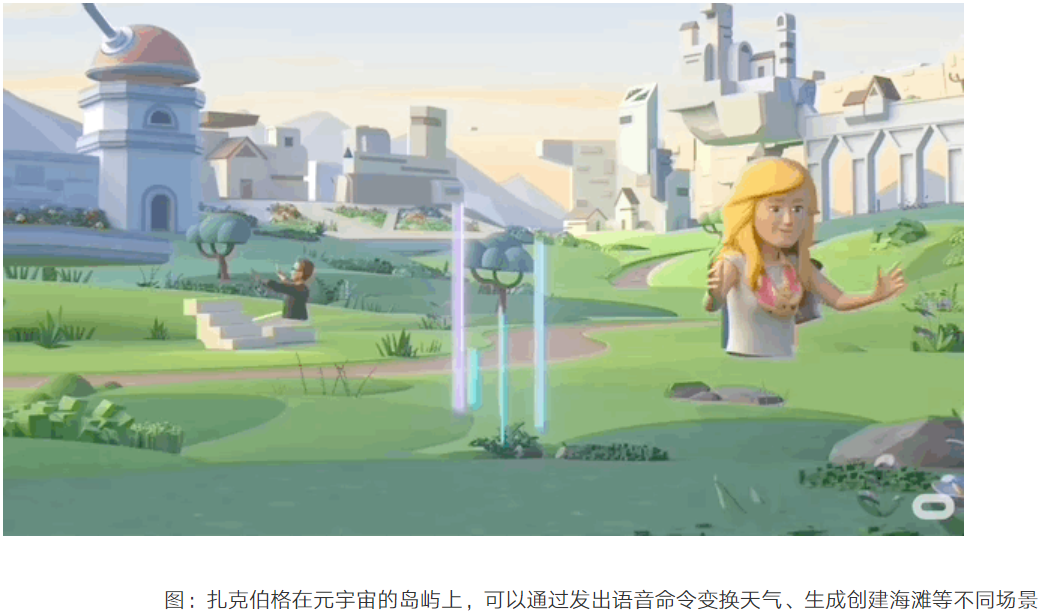
07
AIGC Risks
Intellectual Property Challenges
AIGC has matured in creating content works, but from the perspective of copyright law, AIGC essentially belongs to reorganizational innovation and does not possess true creativity. Currently, there is still a strong emphasis on human-AI collaboration, maximizing the advantages of both in content creation. However, the new copyright infringement risks posed by AIGC have become an urgent issue facing the development of the entire industry.
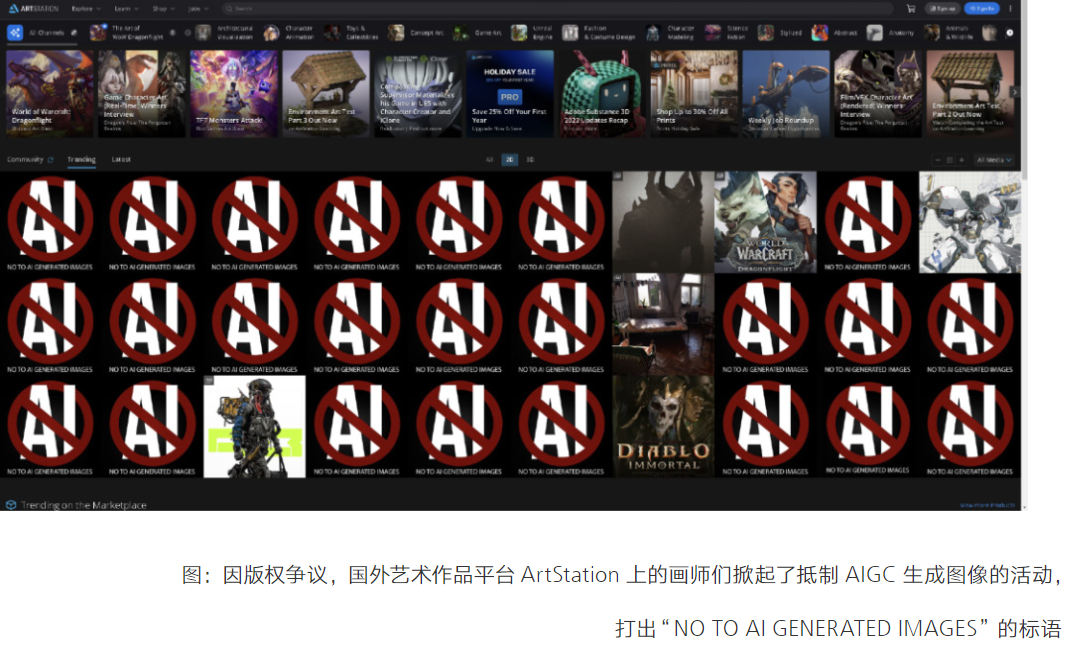
Security Challenges
Security issues are always unavoidable in the development and application of AI technology. Similarly, in AIGC, there are multiple security challenges, including content security, technology abuse, user privacy and identity, and inherent AI security.
For instance, the use of deepfake audio and video content, impersonating and stealing personal identities for fraud, extortion, and other illegal activities have already caused significant economic losses.
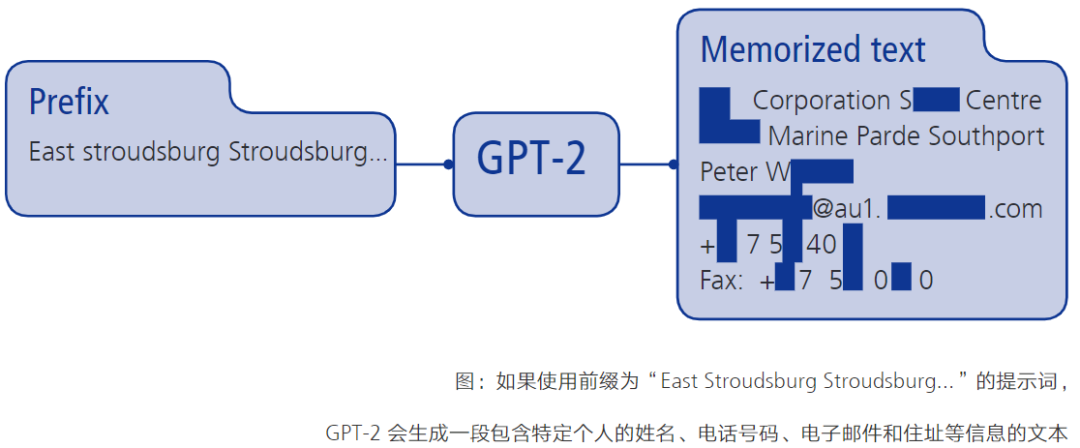
Discrimination Phenomena
Algorithmic discrimination has always been an ethical issue that is difficult to avoid in the innovation and application of artificial intelligence. AIGC based on pre-trained models has serious discrimination problems. For example, DALL-E2 exhibits more gender and racial biases, tending to generate human images of males and white individuals more frequently, and both models have a strong tendency to generate images labeled as male and Hispanic through CLIP.
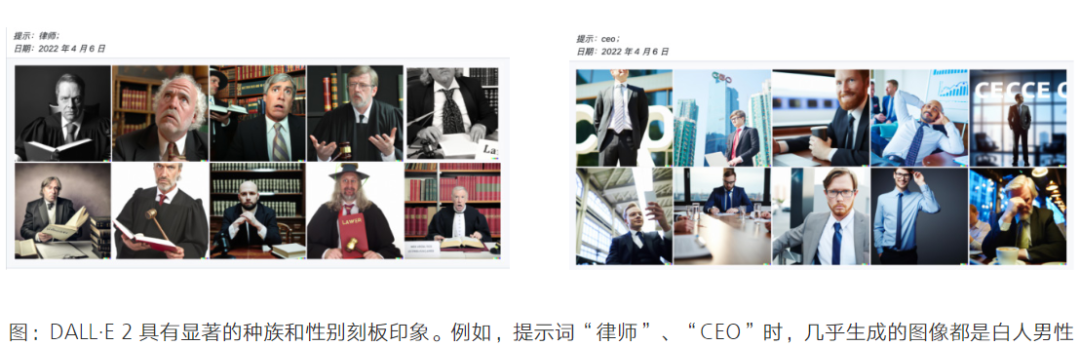
Environmental Challenges
AIGC based on pre-trained models requires a significant amount of computing power for both training and operation, inadvertently increasing energy consumption, and its rapid development poses a huge challenge to environmental protection and climate change, resulting in high carbon emissions. It has been estimated that the carbon dioxide emissions from training the GPT-3 model amount to 552 tons.
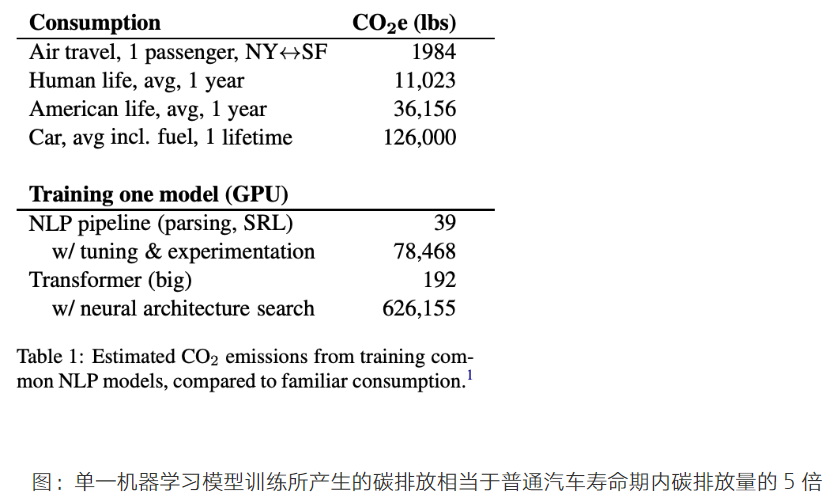
This article is sourced from the internet, copyright statement: published only by the “Huaxia Qihang Research Institute”.
Long press to follow the QR code to download resources for free.
Previous recommendations:
Invitation letter for the National University AIGC Teaching Innovation and Business Application Training Class.
Notice on the commencement of the “Virtual Reality Application Technology Talent” Advanced Training Course.