This project is the open knowledge base of the OpenRAG subproject under OpenKG, which focuses on RAG research and is committed to promoting the development of the RAG field.
You can access it through the link to enter the OpenRAG Base homepage.
Origin
2. The paper list on Github is lengthy and cannot quickly locate content.
4. It cannot provide targeted services and has poor interaction.
We hope to build a more flexible, intuitive knowledge base that can help users analyze and grasp the development of RAG, rather than simply piling up materials.
Therefore, we developed OpenRAG Base based on Notion.
What Is OpenRAG Base?
OpenRAG Base is a part of our entire OpenRAG open project (continuous updates will follow). This is the first comprehensive RAG knowledge base based on publicly available information collection and organization. Its purpose is to provide readers with cutting-edge RAG material summaries and multi-dimensional analyses, including:
-
Academic papers
-
Frontier consulting and articles
-
RAG evaluation and benchmarks
-
Downstream tasks and datasets
-
Tools and tech stacks
-
Summary of researchers and institutions
-
Topic reports
-
……More content will be launched soon (e.g., sample code, baseline testing)
Whether you are new to RAG or a researcher or practitioner related to RAG, you can benefit from it. Based on this repository, you have a flexible, multi-dimensional, dynamically updated Survey that supports highly customizable analysis and summaries.
How to Use?
The entire knowledge base consists of multiple Notion Databases. Click on a specific Database in the homepage Database List to view detailed content. More usage and customization methods can be referenced in the homepage introduction.
Note: When browsing online, you can only see static pages and cannot make modifications. Click “Duplicate” in the upper right corner of the homepage to copy it to your local before making changes.
Academic Papers
We have carefully designed more than ten attributes, including basic information such as authors, institutions, and publication conferences, as well as retrieval sources, retrieval processes, retrieval granularity, downstream tasks, datasets, evaluation metrics, and more to help analyze and summarize.
All Attribute View:
Quick overview view helps compare and analyze current RAG methods from the perspective of retrieval augmentation, including:Retrieval enhancement phase,data sources for retrieval,data types for retrieval,and retrieval processes.
What Downstream Tasks and Datasets Are There for RAG?
In addition, you can enter from the Downstream Task database to see which articles are under the same task. This will better help you build baselines under the same task.
Evaluation and Benchmark
The evaluation of RAG has always been a very important part. We will summarize the current evaluation tools and benchmarks.
Which Papers Have Open Sourced Code?
Check the “code” view to help you locate papers that have open-sourced code and quickly build a baseline.
Where Are RAG Papers Published?
How recognized are RAG papers by top conferences?This may be one of the issues that researchers care about. In the “Conf” view, we have grouped the publication addresses, and you can quickly see which recent top conferences have included RAG articles.
How to Quickly Read Papers?
With so many papers, how can you quickly understand the content? In Notion, each record can also be a page. Hover next to it and click “Open” to expand the details page on the right. This includes:
-
Abstract and author information from the original paper -
Important charts from the paper, such as flowcharts or model architecture diagrams -
Chinese quick read guide
Want to Know Who,Which Institutions Are Researching RAG?
The Scholar and Institution databases summarize the main researchers and their institutions in the RAG field based on papers and blogs. Red avatars represent academia, and green avatars represent industry. You can see that RAG is one of the popular fields of interest in both academia and industry.
View the RAG Scholar Gallery to see researchers, their institutions, and representative works.
Seminar Articles
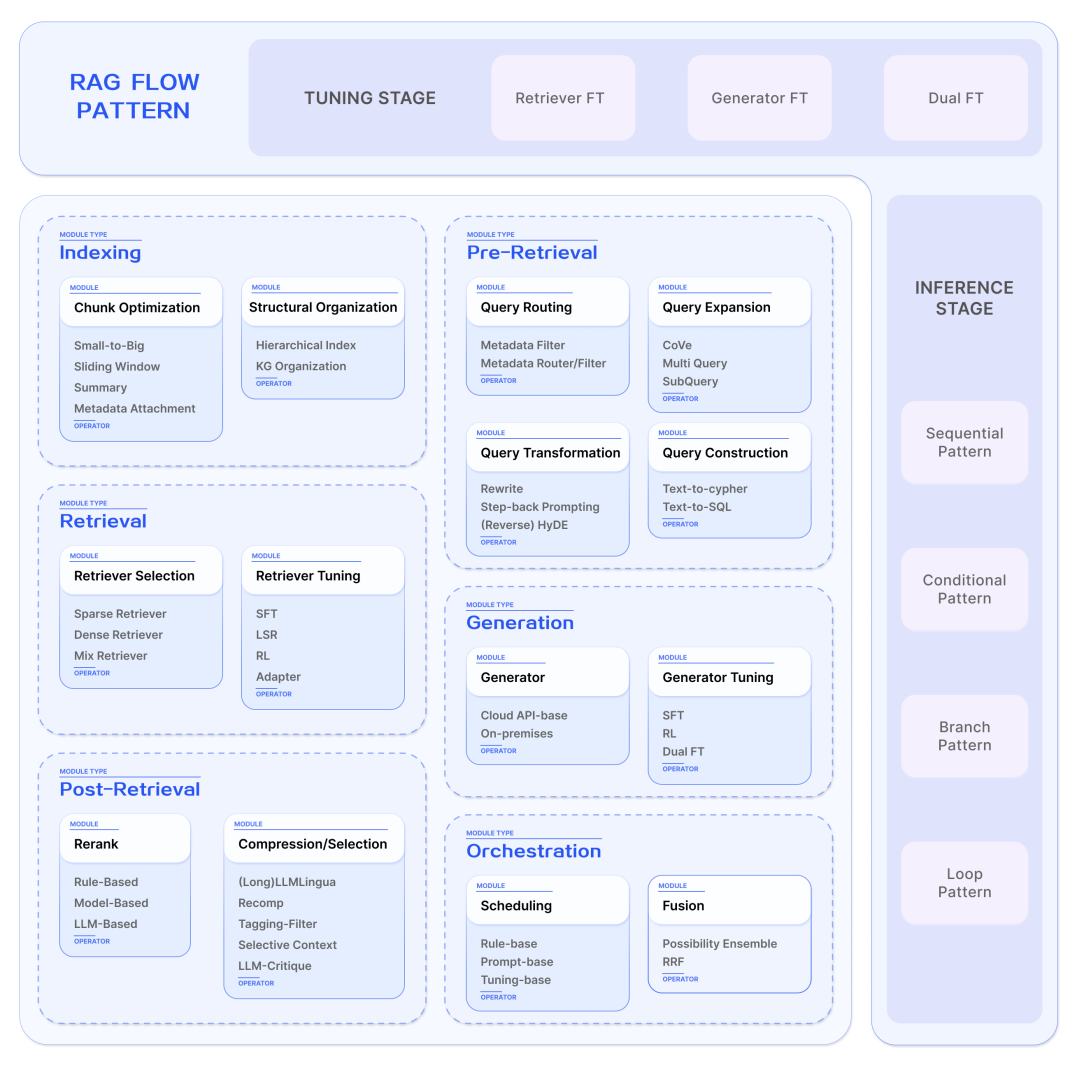
…More content awaits exploration
-
Comments
You can comment and engage in friendly exchanges anywhere you are interested, such as on Database or Pages detail pages. Please maintain good social etiquette and do not discuss content unrelated to RAG.
-
Copy
Anyone can copy the entire repo to local and make freer modifications based on it to build your own RAG knowledge base.
-
Contribute
Who Are We?
This project is jointly initiated by the Tongji University – KGLLM Laboratory (Prof. Wang Haofen and Prof. Wang Meng team), and Fudan University (Shanghai Key Laboratory of Data Science) led by Prof. Xiong Yun.
Contact Us
-
Gao Yunfan (Tongji University) Email: [email protected]
-
Wang Haofen (Tongji University) Email: [email protected] -
Wang Meng (Tongji University) Email: [email protected] -
Xiong Yun (Fudan University) Email: [email protected]
OpenKG
OpenKG (Open Knowledge Graph in Chinese) aims to promote the openness, interconnectivity, and crowdsourcing of knowledge graph data centered on Chinese, and to facilitate the open-source of knowledge graph algorithms, tools, and platforms.
Click to read the original article, and visit the OpenKG website.