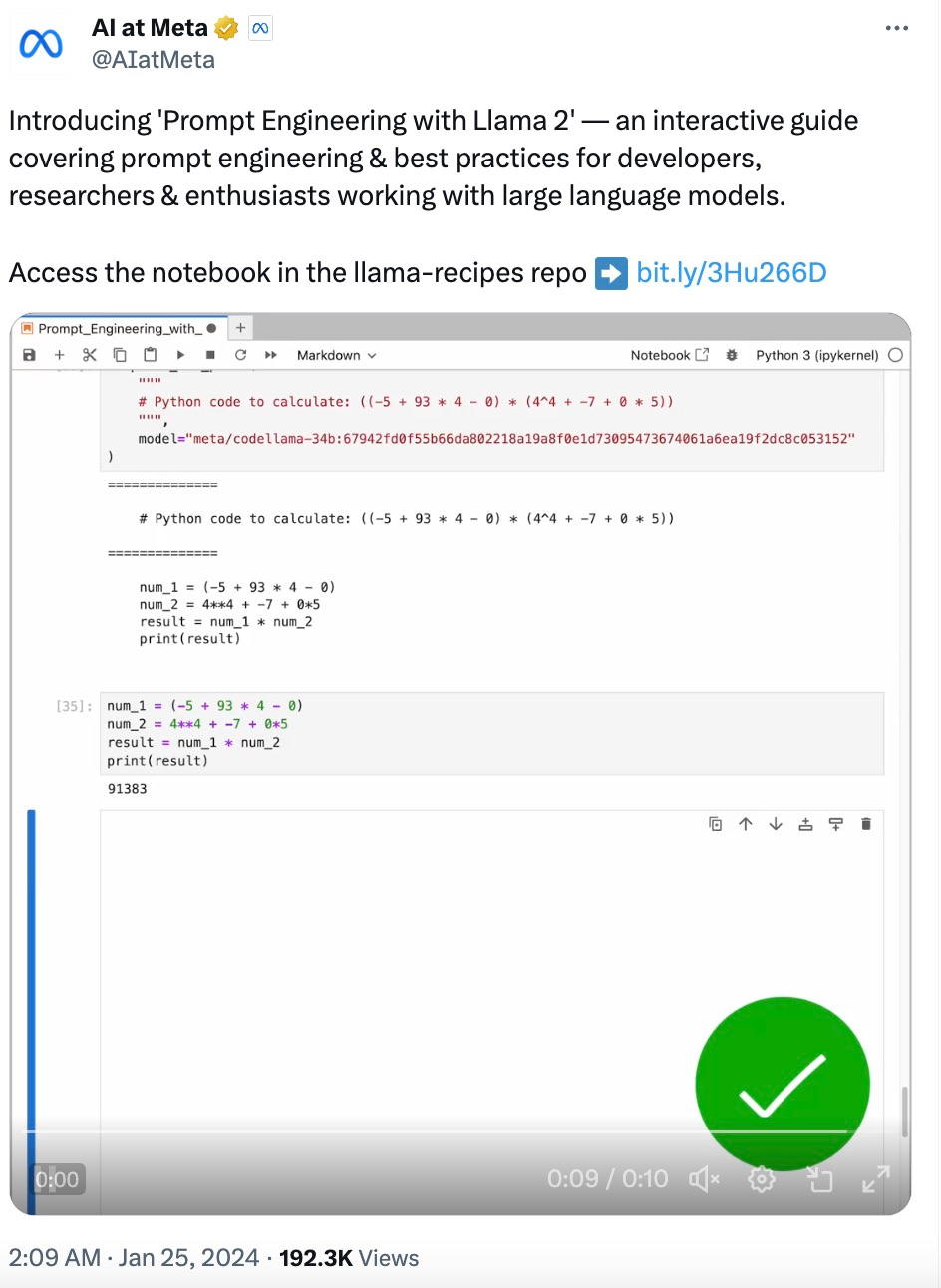
Llama Model
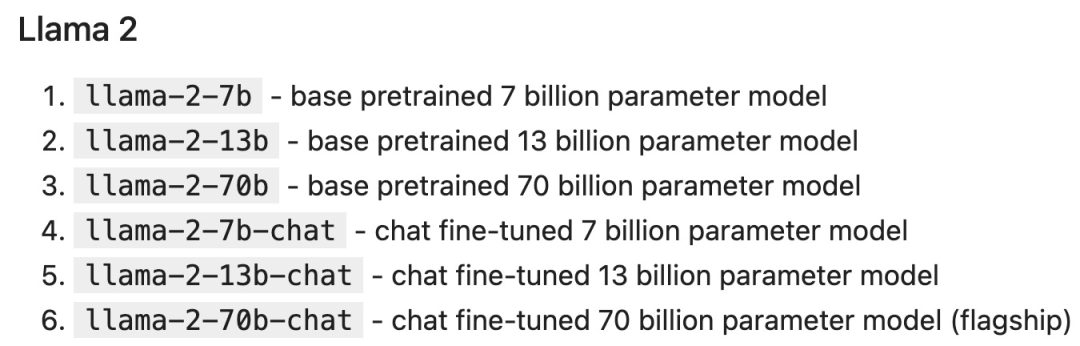
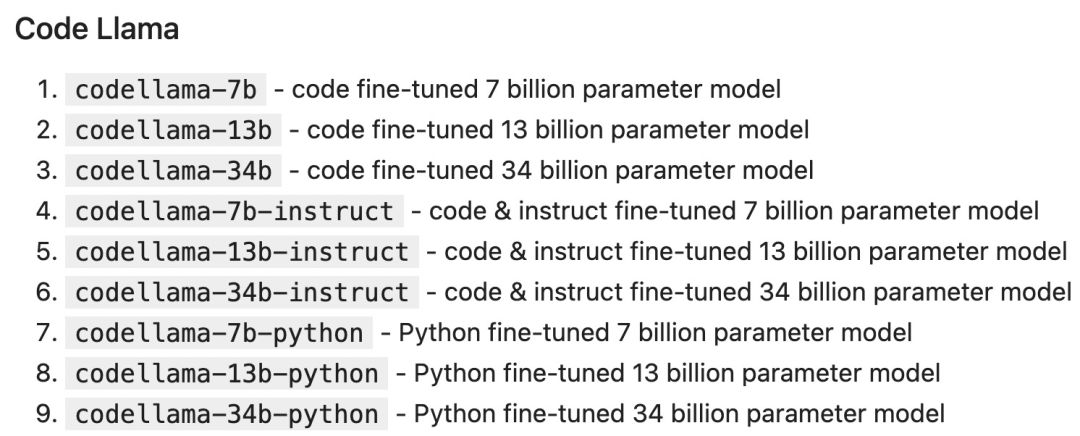
Deploying LLM
Our destiny is written in the stars.
Notebook Setup
pip install langchain replicate
from typing import Dict, List
from langchain.llms import Replicate
from langchain.memory import ChatMessageHistory
from langchain.schema.messages import get_buffer_string
import os
# Get a free API key from https://replicate.com/account/api-tokens
os.environ["REPLICATE_API_TOKEN"] = "YOUR_KEY_HERE"
LLAMA2_70B_CHAT = "meta/llama-2-70b-chat:2d19859030ff705a87c746f7e96eea03aefb71f166725aee39692f1476566d48"
LLAMA2_13B_CHAT = "meta/llama-2-13b-chat:f4e2de70d66816a838a89eeeb621910adffb0dd0baba3976c96980970978018d"
# We'll default to the smaller 13B model for speed; change to LLAMA2_70B_CHAT for more advanced (but slower) generations
DEFAULT_MODEL = LLAMA2_13B_CHAT
def completion(prompt: str, model: str = DEFAULT_MODEL, temperature: float = 0.6, top_p: float = 0.9) -> str:
llm = Replicate(model=model, model_kwargs={"temperature": temperature, "top_p": top_p, "max_new_tokens": 1000})
return llm(prompt)
def chat_completion(messages: List[Dict], model=DEFAULT_MODEL, temperature: float = 0.6, top_p: float = 0.9) -> str:
history = ChatMessageHistory()
for message in messages:
if message["role"] == "user":
history.add_user_message(message["content"])
elif message["role"] == "assistant":
history.add_ai_message(message["content"])
else:
raise Exception("Unknown role")
return completion(get_buffer_string(history.messages, human_prefix="USER", ai_prefix="ASSISTANT"), model, temperature, top_p)
def assistant(content: str):
return {"role": "assistant", "content": content}
def user(content: str):
return {"role": "user", "content": content}
def complete_and_print(prompt: str, model: str = DEFAULT_MODEL):
print(f'==============\n {prompt}\n==============')
response = completion(prompt, model)
print(response, end='\n\n')
complete_and_print("The typical color of the sky is:")
complete_and_print("which model version are you?")
response = chat_completion(messages=[user("My favorite color is blue."), assistant("That's great to hear!"), user("What is my favorite color?")])
print(response) # "Sure, I can help you with that! Your favorite color is blue."
def print_tuned_completion(temperature: float, top_p: float):
response = completion("Write a haiku about llamas", temperature=temperature, top_p=top_p)
print(f'[temperature: {temperature} | top_p: {top_p}]\n {response.strip()}\n')
print_tuned_completion(0.01, 0.01)
print_tuned_completion(0.01, 0.01) # These two generations are highly likely to be the same
print_tuned_completion(1.0, 1.0)
print_tuned_completion(1.0, 1.0) # These two generations are highly likely to be different
Prompt Techniques
complete_and_print(prompt="Describe quantum physics in one short sentence of no more than 12 words") # Returns a succinct explanation of quantum physics that mentions particles and states existing simultaneously.
-
Stylization, for example: -
Explain this to me as if teaching elementary school students on an educational children’s network; -
I am a software engineer using large language models for summarization. Summarize the following text in 250 words; -
Investigate the case step by step like a private detective, and provide your answer.
-
Formatting -
Use bullet points; -
Return in JSON object format; -
Use fewer technical terms and apply them in work communication.
-
Constraints -
Only use academic papers; -
Never provide sources older than 2020; -
If you don’t know the answer, say you don’t know.
complete_and_print("Explain the latest advances in large language models to me.") # More likely to cite sources from 2017
complete_and_print("Explain the latest advances in large language models to me. Always cite your sources. Never cite sources older than 2020.") # Gives more specific advances and only cites sources from 2020
complete_and_print("Text: This was the best movie I've ever seen! \n The sentiment of the text is:") # Returns positive sentiment
complete_and_print("Text: The director was trying too hard. \n The sentiment of the text is:") # Returns negative sentiment
def sentiment(text):
response = chat_completion(messages=[user("You are a sentiment classifier. For each message, give the percentage of positive/neutral/negative."),
user("I liked it"), assistant("70% positive 30% neutral 0% negative"),
user("It could be better"), assistant("0% positive 50% neutral 50% negative"),
user("It's fine"), assistant("25% positive 50% neutral 25% negative"),
user(text),])
return response
def print_sentiment(text):
print(f'INPUT: {text}')
print(sentiment(text))
print_sentiment("I thought it was okay") # More likely to return a balanced mix of positive, neutral, and negative
print_sentiment("I loved it!") # More likely to return 100% positive
print_sentiment("Terrible service 0/10") # More likely to return 100% negative
complete_and_print("Explain the pros and cons of using PyTorch.") # More likely to explain the pros and cons of PyTorch covering general areas like documentation, the PyTorch community, and mentions a steep learning curve
complete_and_print("Your role is a machine learning expert who gives highly technical advice to senior engineers who work with complicated datasets. Explain the pros and cons of using PyTorch.") # Often results in more technical benefits and drawbacks that provide more technical details on how model layers
complete_and_print("Who lived longer, Elvis Presley or Mozart?") # Often gives incorrect answer of "Mozart"
complete_and_print("Who lived longer, Elvis Presley or Mozart? Let's think through this carefully, step by step.") # Gives the correct answer "Elvis"
import re
from statistics import mode
def gen_answer():
response = completion("John found that the average of 15 numbers is 40.\nIf 10 is added to each number then the mean of the numbers is?\nReport the answer surrounded by three backticks, for example:```123```", model=LLAMA2_70B_CHAT)
match = re.search(r'```(\\d+)```', response)
if match is None:
return None
return match.group(1)
answers = [gen_answer() for i in range(5)]
print(f"Answers: {answers}\n",f"Final answer: {mode(answers)}") # Sample runs of Llama-2-70B (all correct): # [50, 50, 750, 50, 50] -> 50 # [130, 10, 750, 50, 50] -> 50 # [50, None, 10, 50, 50] -> 50
complete_and_print("What is the capital of California?", model=LLAMA2_70B_CHAT) # Gives the correct answer "Sacramento"
complete_and_print("What was the temperature in Menlo Park on December 12th, 2023?") # "I'm just an AI, I don't have access to real-time weather data or historical weather records."
complete_and_print("What time is my dinner reservation on Saturday and what should I wear?") # "I'm not able to access your personal information [..] I can provide some general guidance"
MENLO_PARK_TEMPS = {"2023-12-11": "52 degrees Fahrenheit", "2023-12-12": "51 degrees Fahrenheit", "2023-12-13": "51 degrees Fahrenheit"}
def prompt_with_rag(retrieved_info, question):
complete_and_print(f"Given the following information: '{retrieved_info}', respond to: '{question}'")
def ask_for_temperature(day):
temp_on_day = MENLO_PARK_TEMPS.get(day) or "unknown temperature"
prompt_with_rag(f"The temperature in Menlo Park was {temp_on_day} on {day}", # Retrieved fact
f"What is the temperature in Menlo Park on {day}?") # User question
ask_for_temperature("2023-12-12") # "Sure! The temperature in Menlo Park on 2023-12-12 was 51 degrees Fahrenheit."
ask_for_temperature("2023-07-18") # "I'm not able to provide the temperature in Menlo Park on 2023-07-18 as the information provided states that the temperature was unknown."
Program-Aided Language Models
complete_and_print("Calculate the answer to the following math problem:((-5 + 93 * 4 - 0) * (4^4 + -7 + 0 * 5))") # Gives incorrect answers like 92448, 92648, 95463
complete_and_print("""
# Python code to calculate: ((-5 + 93 * 4 - 0) * (4^4 + -7 + 0 * 5))
""", model="meta/codellama-34b:67942fd0f55b66da802218a19a8f0e1d73095473674061a6ea19f2dc8c053152")
# The following code was generated by Code Llama 34B:
num1 = (-5 + 93 * 4 - 0)
num2 = (4**4 + -7 + 0 * 5)
answer = num1 * num2
print(answer)
Scan the QR code to add the assistant WeChat