Unicorn Think Tank: The Leading Industry Research Think Tank
Recruitment for Unicorn Investment Research Intelligence Group
Unicorn Think Tank has developed over 9 years, accumulating a wealth of resources and forming a community of shared interests with top investment research resources. After nearly a year of product testing and small-scale member services for almost two years, we have accurately grasped opportunities in sectors like new productive forces, AI, and Huawei, including companies like Bojie Co., Zhangjiang Hi-Tech, Huali Chuantong, People’s Daily, Inspur, and Daily Interaction.
We are now opening up experience slots. Add WeChat : itouzi6, with a QR code at the end of this article.
1. Overview
-
The Impact of Sora on AI Computing Infrastructure
With the rapid development of the AI industry, models like Sora and Google’s GPT overseas, as well as the rise of numerous domestic AI models, indicate that the artificial intelligence industry is advancing vigorously. Boyun Company has demonstrated strong capabilities in AI infrastructure, building a high-performance computing foundation around cloud computing business, and providing a complete set of solutions covering GPU resource utilization and scheduling. Currently, the global demand for computing power is surging, with text models having saturated last year’s computing power market, and with the popularization of applications like video processing this year, demand for computing power is expected to further increase.
-
The Evolution of Sora and AI Computing Infrastructure
The market’s demand for AI computing power continues to rise, impacting not only hardware manufacturers but also driving the overall evolution of infrastructure AI research software suppliers, model tuning service providers, and AI application development industries. The Pangu large model has achieved significant efficiency improvements in fields like meteorology; for instance, the time to predict weather using handwritten formula algorithms has been reduced from five days to one day for a seven-day forecast, fully reflecting the overall progress in AI algorithm efficiency. However, while large models are efficient, they face issues such as poor interpretability and lack of transparency in results, often requiring more computing power or data support to improve accuracy, leading to increased costs.
-
Analysis of Sora’s Impact on the AI Computing Ecosystem
The performance of Huawei’s Ascend 910B chip is close to model 100, but due to its lack of support for double-precision calculations, it requires conversion operations in large model application scenarios, adding complexity. Huawei faces challenges in production capacity and relatively weak software compatibility in computing products, leading to large investments and lengthy cycles in software development. Coupled with a relatively closed ecosystem, this raises concerns for some customers when adopting Huawei products.
In contrast, domestic company Inspur’s products perform excellently in terms of compatibility, matching international products in performance with significant cost-effectiveness advantages, and possess a more open ecosystem, gaining widespread recognition in the industry.
-
Sora’s Technological Changes in the AI Computing Landscape
Although the Sora series chips perform averagely in large model training, if the new series achieves good results in large model training tests, sales are expected to rise rapidly. Heterogeneous computing environments are now commonly found in government-led computing centers, with domestic chips facing challenges in API compatibility and computing platform support. The difficulty of heterogeneous computing in the training phase is considerable, typically preferring to operate within clusters of the same model GPU, while issues in the pre-inference phase are relatively easier to solve, thanks to the optimization functions of compilers.
-
Future Opportunities and Challenges for Sora Intelligent Computing
New opportunities and challenges in AI infrastructure lie in data processing, model algorithm development, training, and inference stages, where the training phase can significantly enhance model performance. Industry scenario implementation is an important trend for future development. For model development and training platforms, small model customers prefer privatized development, whereas large model customers mostly adopt open-source models. Data privacy issues limit the widespread application of public clouds in sensitive areas like finance and healthcare. The demand for privatized deployment is gradually rising, with customers needing cost-effective training platforms adaptable to various hardware and flexible inference environments. Vendor service models are also shifting towards model subscription + service to meet the needs for computing power and platforms.
-
Analysis of Sora’s Ecosystem and Its Impact in the AI Field
The usability and customer feedback of Huawei’s Kunpeng ecosystem is generally positive, and this ecosystem has been solidified and rapidly developed. Huawei’s Euler operating system, as an important Linux kernel version in the industry, is expected to further promote ecosystem openness. In contrast, the Ascend ecosystem is not as open as Kunpeng, with limited model support, possibly due to technical difficulties and the need to maintain Huawei’s own model competitiveness.
7. Discussion on Investment and Operational Strategies
Capital investment and construction: The main entities constructing domestic computing centers are government-led, with large scale and computing power reaching over 500P, with thousands of cards. The government invests heavily, focusing on large-scale heavy asset investments.
Operational models and challenges: Operations mainly adopt an external leasing model, and maximizing the effectiveness of computing centers becomes a key challenge. Currently, operational systems are relatively rudimentary and urgently need to establish comprehensive resource scheduling, billing, and other management systems.
Current situation of computing chip networking: China has not yet formed a unified leading unit and standards for computing chip networking, mostly following NVIDIA standards, while the operating entities are not clearly defined, with “who builds, who operates” being the norm.
8. Reassessing the Influence of Sora Chips
Analysis of the latest chip models from Inspur shows that the K series is expected to match the performance of A100, but due to the lack of third-party evaluation reports,
specific performance remains to be confirmed; the L series is benchmarked against A40 and theoretically performs on par with 9101, but the K series shows a significant performance improvement over the L series. The advantage of Inspur products lies in supporting double-precision calculations, lowering the technical threshold for model developers and promising better usage results.
Q: What impact has Sora had on AI computing power?
A: From a market perspective, the demand for computing power continues to grow, and this demand is affecting the entire foundational AI research field, from hardware manufacturers to foundational AI model developers, model tuning service providers, AI application developers, and ultimately the various industries using these models. The entire ecosystem is rapidly evolving. Previously, work in the industry primarily involved data processing based on handwritten algorithms, but now, the application of large AI models has significantly improved efficiency. Huawei’s Pangu large model is an example; once adopted in the meteorology field, it has improved prediction efficiency by about seven times, reducing the time to complete a seven-day forecast from five days to one day. This leap in efficiency has led many industries to begin adopting AI models to promote development. Although large AI models have higher computational efficiency, they often exist as “black boxes,” lacking transparency and interpretability. To improve model accuracy, it often requires increasing investment in computing power or data, both of which are relatively costly. However, their speed and optimization capabilities are indeed significant.
Q: How do Huawei’s AI chips perform in terms of performance and cost compared to NVIDIA?
A: Based on current market acceptance and actual data, how Huawei’s AI chips perform is a key consideration for our cooperation and engagement. To compare the performance and overall costs of these chips, we need to examine their performance at customer sites and the levels they can achieve, but currently, more specific data is needed to assess Huawei’s AI chips’ competitiveness compared to NVIDIA and other competitors.
Q: What positive impacts has Sora had on AI computing power? In which specific areas?
A: Customers are generally satisfied with the test results of the Ascend 910B model, which performs comparably to NVIDIA’s V100, far exceeding previous expectations for domestic chips. The 910B performs well under different precision calculations, but it has issues with double-precision support, requiring conversion in large model computing scenarios. The main problem facing Huawei’s ecosystem is tight production capacity, as the use of 7nm technology and smartphone chips share production capacity, leading to delivery cycles generally exceeding three months. Moreover, weak compatibility necessitates developers to rewrite and recompile code to adapt to Huawei’s framework. The best solution when using Huawei cards is to use Huawei’s own Kunpeng GPU and Euler operating system, along with Huawei’s Pangu and Xinghuo models, although this results in a relatively closed system.
Q: How about other domestic chip manufacturers besides Huawei?
A: Inspur’s products have good compatibility domestically, and its API is compatible with NVIDIA’s ID. The new K series products from Inspur perform comparably to V100 and offer high cost-performance ratios. Other manufacturers like Haiguang, Tianzhuxin, and Cambrian have lower production capacities than Inspur, and their performance and compatibility are also lacking. If Moore County is not affected by sanctions, its products are very close to NVIDIA, but currently, there are capacity issues. Cambrian is more suitable for inference phases, with significant gaps in its training scene algorithm library and expanding ecosystem compatibility.
Q: What is the current demand situation for domestic chip manufacturers in the market?
A: Due to price increases and service quality issues caused by sanctions, customers are beginning to consider domestic chips on a large scale, especially since the second half of last year. Huawei’s 910D has already seen large-scale procurement, while Inspur and other manufacturers have not yet delivered in bulk. Confirmed bulk shipments are mainly occurring at companies like iFlytek and Telecom.
Q: How do you evaluate the current domestic chip manufacturers in terms of performance, cost, and migration difficulty?
A: In terms of performance and cost, Huawei’s 910B price has rapidly increased, currently selling for nearly 2 million yuan, while Inspur’s prices are relatively lower and considered high in cost-performance in the industry. Regarding migration difficulty, both Huawei and Inspur’s ecosystems are relatively open, with good compatibility, aiding large model compatibility, while other manufacturers are relatively weaker in this regard. Overall, most ecosystems and large model compatibilities are still in the research phase, with actual applications mainly concentrated in the inference part, and training being used less.
Q: How does Sora perform in AI computing power? What are its performances in large model training?
A: In terms of AI computing power, Huawei and Inspur’s products perform outstandingly in the inference domain, especially showing significant effects in small model training. For large model training, these devices may be somewhat lacking. Currently, the industry holds a wait-and-see attitude towards the performance of Inspur’s new series products, mainly looking forward to their performance in large model training. If the new series can perform well in large model training, its market acceptance is expected to rise quickly. The performance of domestic chips in large model training has not yet met expectations, mainly because API compatibility issues and support for computing scheduling platforms need to be resolved. Regarding the Yigou computing center, domestic cards are mainly used for inference tasks, as this phase is relatively easier to resolve, while challenges in the training phase are greater.
Q: What are the commonalities and challenges of mixed deployment of multi-brand GPUs suitable for various computing tasks?
A: In government-led computing centers, mixed deployment of GPUs regardless of brand has become quite common, especially in intelligent computing centers led by or referencing Huawei’s model. The challenges of mixed models mainly lie in the technical aspect, needing to overcome API compatibility issues and computing base platform support. From the application perspective, model development and training require software manufacturers to adjust API compatibility; some universities and research institutions are working on this and collaborating with manufacturers like Huawei. In practical applications, single model training tends to run on the same brand and type of GPU, mainly for computational synchronization and resource efficiency considerations. Nevertheless, models of different parameter scales can be deployed on different brand GPU clusters. In the inference phase, because of compiler involvement, and the model has been trained, issues at this stage are relatively easier to resolve. In computing centers, the Yigou computing center model has become very common.
Q: What problems exist in model training in heterogeneous computing environments under the current technical environment, and what measures are in place to address these issues?
A: Currently, model training in heterogeneous computing environments still faces challenges, primarily because heterogeneity may lead to desynchronization during the computational process, affecting task efficiency and final results. Existing solutions include fine-tuning tasks, but still require computation on similar models and types of GPUs to ensure computational synchronization and the uniformity of hardware resources like network bandwidth and memory. Although some tasks can run on different types of GPUs, this practice is not common. Further compatibility solutions include optimizing compilers to ensure operational compatibility across different GPUs at the compilation level. Currently, there is still a lack of particularly effective measures regarding these issues.
Q: What new opportunities and challenges exist at the infrastructure level in the context of AI?
A: In AI scenarios, the entire process includes data processing, model algorithm development, training, and post-training inference. Currently, the training phase has received considerable attention, as it best reflects the effects. When models perform well, whether in video output or conversational services, they can greatly impact users. Subsequently, industry scenario implementation is necessary. A few focus on model development, while platforms like MOOS support small models extensively, large models tend to adopt open-source models and expect to develop independently to prevent data leakage. Customers are now inclined towards privatized deployment, needing cost-effective lightweight training platforms. Additionally, there is significant demand for self-built platforms, but cost considerations lead them to prefer lightweight solutions. Neutral manufacturers are more favored as they can maintain model compatibility.
Q: What specific impacts does Sora have on AI infrastructure and computing platforms?
A: Sora provides a lightweight training platform and GPU resource pooling and scheduling management. In this regard, customers have concerns when selecting large factory models, model manufacturers, or GPU manufacturers, as once they choose a particular product or service, they may be limited by its ecosystem, while neutral manufacturers can offer better compatibility. In terms of computing bases, support for various inference cards is needed, for example, we have already compatible with multiple KD cards. Although current focus on the inference phase is insufficient, the flexibility of computing usage here and stability has become an important consideration. Sora is also focusing on the stability of inference and is committed to improving service efficiency and performance. Similar to mid-platform services in cloud computing, we are building engineering support for AI inference applications to meet various needs from development to scenario implementation. In summary, the core influence of Sora’s AI infrastructure and computing platform lies in providing lightweight, highly compatible solutions for training and inference, with optimizations and innovations in stability and efficiency.
Q: What level has the Kunpeng ecosystem developed to? Mainly from usability and customer feedback perspectives.
A: The Kunpeng ecosystem has evolved from an early stage of low trust to a significantly large domestic shipment volume currently, with a notable development speed. Currently, in China, besides Intel being mainstream, Kunpeng has become a major alternative ecosystem. Huawei’s Kunpeng mainly builds its ecosystem around its own Euler operating system. Huawei’s strategy is to open the Kunpeng ecosystem as much as possible, and there are rumors that domestic Linux operating systems will be replaced by Euler, making it appear more open. Regarding software ecosystem, Huawei emphasizes that its software performs 10% to 20% better on Kunpeng hardware than on Intel.
Q: How is the development rate of the Ascend ecosystem? Will it surpass Kunpeng in the future?
A: Currently, the Ascend’s degree of openness is not as high as Kunpeng, and it is relatively bound to its own Kunpeng CPU during operation. In terms of upper-layer model support, Ascend mainly relies on officially announced support for iFlytek’s Xinghuo and Huawei’s own models, while support for other models is relatively small and scarce. This may be due to the competition of Huawei’s full set of models with other models, while third-party model manufacturers believe that the workload of API compatibility is too large and are not very active under the current high demand.
Q: The limited model support of Ascend is due to Huawei’s willingness or because other models are difficult to run on it?
A: This issue is influenced by two factors. First, Huawei’s own Pangu model competes with other models, and Huawei may not be particularly inclined to support other models. On the other hand, other manufacturers may find that the investment in API compatibility work is too large, and in the face of high demand, they are not very proactive.
Q: What is the current status of construction and operation of domestic computing centers?
A: Currently, the main entities constructing domestic computing centers are government-led. The investment scale is usually large, with computing power reaching 500P or more, equipped with thousands of cards, mainly through government investment. The government invests at various levels, including city and provincial levels. The operational model after construction mainly adopts external leasing. The sales and operations of computing centers face many challenges, such as sales difficulties, resource scheduling, account management, etc. Currently, under seller’s market conditions, many buyers actively seek computing resources, and some computing centers have even sold resources without establishing basic operational systems. Besides government-led situations, enterprises also build their own computing centers, especially state-owned enterprises, central enterprises, and financial enterprises, but their scales are usually smaller and their operational management is more refined. Moreover, under changing market conditions, these computing centers will also build necessary operational management systems.
Q: Is there a leading unit responsible for the networking of domestic 3D chips? At the same time, how is the API compatibility at the chip level?
A: For the networking of 3D chips, the Ministry of Industry and Information Technology and the National Information Center have led the national computing power scheduling network planning, hoping to utilize idle computing power, but the operation subject of this system has not yet been clearly defined. Operators and local governments are competing for operating rights, but local governments often prefer to control it themselves, leading to the current operational subjects still being dominated by builders. In terms of chip compatibility, there has long been a lack of unified standards in China, and manufacturers usually follow their own standards. However, the industry generally accepts NVIDIA’s API, as its operator library has excellent performance and high compatibility, so even other manufacturers’ hardware tries to maintain compatibility with NVIDIA’s API.
Q: Can several important products in the computing chip market, such as Cambrian 590 and Haiguang Deep Learning No. 3, compete with Huawei’s 910D chip?
A: I am not very familiar with Cambrian’s 590 model, and based on past experience, it may be quite difficult to defeat Huawei’s 910D model. As for the Haiguang Deep Learning No. 3 chip you mentioned, I am not clear about which model it is. If you can provide more detailed information, I may be able to give a more specific evaluation.
Q: How are the latest products and their performance in the AI computing field from Shenzhen No. 3?
A: The latest products from Shenzhen No. 3 should belong to the K series. According to Inspur’s introduction, this series can achieve performance comparable to NMIDAA100. However, since there have not yet been customer evaluation reports, its performance cannot be fully confirmed. Compared to the previous L series, the K series has certainly seen a significant performance improvement. The previous version is benchmarked against NVIDIA A40, and if it can achieve the claimed performance level, it should also be comparable. Inspur’s products support double-precision calculations, which is an important difference from 910B, as 910B does not support double-precision. Therefore, models based on double-precision calculations can run directly without needing model compression to support single-precision operations, which may have advantages in operational efficiency and model development difficulty.
Experience slots for investment research intelligence group are now open. For more investment research intelligence services, please see below.
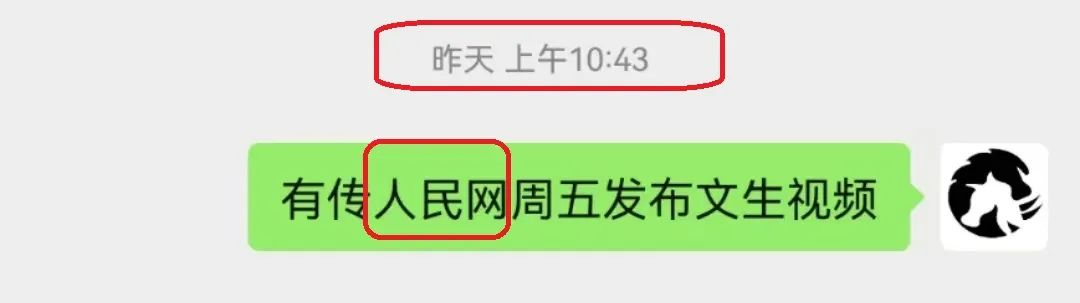
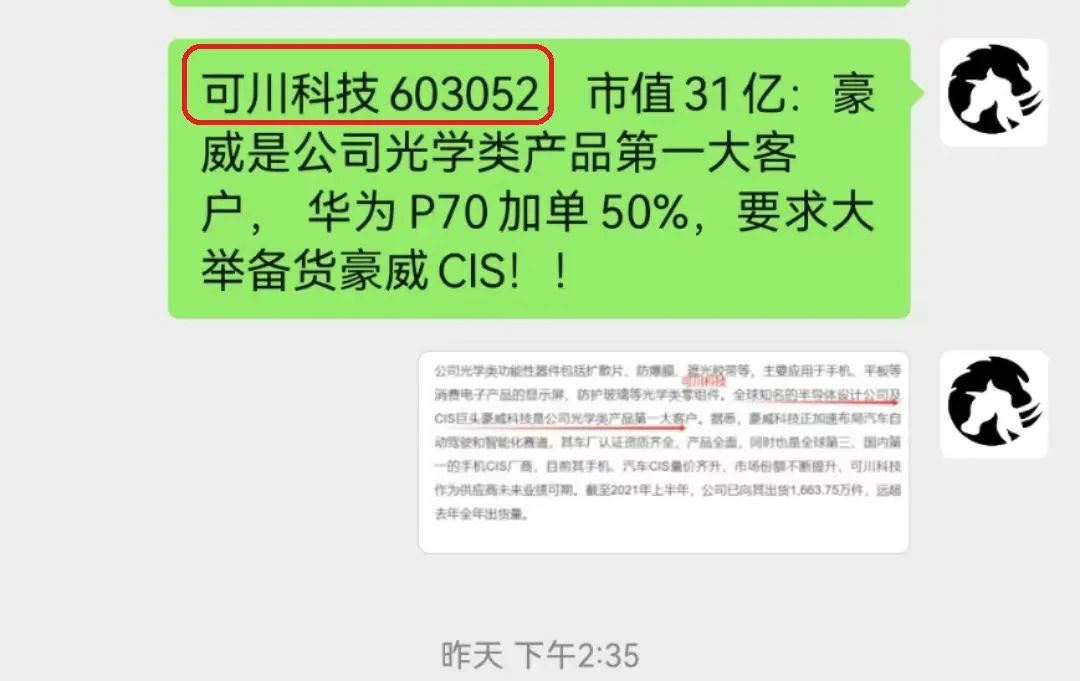
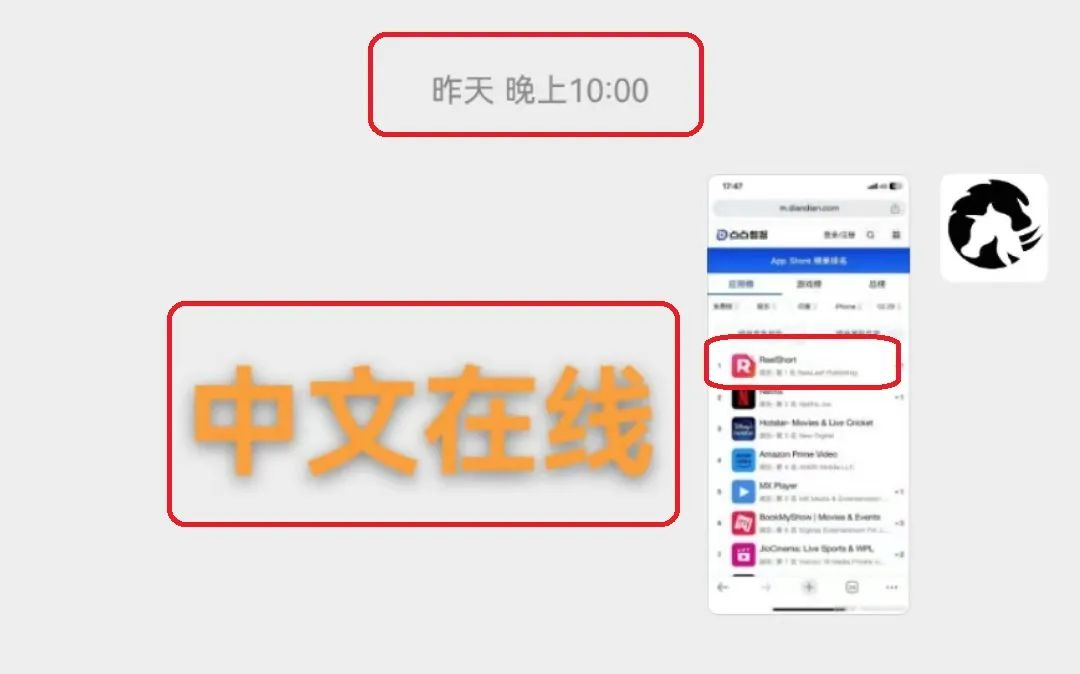
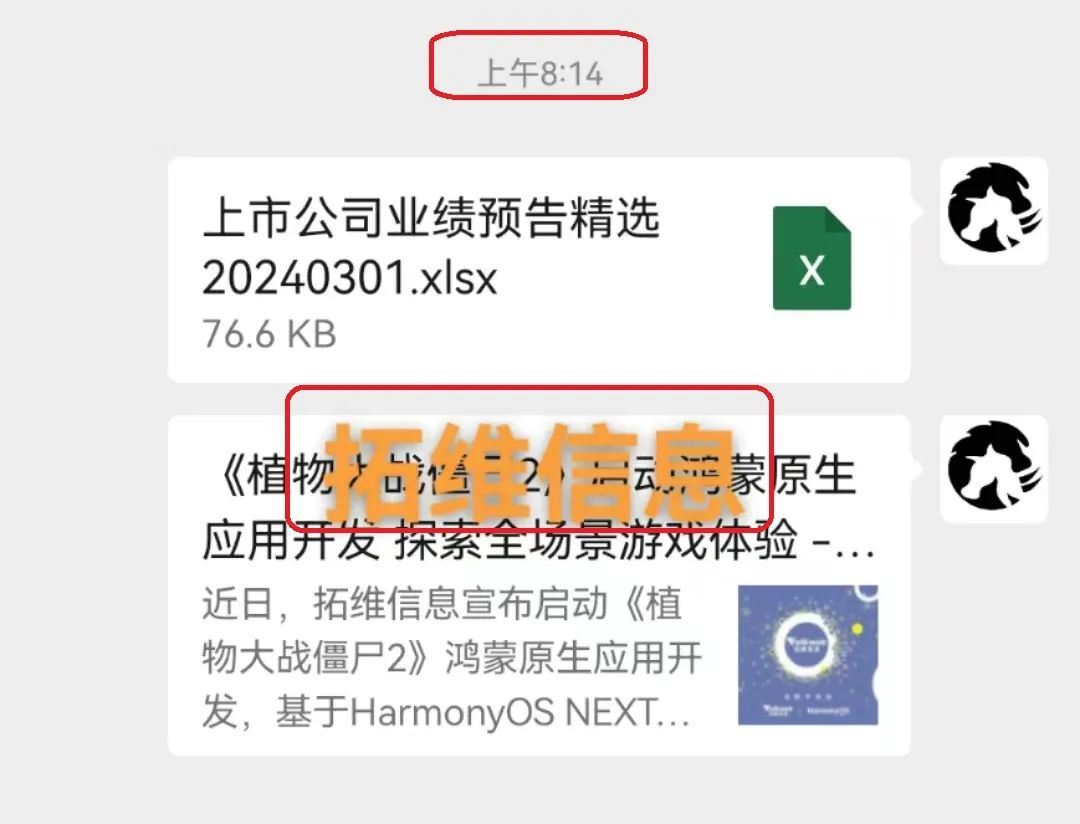
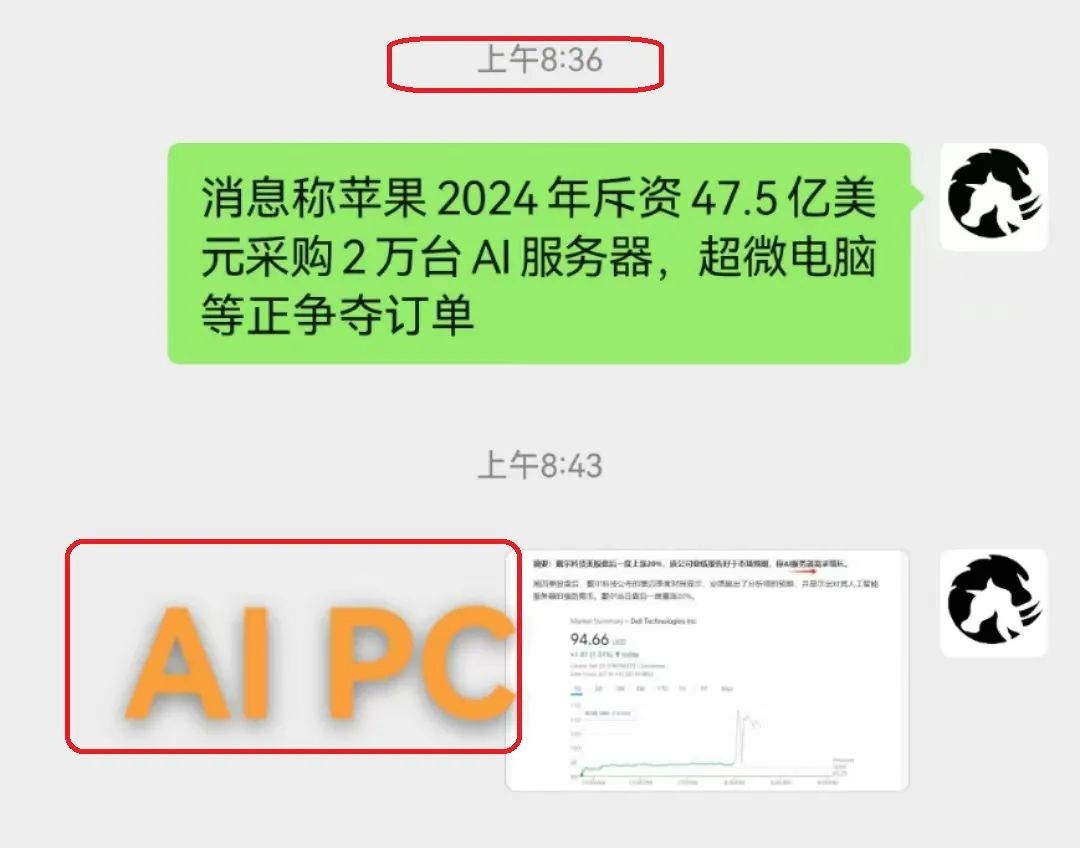
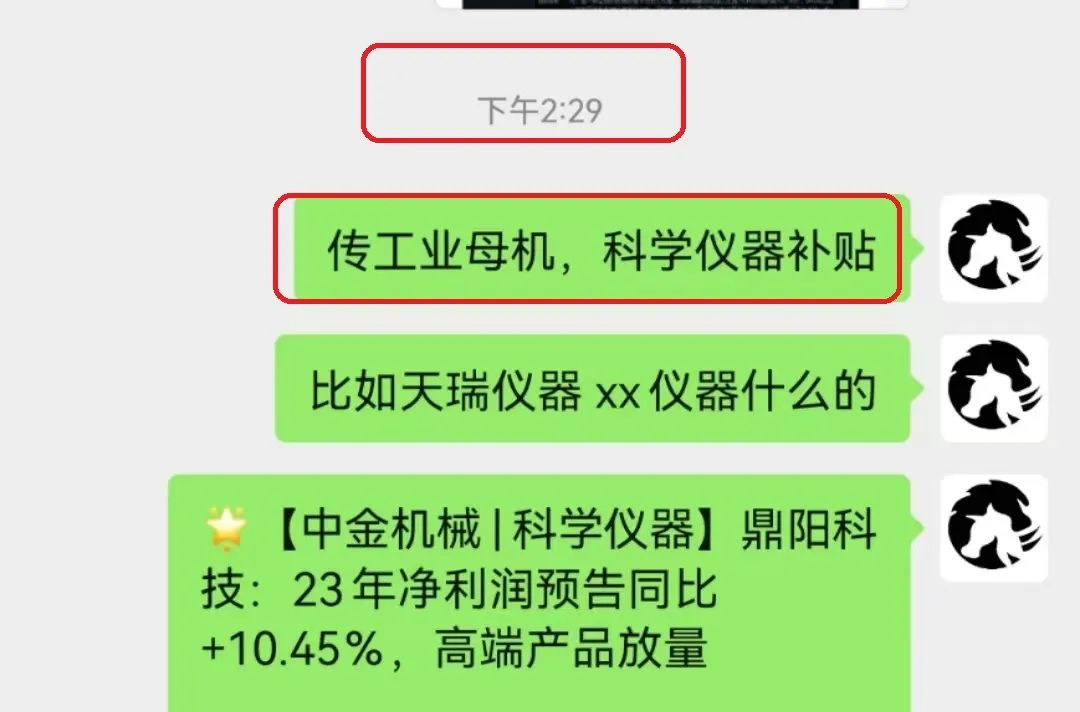
Unicorn Investment Research Intelligence Membership Service
Service Overview
In today’s A stock market, style switches rapidly, whether it is about track growth, wind direction segments, value investment, leading stocks, or technical short-term trading, most of the time results in losses. The only thing that continues to hold value is information that is a step ahead. This information will not be the news from financial media, nor the research summaries from knowledge platforms, nor the logic of ticket-pushing from communities.
Service Purpose
Provide various investment research information that is a step ahead, allowing you to clearly understand market movements.
Intelligence Sources
The Unicorn Think Tank’s investment research intelligence team has deeply rooted itself in various ecological levels of the big A:
1: Core circle of public offerings, obtaining the general direction and main attack areas favored by public offerings in advance.
2: Circle of brokerage analysts, delving into the core customer groups of major brokerages to obtain the main推 logic in advance.
3: Core circle of speculative funds, having a place in the small circle of speculative fund bigwigs, obtaining large fund movements in advance.
4: Industrial chain circle, digging into the core circles of new emerging industries’ technologies in advance to uncover the A stock speculation logic driven by technological changes.
Service Content
1, Movements of large funds.
2, Early knowledge of leading stocks in collective bidding.
3, Early small essays.
4, Main推 directions and logic of brokerages.
5, Market opportunities and directional prompts.
6, Avoiding risks in individual stocks and industries.
Service Method:
WeChat group — only WeChat group messages can achieve first-time information delivery.
Experience slots now open (not free, please do not disturb)
Joining experience method (if you are interested in short-term trading)
Please add WeChat: itouzi6, note: Experience + Name + Company + Position
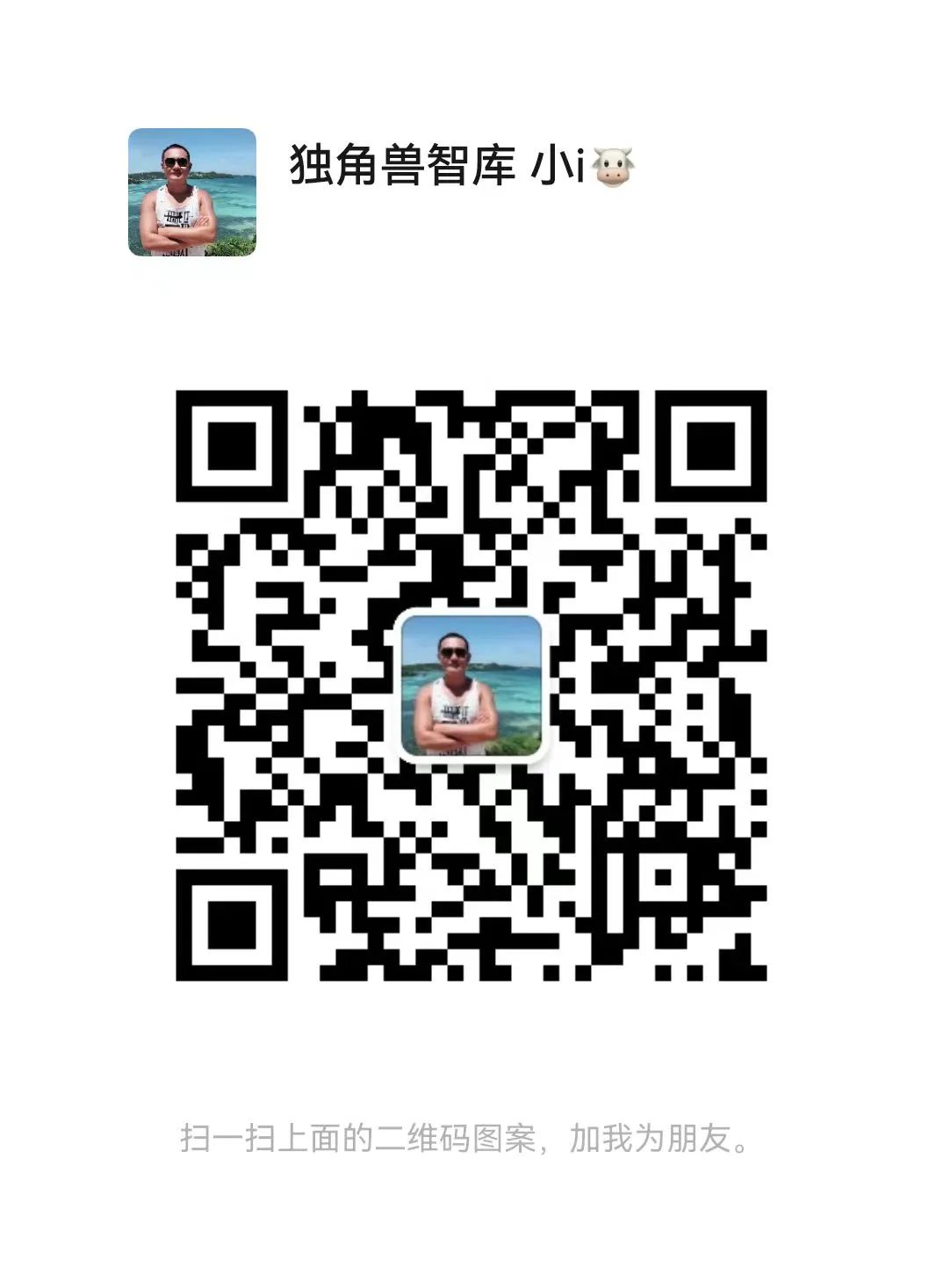
If you are interested in fundamentals, doing segments, or value investing
Please add WeChat:itouzi5, note:Experience + Name + Company + Position
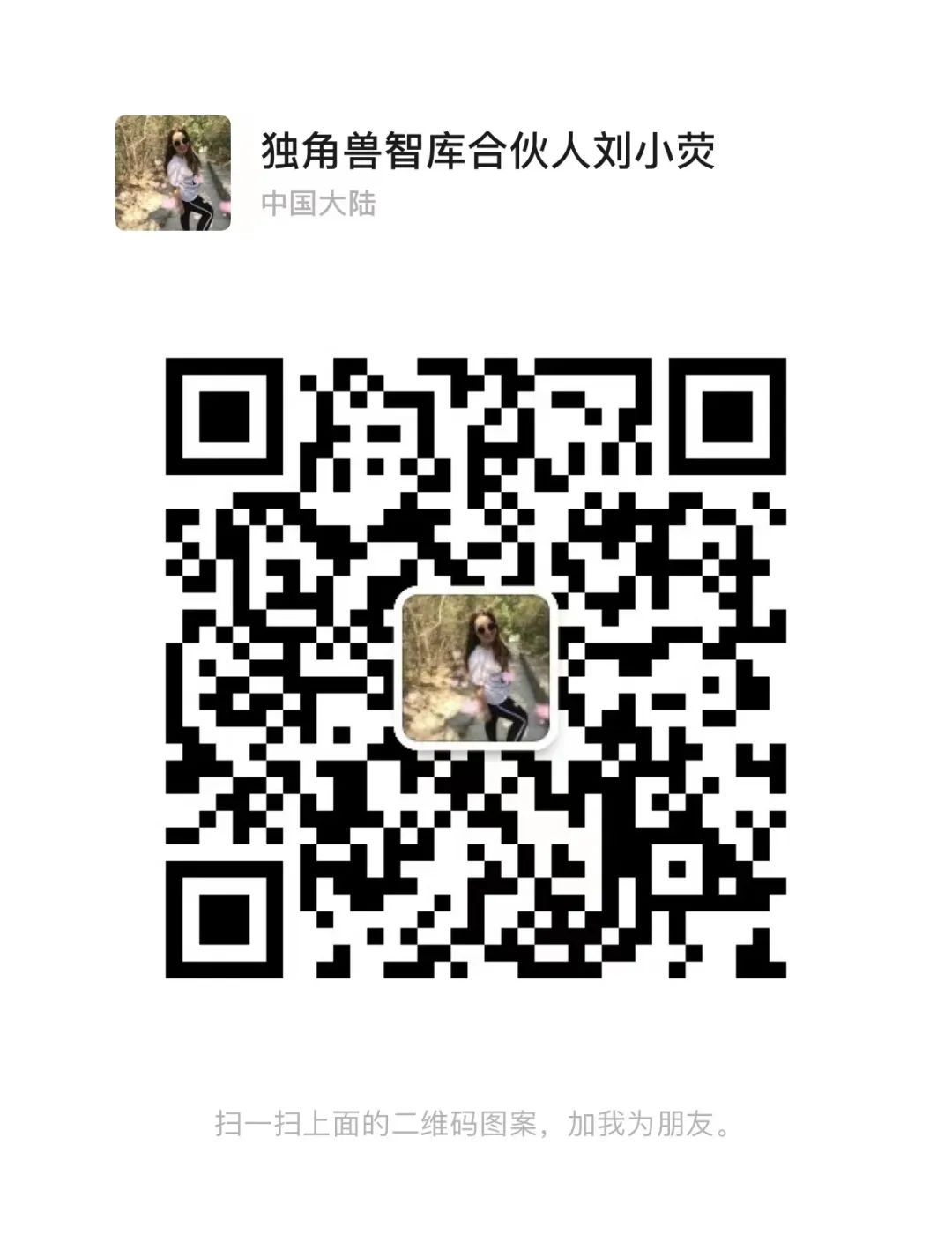
Other historical records in the group are as follows, you can verify the value of its intelligence. There are screenshots of historical chat records in the investment research intelligence group, which are sent in the daily articles after the market closes. Please check the historical articles for verification (selected intelligence from February)
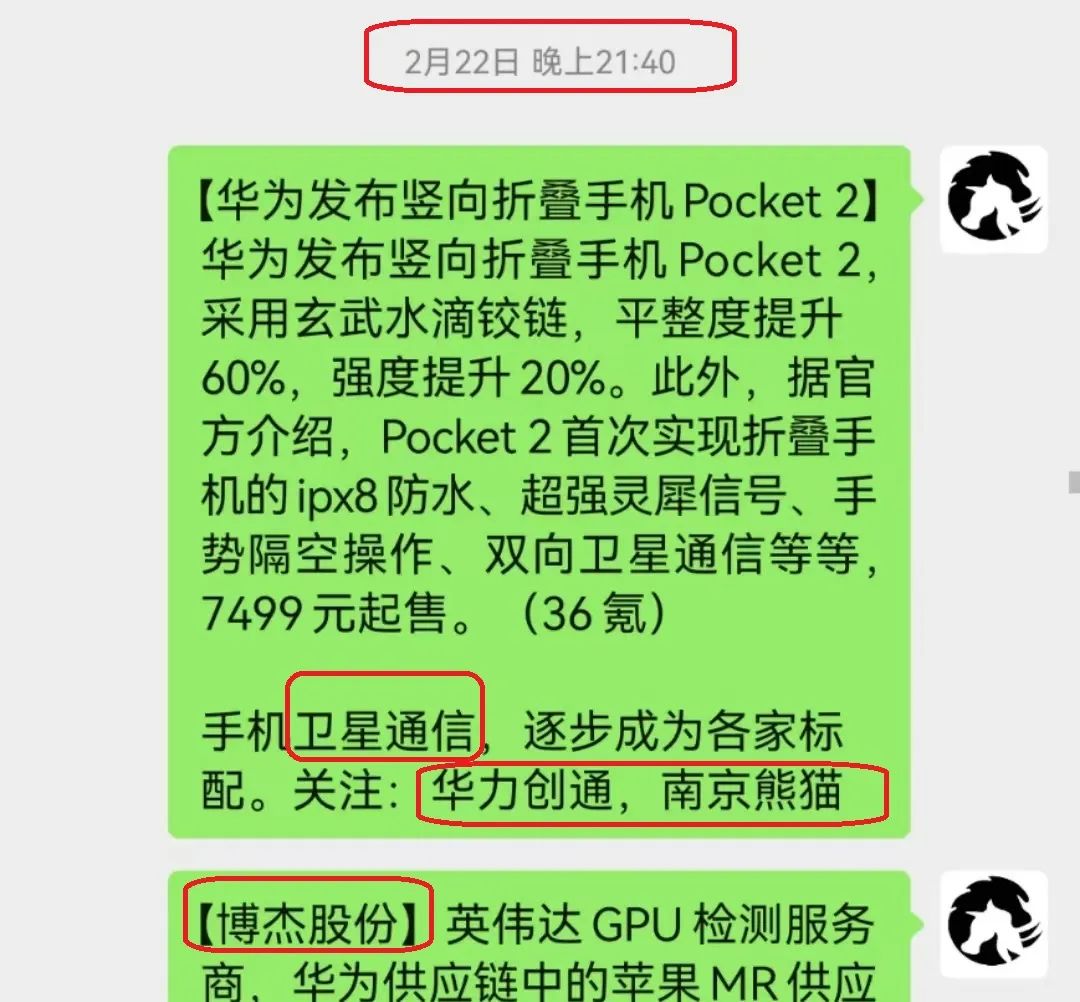
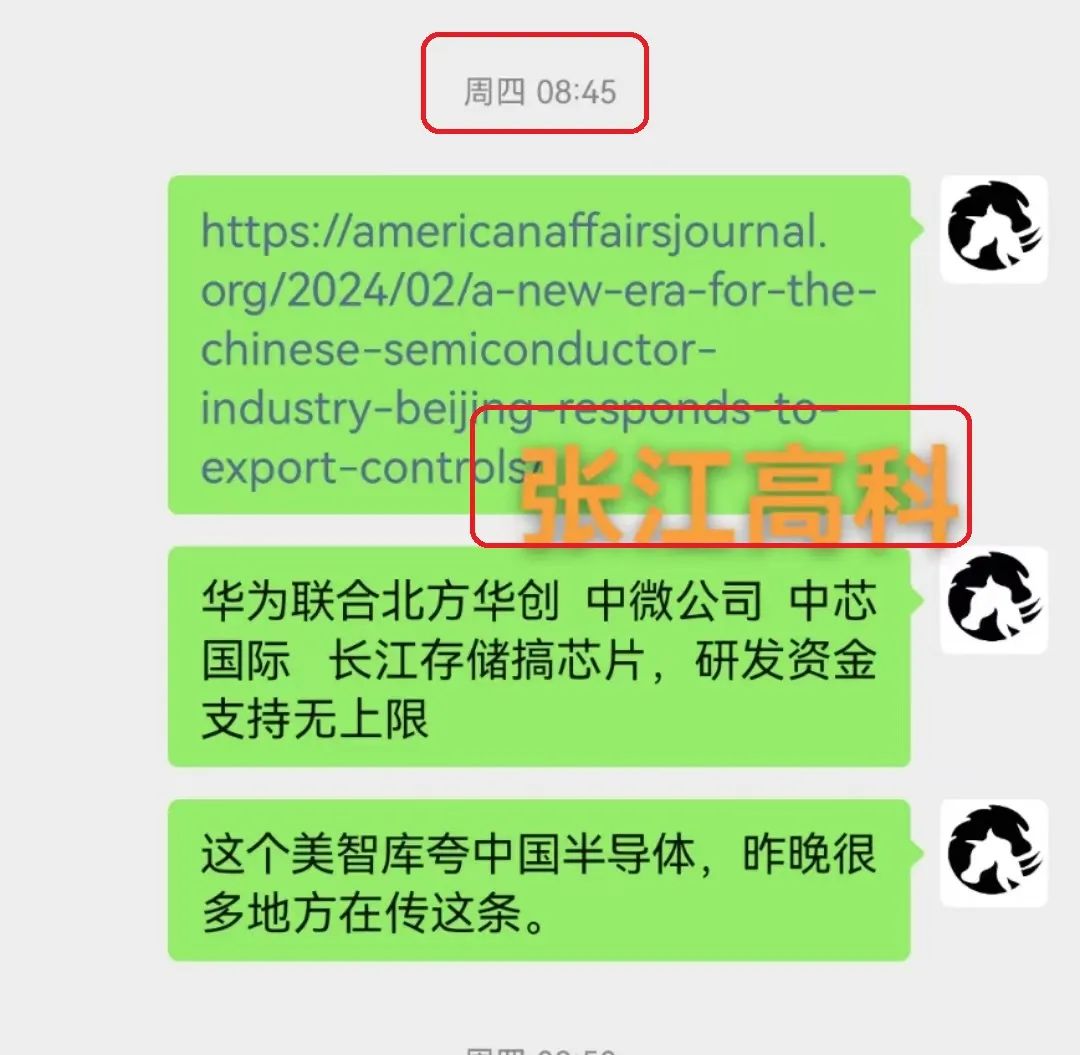
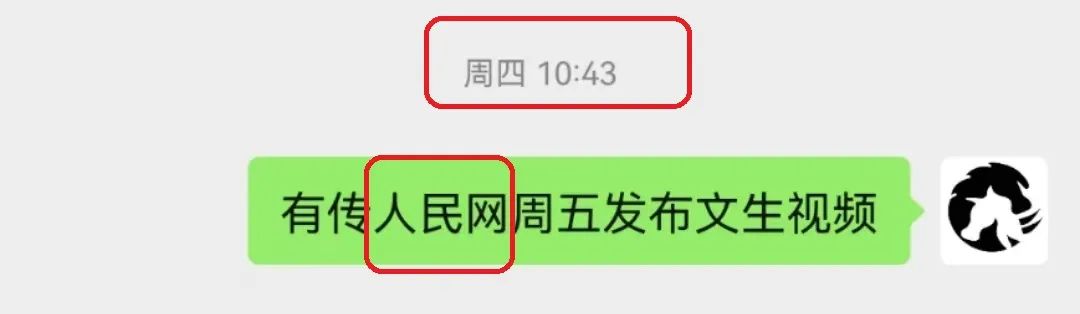
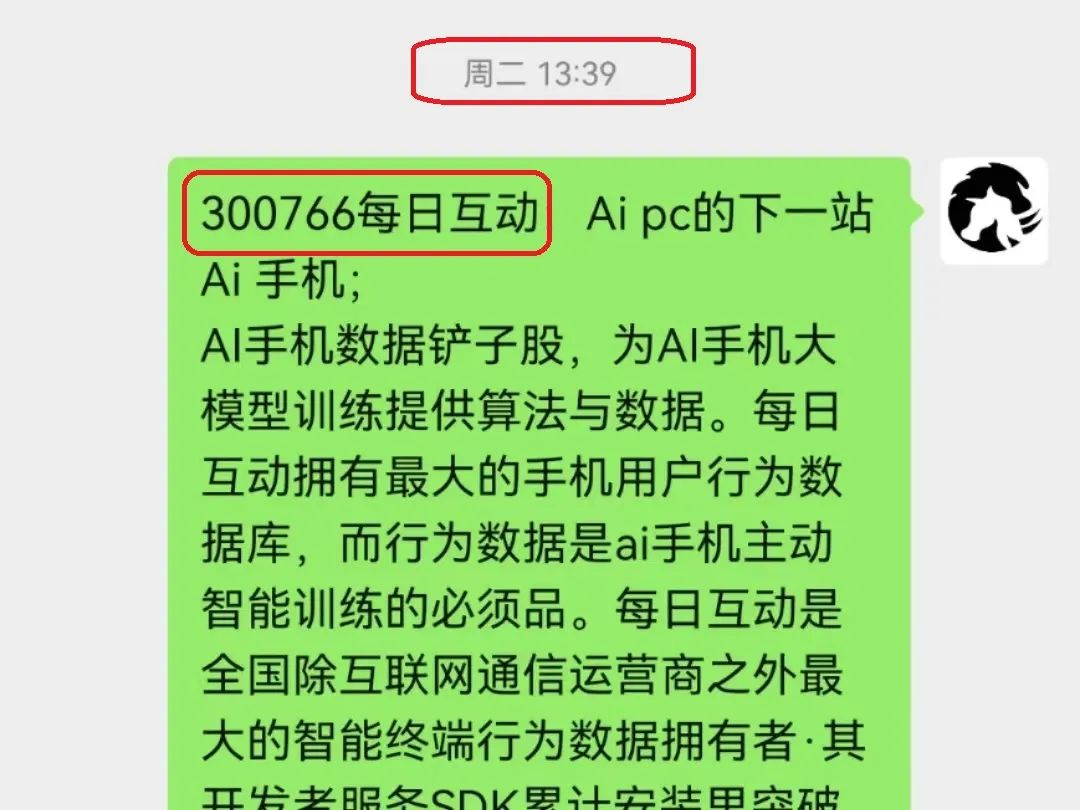
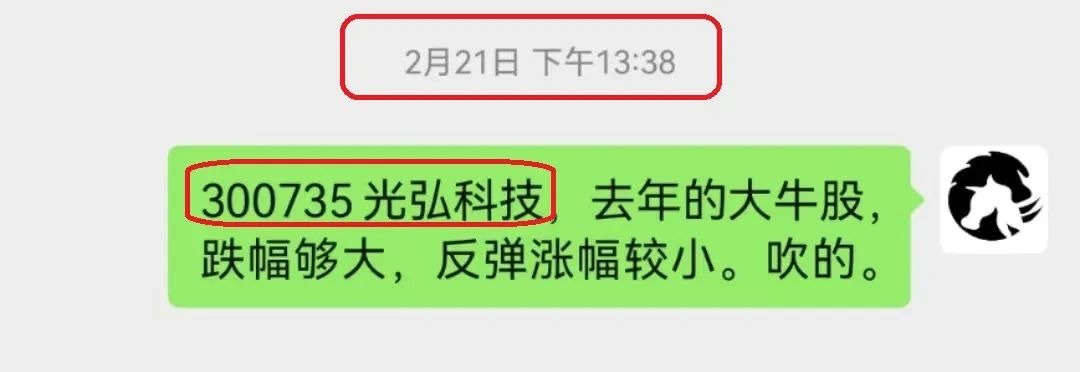
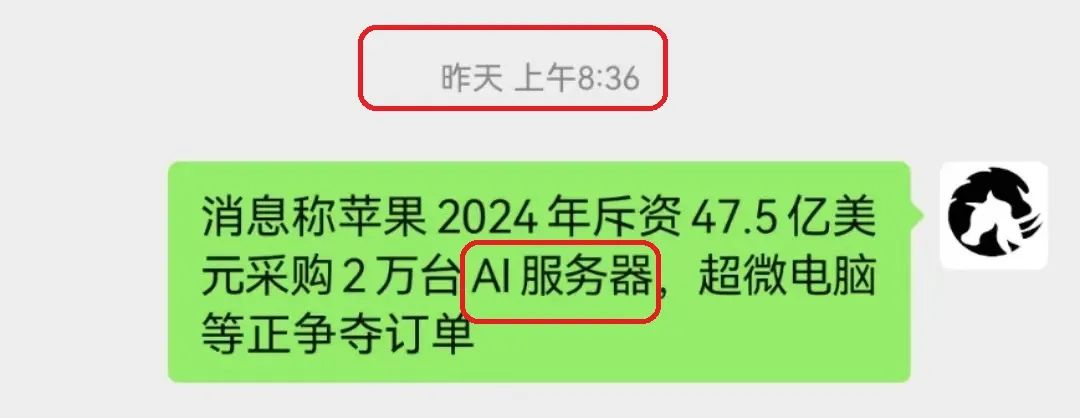
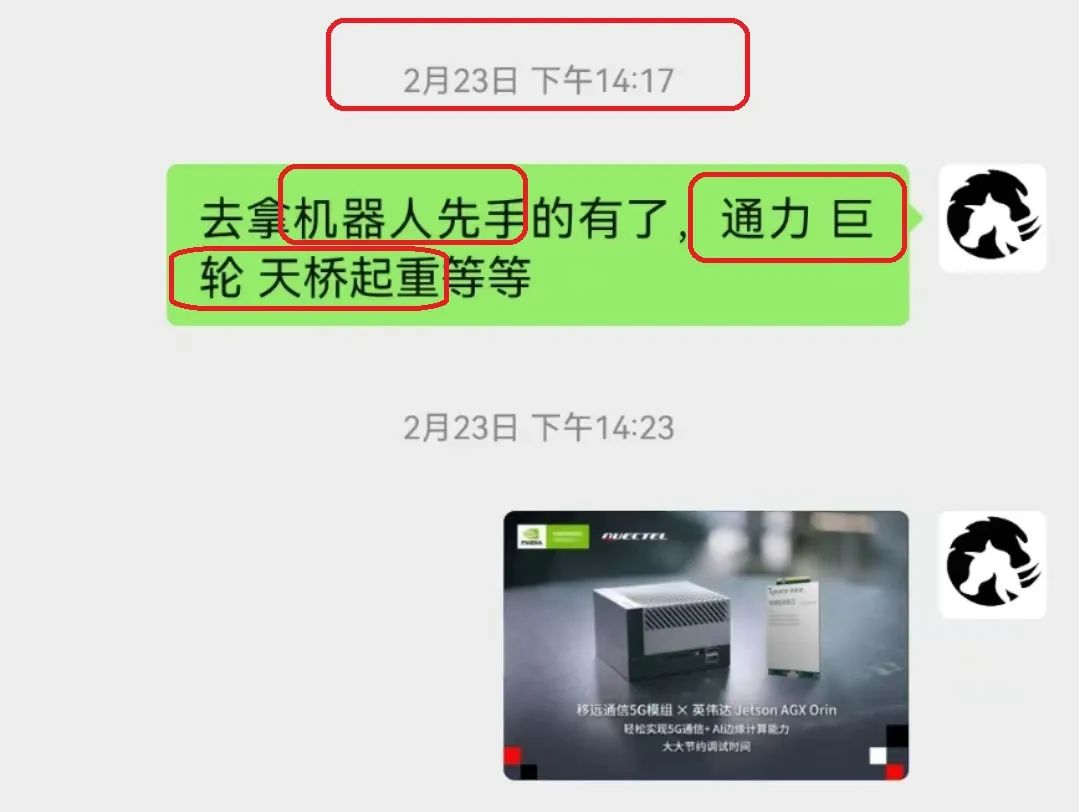
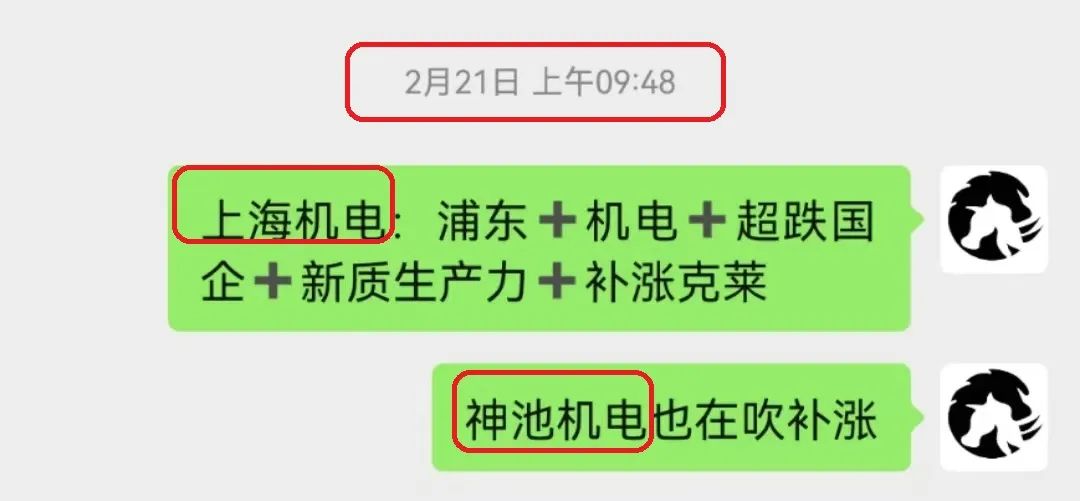
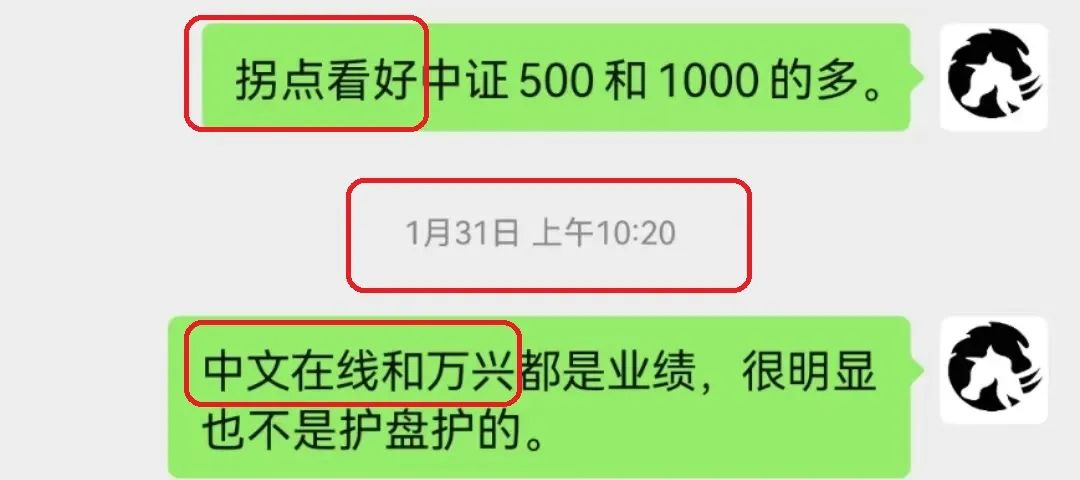
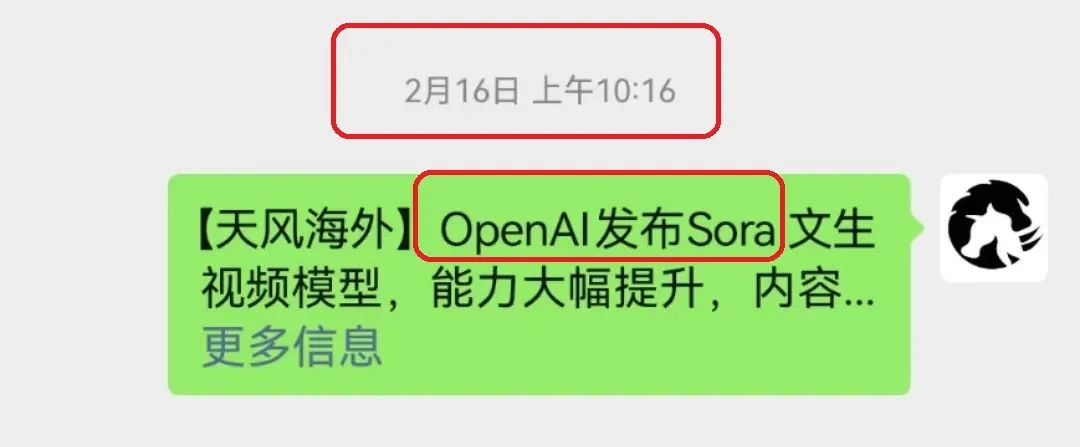
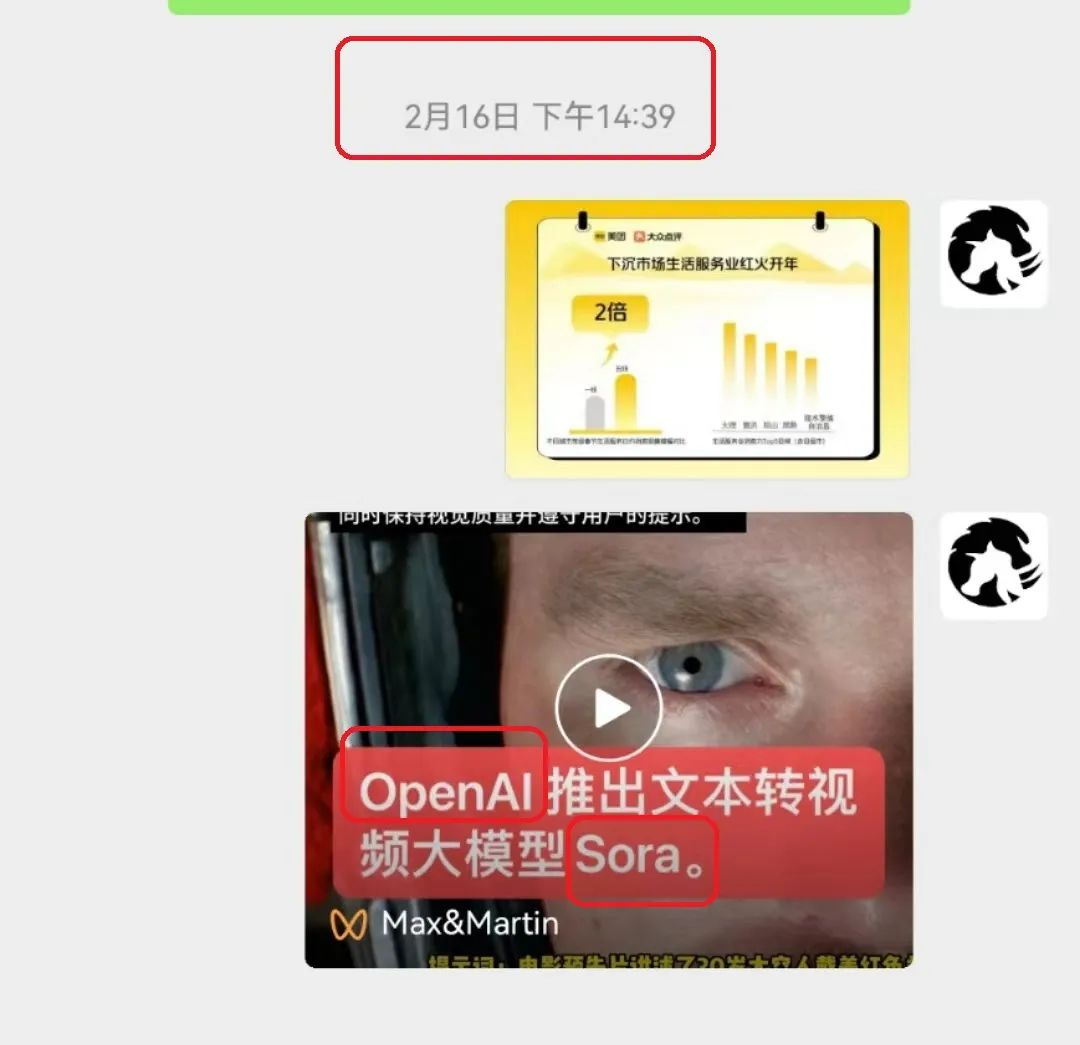
Prevent Losing Contact, Follow Backup Number