Introduction: Deep learning can be applied in various fields, and the shapes of deep neural networks vary according to different application scenarios.
The common deep learning models mainly include Fully Connected (FC), Convolutional Neural Network (CNN), and Recurrent Neural Network (RNN).
Each of these has its own characteristics and plays an important role in different scenarios.This article will introduce the basic concepts of these three models and the scenarios suitable for each.
01 Fully Connected Network Structure
The Fully Connected (FC) network structure is the most basic layer of neural networks/deep neural networks, where each node in the fully connected layer is connected to all nodes in the previous layer.
Initially, fully connected layers were mainly used for classifying extracted features; however, since all outputs and inputs of the fully connected layer are interconnected, it generally has the most parameters, requiring considerable storage and computational space.
The redundancy of parameters makes conventional neural networks composed purely of FC layers rarely applicable in more complex scenarios. Conventional neural networks are generally used in simple scenarios that rely on all features, such as housing price prediction models and online advertising recommendation models that use relatively standard fully connected neural networks. The specific form of conventional neural networks composed of FC layers is shown in Figure 2-7.
▲ Figure 2-7 Conventional neural network composed of FC layers
02 Convolutional Neural Network
A Convolutional Neural Network (CNN) is a type of neural network specifically designed to process data with a grid-like topology, such as image data (which can be viewed as a two-dimensional pixel grid). Unlike FC, the neurons in the upper and lower layers of CNN are not all directly connected; instead, they are connected through a “convolution kernel” as an intermediary, significantly reducing the parameters in the hidden layers through the sharing of kernels.
A simple CNN consists of a series of layers, each transforming one quantity into another through a differentiable function. These layers mainly include convolutional layers, pooling layers, and fully connected layers.
Convolutional networks have shown excellent performance in many application fields, particularly excelling in large image processing scenarios. Figure 2-8 illustrates the structure of a CNN, where a neuron is arranged in three dimensions to form a convolutional neural network (width, height, and depth). As shown in one of the layers, each layer of the CNN transforms a 3D input into a 3D output.
▲ Figure 2-8 Structure of CNN
03 Recurrent Neural Network
A Recurrent Neural Network (RNN) is also one of the commonly used deep learning models (as shown in Figure 2-9). Just as CNNs are specialized for processing grid-like data (such as an image), RNNs are designed for handling sequential data.
Since audio contains time components, audio can be represented as a one-dimensional time series; similarly, words in language appear one by one, so the representation of language is also sequential data. RNNs perform exceptionally well in areas such as machine translation and speech recognition.
▲ Figure 2-9 Simple RNN structure

-
Latest! Digital RMB launched, 50,000 people in Shenzhen share a 10 million red envelope!
-
The “Digital Twin Technology White Paper” is released (with full version download attached)
-
Two example problems explaining Bayes’ theorem
-
What is HBase? How does it work? Finally, someone explained it clearly
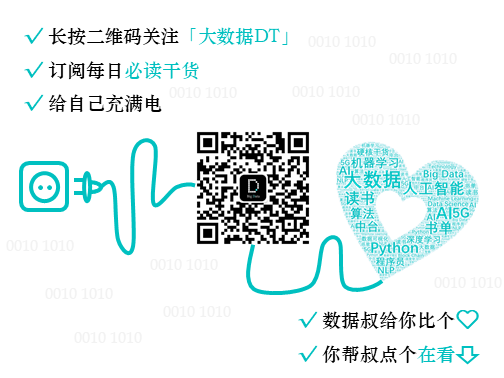