Click the blue words to follow us
In recent years, general artificial intelligence technology has rapidly developed and has become an important driving force for the digital upgrade and intelligent enhancement of the automotive industry. Many companies have successively established cloud-based large models to support the iterative updates and application expansion of vehicle-side functions. However, as user scale expands and usage frequency increases, the focus of AI processing is gradually shifting from the cloud to the edge. Compared with cloud-based large models, compressed edge large models have significant advantages in privacy protection, low-latency response, and personalized customization. Therefore, under the backdrop of continuously increasing application demands and strengthening computing power support on the vehicle side, the deployment of large models on the edge is becoming an important trend in the industry. However, since the industry is still in its early development stage, edge large models still face industrial challenges such as model compression and optimization, balancing parameters and performance, and collaborative iteration between cloud and vehicle models, while the ecological system for future application scenarios and multi-agent systems also needs further improvement.
Based on the above background, exploring the development trends and application scenarios of edge large models for smart vehicles, uncovering technical architecture pain points and solutions, and facilitating resource sharing and ecological interconnection among multiple agents have become the current focus of the industry. To this end, the China Society of Automotive Engineers held the 16th “Automotive Technology Review” closed-door seminar in Shanghai on the afternoon of October 30, 2024, inviting high-end experts from related fields to conduct in-depth discussions and exchanges around the theme of “Interpretation of Edge Large Model Technology for Smart Vehicles and the Application Ecology of Agents.”
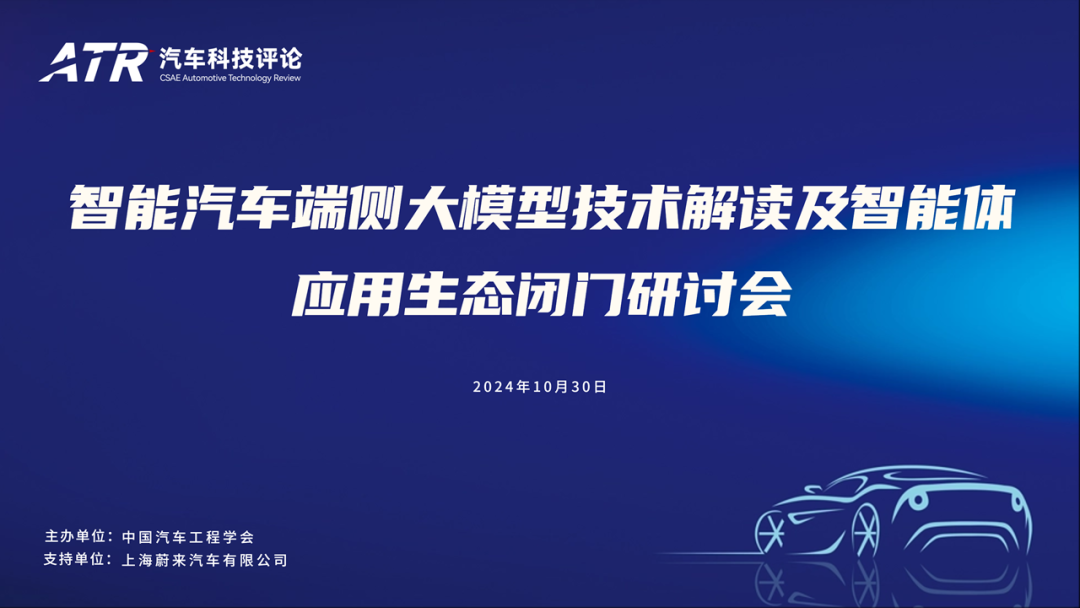
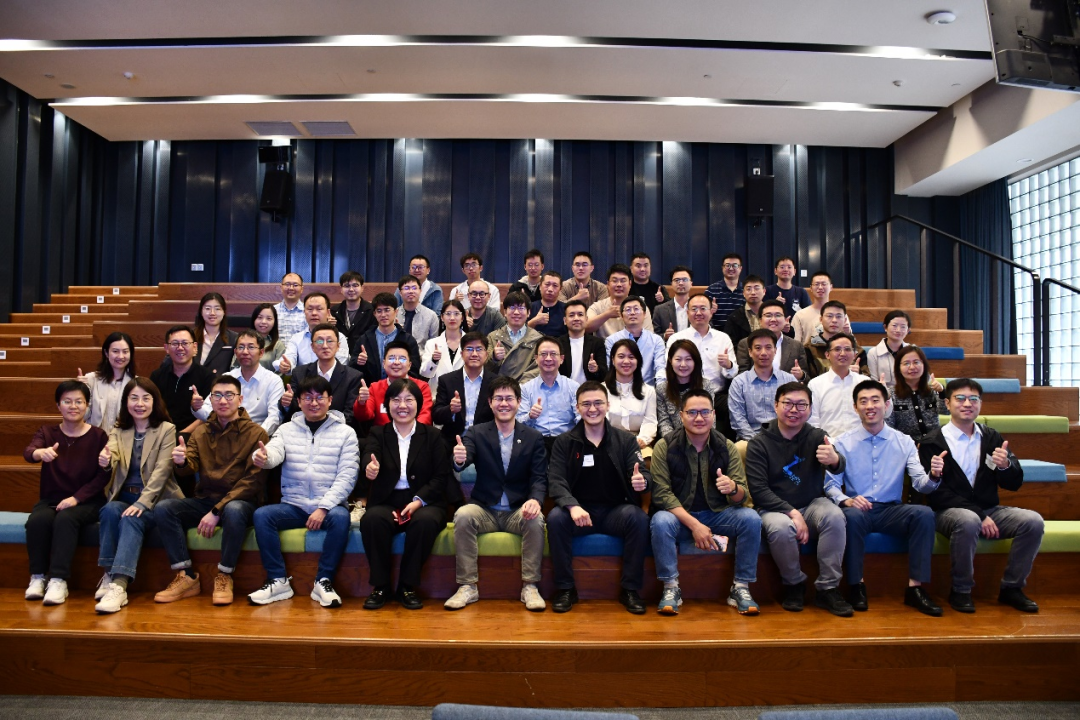
The seminar focused on two major dimensions: the development trends and application prospects of edge large models for smart vehicles, and the technical architecture and ecological interconnection, with in-depth discussions on seven sub-topics.
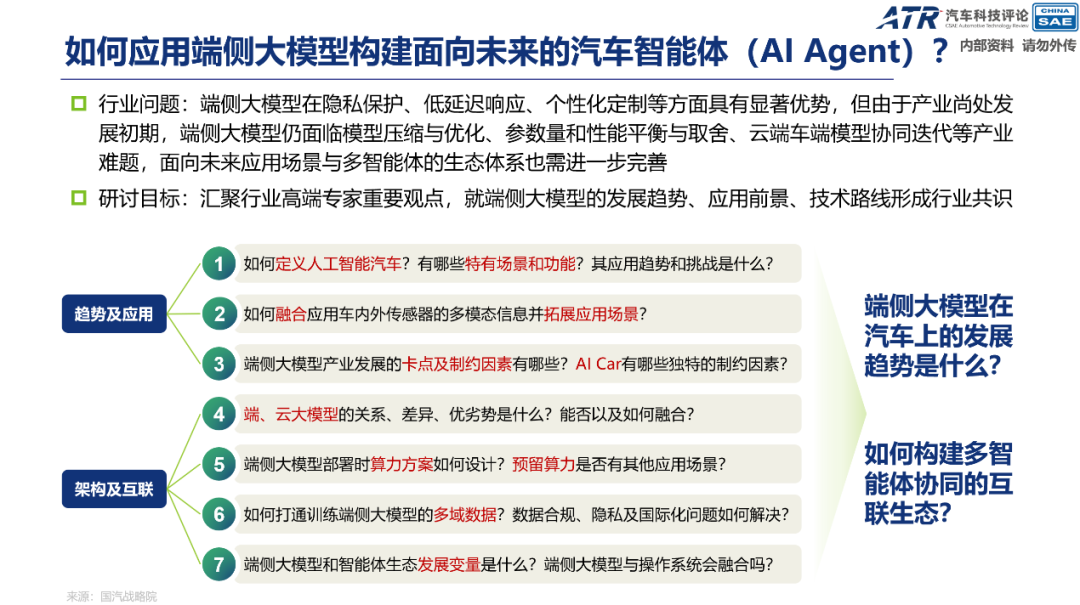
The seminar was introduced by Zheng Yali, Assistant Secretary-General of the China Society of Automotive Engineers and Deputy Director of the National Automotive Strategy Institute. Hu Chengchen, Chief Expert of Technology Planning at NIO and Vice President, chaired the thematic speeches and discussions. Miao Junwei, Vice President of Beijing Wall Smart Technology Co., Ltd., and Lei Zong, head of the NLP group for Xiao Ai at Beijing Xiaomi Pinecone Electronics Co., Ltd., delivered keynote speeches in turn.
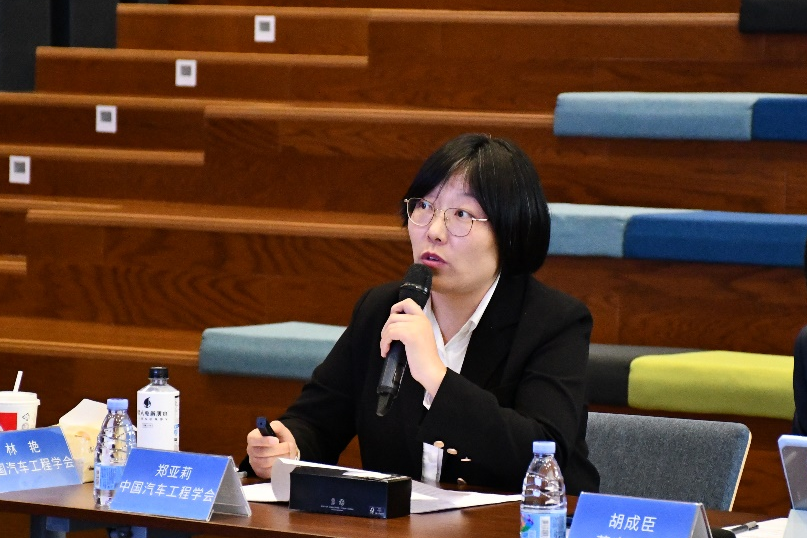
Zheng Yali, Assistant Secretary-General of the China Society of Automotive Engineers, and Deputy Director of the National Automotive Strategy Institute
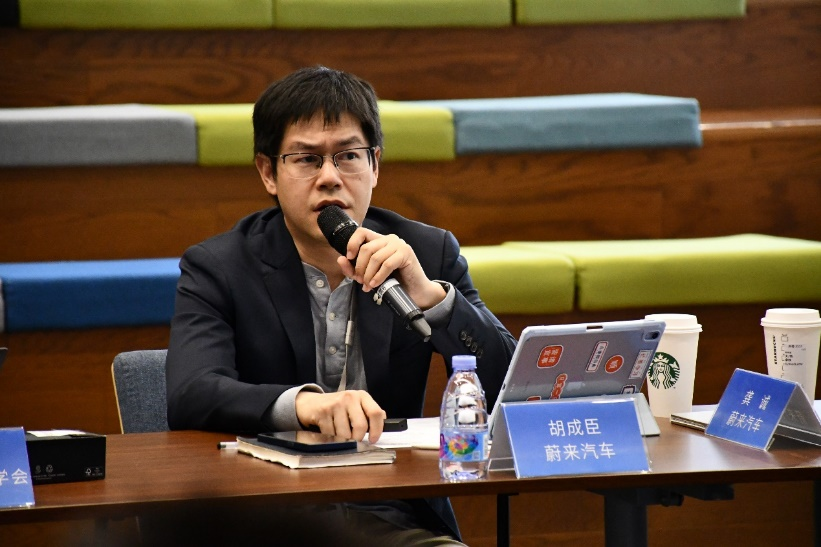
Hu Chengchen, Chief Expert of Technology Planning at NIO, Vice President, and Head of the General Artificial Intelligence Committee
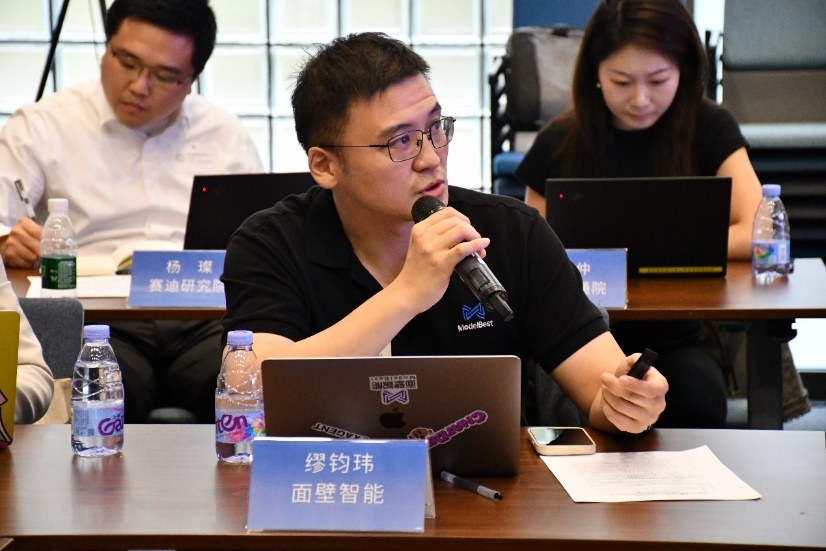
Miao Junwei, Vice President of Beijing Wall Smart Technology Co., Ltd.
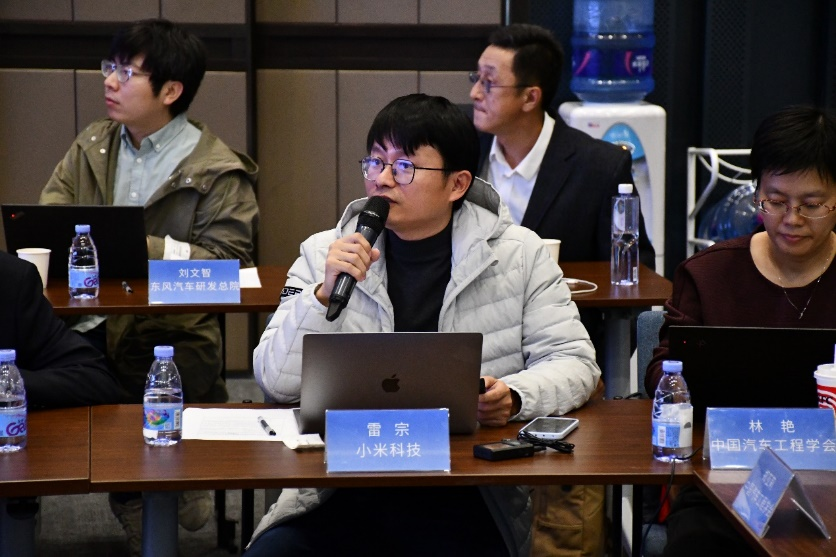
Lei Zong, Head of the NLP Group for Xiao Ai at Beijing Xiaomi Pinecone Electronics Co., Ltd.
In addition, experts from various vehicle manufacturers such as FAW Group, Dongfeng Motor, Lantu Motors, Changan Automobile, GAC Group, BAIC Group, BYD Auto, Li Auto, and Hozon New Energy, as well as related component companies such as Hezhima Intelligent, ZTE Communications, Neusoft Ruichi, Zebra Smart, Yiswei Computing, Huixi Intelligent, Bosch China, Denso China, and United Automotive Electronics, and experts from universities and industry institutions such as the University of Science and Technology of China, Fudan University, East China Normal University, CCID Research Institute, and China Academy of Information and Communications Technology shared their innovative explorations on building future automotive intelligent agents with edge large models and exchanged views on the seminar topics with participating guests.
This seminar formed the following main viewpoints based on the guest discussions:
1. In the short term, AI Car refers to smart vehicles that deploy edge large models. In the future, AI Cars are expected to integrate physical and digital worlds using multimodal native world models, becoming embodied intelligent agents that integrate human needs and optimize collaboration with traffic systems. AI Cars still face challenges in scenario application exploration, edge computing deployment, software ecosystem integration, and balancing cloud-edge collaboration with privacy security.
2. Compared with terminal devices such as AI PCs and AI Phones, the core difference of AI Cars lies in the multi-sensor inputs inside and outside the vehicle, making multimodal information fusion crucial. Therefore, it is necessary to build multimodal large models that can simultaneously process visual, audio, spatial, and other dynamic information, possessing comprehensive environmental understanding capabilities, and to expand innovative applications based on driver state for in-vehicle environment adjustment, external traffic participant analysis, and traffic flow dynamics.
3. The development of the edge large model industry still faces many bottlenecks and constraints. In terms of algorithms, further research is needed on edge model architecture, model compression, and model acceleration technologies, exploring knowledge-driven model training and intelligent agent development solutions; in terms of computing power, terminal hardware resources (chip architecture, computing performance, etc.) need to be compatible with edge large models, focusing on heterogeneous characteristics; in terms of data, the demand for data in the three stages of pre-training, instruction fine-tuning, and preference alignment should form a scalable and diversified data construction system.
4. Edge large models are typically compressed from cloud-based large models, focusing on enhancing model knowledge density. Future cloud-edge collaboration will become the basic form in the AGI era, achieving functional and data complementarity in different scenarios, with strong perceptual understanding and high privacy-related and real-time requirements prioritized for deployment on the edge, while more complex generative models and knowledge retrieval models will tend to be placed on the cloud.
5. The computing power demand of edge large models mainly relies on the comprehensive performance enhancement of edge-side chips. When vehicle-side computing power is insufficient, mobile phones and other expansion devices can also serve as options for shared computing power. When reserved computing power is idle, it should first be considered for use by the vehicle owner’s other devices, and secondly, it can be scheduled as distributed computing power under the premise of ensuring data privacy and security.
6. To facilitate the collection, processing, and storage of multi-domain data required for training edge large models, the electronic and electrical architecture design must first support the efficient transmission of data from the vehicle to the cloud; secondly, it is essential to define and acquire high-quality data available for model training from the scenario perspective, as well as the data required for the deployment inference phase of large models and intelligent agents. Regarding data security, it is crucial to provide localized and compliant solutions.
7. The transformation of interaction methods is a key variable in the development of edge large models and the intelligent agent ecosystem. In the future, edge large models will integrate with operating systems, with operating systems evolving towards AI OS. On one hand, the improvement of underlying technologies will give rise to various innovative applications, while on the other hand, the emergence of phenomenal applications will also prove the value of edge large models and AI OS.
The above content is organized based on the opinions of the guests on site and does not represent the final viewpoint. The research team of the China Automotive Society will soon form the final research report of this seminar, and we welcome your attention!
Contact Person:
Chen Min
Phone: 13810027168 (same as WeChat)
Email: [email protected]
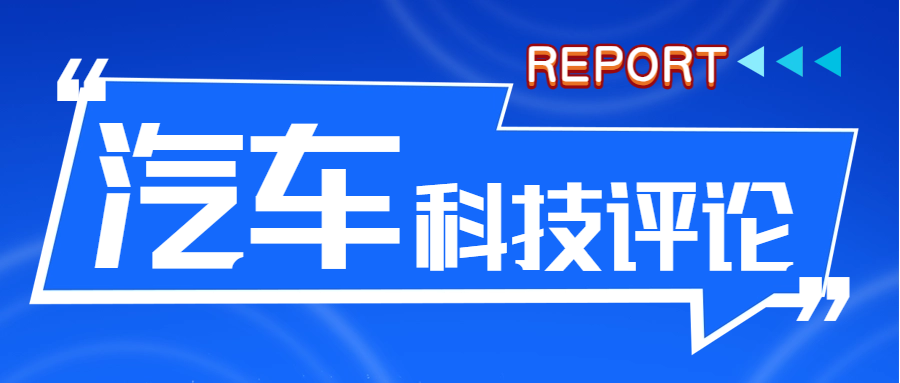
Copyright and Disclaimer:
1. If you need to reprint or quote, please indicate at the beginning or end of the article: Content sourced from the γEnergy Saving and New Energy Vehicle Technology Roadmapγ public account.
2. If there are any copyright issues with the videos, images, or texts used in this article, please notify us as soon as possible, and we will confirm the copyright based on the proof materials you provide and pay remuneration according to national standards or delete the content immediately!
Contact phone: 18513410660