At the recent “2022 China Artificial Intelligence Industry Annual Conference” main forum, Jiang Changjun, Supervisor of the Chinese Association for Artificial Intelligence and Academician of the Chinese Academy of Engineering, stated in his report that the development of artificial intelligence has undergone several decades. The large model ChatGPT suddenly emerged around the Spring Festival this year, and everyone has not had time to think deeply before it has already “hit us in the face”.
Jiang Changjun
The research of science and technology is roughly divided into two paradigms: one is the theoretical calculation paradigm established by Newtonian mechanics, and the other is the data paradigm initiated by Kepler. It has gone through experimental induction, logical deduction of theories, as well as computational simulations and recent data-intensive scientific discoveries. Especially recently, scientific discoveries based on data, mainly fitting high-dimensional complex functions with big data to discover their inherent laws and patterns, are referred to as the fourth paradigm.
The human brain makes decisions by utilizing past background knowledge and current perceived data; large models make decisions for machines by learning to adapt to downstream tasks. In this process, large models integrate the results of some general tasks and provide good representations for downstream tasks, similar to the experience provided by background knowledge in the human brain, and also similar to how the human brain quickly adapts to new environments with background knowledge.
Traditional models are generally smaller in scale, such as expert systems and knowledge bases. They are models with some knowledge reasoning, where a small amount of proprietary knowledge can be processed through centralized algorithms under low computing power, thus the problems they can solve are relatively limited. However, large models are different, with large-scale improvements in computing power, data, and models. They drive reasoning models of generalized knowledge through distributed algorithms supported by massive generalized data.
The support of large computing power leads to great intelligence. The algorithm of ultra-large-scale model parameters, that is, ultra-multi-layer neural networks, has data that consists of ultra-large-scale data samples, and computing power belongs to the category of ultra-large-scale computation. Under such conditions, our understanding and processing of natural language evidently have a gathering effect. Therefore, the leap of this large model can be summarized as the optimization of large model algorithms, which not only improves the utilization of data but also alleviates the bottleneck of computing power demand. This is how we mine complex rules from large models and big data.
The optimization of large models has gone through several processes. First, it started with early statistical language models, then moved to task-specific neural network embedded models, and then to pre-processing models based on deep learning. Pre-trained models provide good representations for natural language processing. ChatGPT is the latest derivative of natural language processing. This process has shown performance in some specific tasks, such as achieving an average score of medium level in the college entrance examination in the United States. Similar to AlphaGo’s early games with humans, it can self-train and endlessly improve its own level. The space expanded by AlphaGo is far greater than that of humans, with a broader experience during the game process. However, on the other hand, Go is ultimately a limited space, and playing on a 19×19 board has rules. The sample space of ChatGPT’s data is infinite, and the process has no fixed rules, making its problems more complex. In this situation, ChatGPT can approach a basic level of human intelligence, which surprises us.
The key technologies in large models mainly include language generation, context learning, and world knowledge. These three abilities come from the pre-training process of large models, code training, instruction fine-tuning, and reinforcement learning based on human feedback. Currently, ChatGPT4 has emerged, and this process has sparked strong reactions in academia. For example, Geoffrey Hinton initially remained silent but recently left Google and expressed deep concerns.
Convolutional neural networks and recurrent neural networks are among the key technologies, and the process of Transformer is particularly noted. The development of ChatGPT, along with the basic models, optimization of Transformers, plus the “support” of computing power and data, has made the performance of this large model quite extraordinary. Key technologies of large models also include thinking chains, which divide cognitive processes into several stages, with each stage further refined and represented. Thinking chains play a very important role in studying cognitive behavior by breaking down a larger problem into smaller problems and predicting the thinking process to provide hints. In terms of large models, they can better utilize corpus databases to provide more accurate reasoning. To some extent, the output thinking process for each prompt can explain the model’s output.
Great intelligence includes three aspects: large models, large computing power, and big data. One of its research trends is to continue to seek qualitative changes through the scale of data, computing power, and algorithms in the changing quantitative process. In the process of seeking emergence from quantitative change, we can observe the performance of data, algorithms, and computing power in the vertical direction. First is high-quality data, as the quality of data is very important; second is efficient algorithms and effective computing power, which can ensure the accuracy and precision of cognitive processes. In these areas, we are still continuously exploring and researching, improving data utility through the collection of multimodal data. In terms of algorithms, improving algorithm efficiency is our goal; in terms of computing power, the effective performance behavior of computing power can also be advanced and progressed.
The verticalization of large models is the second trend of great intelligence. One is domain-based foundational models, and the other is industry-based foundational models. For instance, the widely concerned foundational model for risk control in security—this is not only a problem in a specific industry but a common issue across related industries. For example, in the financial industry, the foundational model for financial business is the industry’s foundational model. Just like the knowledge structure of humans, how to apply domain and industry common sense based on general knowledge is an important trend of verticalization. Of course, there are some problems faced in this process. For instance, the current industry situation often directly uses foundational models, which often have a certain gap with the specialized application needs in scenarios, reflecting issues such as a lack of industry knowledge, high usage thresholds, and relatively difficult deployment. These are the three major foundational problems we currently face.
Regarding the industrial model issues of vertical industry large models, one starts from social division of labor, and the other from industrialized mass production, encompassing industry task data as well as corpus and knowledge. The large model platform corresponds to industry large models, general large models, and general big data, filtering effective knowledge from data and making use of it. Therefore, overall, its verticalization is a collaborative effort across the entire industry, viewed from the data perspective, including static data, data in transmission, and other data sharing mechanisms. Additionally, industry large models focus on specific industry attributes, accelerating intelligent upgrades. The application of foundational large model research achievements must have specific applications, and the common goal is to obtain knowledge from data.
Currently, various industries are trying to establish their own large models. In vertical industries, computing power supply also faces challenges: firstly, it needs real-time updates, as timely knowledge in the field needs to be updated, requiring comprehensive satisfaction of diverse service types to adapt to their respective business needs. Secondly, it needs to respond promptly, especially for some sudden computing tasks; for example, risk control in the financial transaction field often faces sudden situations, requiring vertical domain computing power supply to adapt to on-demand characteristics.
In terms of comprehensive computing power supply, the aggregation of computing power includes data from endpoints, edge data, and cloud data. In the combination based on computing networks, intelligent computing networks, supply-demand balance, unified orchestration, and other technologies need to be strengthened. Additionally, usage should be on-demand; for instance, in intelligent inference, different industrial internet environments, remote medical care, etc., there are different requirements. Especially in highly mobile areas, dedicated allocation of computing power is very important. Based on demand, scalable and customizable computing power supply can be generated. In this process, we have established a modular computing mode that combines computing power, algorithms, and data, forming a flexible and mobile supply method, which is very important for the overall computing network.
Model risk is also one of the risks. Model risk refers to solving some false issues, which is one of the core challenges in further developing foundational models. It also includes safety issues, data risks, and infringement, etc. Data privacy will face increasingly severe challenges; how to protect privacy issues must be considered in the safety of large models. Additionally, there are multivariate risks in security, integrating computing power, algorithms, and data, with overall security issues arising from the combination of data security, system security, and model security, all of which need to be considered as foundational issues.
The development trend of large models is towards secure, trustworthy, and controllable models, expanding from single industries to vertical industries and fields, which brings social issues that should be taken seriously. In summary, first, the progress of great intelligence research is “enriching data + expanding models + increasing computing power,” thus generating “thinking chains + self-attention mechanisms” as the key principles. Second, the development trend of large models, seeking emergence from quantitative changes, as well as the verticalization and enhancement of large model security, are issues that must be addressed.
(Compiled from the report of the “2022 China Artificial Intelligence Industry Annual Conference”, with some omissions)
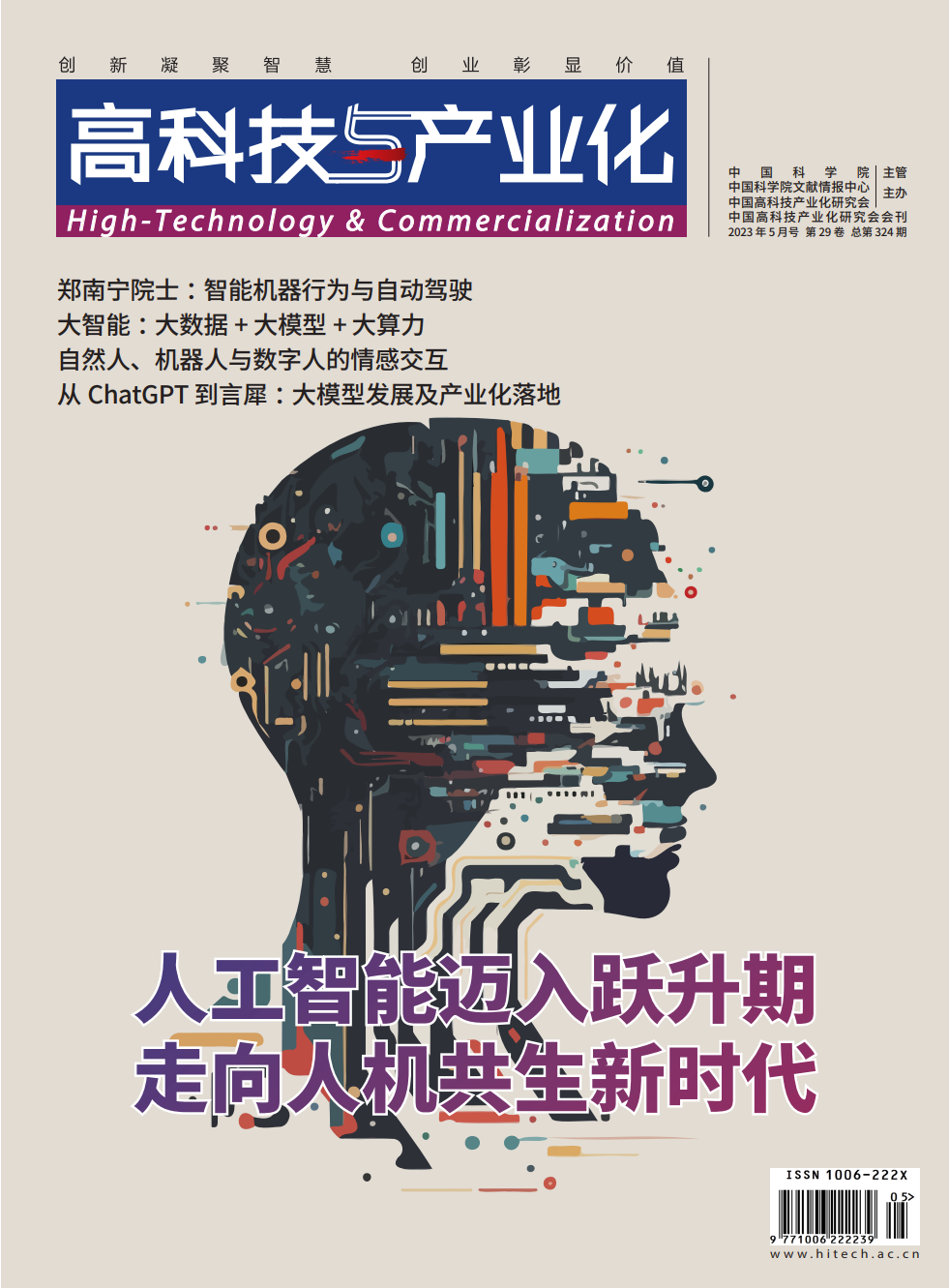
For more content, please subscribe to “High Technology and Industrialization” magazine
Address: 33 North Fourth Ring West Road, Zhongguancun, Haidian District, Beijing (100190) Phone: 010-62539166
Email: [email protected]
Website:http://www.hitech.ac.cn
12 issues a year, 58 yuan per issue, annual price 696 yuan
Postal code: 82-741
ISSN: 1006-222X CN11-3556/N