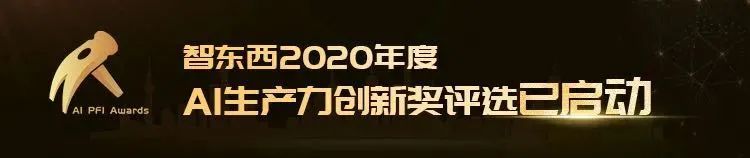
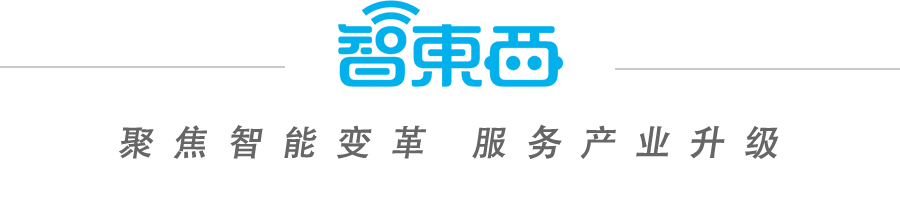
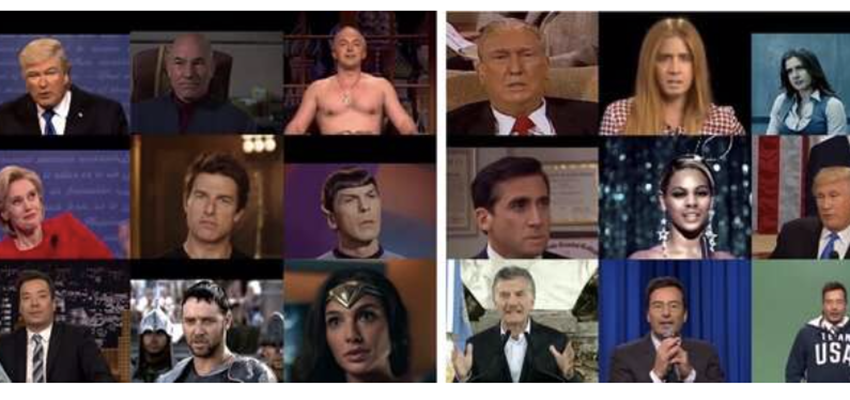
Rumors Stop at FakeCatcher.
On January 13, ZhDongxi reported that with the popularity of one-click face-swapping applications like Deepfake, more and more ordinary people can easily modify the images and even the voices of people in videos, achieving a level of realism that is hard to distinguish from the real thing. However, behind the fun, there are also risks that promote rumors and invade privacy.
In January 2019, Binghamton University collaborated with Intel to launch a video image detection tool called FakeCatcher. This August, researchers published a paper titled “How Do the Hearts of Deep Fakes Beat? Deep Fake Source Detection via Interpreting Residuals with Biological Signals,” achieving an accuracy of 97.29%.
How does FakeCatcher realize its “anti-fake” function? Will it lead to Deepfake “anti-anti-fake”? And what new breakthroughs and innovations will FakeCatcher have two years later? Today, ZhDongxi will explain it all to you.
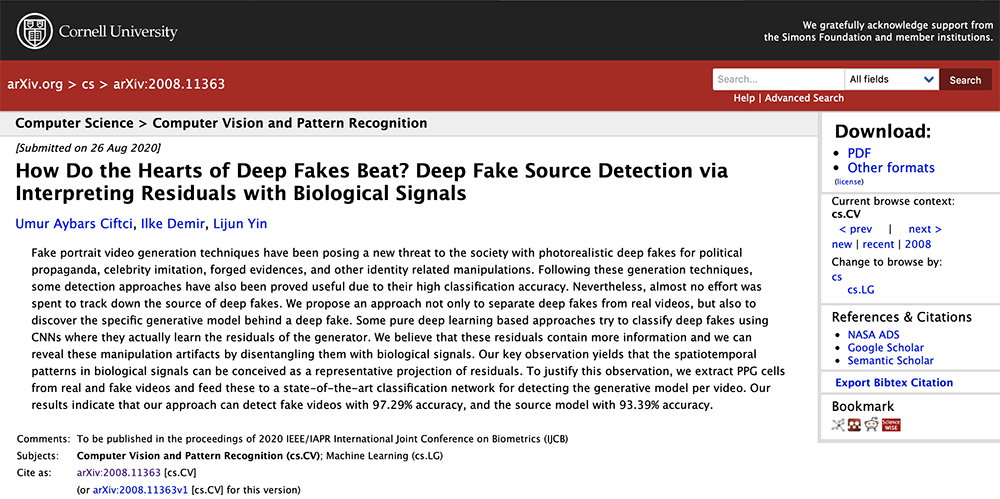
Is Deepfake All-Powerful? Heartbeat and Pulse to “Fight Fake”
FakeCatcher is based on the principle of the subtle skin color differences produced by the human heartbeat, and specifically applies the same technology as the fingertip pulse oximeter and Apple Watch used to measure exercise heart rate—photoplethysmography (PPG).
Ilke Demir, a senior research scientist at Intel, stated: “We extracted several PPG signals from different parts of the face and detected their spatiotemporal consistency. In videos synthesized through deep learning, the heartbeat signal has neither consistency nor any relation to the pulse signal. In real recorded videos, the blood flow in the human face is consistent with the pulse, which is the heartbeat signal.”
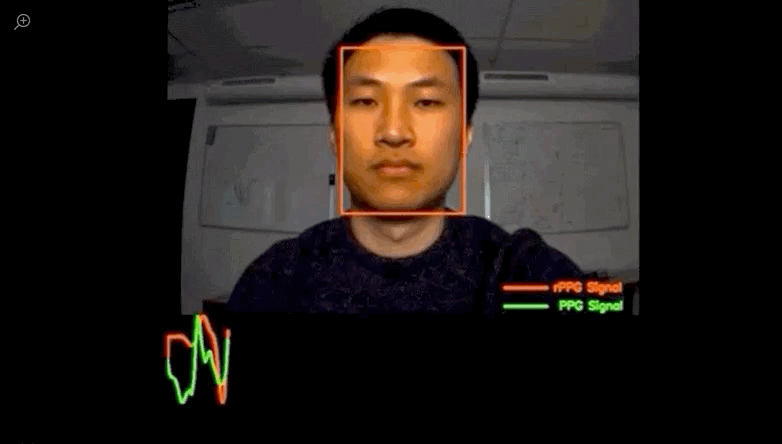
▲PPG and rPPG signals of the human face
Collaborating with Demir is PhD Umur A. Ciftci from Binghamton University’s Computer Science Department and his advisor, Professor Lijun Yin from the Graphics and Image Computing Laboratory. The laboratory’s multiple 3D face and expression databases have been used by several Hollywood filmmakers and video game creators for film or game projects.
In the laboratory, Ciftci established the most advanced physiological signal collection equipment in the United States using 18 cameras and infrared cameras. During the experiment, subjects had to wear devices that monitor breathing and heart rate, and 30 minutes of data required 12 hours of computational processing.
Yin said: “Umur did a lot of physiological data analysis and processed the signals with our first multimodal database. We collected not only 2D and 3D visible image data but also data from thermal cameras and physiological sensors. Using physiological features to detect image forgery will be a new approach in the future.”
To Fight “Fake”, First Create “Fake”
Compared to the images collected in the experiment, the quality of “fake photos” processed by Deepfake is much lower, which means that synthesized photos and videos can be more easily detected.
Ciftci said: “We will process the 3D images with the collected physiological signals and synthesize some ‘fake’ videos. Unlike Deepfake, we use real subjects’ data for processing, while Deepfake uses data from the internet. But if we only consider the ‘fake’ aspect, there is not much difference.”
“Just as police know how criminals commit crimes, if we want to find those fake photos, we must first know how they are made. Even when we create our database, we also used some methods from Deepfake.”
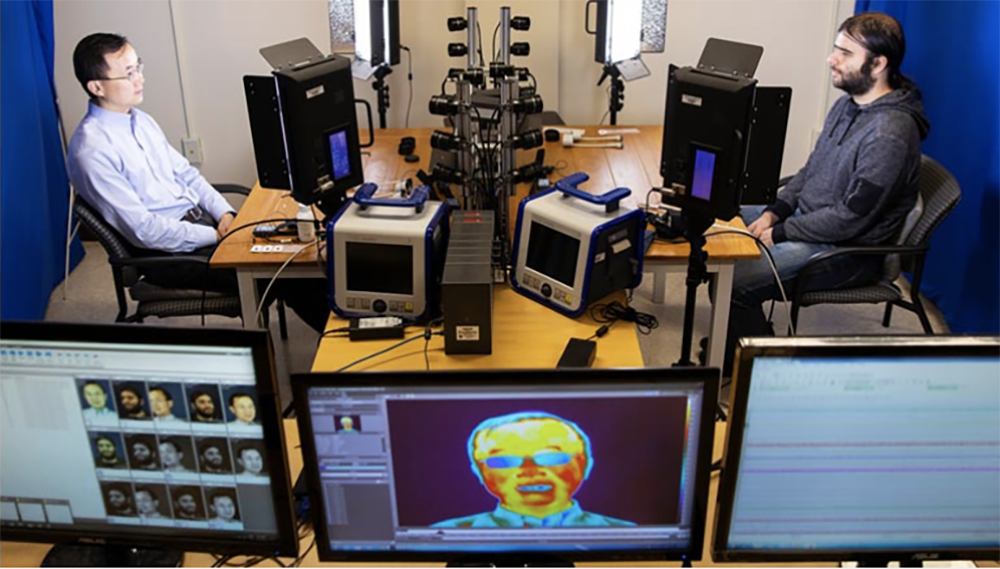
▲Lijun Yin (left) and Umur Ciftci (right) in the 3D scanning laboratory, source: Jonathan Cohen
Since the publication of FakeCatcher, a total of 27 researchers worldwide have used the algorithm and dataset in their own studies. However, many are concerned that if these research results are made public in the future, Deepfake creators might learn the verification process and modify algorithms to upgrade Deepfake, making synthesized photos harder to detect in the future.
But Ciftci is not too worried about this: “For those who do not understand physiological signal processing, breaking through physiological signal detection is not easy. Without major software updates, Deepfake creators cannot simply use existing technology to achieve this goal.”
Besides Being Able to “Fight Fake”, It Can Also Make Movies
One of the main reasons Intel is involved in FakeCatcher is due to its interest in volumetric capture, which is capturing information about the same person from multiple cameras in all directions and combining it to create a three-dimensional model that can be seamlessly placed in any environment, which will have significant implications for AR and VR.
Intel claims to be conducting the world’s largest volumetric capture experiment: under a 10,000 square foot grid dome with 100 cameras, the venue can accommodate about 30 people at the same time, and subjects can even ride several horses.
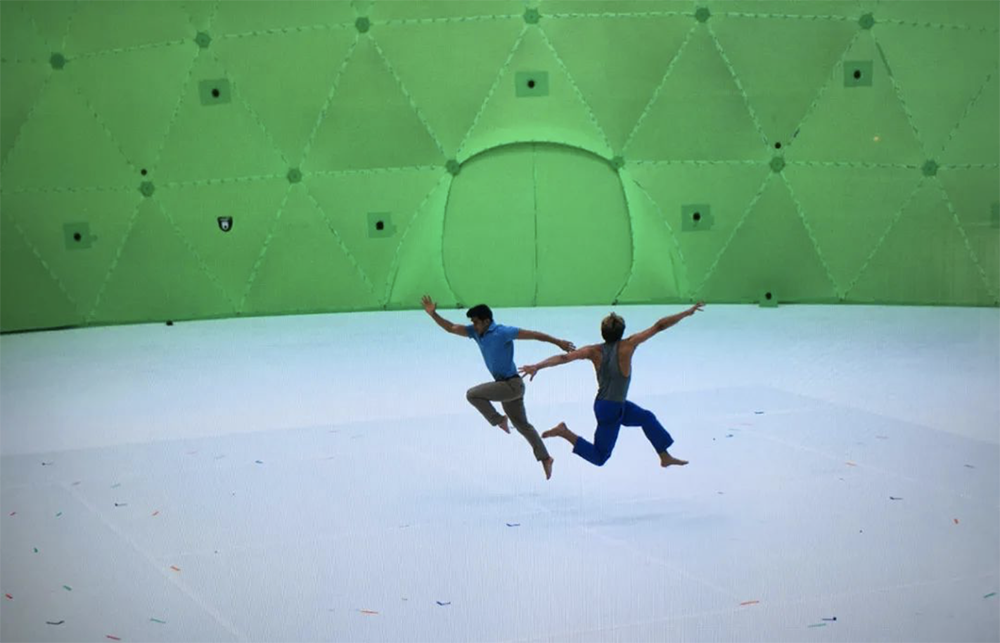
▲Intel’s experimental venue
By compiling and reverse-engineering FakeCatcher data, Intel hopes to create more realistic effects by combining real biological information.
Intel’s future plans include applying volumetric capture technology in television shows, sports events, and augmented reality, allowing viewers to immerse themselves in these scenarios. In addition, Intel will also venture into 3D and VR film production, with a recent VR project involving Intel premiering at the Venice Film Festival.
Demir stated that Intel is transitioning from a chip-centric approach to focusing on AI, edge computing, and data, exploring AI applications in its business as much as possible.
Endless “Fighting Fake”, Further Progress of FakeCatcher
In the paper published this August, Demir and others proposed a deep forgery source detection model using biological signal residuals, achieving an accuracy of 97.29% in detecting forged videos, and an accuracy of 93.39% in inferring the generative model behind the forged videos.
Because the PPG signals used to detect physiological changes such as heartbeat and blood flow are difficult to mimic, there has not yet been a generative model that can create forged videos consistent with PPG signals. Therefore, researchers can not only identify forged videos by the inconsistency of PPG signals but also find the generative model behind the forged videos through the residuals between PPG signals.
Researchers extracted 32 types of raw PPG signals from different facial locations through a frame window from videos and encoded the signals and their spectral density into a spatiotemporal block, known as a PPG unit. The PPG units are then processed through a neural network to identify the different residual features of the source generative model. Finally, the feature sequences are combined using probabilistic average logarithm to predict the generative model behind each video.
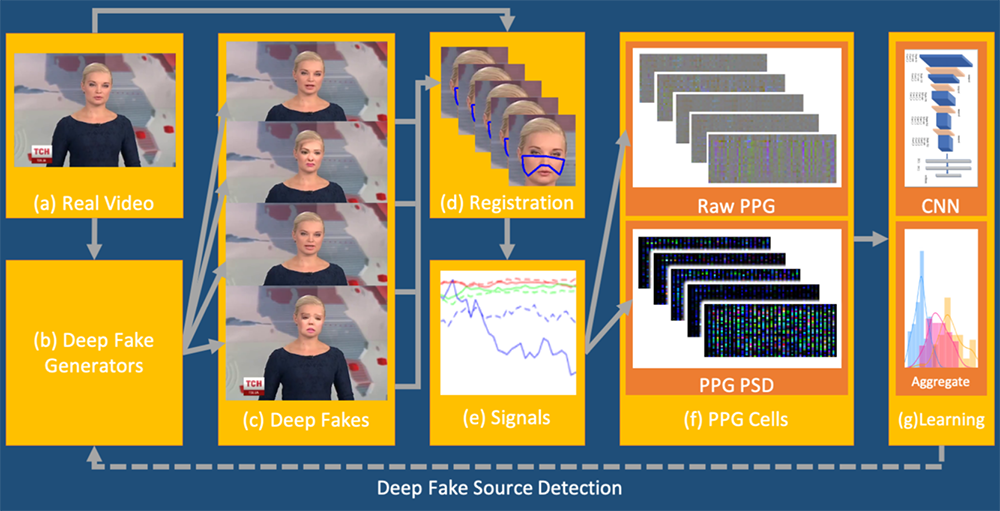
▲Deepfake detection process
Conclusion: Collaboration Between Industry and Academia, Aiming for Benchmark Dataset
A large number of forged videos and images are widely disseminated on the internet and social media, so designing benchmark datasets for deep detection research is increasingly urgent. Researchers stated that in the next phase, they will create a new dataset containing PPG signals to take a step further toward this goal.
Yin also expressed hope for continued collaboration with Intel in the future, allowing research results to not only have an impact in academia but also be practically applied in the industry.
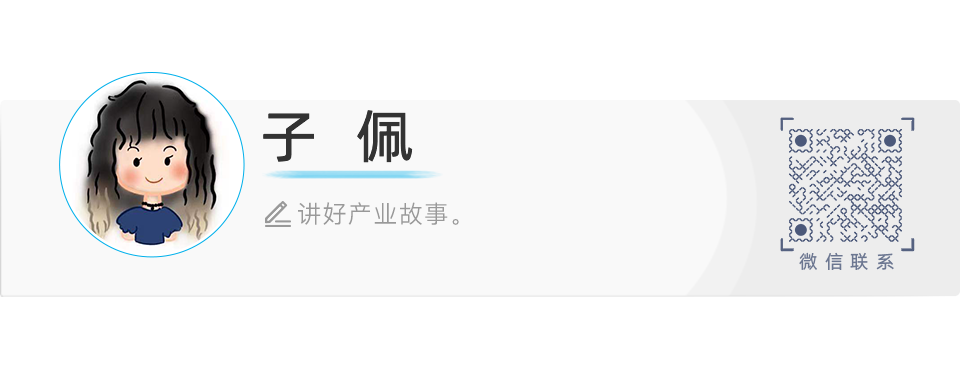
(This article is original content from NetEase News • NetEase account feature content incentive program signed account [ZhDongxi], unauthorized reproduction is prohibited.)
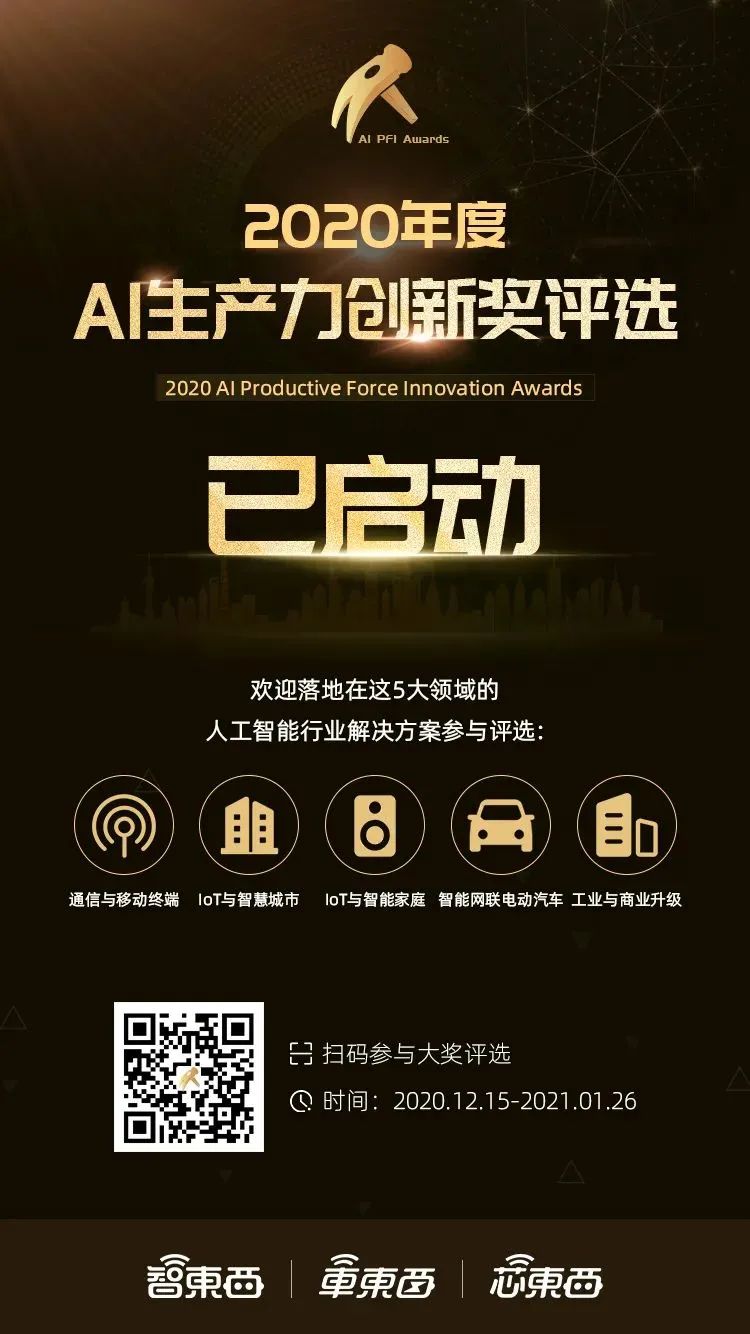
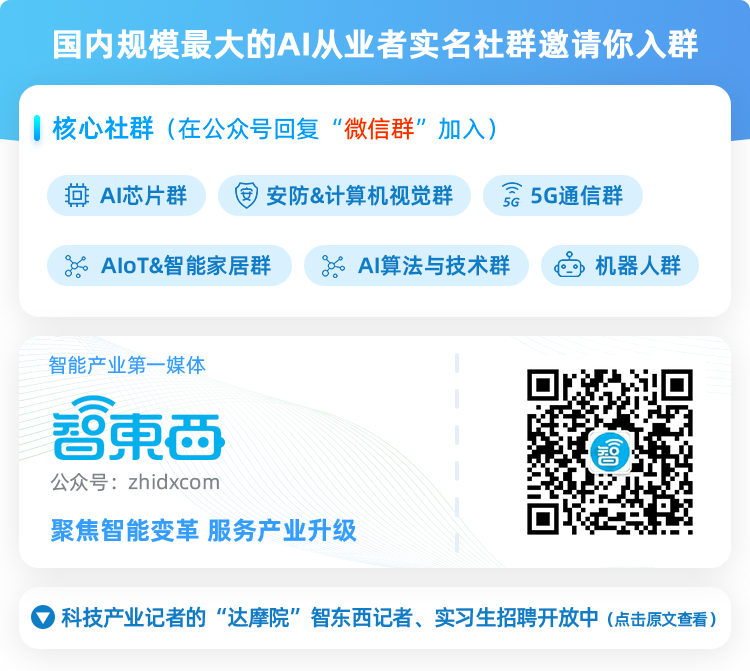
