In April 2024, Academician Zhang Bo of the Chinese Academy of Sciences, Professor of Computer Science at Tsinghua University, and Honorary Dean of the Tsinghua University Artificial Intelligence Research Institute gave a lecture titled “Entering the ‘No-Man’s Land’: Exploring the Path of Artificial Intelligence” at Tsinghua University’s “Humanities Tsinghua Forum”.
Academician Zhang elaborated on the two paths of artificial intelligence, the three stages of AI development, the insecurities of deep learning, the four steps towards general artificial intelligence, and the three major avenues for foundational models.
He believes that the success of current AI tools primarily stems from two “big” factors: the big model and the big text. Transitioning from large language models to general artificial intelligence requires four steps. The first step is to interact with humans and align with human understanding; the second step is multimodal generation; the third step is interaction with the digital world, which manifests as AI agents; and the fourth step is interaction with the objective world, which reflects embodied intelligence.
He asserts that the goal of the first generation of AI is to make machines think like humans. The biggest issues with the second generation of AI are insecurity, untrustworthiness, uncontrollability, unreliability, and difficulty in promotion. Both the models and algorithms of the first and second generations of AI have many flaws. So far, artificial intelligence lacks a well-formed theory, relying more on models and algorithms. Therefore, it is essential to vigorously develop a scientifically complete theory of artificial intelligence, which will serve as the foundation for developing safe, controllable, trustworthy, reliable, and scalable AI technologies.
Although current AI technologies have improved efficiency and quality, the more informationized and intelligent the system becomes, the more insecure it is. He noted that the first generation of AI utilized three elements: knowledge, algorithms, and computing power, with knowledge being the most crucial. The second generation of AI mainly employed data, algorithms, and computing power. To overcome the inherent shortcomings of AI, the only way is to simultaneously apply these four elements: knowledge, data, algorithms, and computing power.
He believes that in the future, only a few jobs may be replaced by artificial intelligence. AI is about exploring the “no-man’s land,” and its allure lies in the fact that it is always on the journey. “We should not be overly optimistic due to its progress, nor should we be discouraged by its setbacks. What we need is persistent effort.”
The following is the full transcript of the lecture:
Entering the ‘No-Man’s Land’ Exploring the Path of Artificial Intelligence
Two Paths of Artificial Intelligence
To date, there is no unified understanding of “what intelligence is” worldwide. However, after years of exploration, artificial intelligence has indeed taken two paths. One path is behaviorism, and the other is internalism.
Among them, behaviorism advocates for simulating human intelligent behavior with machines. “Intelligence” and “intelligent behavior” are two entirely different concepts. “Intelligence” resides in our brains, and humans still know very little about it; “intelligent behavior” is the external manifestation of intelligence, which can be observed and simulated. Therefore, the goal of behaviorist AI is the similarity of machine behavior to human behavior, rather than the consistency of internal working principles. Currently, the mainstream of artificial intelligence is machine intelligence, which only shares behavioral similarities with human intelligence but is not entirely consistent. Internalism asserts that machines must simulate the working principles of the human brain, i.e., brain-like computation. These two schools explore artificial intelligence from different perspectives. The former suggests that aside from humans, machines or other methods can also forge a path to intelligence, while the latter argues that the path to intelligence can only rely on humans. At present, both approaches are still in the exploration stage.
Human exploration of the path of artificial intelligence began in 1956. A seminar on artificial intelligence was held in the United States, where ten experts from various fields such as mathematics, computer science, cognitive psychology, economics, and philosophy defined artificial intelligence after eight weeks of discussions. They advocated for creating a machine capable of thinking like a human through symbolic reasoning and representation. During this meeting, Newell and Simon demonstrated a program called “The Logic Theorist,” which proved some principles from Chapter 2 of mathematical principles, showing that machines can perform similar reasoning tasks. Ultimately, “artificial intelligence” was defined at this conference.
In 1978, Tsinghua University established the teaching and research group for artificial intelligence and intelligent control, which was China’s earliest teaching and research institution for artificial intelligence. Over thirty teachers participated in this group, most of whom came from the field of automatic control rather than artificial intelligence. In 1978, the group recruited its first master’s students, and in 1985, it began enrolling its first doctoral students, capable of conducting some teaching related to artificial intelligence, but research progress was limited. From 1982 to 1984, the group conducted surveys, visiting numerous research institutes and factories in Southwest and Northeast China. Based on their observations, the group determined that intelligent robotics would be the main research focus.
In 1985, Tsinghua University established the Intelligent Robotics Laboratory, and in 1986, the national “863” development plan was initiated, with intelligent robotics as one of its themes. Tsinghua University participated in the first “863” high-tech research project themed on intelligent robotics, serving as an expert unit in the committee from the first to the fourth session. By the fifth session, Tsinghua University became the leading unit for intelligent robotics research, and in 1997, it became the leading unit for space robotics research. The “Intelligent Technology and Systems” National Key Laboratory began construction in 1987 and was officially established in 1990.
Based on these efforts, relevant research was initiated. At that time, two theories were first established. One is the theory of problem-solving search space and the theory of granular computing, which had a significant impact internationally. In 2005, Tsinghua University initiated and organized the International Granular Computing Conference, which continues annually to this day. The second is the extensive early work done in artificial neural networks.
Three Stages of Artificial Intelligence
From 1956 to the present, the development of artificial intelligence can be divided into three stages: the first generation of artificial intelligence, the second generation of artificial intelligence, and the third generation of artificial intelligence.
The goal of the first generation of artificial intelligence is to make machines think like humans. Thinking refers to reasoning, decision-making, diagnosis, design, planning, creation, and learning. Whether in management or technical work, two abilities are required: one is to have rich knowledge and experience in a certain field, and the other is to possess strong reasoning ability. Reasoning is the ability to apply knowledge, or in other words, the ability to derive new conclusions and new knowledge from existing knowledge.
Based on this analysis, the founders of artificial intelligence proposed a “knowledge and experience-based reasoning model,” whose core idea is that to enable machine thinking, relevant knowledge must be input into the computer. For example, to enable a computer to diagnose patients like a doctor, one only needs to input the doctor’s knowledge and experience into the knowledge base and incorporate the reasoning process of the doctor into the reasoning mechanism, allowing the computer to perform machine diagnosis for patients. The core idea of this reasoning model is knowledge-driven, aiming to achieve machine thinking like humans through computational models. The biggest flaw of this model is the lack of self-learning ability, making it difficult to learn knowledge from the objective world, as all knowledge is derived from human input. Therefore, the first generation of artificial intelligence will never surpass humans.
The second generation of artificial intelligence emerged during the low tide of the first generation, primarily based on artificial neural networks. In 1943, the artificial neural network model was proposed, which mainly simulates the working principles of human brain neural networks. The main problem faced by the second generation of artificial intelligence is the transmission of perceptual knowledge. The first generation of artificial intelligence mainly operated under the guidance of symbolism, aiming to simulate human rational behavior. However, humans also engage in a significant amount of perceptual behavior, which must be simulated using artificial neural networks.
We often say that knowledge is the source of human wisdom and the foundation of rational behavior. This knowledge comes from education, mainly referring to rational knowledge, methods of analyzing problems, etc. However, perceptual knowledge is difficult to convey through language and cannot be acquired from books. Each person’s initial perceptual knowledge comes from their understanding of their mother. But when exactly does one begin to recognize their mother? How is this recognition achieved? These questions remain difficult to answer.
All perceptual knowledge is accumulated through continuous observation and listening. The deep learning of the second generation of artificial intelligence has inherited this method. For example, in the past, we primarily told computers the specific characteristics of horses, cows, and sheep through programming. Now, we create training samples from a vast number of photos of horses, cows, and sheep available online, allowing the computer to observe and learn. After learning, the remaining samples are used as test samples, achieving a recognition rate of over 95%. The process of observation and listening is conducted through artificial neural networks, treating the recognition problem as a classification problem and utilizing artificial neural networks for classification. The learning process through neural networks is called deep learning, which enables classification, prediction, and generation.
However, all data (images, voice, etc.) of the second generation of artificial intelligence comes from the objective world, and its recognition can only distinguish different objects without truly understanding them. Therefore, the biggest issues with the second generation of artificial intelligence are insecurity, untrustworthiness, uncontrollability, unreliability, and difficulty in promotion.
The fundamental idea of the third generation of artificial intelligence is the necessity of developing a theory of artificial intelligence. To date, there is no well-formed theory of artificial intelligence; it relies more on models and algorithms, and both the models and algorithms of the first and second generations of artificial intelligence have many flaws. Therefore, it is crucial to vigorously develop a scientifically complete theory of artificial intelligence, which will serve as the foundation for developing safe, controllable, trustworthy, reliable, and scalable AI technologies.
Regarding current AI technologies, while they have improved efficiency and quality, the more informationized and intelligent a system becomes, the more insecure it is. The first generation of AI utilized three elements: knowledge, algorithms, and computing power, with knowledge being the most crucial. The second generation of AI mainly used data, algorithms, and computing power. To overcome the inherent shortcomings of AI, the only way is to simultaneously apply all four elements: knowledge, data, algorithms, and computing power. Currently, widely used AI tools (large language models) can fully leverage these four elements. The Tsinghua University team proposed a three-space model for the third generation of artificial intelligence, connecting the entire perception and cognition system, providing excellent conditions for developing artificial intelligence theory.
Insecurities of Deep Learning
During their research, researchers discovered the insecurities of deep learning in artificial intelligence.
One typical case is: researchers created a comparison image of a snow mountain and a dog. Both the computer and humans could identify the snow mountain, but as soon as a little noise was added to the image, humans still recognized it as a snow mountain, while the computer misidentified it as a dog. This case illustrates that the current pattern recognition of artificial intelligence based on deep learning is entirely different from human vision. Although it can distinguish between a snow mountain and a dog like a human, it does not recognize either.
The key question here is—what is a dog? How should a dog be defined? Humans typically distinguish through vision, primarily looking at the dog’s shape, but what constitutes a dog’s shape? Dogs come in various forms and postures. Why can human vision identify a target as a dog amidst countless variations? This question remains unanswered to this day. Initially, when computers recognized dogs, they could not recognize them when their position changed; this was a problem of translational invariance, which has now been resolved.
However, many unresolved issues still exist. For example, computers can recognize dogs of fixed sizes, but they struggle to identify them when their size changes. Currently, computers can only differentiate between dogs and snow mountains using local textures. Therefore, if a texture on the snow mountain image is changed to fur texture, even if the shape of the snow mountain remains unchanged, the computer will still misidentify it as a dog. Thus, up to now, deep learning in artificial intelligence remains insufficiently safe and reliable.
The “Big Model” and “Big Text” of Large Language Models
Currently, the success of AI tools primarily stems from two “big” factors: the big model and the big text.
The first “big” model refers to large artificial neural networks, which can be used for classification and learning relationships within data, as well as for prediction. This enormous artificial neural network is called a “transformer.” The power of AI tools relies on the strength of deep neural networks. The original neural networks took word-by-word input, but now they can input over 2000 characters (one token roughly corresponds to one Chinese character) at once. Humans spent 56 years from 1957 to 2013 exploring the semantic representation of text. Now, text is represented not by symbols but by semantic vectors, marking a significant breakthrough.
In the past, computers processed text only as data; now they can treat it as knowledge, that is, vector representation. Additionally, “self-supervised learning” has been proposed. Previously, text for computer learning required preprocessing and prior labeling, which was too labor-intensive to support large-scale learning. Self-supervised learning means that original text can be learned by computers without any processing, predicting the next word based on the preceding text, and the predicted content becomes the next input, somewhat akin to a chain-style learning method.
The second “big” refers to big text. After computers achieve self-supervised learning, all text can be learned without any preprocessing, evolving from gigabyte-level to terabyte-level. Currently, relatively successful artificial intelligence has learned over 40 terabytes of data, equivalent to over ten million Oxford dictionaries, and this learning process is not rote but involves understanding the content. This marks our entry into the era of generative artificial intelligence. Both the first and second generations of artificial intelligence faced three limitations—specific models for specific tasks in specific domains. The “three specifics” define what is known as “narrow artificial intelligence,” or specialized AI.
Currently, successful AI tools can engage in conversations without domain restrictions due to their powerful language generation capabilities, representing a significant advancement in artificial intelligence. Moreover, the diversity of generated outputs is an important feature of current AI tools. The more diverse the outputs, the greater the potential for innovation, as diverse outputs make it difficult to ensure correctness; thus, the more we desire creativity, the more we must allow for errors. In our daily use of various AI tools, we find that sometimes AI responses are clever and insightful, while at other times they are clearly nonsensical, which is the result of diverse outputs.
Currently, AI tools have achieved two major breakthroughs: first, generating semantically coherent text similar to that of humans; second, enabling natural language dialogue between humans and machines across various domains. Large language models represent a step towards general artificial intelligence, and some Western experts believe this is the dawn of general artificial intelligence, but it is not general artificial intelligence itself, and the journey towards general artificial intelligence remains long and arduous.
Transitioning to General Artificial Intelligence Requires Meeting Three Conditions.
First, the system must be domain-independent. Currently, relatively successful AI tools have achieved domain independence in dialogue and natural language processing, but they still struggle with many other issues.
Second, the system must be task-independent, meaning it can perform any task. Currently, AI tools can engage in dialogue, perform arithmetic operations, compose poetry, write code, and handle various tasks, but they still find it challenging to complete complex tasks in complicated environments.
Third, a unified theory must be established. Therefore, artificial intelligence has a long way to go.
Four Steps Towards General Artificial Intelligence
Transitioning from large language models to general artificial intelligence requires four steps. The first step is to interact with humans and align with them; the second step is multimodal generation; the third step is interaction with the digital world; and the fourth step is interaction with the objective world. It is not to say that completing these four steps guarantees the achievement of general artificial intelligence, but rather that these four steps are at least necessary for the goal of general artificial intelligence.
The first step is alignment with humans. Currently, the content output by AI tools may not be correct. To address this issue, human assistance is needed to help AI tools align with human understanding. From the application practices of AI tools, their errors require human correction, and their error correction speed and iteration speed are quite rapid. Meanwhile, we must recognize that errors in output still exist, but if we want AI to be creative, we must allow it to make mistakes.
The second step is multimodal generation. It is now possible to generate images, sounds, videos, code, and other modalities using large models. As technology advances, distinguishing whether content is generated by machines or humans will become increasingly difficult, providing excellent opportunities for “fakes.” “Fakes,” also known as “deep fakes,” are created using deep learning methods. Imagine if 95% of the text on the internet were generated by AI in the future; how could we still obtain true knowledge and facts from the internet? For instance, after an event occurs, if a wave of supporting or opposing opinions floods the internet, how can we tell if these opinions genuinely represent the majority or if they are manipulated by a few using AI to distort the facts? How to effectively prevent AI tools from manipulating public opinion and obscuring the truth is something we need to consider seriously.
Currently, three breakthroughs have been achieved in the field of artificial intelligence: generating semantically coherent text similar to that of humans across various domains. Among them, semantic coherence is the most crucial breakthrough; following this, breakthroughs in images have emerged. Since images only require spatial coherence, while videos demand temporal coherence, we will see breakthroughs in images following breakthroughs in language, and subsequently in videos. Throughout this development process, the demands for computational resources and hardware will increase significantly.
As artificial intelligence develops, many have noticed the phenomenon of “emergence.” For instance, when the system scale does not reach a certain level, the generated images are poor and of low quality. However, once the scale reaches a certain threshold, the quality of most generated images suddenly improves. This process is termed “emergence,” which is the transition from quantitative change to qualitative change. To date, the reasons for the emergence phenomenon remain incompletely understood worldwide.
The third step involves AI agents. Large language models must connect with the digital world to transition toward general artificial intelligence, initially operating in the digital world to solve problems, perceive the quality of their outcomes, and provide feedback. This work greatly benefits the advancement of large model performance.
The fourth step involves embodied intelligence. Embodied intelligence refers to intelligence that possesses a body. Intelligence alone is insufficient; it must also have a body to act and interact. Therefore, large language models must connect with the objective world through robotics to transition toward general artificial intelligence.
Where is the Path for Foundational Models?
Currently, the information industry is developing rapidly, attributed to the establishment of relevant theories, which guide the creation of both hardware and software that are general-purpose. In the past, some large enterprises with global influence emerged in the information industry, applying and promoting relevant technologies to achieve informatization, resulting in rapid development of the entire chain.
However, the development of the artificial intelligence industry lacks theory; it consists only of algorithms and models, and the hardware and software built based on these algorithms and models are all specialized. “Specialized” implies a small market. To date, the artificial intelligence industry has not produced any large enterprises with global influence, so the AI industry must deeply integrate with vertical fields to have the potential for development. Nevertheless, the situation is changing; the emergence of foundational models with some general applicability will undoubtedly influence industry development.
In 2020, there were 40 unicorn enterprises in the global artificial intelligence industry valued over $1 billion. By 2022, this number increased to 117, and by early 2024, it reached 126. This trend indicates steady growth. Currently, there are 100 to 200 enterprises in China working on large models.
With so many people working on foundational models, what is their future path?
The first path is to transfer to various industries and create large models for different vertical fields. Many industries are considering this issue; for example, the oil industry is contemplating a large model for its field, and the financial industry is considering a financial large model. Thus, the number of general large models will decrease, while most people working on large models will shift toward various vertical fields.
The second path is the most important, which is to fine-tune applications in industries. In other words, providing open-source large model software for everyone to develop applications.
The third path involves integrating with other technologies to develop new industries. Many unicorn enterprises abroad have combined AI tools with other technologies to develop new industries, some transferring to various sectors, while others specialize in images, videos, voice, etc. Some domestic large models have also achieved considerable development.
Based on this, it is imperative to promote industrial transformation in the field of artificial intelligence. In the future, whether in hardware or software development, it is essential to integrate into the foundational model platform. In the past, software was developed in a zero-base computer, which was highly inefficient. Now, the platform has learned from over ten million Oxford dictionaries, achieving a capability level at least equivalent to that of a high school student. If the same work is carried out on the foundational model platform, it will yield better results with less effort, making this platform an unstoppable trend. These “high school students” come from the open platforms provided by large model enterprises.
Limitations of Large Models
All tasks performed by large models are externally driven, executed based on external prompts. They lack initiative; when doing something based on external prompts, they primarily rely on probabilistic prediction methods, leading to some disadvantages not present in humans, namely, the uncontrollability of output quality. Moreover, they do not discern right from wrong, making their outputs untrustworthy. Meanwhile, they are overly influenced by external factors and can only follow instructions to complete tasks. Humans, however, are entirely different; even when tasks are assigned by others, they can still operate under their conscious control, thus being controllable and trustworthy.
It is evident that current artificial intelligence does not understand its actions. AI tools cannot accurately distinguish right from wrong and still struggle to initiate self-iteration, remaining dependent on human intervention. In the future, artificial intelligence may serve as an assistant to humans, operating under human supervision, with only a few tasks fully entrusted to machines.
Research institutions have conducted statistics on the impact of artificial intelligence on various industries, listing numerous sectors where only a few jobs may be replaced by AI in the future. This indicates that while AI will significantly impact various industries, most of its role will be to assist humans in improving work quality and efficiency, rather than replacing human labor.
Artificial intelligence is about exploring the “no-man’s land,” and its charm lies in its perpetual journey. We should not be overly optimistic due to its progress, nor should we be discouraged by its setbacks. What we need is persistent effort.
END

● The wave of embodied intelligent robots is coming; another industry grand event is about to set sail
● Interview with Zhao Mingguo from Tsinghua University: Accelerating the evolution to replicate Boston Dynamics’ movements; what we need is confidence and innovation!
● The preliminary results of the 2023 National Science and Technology Award have been announced! 9 985 universities leading the pack! Xi’an Jiaotong University and Huazhong University of Science and Technology in the top three! (Full list attached)
● Unprecedented! American engineers collaborate with ChatGPT4 to design artificial intelligence chips
● Russian President Putin approves the new version of the “2030 National Strategy for Artificial Intelligence Development”
● A small town in Denmark with a population of less than 200,000! How did Odense become a global robotics center?
● The “commercialization year” has begun, and humanoid robots gain new players with strength
● Swiss researchers develop a new type of artificial muscle that is lighter, safer, and stronger!
● The EU halts Amazon’s acquisition of iRobot; where will the former vacuuming robot giant go?
● Top ten news stories in the robotics industry for 2023
● Muscle tissue-driven bipedal robots have emerged, marking a breakthrough in biohybrid robotics!
●Professionals discuss the robot-as-a-service model—the future of automation
● A comprehensive overview of China’s humanoid robot research teams
Under the heat of humanoid robots, a battle of advances and resistance
Who is the most eye-catching? Highlights from the 2023 mid-year reports of 53 listed robotics companies
53 listed robotics companies’ financial report for the first half of the year (with PDF attached)
Academician’s report|Pan Yunhe: The Behavioral Intelligence and Product Intelligence of Artificial Intelligence
Academician discusses new momentum for promoting collaborative intelligent manufacturing in robotics
Academician discusses six key technologies for innovative design in robotics
Westwood Technology expands new angles in humanoid robot research
Academician discusses the dual-driving model for future artificial intelligence development
Academician discusses how institutional intelligence brings “Transformers” from the screen into reality
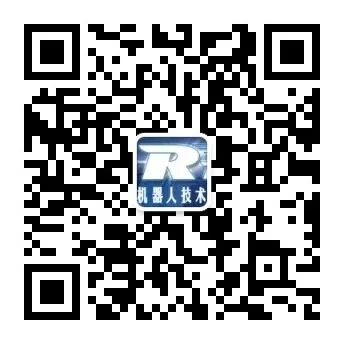