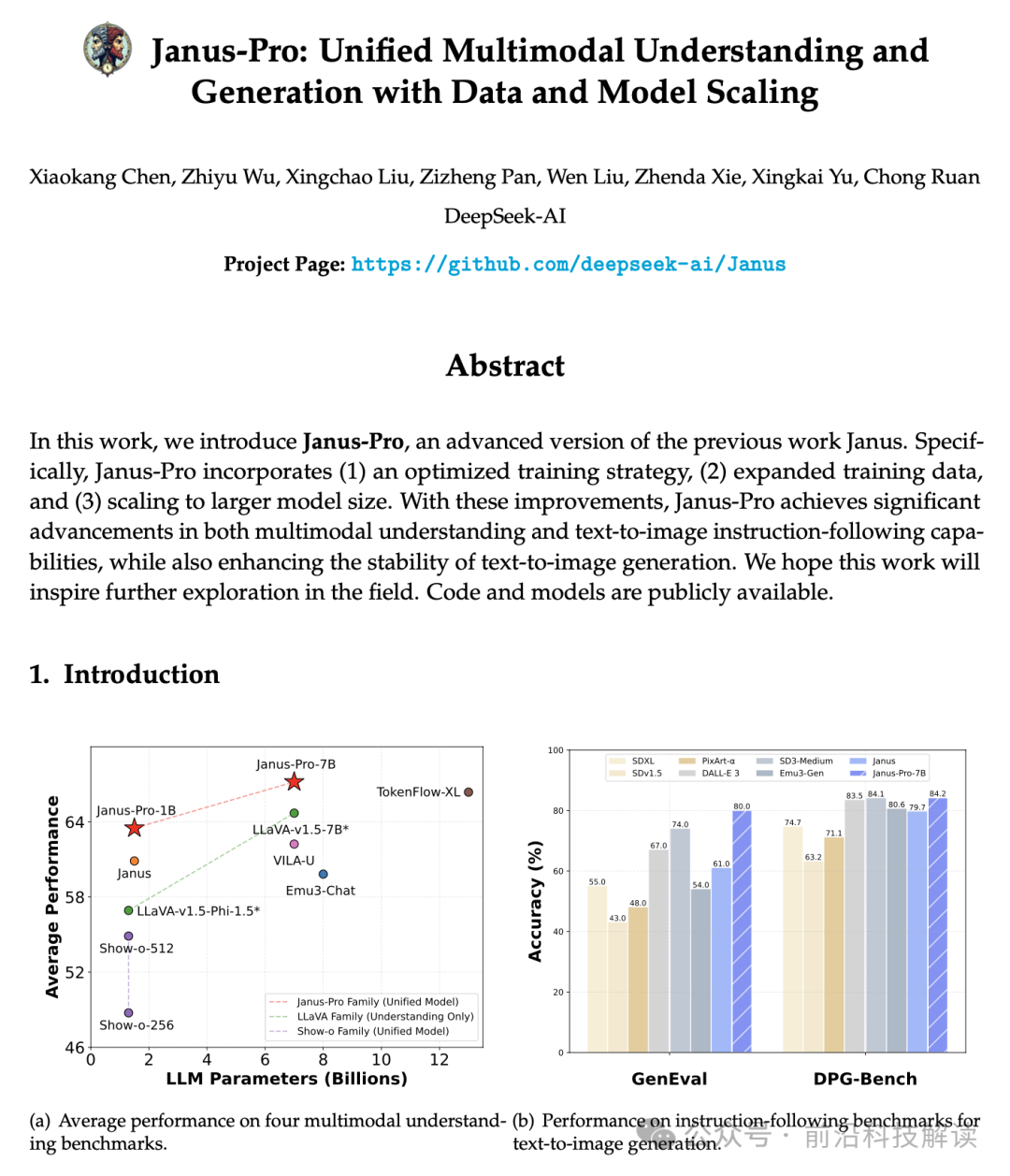
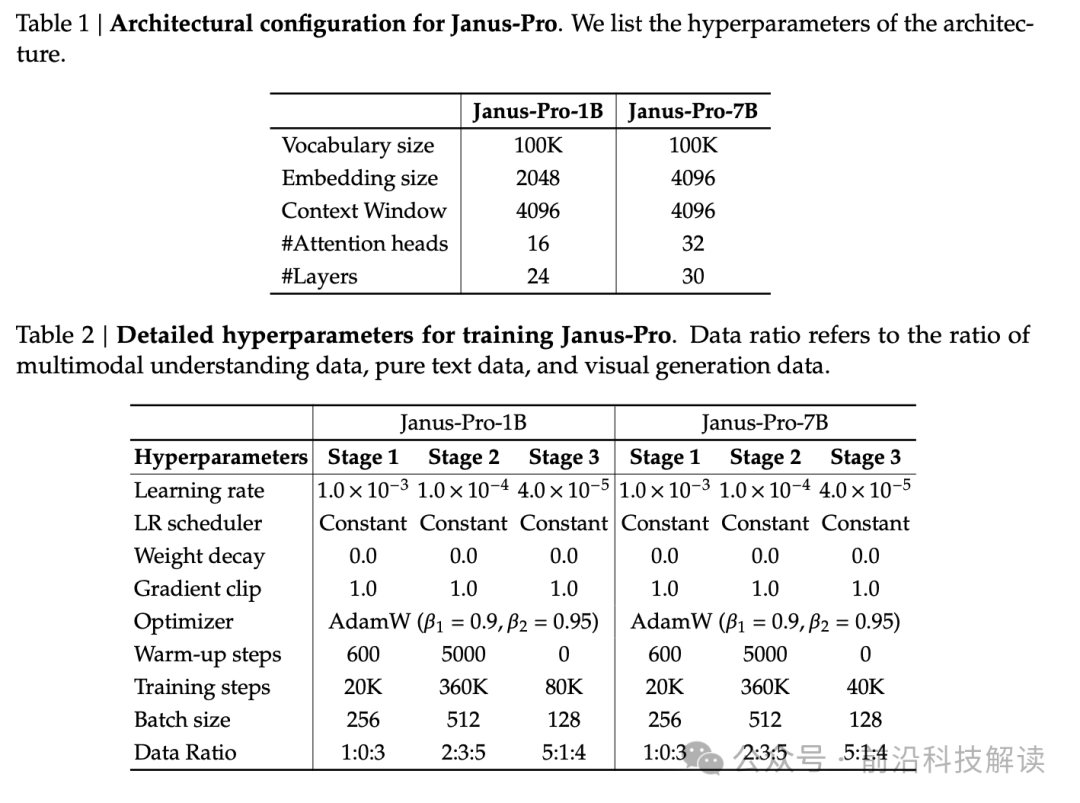
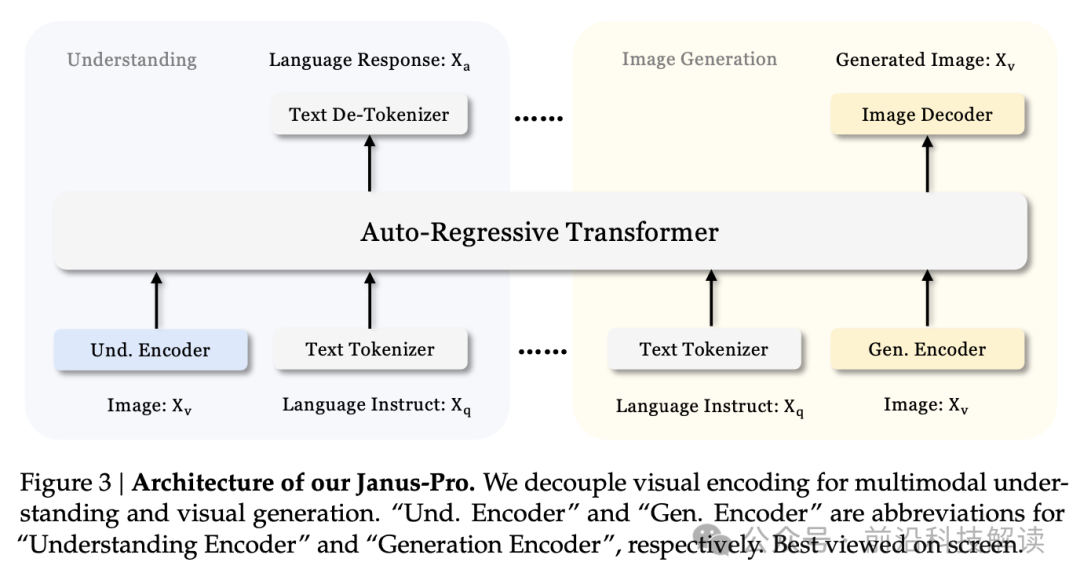
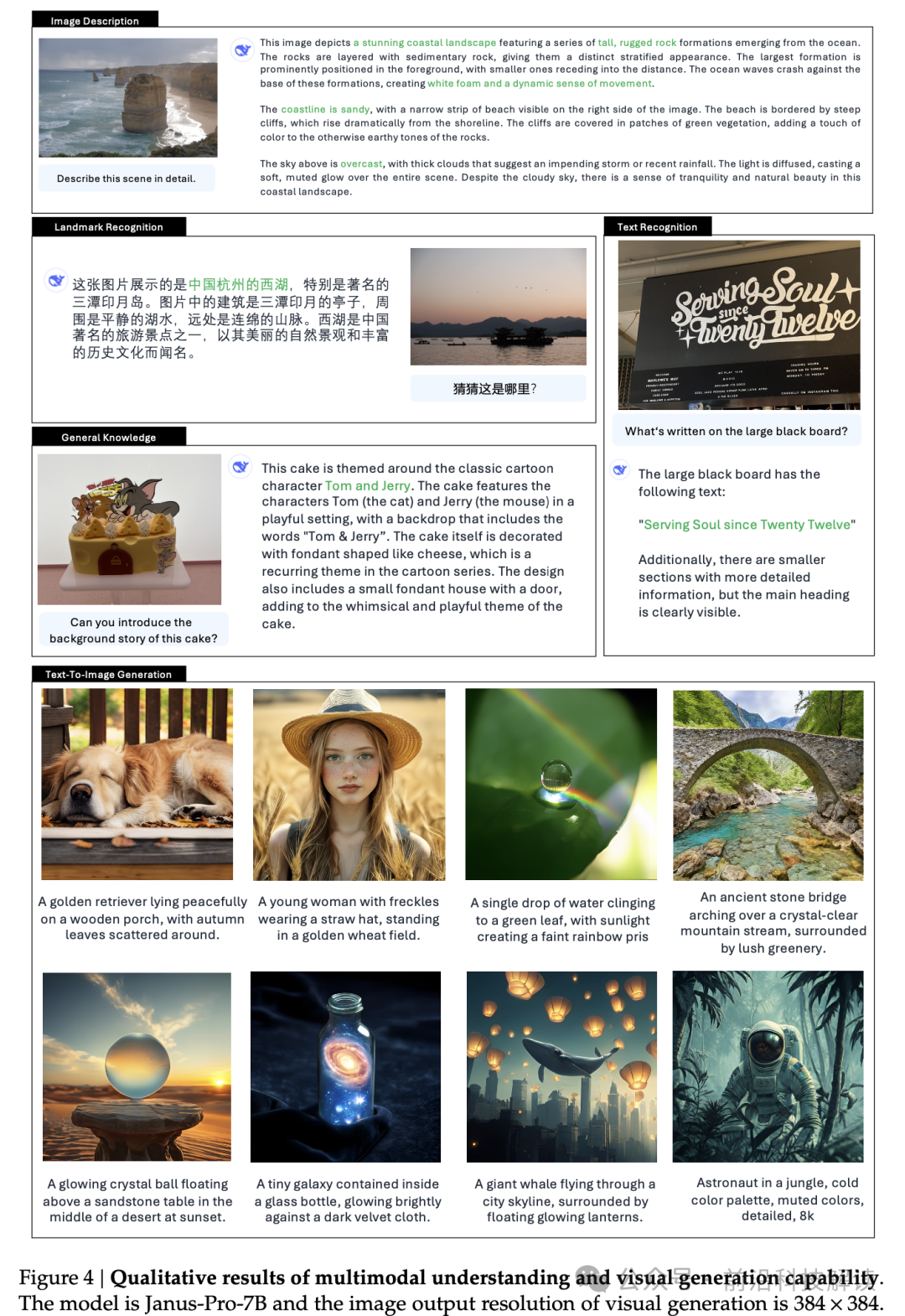
Janus-Pro is an advanced multimodal understanding and generation model developed by the DeepSeek-AI team, which is an upgraded version of the previous Janus model. Janus-Pro has improved in three aspects: optimized training strategies, expanded training data, and increased model scale. These improvements have enabled Janus-Pro to achieve significant progress in multimodal understanding and text-to-image instruction-following capabilities, while also enhancing the stability of text-to-image generation.
The Janus-Pro series includes model sizes of 1B and 7B, showcasing the scalability of the visual coding-decoding approach. In multimodal understanding benchmarks, Janus-Pro-7B scored 79.2 on MMBench, surpassing several state-of-the-art unified multimodal models, including Janus, TokenFlow, and MetaMorph. In the text-to-image instruction-following GenEval leaderboard, Janus-Pro-7B achieved a score of 0.80, outperforming Janus, DALL-E 3, and Stable Diffusion 3 Medium.
The architecture of Janus-Pro is the same as that of Janus, with the core design principle being the decoupling of visual encoding for multimodal understanding and generation. For multimodal understanding, a SigLIP encoder is used to extract high-dimensional semantic features from images; for visual generation tasks, a VQ tokenizer converts images into discrete IDs. These feature sequences are concatenated into multimodal feature sequences and then fed into the LLM for processing.
In terms of training strategy, Janus-Pro optimized the three-phase training process of Janus. In the first phase, the number of training steps was increased, allowing for thorough training on the ImageNet dataset. In the second phase, ImageNet data was abandoned, and normal text-to-image data was directly used to train the model, improving training efficiency and overall performance. Additionally, the data ratio of different types of datasets during the third phase of supervised fine-tuning was adjusted.
Regarding data expansion, Janus-Pro has expanded training data for both multimodal understanding and visual generation. For multimodal understanding, approximately 90 million samples were added, including image caption datasets and tabular, chart, and document understanding data. For visual generation, about 72 million samples of synthetic aesthetic data were introduced, achieving a 1:1 ratio of real to synthetic data.
In terms of model scale, Janus-Pro has scaled the model up to 7B, and compared to the 1.5B LLM, the convergence speed of loss for multimodal understanding and visual generation has significantly improved when using a larger LLM.
Experimental results indicate that Janus-Pro outperforms other unified models and models that only focus on understanding in multimodal understanding tasks. In visual generation, Janus-Pro also surpassed other unified or solely generative methods on GenEval and DPG-Bench, demonstrating its capability in following text-to-image instructions.
Despite the significant progress made by Janus-Pro, there are still some limitations. For instance, the input resolution is limited to 384×384, which affects performance in fine-grained tasks such as OCR. In text-to-image generation, due to the reconstruction loss introduced by the visual tokenizer, although the images are rich in semantic content, they still lack detail. Increasing the image resolution may alleviate these issues.