Skip to content
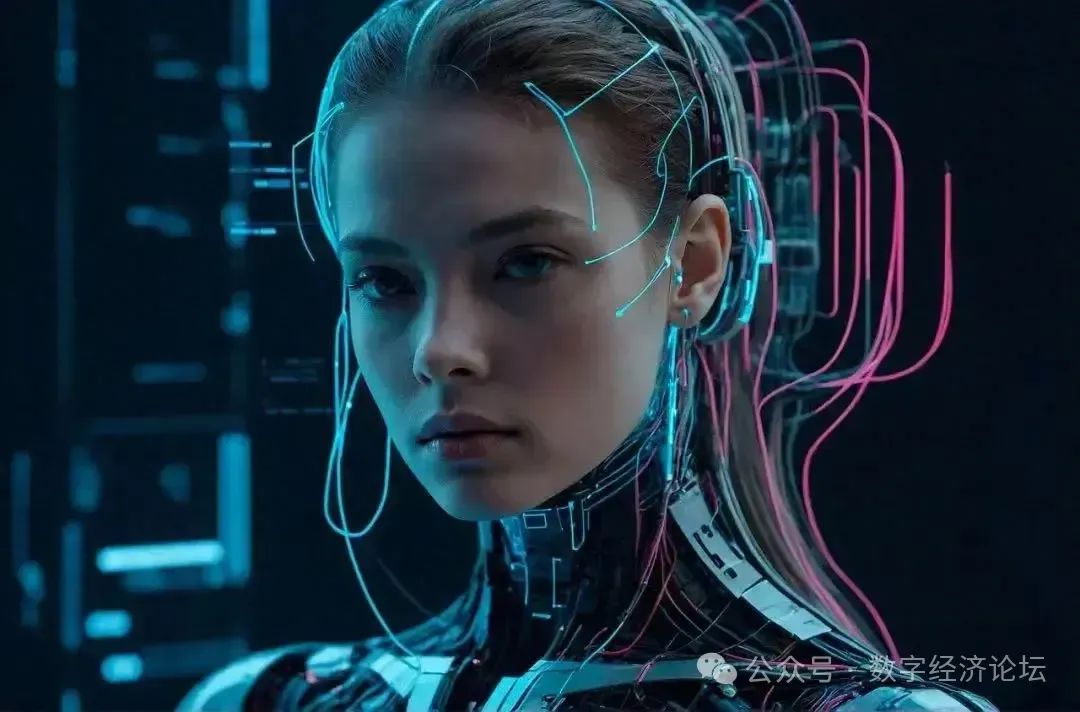
AI digital humans are virtual characters that use artificial intelligence technology to simulate the appearance, voice, movements, and expressions of real people. AI digital humans can interact with humans in various scenarios, providing services or entertainment. The technological development and application prospects of AI digital humans have attracted increasing attention and discussion. The following sections delve into the technical principles, progress, and prospects of AI digital humans.
Technical Composition of AI Digital Humans
The technical composition of AI digital humans mainly includes four aspects: anthropomorphic image, interaction ability, multi-terminal deployment capability, and decision-making ability.
The anthropomorphic image is the external representation of AI digital humans. It requires technologies such as computer graphics, computer vision, and speech synthesis to generate realistic images, movements, and sounds.
To create different virtual images, AI digital humans can use videos of real people or 3D models, or methods like Generative Adversarial Networks (GANs). GAN is a technique that uses two neural networks (a generator and a discriminator) to generate high-quality images from noise through adversarial training. There are many improved models of GAN that can achieve more diverse, stable, and controllable image generation, as well as cross-domain and unsupervised image generation. Examples include Conditional GANs, Variational Autoencoder GANs, Auxiliary Classifier GANs, and Cycle Consistent GANs.
To generate realistic movements and sounds, AI digital humans need to consider multiple factors such as posture, speed, rhythm, and pitch. Currently, technologies like motion capture and speech synthesis can generate smooth movements and sounds, but still have some flaws, such as monotonous movements, dull sounds, and lack of expressions.
To address these issues, researchers have proposed many deep learning-based methods for generating movements and sounds, enabling more natural, expressive, and adaptable generation of movements and sounds for different scenarios and styles. For example, motion generation based on Recurrent Neural Networks or Variational Autoencoders, and speech synthesis based on sequence-to-sequence models like WaveNet or Tacotron.
Interaction ability is the capability of AI digital humans to communicate and converse with users, requiring technologies such as natural language processing, speech recognition, image recognition, and sentiment analysis to achieve multimodal interaction, including voice, text, images, and video.
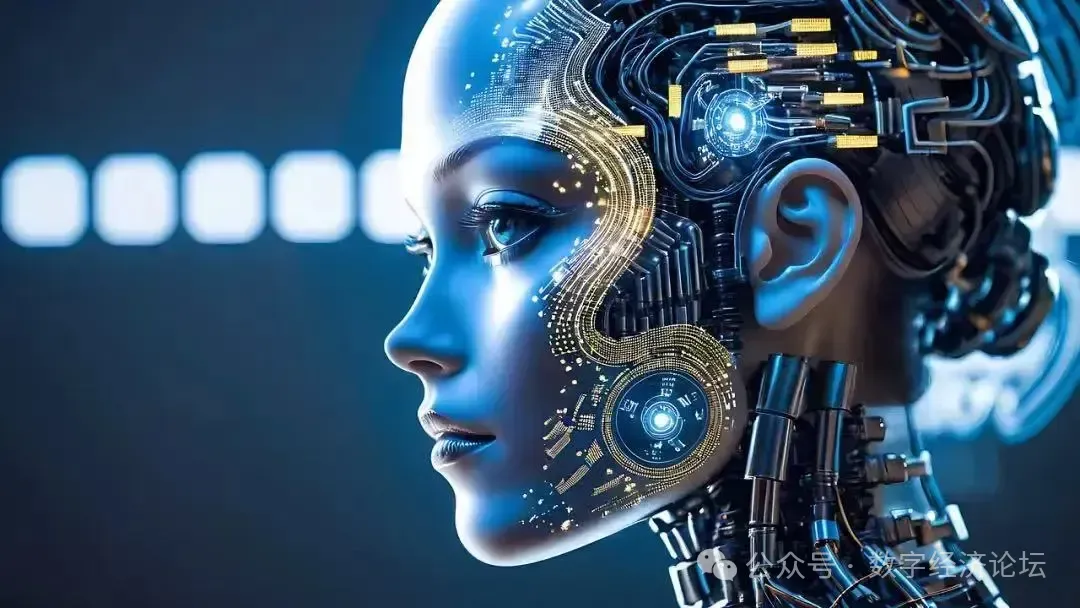
AI digital humans can generate appropriate responses and feedback by understanding user intentions and emotions, achieving human-like dialogue and communication. Natural language processing is a technique that uses computers to process natural languages (such as Chinese, English, etc.), including tasks like word segmentation, part-of-speech tagging, named entity recognition, syntactic analysis, and semantic analysis. Natural language processing plays a crucial role in interaction ability but also faces issues such as language ambiguity, lack of common knowledge, and difficulty capturing context.
To solve these problems, researchers have proposed many deep learning-based natural language processing methods, such as text classification based on Convolutional Neural Networks or Recurrent Neural Networks, machine translation based on attention mechanisms or transformers, and text understanding and generation based on pre-trained models like BERT or GPT. These methods can achieve more accurate, fluent, and versatile text processing, as well as cross-language and cross-modal text processing.
AI digital humans also need to understand user intentions and emotions, taking into account multiple factors such as goals, motivations, and attitudes. Currently, technologies for intent recognition and sentiment analysis can identify some basic intentions and emotions, but still face challenges such as ambiguous intentions, complex emotions, and emotional transitions.
To address these issues, researchers have proposed many deep learning-based methods for intent and sentiment recognition, such as intent recognition based on Bidirectional Long Short-Term Memory Networks or Gated Recurrent Units, and sentiment analysis based on Convolutional Neural Networks or attention mechanisms. These methods can achieve more fine-grained, dynamic, and multi-dimensional intent and sentiment recognition.
03/Multi-Terminal Deployment Capability
Multi-terminal deployment capability is the ability of AI digital humans to operate on different platforms and devices, requiring technologies such as cloud computing, edge computing, and streaming transmission to provide a high-definition and low-latency user experience.
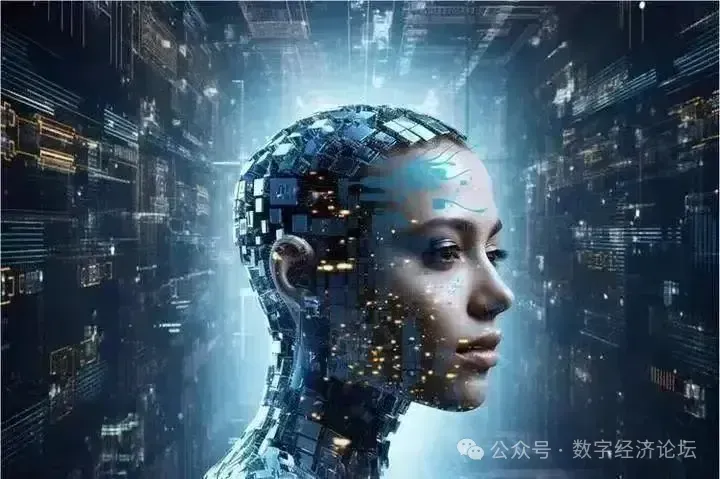
AI digital humans can provide a high-definition and low-latency user experience by optimizing rendering effects and network delays. Cloud computing is a technology that provides on-demand shared computing resources and services over the network, enabling large-scale parallel computing and storage. Cloud computing has significant advantages in multi-terminal deployment capability but also faces issues such as security risks, data isolation, and network dependency.
To address these issues, researchers have proposed many deep learning-based cloud computing optimization methods, such as resource allocation based on neural networks or tensor decomposition, data fusion based on attention mechanisms or graph neural networks, and network compression based on transformers or pre-trained models. These methods can achieve more efficient, secure, and stable cloud computing services, as well as cross-platform and cross-device cloud computing services.
AI digital humans also need to utilize technologies such as edge computing and streaming transmission to achieve high-quality user experiences in low-bandwidth and high-latency network environments. Edge computing is a technology that provides local computing resources and services at the network edge (such as routers or terminal devices), enabling faster, more flexible, and more reliable data processing. Streaming transmission is a technology that transmits continuous audio or video data over the network, enabling smoother, clearer, and more synchronized media playback.
Edge computing and streaming transmission play an important role in multi-terminal deployment capability but also face challenges such as resource constraints, load balancing, and synchronization guarantees. To address these issues, researchers have proposed many deep learning-based optimization methods for edge computing and streaming transmission, such as task migration based on Convolutional Neural Networks or Recurrent Neural Networks, load scheduling based on attention mechanisms or transformers, and synchronization control based on pre-trained models like BERT or GPT. These methods can achieve more efficient, balanced, and coordinated edge computing and streaming transmission services, as well as cross-scenario and cross-media edge computing and streaming transmission services.
04/Decision-Making Ability
Decision-making ability is the capability of digital humans to make reasonable judgments and choices based on different scenarios and tasks, requiring technologies such as knowledge graphs, machine learning, and reinforcement learning to enable real-time interaction and autonomous learning.
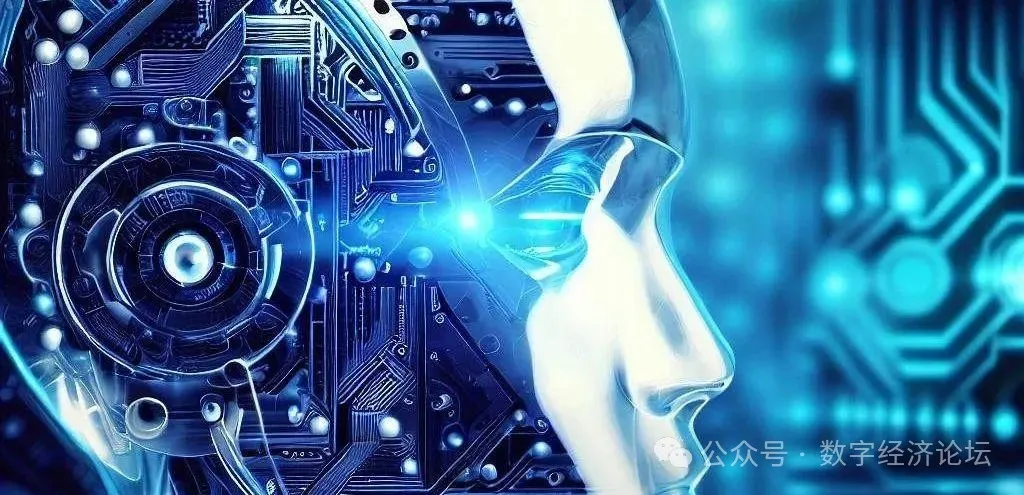
AI digital humans can achieve real-time interaction and autonomous learning by constructing knowledge systems. A knowledge graph is a technology that represents and stores large-scale structured and semi-structured knowledge using graph structures, enabling knowledge representation, reasoning, and querying. Knowledge graphs play an important role in decision-making ability but also face challenges such as knowledge acquisition, knowledge fusion, and knowledge quality.
To address these issues, researchers have proposed many deep learning-based methods for constructing and applying knowledge graphs, such as knowledge representation learning based on neural networks or tensor decomposition, knowledge reasoning based on attention mechanisms or graph neural networks, and knowledge question answering based on transformers or pre-trained models. These methods can achieve more effective, accurate, and flexible knowledge processing, as well as cross-domain and cross-modal knowledge processing.
AI digital humans also need to achieve autonomous learning and optimization through technologies such as machine learning and reinforcement learning. Machine learning is a technique that involves training and predicting models using data, including types such as supervised learning, unsupervised learning, and semi-supervised learning; reinforcement learning is a technique that involves learning and decision-making based on environmental feedback, including methods such as policy gradients, value functions, and deep reinforcement learning.
Machine learning and reinforcement learning play an important role in decision-making ability but also face challenges such as data sparsity, signal delay, and generalization ability.
To address these issues, researchers have proposed many deep learning-based methods for machine learning and reinforcement learning, such as feature extraction based on Convolutional Neural Networks or Recurrent Neural Networks, sequence modeling based on attention mechanisms or transformers, and transfer learning based on meta-learning or multi-task learning. These methods can achieve more efficient and adaptive model training and prediction, as well as cross-domain and cross-task model applications.
Trends and Directions of AI Digital Human Technology
As a cutting-edge technological product, the development direction and trends of AI digital humans are also worth paying attention to and anticipating. AI digital humans will gradually transition to a purely AI-driven stage, achieving a higher degree of intelligence and autonomy. Currently, AI digital humans still rely on human input and guidance, such as image design, corpus, and knowledge base; in the future, AI digital humans will be able to generate their own images, language, knowledge, and emotions through self-learning and creation, forming their own personality and style, and even developing their own consciousness and values. This will require more powerful computing capabilities, richer data resources, more advanced algorithm models, and more comprehensive evaluation mechanisms.
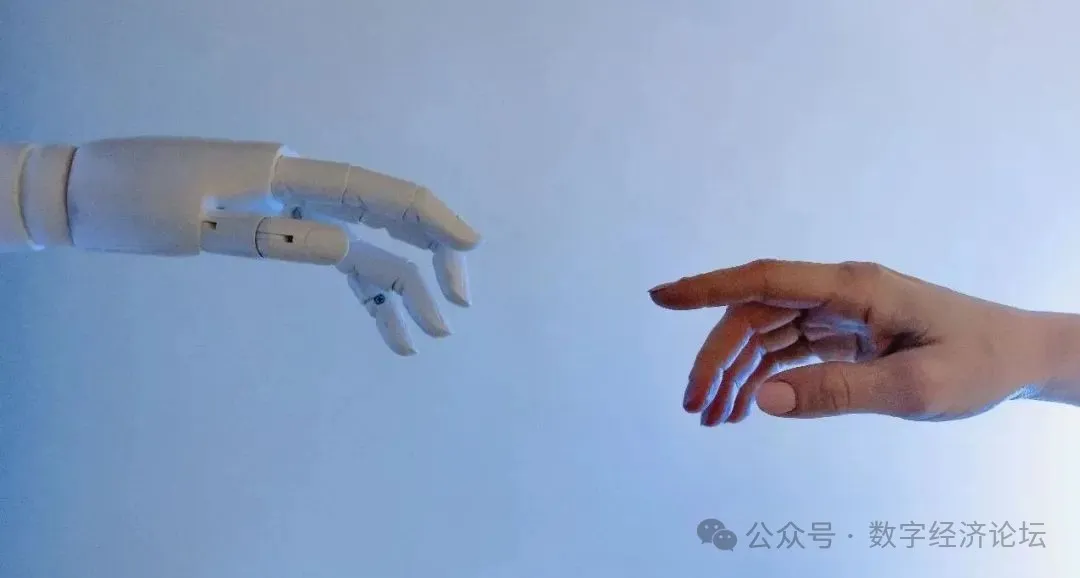
AI digital humans will demonstrate their commercial value and social influence in more application scenarios. Currently, AI digital humans are mainly applied in some vertical fields, such as finance, government affairs, culture and tourism, education, and entertainment. In the near future, AI digital humans will involve more horizontal fields, such as healthcare, law, consulting, media, and gaming. AI digital humans will not only be service providers or entertainment objects but also partners or social members, which will require more industry knowledge, more user needs, more scenario adaptability, and more social responsibility.
AI digital humans will connect more closely with the real world, forming new content output forms. Currently, AI digital humans are mainly presented on screens in the form of images or videos, isolated from the real world; in the future, AI digital humans will achieve integration and interaction with the real world through VR/AR technologies, providing users with a more immersive and realistic experience. AI digital humans will not only be a virtual presence but also a mixed reality, which will require higher rendering effects, lower network latency, better device compatibility, and stronger user engagement.
AI digital humans will form deeper interactions and symbiosis with humans, raising new ethical and legal issues. Currently, AI digital humans mainly interact with humans in the role of tools or toys, controlled and constrained by humans; in the future, AI digital humans will coexist with humans in the role of partners or companions, enjoying human rights and obligations. AI digital humans will not only be machines or dolls but also a form of life, which will require more trust and respect, more understanding and communication, and more norms and protections.
AI digital humans are virtual characters that utilize artificial intelligence technology to simulate the appearance, voice, movements, and expressions of real people, and they are also a technology product with enormous potential and influence, deserving our ongoing attention and exploration.
Source: Maijike Technology