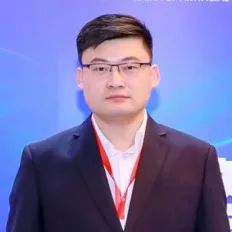
Peng Lv
Central South University
Director of Social Computing Research Center
Peking University
Deputy Director of Wuhan Artificial Intelligence Research Institute

Agent-Based Simulation: Advancing Research on Action-Structure Interconstruction
Source | Sociological Research, 2024, Issue 4
Author | Peng Lv
Editor | Yang Ke
The operation of social complex systems is a multi-level “action-structure” dual interconstruction process. Current theoretical research provides subjective interpretations of the relationship between action and structure, but often lacks objective process evidence; quantitative research can objectively reveal changes in states and trends, but it is difficult to collect direct data on the linkage between action and structure. Agent-Based Modeling (ABM) can integrate the flexibility of theoretical interpretation with the objectivity of quantitative research, studying action through mechanisms, “from action to structure” through emergence, and “from structure to action” through learning, thereby advancing dual interconstruction research. ABM expands the boundaries of sociological knowledge through fitting real results and counterfactual reasoning. ABM should be linked with theoretical research and quantitative research to jointly build a computational sociology methodological ecology, aiding the formation of academic communities.
The perspective of complex systems is one of the basic perspectives in social research. Systems theory posits that systems are composed of micro-elements, which interact, communicate, and share relationships; systems have relatively stable macro-structures and universality across natural and social realms. Society is also a complex dynamic system composed of numerous actors. Qian Xuesen et al. (1990) regarded society as a typical open complex mega-system. Luhmann (Niklas Luhmann) posits that the complexity of social systems is absolute and universal (Jiao Yaoguang, Lv Shouwei, 2007), and domestic scholars share similar views (Liang Yucheng, Jia Xiaoshuang, 2016; Luo Jiade et al., 2018). The perspective of complex systems expands the boundaries of social research. Coleman (1999) pointed out that explaining the activities of social systems is the core task of sociological research. Social phenomena and social governance can be viewed as optimization problems of the operation and control of social complex systems.
One of the important methods for studying social complex systems is simulation, which solves complex system problems in reality by constructing artificial dynamic systems. Luhmann pointed out early on that the complexity of social systems requires research tools to possess corresponding complexity (Ding Donghong, 2005). Qian Xuesen et al. (1990) also advocated adopting the method of “system simulation modeling” considering the complexity of social systems. The foundational work of simulation in the field of sociology is to construct “artificial societies” through system dynamics, cellular automata, and agent-based simulation. Zheng Zuoyu (2018) traced back to Simmel, arguing that simulation can reveal issues of “formal sociology”. In the era of computational sociology, the structural characteristics of social systems are increasingly revealed. Social simulation, as an important method for studying social systems, will play a greater role.
Agent-Based Modeling (ABM) is the latest stage of social simulation. The ABM method can be traced back to the life game in the 1970s. In the 1987 study of the “prisoner’s dilemma repeated game” led by Axelrod (Axelrod, 2017), ABM was in the era of cellular automata. Subsequently, Reynolds (1987) and others used simulation to study the evolution of life, marking ABM’s entry into the “artificial life” stage (Langton, 1998). The introduction of the sugarscape model in 1996 (Epstein & Axtell, 1996) marked the entry of social simulation into the ABM era. ABM, by constructing numerous agents, simulates the dynamic evolution of systems, revealing the “black box” process of how individual actions shape macro-society (Coleman, 1999). Currently, ABM has become an important paradigm in computational social science and has developed across multiple disciplines domestically, involving social psychology (Ye et al., 2021), group behavior (Lv Peng et al., 2021; Lv Peng, 2021; Wang Shuangyan et al., 2021), group decision-making (Nie et al., 2021; Wang et al., 2022), policy simulation (Chen Chan, 2021), urban planning (Ma Yan et al., 2019), public health (Xie Li et al., 2020), emergency evacuation (Yin Xuanpeng et al., 2020; Feng Jiahao et al., 2022), enterprise management (Zhao Xiaoli et al., 2013), and finance (Wang Yihan, Wang Guocheng, 2017), among other topics.
Reviewing the progress of ABM in the domestic sociological community, Sha Lianxiang et al. (2005) were among the first to use ABM to simulate public sentiment. Feng Zekun (2021) simulated the formation mechanism of social status. In 2021, Qiu Zeqi and Huang Shiman published an article in Sociological Research simulating the mechanism of innovation imitation in rural e-commerce entrepreneurship (Qiu Zeqi, Huang Shiman, 2021), which is representative in the application of ABM in sociological research. Currently, ABM is an “inescapable methodological issue” in social research (Qiao Tianyu, Qiu Zeqi, 2020). There have been reviews of the use of ABM (Liang Yucheng, Jia Xiaoshuang, 2016; Qiu Zeqi, 2022) in China, but they focus on reviewing related research, while this article emphasizes a systematic review of the ABM method itself. As a new method and new phenomenon, ABM has advantages in studying complex, systematic phenomena (Janssen, 2004). How to use ABM to help solve significant concerns and core issues in the discipline? Given that action and structure issues are among the core topics of sociology, this article focuses on action-structure interconstruction, examining how ABM analyzes and presents this interconstruction relationship in terms of ideas, logic, and methods, thereby enhancing the research capabilities to address the action-structure dual dilemma.
2. The Action-Structure Dual Dilemma in Social Research
Comte first proposed the topics of statics (structure and order) and dynamics (action and change). This topic runs through the entire process of sociological development. From a systems perspective, social complex systems exhibit duality in action and structure.
In social complex systems, social action is characterized by its micro and dynamic nature, with actors being the starting point of research. Parsons systematically discussed this. Social action includes four logical elements: actors, purposes, situations (conditions and means), and normative orientations. Specifically, an actor is an entity that can make decisions based on conditions, purposes, and contexts. Conditions are unchangeable by individuals, but means can be chosen. Actions are continuous and spontaneous, taking place under specific norms. Norms are broad structural constraints in society, linking actions with social order (Parsons, 2012). In terms of behavioral mechanisms, decision-making conditions, interaction processes, and learning evolution, methods such as questionnaires, case studies, and psychological analysis can study individuals but cannot achieve the unity of “presentation-analysis-validation”.
Social structure is the macro pattern of social complex systems, characterized by its macro and relatively static nature, representing stable social relationships. For example, Giddens (2016) posited that social structure is the structuring characteristic that binds social systems in time and space together. Smelser (Neil Smelser) summarized structure as stable relational patterns between different social categories, such as relationships between sectors, classes, and interest groups (Wang Sibin, 2021). Giddens argued that rules and resources combine to form social structure (Zheng Hangsheng, Zhao Wenlong, 2003). Scholars primarily use statistical surveys and data mining methods to characterize the structural characteristics of social complex systems.
Action generates structure, and structure in turn guides action (Tong Qingcai, 1980). The relationship between action and structure, as well as the relationship between micro and macro, has been one of the “most challenging old problems” in social research (Su Guoxun, 1996). Due to the long-standing lack of effective research methods for the action-structure interactive relationship, there has been a disconnect between micro and macro research, resulting in the action-structure dual dilemma (Zhao Dingxin, 2020). The academic community has been striving to address this dilemma, achieving some theoretical progress. Parsons is a theoretical synthesizer. He proposed structural functionalism and established theoretical links between social action and social structure. He integrated social entities at various levels through the AGIL model, forming social structure (Zhou Yi, 2000). Giddens proposed the theory of structuration, explaining the transformation relationship between macro structure and micro action (Giddens, 2016), theoretically opening up a channel for interconstruction. Moreover, Yang Fang (2010) also attempted to connect actors with social systems through the theory of modal variables. However, compared to theoretical progress, methodological development has always lagged behind. Coleman used the “boat model” to illustrate the bidirectional interaction between macro and micro, but did not specify methods (Hestrom, 2010). Quantitative methods and big data approaches can characterize groups and societies but struggle to collect direct data on how individual actions lead to changes in social structure. These two types of research often provide subjective statements based on changes in objective results, lacking direct support and independent verification from process and dynamic data.
Developing methods to analyze the processes of action and structure and promote research on the action-structure dual interconstruction is a long-standing internal demand in sociological research. ABM is increasingly adopted because it can simultaneously achieve the following three goals. First, it integrates micro-individuals and macro-society into the same system. The “artificial society” constructed by ABM is a multi-agent system (MAS) that can simultaneously present individuals and society, enhancing the overall integrity of the research. Second, it takes action as the starting point of research. The micro is the support of the macro, and action supports structure. ABM models based on social actors capture the logical starting point of action-structure interconstruction. Agents characterized by ABM possess perception, cognition, decision-making abilities, and adhere to social norms (structural factors). Through behavioral rule settings, ABM can greatly approximate and fit real individuals (Zheng Yaodong et al., 2022). Third, it directly presents the dual interactive process. Structuring is neither structure nor non-structure, but a dual interconstruction process. ABM can transcend theoretical analysis to directly model and showcase the process characteristics of structuring. Of course, empirical data verification can enhance the accuracy of the “artificial society” in terms of context and field. ABM mitigates the helplessness of modeling social processes (Qiao Tianyu, Qiu Zeqi, 2020), becoming an important tool for studying complex social processes.
3. The Intelligent Agent Simulation Framework for Studying the Action-Structure Dual Problem
(1) Induction of the Boat Theory on the Action-Structure Problem
Regarding the “action-structure” relationship, Western theoretical circles have formed two factions emphasizing individuals and structures. In bridging the relationship between the two, Parsons, Luhmann, Giddens, and Coleman have made contributions. Among them, Coleman’s “boat model” focuses on the transformation problem between micro and macro (Wu Wei, Shi Jinglin, 2022), summarizing the dual problem (see Figure 1). To explain the activities of social systems, Coleman (1999) divided his social rational choice theory into three parts: from macro to micro (from A to B), micro-level personal interactions (from B to C), and micro to macro transformation (from C to D). This theory takes micro action as the starting point of research, with macro social systems as the research objective, explaining purposeful social action through rationality. He studied how actions produce institutional structures, giving birth to social systems, achieving the connection from micro to macro (Qiu Haixiong, Zhang Yingxiang, 1998). ABM, through emergence (from C micro actions to D macro results) and learning (from A macro conditions to B micro actions), helps bridge the “Coleman boat”, facilitating research on action-structure dual interconstruction.
(2) The Emergence Function of ABM: Analyzing the Process from Action to Structure
“Emergence” refers to the results caused by micro individuals and local behaviors at the macro system level (Holland, 2006). Due to qualitative and quantitative methods struggling to handle the complexity of social systems, we should return to the explanation of individual behaviors. From the boat model in Figure 1, the path from C to D is often quite ambiguous (Qiao Tianyu, Qiu Zeqi, 2020), while ABM can clarify the relevant mechanisms based on emergence. Taking racial segregation as an example, this macro-level social phenomenon objectively exists, but survey data can only provide statistical characteristics, geographical distribution, and other information; if social structural factors such as culture and status are invoked, they do not enhance understanding of the micro behaviors behind racial segregation; even with household surveys, it is difficult to obtain individuals’ true psychological motivations within it. In short, traditional methods are “insufficient” on this topic, while Schelling (1971) constructed individual psychological thresholds revealing the micro-emergent mechanisms of racial segregation. Similarly, Epstein and Axtell (1996) developed the first social research ABM model (the sugarscape model) to simulate the emergence mechanisms of social resource (wealth) distribution, involving a series of actions such as individual death, reproduction, inheritance, transfer, transaction, and dissemination, to study social change and social systems.
(3) The Learning Function of ABM: Analyzing the Process from Structure to Action
The concept of “emergence” comes from systems science and complexity science, but it is insufficient to stop there. The “C micro action – D macro result” path in the “A macro condition – B micro action” chain highlights the emergence function of ABM, while the existing “A macro condition – B micro action” path needs further realization by social researchers. To this end, ABM can address this through its “learning” function. Learning reflects the process from structure to action, which is the internalization and feedback of knowledge by individuals under macro conditions, referred to by Parsons as the social reference structure (Tong Qingcai, 1980). Learning must incorporate three aspects of structural knowledge. First, historical memory. In the process of practice, historical memory serves as a trace of memory guiding individual behavior (Giddens, 2016). Second, social environment. Individuals acquire knowledge from the social environment, context, and structure to carry out autonomous actions. Bandura (2019) developed social learning theory based on this. Third, counterfactual results. Counterfactuals (anti-empirical) also hold research value (Pearl, Mackenzie, 2019), and ABM can realize counterfactual simulations to model individuals’ indexical, agentive, and reflective behaviors in daily life.
(4) ABM Simulating Dynamic Social Systems: A Continuous Interconstruction Process
In 2019, Professor Jon Elster of Columbia University published the book “Explaining Social Behavior: Mechanisms in the Social Sciences”, proposing that mechanisms are the core concept of social research (Elster, 2019). Mechanisms transcend causal explanations, becoming the core link connecting action and structure. ABM uses social mechanisms to reveal social processes and can depict complete single-instance interconstructions. In Figure 2, A (macro condition) and D (macro result) represent macro structural characteristics, while B (micro action) and C (micro action) represent social actors. The transition from B (micro action) to C (micro action) indicates individual behavioral interactions. C (micro action) achieves social system-level D (macro result) through emergence. D (macro result) has already occurred, constituting the a priori part of the next round of social system A’ (macro condition), and constraining and influencing individuals’ next round of learning, action, and interaction processes. Thus, the single-instance dual interconstruction analysis is completed. In fact, the operation of social systems is a continuously evolving process. ABM can not only depict single-round interconstructions (as shown in Figure 1) but also simulate multi-round interconstructions (as shown in Figure 2), making the Coleman boat “move”. The first-round macro results (D) and counterfactual results (D’) are both included in the macro conditions (A). If there are no significant changes (A’ = A), the social operation enters an “involution” loop mode (as shown in the left half of Figure 2). If major changes occur (A’ ≠ A), it enters a new interconstruction process mode (as shown in the right half of Figure 2), using the previous round’s emergent results as the next round’s macro conditions. This process can continue, allowing ABM to achieve continuous dual interconstruction process analysis.
(5) Three Stages of ABM Dual Interconstruction Research
ABM dual interconstruction research is divided into three stages, forming a three-tiered realm from shallow to deep (see Table 1). The first stage is the basic modeling realm, characterized by structural factors guiding model construction, with the task of establishing an “artificial society” to provide environmental and situational parameters for individual simulations. The second stage is the system emergence realm, characterized by social actions mapping social structures; its logical process includes conducting mechanism design to simulate individuals’ dynamic operations in the “artificial society”, collecting emergent social results at the system level, and accurately matching simulation and real social results to extract effective knowledge of real phenomena. The third stage is the intelligent learning realm, focusing on enhancing the research capabilities from structure to action. Social structure is both constructed by human actions and serves as the conditions and intermediaries producing human actions (Zhou Yi, 2000). ABM combines factual and counterfactual inferences to construct a social knowledge matrix, responding to and simulating the “duality of structure” perspective based on “structured practices of social knowledge”.
4. The Process Logic of ABM Researching Action-Structure Dual Interconstruction
(1) Defining Research Problems and Case Objects
The research problems of ABM have universal scientific orientations and pose requirements for the matching of methods. Research problems suitable for ABM should possess emergent, processual, and dynamic characteristics (Epstein, 1999), such as the mechanisms of cooperative behavior (Wang Shilong, 2017), group behavior evolution (Zheng Hao, 2021), etc. Moreover, real target cases suitable for ABM should meet at least three criteria. First, determinacy of boundaries. Cases can be completely extracted from real social fields without key omissions, ensuring the relative independence of behavioral mechanisms (avoiding, incorporating, or resolving externalities). Second, determinacy of outcomes. Simulation requires precise fitting of targets, and the results of real cases must be clear, measurable, quantifiable, and evaluable. Third, dynamism of processes. Real target cases represent social facts, exerting structural constraints, and thus should have clear and observable dynamic processes, such as raw data and historical data, to facilitate the verification of simulation results’ accuracy throughout the entire process.
(2) Strengthening the Theoretical Foundation of Social Simulation
Theory constitutes a form of precondition and structural constraint. ABM is not a “one-man show” of computer programming; it requires theoretical support. In the era of computational social science, Lv Peng et al. proposed a triangular methodological ecology of theory, (big) data, and simulation (Lu et al., 2023). Theory occupies the highest position, guiding the collection, processing, and analysis of data, big data, and empirical materials, as well as guiding ABM. One of the important tasks of ABM is to develop theory. First, it is necessary to develop classical theories of the discipline, including theories of social development, social change, social networks, urban-rural duality, and social environment, to guide the modeling of social environments in ABM. Second, new theories should be used and developed, including methods of computational social science, systems science theory (Ye Liguo, 2013), social physics, dissipative structure theory, etc., to guide dynamic modeling. Third, emphasis should be placed on exploring individual-level theories. Agents possess autonomy, interactivity, and adaptability, requiring guidance from individual-level theories (Bandini et al., 2009), including psychological, interpersonal interaction, human-machine interaction, and reinforcement learning theories, to enhance the anthropomorphism of actors.
(3) Characterizing Social Environments and Presenting Situations
ABM enhances the vividness of characterizing and presenting society. Social processes (events) occur within specific social environments, which serve as “containers” that carry individual behaviors and events, exerting total constraints. Using ABM to simulate specific social environments can be categorized into two types based on fidelity. The first is ideal-type environments, reflecting abstract, reductive, simplified analytical ideas, often used to study topological structures and essential issues. Such research has low fidelity to specific environments, as seen in social network studies (Hamill & Gilbert, 2009) and game theory research. Theoretical development based on ABM (Meng Qingguo, Luo Hang, 2017) also follows this idea. The second is high-fidelity environments, which build models that are fully or highly approximated to reality to simulate real societies. This idea is evident in various evolutionary dynamics studies, such as crowd dynamics research (Wang Shuangyan et al., 2021), traffic dynamics research (Shi Xueying et al., 2019), etc. Additionally, necessary environmental area settings refer to restrictions on individual and group action spaces, including impassable areas (walls, railings, etc.), passable areas (roads, squares, etc.), special areas (doors, stairs, etc.), and obstructive areas (tables, chairs, roadblocks, trees, etc.).
(4) Properties of Social Actors and Rule Design
ABM generates agents within specific social models. The number of agents is generally set using two approaches. One is single-PC simulation, using absolute setting (1:1 matching) or relative setting (proportional scaling). The general experience is that under conventional conditions (computer configuration of 32G memory, 8 cores, NetLogo 6.0.0), if the agent scale is below a thousand, absolute setting is used; otherwise, relative setting is employed. The second is large-scale simulation. With the use of supercomputers, large-scale social simulations based on digital twin cities have become possible (Makarov & Bakhtizin, 2018). If data is available, direct design methods can be used, setting heterogeneous individuals based on gender, age, occupation, social network, etc. If no data is available, indirect design methods can be used to approximate relevant variables, for example, in public safety (terrorism) simulations where individual life attributes are hard to measure, age variables can be used for approximation (Lu & Wenet al., 2021). In traffic simulations, herd behavior can be quantified into coefficients to study crowd evacuation (Li Changyu et al., 2016). Behavioral rules, as independent variables (X), are the mechanistic causes driving individual actions and producing macro emergence (Y). Normal behavioral modeling primarily employs basic physical rules, such as Brownian motion, social forces, fluid dynamics, etc. (Bain & Bartolo, 2019). In emergencies, crowd behavior patterns will change, requiring behavioral rules to be designed based on psychology, sociology, and behavioral sciences, and simulations of multiple rules can be refined using the “Occam’s razor” principle (Wei Zhixun, 2011).
(5) Obtaining System Emergent Data Based on Social Simulation
In addition to empirical data, the simulation (counterfactual) data generated by ABM will become a new type of material. First, let’s look at the relationship between emergence mechanisms and system results (Y variable). For example, in public safety simulations, the Y variable is the number of deaths or injuries, and the entire process result {Y1, Y2, Y3…YT} constitutes the observation of the simulation result function fsim(·). Next, we focus on the causal mechanism parameter traversal data (X variable). Actions have complexity, requiring multiple parameters to be set. Parameters are the X variables driving behavioral emergence. Considering the complexity and diversity of social results, ABM needs to simulate numerous combinations of parameter values. Assuming there are k X variable parameters {X1, X2…Xk}, corresponding to {n1, n2…nk} parameter values, there are a total of combinations of values (non-repeating). Each unique combination of parameters represents a (type of) simulation and a possible behavioral mechanism (hypothesis). Finally, we look at robust data for social outcomes (X-Y mapping). Each simulation can simultaneously obtain behavioral rules (X variables) and result data (Y variables) (Wang Feiyue, 2004). Due to random disturbances, multiple simulations need to be repeated. Lastly, parallel result data. By repeatedly simulating various combinations of X variable parameter values, the robust (average) results obtained constitute parallel simulation results (Bonabeau, 2002), which can be used to characterize and study the diversity, randomness, and complexity of social phenomena. Ultimately, X-Y mapping data can be constructed, which we can use for our research or for peer research verification.
(6) Fitting Social Empirical Data Based on Simulation Data
Social simulation cannot disregard facts and needs to use and fit empirical data. We define the best fit and optimal matching of a set of parameter values as the optimal solution, which is a global search problem of “1 to N”. “1” refers to real social events, which occur only once, represented by the target function freal(·). By traversing all parameter value combinations, N parallel simulation results are formed, i.e., fsim(·) data. We solve for the minimum difference between the simulation result fsim(·) and the target function freal(·). The confirmation of the optimal solution should be based on four criteria: First, validity. As the most basic requirement, simulation results should accurately fit reality (Grazzini & Richiardi, 2015), precisely hitting the “bullseye”. Second, robustness. This seeks stability and multiple hits; based on the optimal solution, repeated simulations examine the fitting degree of distribution characteristics. Third, full-process inversion (back-calculations). Based on real data, ABM can match and invert the “lifecycle” of real social events (Abar et al., 2017), thereby uncovering the real dynamic mechanisms. Fourth, interpretability. The behavioral mechanisms and social environmental parameters contained in the optimal solution should directly reflect the action-structure and dual interactions, thus being interpretable in social terms.
(7) Revealing Social Process Mechanisms Based on Empirical Data Fitting
Compared to statistical causal relationships, ABM reveals a causal relationship starting from social action, enhancing the capacity for causal research. First, discovering and confirming causal relationships. Through behavioral mechanisms (X), ABM can reproduce macro results (Y). The set of variable parameter values contained in the optimal solution provides a social process explanation of the causal mechanism. Additionally, behavioral spatial data can be reproduced and traced (using random seeds), enhancing repeatability. Second, falsifying and denying causality. All scientific theories (propositions) have boundary conditions and can be falsified (Popper, 2002). The academic community has recognized ABM’s role in discovering and verifying causal mechanisms (Cai Jingjing, 2016), but the significance of falsification is equally important and should not be overlooked. Causal propositions are usually expressed as “if behavior X occurs, then result Y will occur”. ABM implements falsification by examining whether there exists a situation of “if X, then not Y”. We establish the ABM model based on the original “X hypothesis”; if simulations find that the conditions of X do not (or do not necessarily) lead to Y, or that non-X conditions lead to Y, the causal mechanism should be questioned or denied. ABM possesses both confirming and falsifying effects, expanding the channels for causal research and providing an effective “touchstone” for causal mechanisms.
(8) Achieving Ubiquitous, High-Dimensional Social Counterfactual Inference
Counterfactuals are an inevitable demand for high-quality development in social research. In 2018, Pearl et al. proposed three levels of causal relationships, asserting that counterfactual inference is the highest level (Pearl, Mackenzie, 2019). ABM conducts social parallel inference through factual fitting and counterfactual inference. The counterfactual concept has been widely applied in sociology. For example, propensity score matching (PSM) is essentially a two-stage regression. PSM matches groups by calculating the probability of inclusion, thereby calculating differences in results (treatment effects) (Huanning, 2012), but it still has limitations: First, logical flaws. The “counterfactual individual” is merely a close match in probability of inclusion and is not the same individual in the case. Second, issues of rationality. The range of variables used for estimation in PSM is limited by data, interests, and costs, making it difficult to self-validate its rationality (Brand & Xie, 2010). Third, the dimensionality of state space is limited. PSM typically infers a single counterfactual state (Shao Yuxin et al., 2019). ABM can achieve counterfactual inference for any individual and any dimension, theoretically ensuring it is the same person. ABM counterfactual inference is based on facts, rather than discarding facts. “Facts” refer to the information (empirical data) collected and observed about target cases, as well as the social processes reproduced by the optimal solution. ABM can derive simulation results under various scenarios, conditions, and hypotheses through parameter generalization.
(9) Constructing the Action-Structure Interconstruction Based on the Social Knowledge Matrix
“Structuring” is a theoretical perspective for explaining the action-structure interconstruction process. Structure is constructed by behavior (action), which in turn constrains human behavior (Liu Shaojie, 2002). The social knowledge matrix is a core tool for ABM research on action-structure interconstruction. From the static attribute perspective, social knowledge refers to structural factors at the (social) system level, including theories, results, laws, principles, mappings, associations, etc., possessing macro and structural characteristics. In ABM, social theories, case information, dynamic mechanisms, and a priori results can all be viewed as sources of knowledge that guide individual actions. From the perspective of achieving ABM, social knowledge is not static but an evolutionary process; thus, it requires the simultaneous inclusion of facts and counterfactuals to construct the social knowledge matrix. ABM uses optimal solutions to fit and extract social factual knowledge, inferring counterfactuals through parameter generalization, systematically storing results from social parallel inferences. As a structural guide, the data structure of the social knowledge matrix should be a structural macro summary of “if X conditions, then Y results”. The X conditions include both real scenarios and counterfactual scenarios. Centered on the social knowledge matrix and its dynamic updates, ABM provides a scheme for studying the bidirectional interconstruction process of behavior and structure.
(10) Forming an Intelligent Agent Simulation Framework for Social Learning Algorithms
From the perspective of the social knowledge matrix, the dynamic evolution of social systems can proceed in three directions: involution, evolution, and degeneration. How to understand social involution? If the social system evolves while the social knowledge matrix remains unchanged (A’ = A), and there is no substantial update to the social knowledge matrix, individuals will act according to social traditions (old matrix) (as shown in the left half of Figure 2). If the social knowledge matrix changes (A’ ≠ A), there will be a necessity for updating, and individuals will need to learn new knowledge (as shown in the right half of Figure 2). A third change is social degeneration, where social outcomes worsen (A’ < A). However, people prefer to see social evolution, where social outcomes improve (A’ > A). The “social learning” algorithm based on ABM can guide individuals in learning based on past a priori knowledge and experience, simulating a continuous interconstruction process. Social evolution requires a comprehensive examination of both individual actions and macro structures. Actors (individuals, legal persons) play a key role; their actions are an indexical, reflective, evolutionary expression. ABM can clearly display individuals’ learning and can use social empirical data for verification.
The “social learning” algorithm is based on real social scenarios. The forms and sources of learning individuals engage in social life are diverse, including news reports, daily conversations, collective memories, emergency training, etc., all of which will form social knowledge in individuals’ minds. When individuals face similar situations next time, they will act according to the knowledge learned, resulting in new social outcomes. This is a continuous practical process. In previous research, Bandura’s “social learning theory” emphasizes the interaction among subjects, behaviors, and environments (Bandura, 2019), focusing on cognition and mentality, without proposing simulation ideas. Qiu Zeqi and Huang Shiman (2021) considered learning and imitation mechanisms, but their study focused on the “diffusion of innovation”, rather than the reflective, indexical, and evolutionary processes of individuals. The algorithm proposed by the author realizes the visualization, matrixing, automation, and anthropomorphization of individual learning, with Figure 3 detailing this logical process. Individuals possess perception, learning, and decision-making abilities. Individuals query, index, and call upon the corresponding values of the previous knowledge matrix K0 to determine action strategies and interaction strategies, taking social actions to simulate the process from structure to action. Meanwhile, researchers conduct statistics, learning, reflection, summarization, evaluation, and structuring of the action results at the social system level, dynamically updating the knowledge matrix to K1 (Lu et al., 2022), simulating the process from action to structure. This process continues, with individuals learning under the new matrix (K1), adjusting their behavior and interaction strategies, producing new outcomes, conducting statistics and calculations, and updating the matrix again (K2).
5. Exploring Social Research Instances of Action-Structure Dual Interconstruction
ABM is suitable for researching cross-level, cross-system, dynamic social system issues. This article uses the social safety event simulation research conducted by my research team as an example to illustrate how ABM aids research on action-structure dual interconstruction.
(1) The Necessity of Using ABM to Study Social Safety
Prior methods to ABM could only analyze and present simple social phenomena, and effective methods for researching complex social issues have not been found (Qiao Tianyu, Qiu Zeqi, 2020; Lv Peng, Chen Dianhan, 2023). For social safety events, current research primarily employs empirical data to study structural causes at the macro level. However, the limitation of this method lies in its difficulty in revealing individual action processes. The occurrence of social safety events has suddenness, urgency, and social harm, making it challenging to directly study them through social surveys, social experiments, or big data analysis. Furthermore, events occur only once, making it hard to verify research conclusions a second time. In fact, actors often timely learn from the last experience, adjusting their behavioral strategies when encountering social safety events next time. Relying solely on empirical data and social facts cannot reveal the agency, reflectivity, learnability, and evolution of actions; thus, ABM is needed to infer counterfactuals and conduct dual interconstruction research focused on social safety events, revealing the interactive processes between individual behaviors and social outcomes.
(2) Proposed Social Action-Structure Problems for Research
In social life, individuals often face a social dilemma: most people are passively avoiding during social safety events (such as terrorist attacks), with few instances of self-organized resistance. Game theory provides a theoretical perspective, using concepts of cooperation and selfishness to explain this. For individuals, although cooperative strategies (resistance) are crucial for society (Olson & Spelke, 2008), adopting selfish strategies (free-riding) is more common (Fischbacher & Gächter, 2010). Game theory analysis is abstract, structural, and macro-level, and cannot accurately depict the objective behaviors of individuals in crisis situations. The social dilemma problem possesses both action and structural characteristics, necessitating suitable methods to present and analyze the action-structure dual interconstruction process mechanisms. We need to find a social institutional design that addresses individuals’ concerns while enhancing social safety levels and ensuring the safety of those who stand up.
(3) Major Obstacles to Individual Resistance Mechanisms
Individual resistance requires two decision-making elements. (1) Necessity. Understanding that standing up is important is a prerequisite, which includes both proactive awareness (having endogenous, strong pro-social tendencies) and passive awareness (having to resist). ABM can model pro-social tendencies, psychological characteristics, learning processes, behavioral traits, and decision functions through agent settings (Lu et al., 2022), simulating field risk levels. (2) Effectiveness. Knowing that it is important is not enough; individuals may not act because they also do not know whether resistance is effective. One person’s resistance likely leads to death; it is the collaboration of more individuals that increases the odds of success. So, how many people need to resist to be effective? Emergency drills, social experiments, and other methods have participant effects, making it necessary to use ABM to infer counterfactual results. The author’s team simulated the 2014 terrorist attack in Pakistan, preliminarily inferring the effectiveness range of resistors subduing perpetrators (Lu & Yang et al., 2021). In this case, with 0 people resisting, 18 died. The counterfactual results (Figure 4) indicate that if 1 person resists, 17.66 will die. This suggests that if someone resists alone, not only is that person in danger, but the objective gain is limited. This aligns with the majority’s perception of “the nail that sticks out gets hammered down”, leading people to choose not to resist (free-riding). This further deteriorates the social safety situation, allowing perpetrators to attack everyone at will. ABM can also infer broader scenarios. If 2 people resist, 7.06 will die; if 3 people resist, 1.2 will die; if 4 people resist, no one will die. Thus, ABM has provided specific effective boundaries (3-4 people). If individuals know the effectiveness boundary, they will adjust their behavior, leading to different outcomes.
(4) Extracting Social Factual Knowledge Based on ABM Simulation
On March 15, 2019, a terrorist attack occurred in New Zealand where a gunman entered a mosque with about 190 people, slaughtering civilians. After the first round of shooting, the gunman calmly returned to his car to reload before returning for a second round of shooting, during which one person resisted. We constructed a basic simulation environment for the mosque based on Google Maps, including 5 prayer rooms and interior passages. The mosque had 5 emergency exits, of which only 1 was open during the incident. In the external environment, we replicated the courtyard, roads, cars, parking lots, and other surrounding environments. Due to a lack of knowledge about the population characteristics on site, we set initial life health status values according to national population characteristics. In terms of on-site numbers, we set 190 civilian individuals and 1 perpetrator. At the same time, we set individuals to be harmed by the perpetrator’s gunfire within a certain distance, using social forces to simulate collisions and stampedes among individuals. In terms of crowd behavior, we simulated panic escape, competition for exits, crowding, and other abnormal patterns, with individuals falling injured also causing secondary harm. Based on individual psychology and pro-social preferences, we set anthropomorphic and social rules. Furthermore, we determined individual existence states (healthy, injured, fallen, dead, etc.) based on dynamic life values (blood levels). Specific behavioral mechanism designs are detailed in Table 2.
We statistically recorded the number of civilian deaths (Y1), injuries (Y2), deaths of resistors (Y3), and deaths of perpetrators (Y4) at the system level, using the real case results (civilian deaths Y1 = 44, civilian injuries Y2 = 35, resistor deaths Y3 = 1, perpetrator deaths Y4 = 0) as fitting targets, comprehensively solving for the optimal parameter solution across the four result variable dimensions (Y1 ~ Y4). Figure 5 shows that ABM’s multiple simulation results closely match real situations. The optimal solution contains a complete set of parameters (Table 2, third column) revealing the social laws of individual and group dynamics in crisis situations: life values follow a normal distribution (mean blood level 100 with a standard deviation of 20), perception radius of 3, average movement speed of 0.1-0.2, each collision causing 0.1 damage, and each trampling after falling causing 0.2 damage, when facing a perpetrator with indoor speed of 0.1-0.2, outdoor speed of 0.2, effective shooting range of 10, and each shot causing 54.5 damage, can reproduce real results. Meanwhile, the courageous resistor attacks the perpetrator bare-handed, causing 5 damage per hit, while the perpetrator has a 50% probability of prioritizing attacking him (with the remaining 50% probability attacking randomly).
(5) Generalizing Real Scenarios to Reveal Counterfactual Knowledge Based on ABM
ABM methods have opened up new paths for knowledge discovery. Knowledge can originate not only from empirical data mining but also from simulations and counterfactuals. Social knowledge encompasses both factual knowledge and counterfactual knowledge. Based on the factual knowledge represented by the optimal solution, ABM can also conduct counterfactual inferences to construct social knowledge regarding social safety. Social knowledge is an indexical structure that can be logically understood, internalized, and executed by individuals. The situational and result data presented by my research team in studying the Pakistan terrorist attack (see the left half of Figure 4) is merely a one-dimensional knowledge expression, which is difficult to serve high-dimensional simulation needs. We later simulated the 2012 shooting incident in a Colorado cinema, employing a more high-dimensional matrix form, systematically incorporating “scene-result” data types (Lu & Wenet al., 2021). Figure 6 specifically showcases the results of real scenarios and counterfactual scenarios. In Figure 6, A1 reflects the result of the real scene (perpetrator in the corner), while B2 (perpetrator centered), C2 (perpetrator at the exit), D2 (perpetrator randomly distributed), and E2 (occupying three corners) reflect various counterfactual scenarios’ death and injury results.
(6) Constructing a Social Knowledge Matrix for Public Safety
The social knowledge matrix used in the New Zealand terrorist attack study consists of a series of “condition-result” values. “Conditions” refer to physical environments, social contexts, the number of actors, behavioral mechanism parameters, etc. In this study, we kept other conditions constant, varying the numbers of perpetrators and resistors to calculate the survival and risk rates of the three subgroups of the public, perpetrators, and resistors under joint conditions, forming data units as “results”. The left side of Figure 7 displays factual results (1 perpetrator, 1 resistor) and simulated inferred counterfactual results (1-5 perpetrators, 1-10 resistors), using formula 2 to calculate survival rate levels (S1, S2, S3) and risk rate levels (100% minus survival rate). The social knowledge matrix facilitates the resolution of social dilemmas. On one hand, this matrix can accurately inform risk rate levels, addressing the “necessity problem”. Individuals and groups share the same (common) risk rate levels. As the attack progresses, everyone is pushed into a corner, and risk gradually increases, heightening the necessity for resistance. On the other hand, by examining the survival rates of perpetrators, the threshold interval for effectively resisting perpetrators can be calculated. Specifically, by using formula 3, we draw the effective boundary with the condition that the survival rate of perpetrators (the group) is below 50% (losing attack capacity).
(7) Optimizing Social Outcomes Based on Individual Social Learning
In the New Zealand terrorist attack, there was only 1 resistor, who ultimately died, and the resistance was ineffective, which reinforced the perception of “the nail that sticks out gets hammered down” (1 person resisting is ineffective). If everyone thinks this way, no one will stand up. We explore a counterfactual possibility: if individuals know the broader knowledge landscape, will they spontaneously resist and subdue the perpetrators? The social knowledge matrix aimed at public safety provides effective information. The left side of Figure 7 shows the inferred effectiveness boundary for the New Zealand terrorist attack: for 1 perpetrator, at least 3 people need to resist; for 2 perpetrators, 5 people need to resist; for 3 perpetrators, 6 people need to resist; for 4 perpetrators, 8 people need to resist. As the attack continues, the risk levels of each individual present are rising, and the necessity for resistance increases, making everyone a potential resistor. In the real case of an attack by 1 perpetrator, the effectiveness boundary is 3 resistors. This means that the resistor needs to mobilize 2 others besides themselves. In emergencies, it is essential for individuals (potential resistors) to conduct urgent communication and coordination, such as through calling, gestures, or physical actions. If feedback confirmation is received from the target, it counts as one mobilization. Next, we statistically record the number of mobilizations at the social system level, simulating the confrontation process and results, then conducting parallel simulations to infer counterfactual situations and calculate social outcome gains.
ABM can systematically assess the social gains brought by learning. Simulation results indicate that learning achieves individual optimization while enhancing the overall safety level of social groups. We use the real results without learning (1 exit) as baseline results to simulate counterfactual results under generalized scenarios, examining the results of both learning and non-learning (counterfactual) in five situations (opening 1-5 exits) (see the right side of Figure 8). The results show that learning mechanisms facilitate the emergence of resistance behaviors. In social life, without learning mechanisms, individuals will remain in a state of chaotic escape and passive beating for long periods, which is evident in social safety events both domestically and internationally. The memory traces of previous events and lessons learned constitute a priori knowledge; individuals will not remain in a state of chaotic escape forever but will learn, reflect, and draw lessons. Over time, terrorist attacks will be timely controlled at the grassroots level during their nascent stages, making society increasingly safe while achieving optimal outcomes for individuals and groups. This form of social optimization does not sacrifice the core interests of resistors, ensuring their life safety. Individual social learning is a process of dual continuous interconstruction and a driving force for social evolution.
6. Conclusion and Discussion
Based on the uniqueness of concepts, logic, and practice, ABM can empower social research in causal discovery (Sha Lianxiang, 2007), social prediction, and policy formulation (Sha Lianxiang et al., 2005), opening a “new paradigm rooted in pragmatism and complexity” (Qiao Tianyu, Qiu Zeqi, 2020). ABM has pushed the research of action-structure interconstruction to a new realm.
ABM has initially achieved continuous interconstruction of action and structure, becoming an effective tool for discovering, verifying, and expanding knowledge. ABM can expand the field dimensions through counterfactual research, enhancing the utilization of research materials. ABM completes the tasks of “situating”, “emergence”, and “learning” through three steps, achieving interconstruction research. The unique research advantages of ABM manifest in four aspects. First, it expands the boundaries of research. ABM can simulate fine, dynamic, and complex social processes, revealing the entire process of individual and group actions. Second, it maximizes the use of empirical data. ABM infers scenarios and results that have not occurred in reality based on empirical data, helping us gain more information and knowledge. Third, it can present the continuous interconstruction process of action and structure. Individual behaviors are situational and indexical expressions. ABM enables individuals to engage in dynamic learning based on the social knowledge matrix, autonomously adjusting behaviors and engaging in social interactions. Fourth, it can be used to discover, formulate, feedback, and evaluate social policies, empowering social governance.
ABM should organically integrate with social research, enriching the toolbox of social research methods (see Figure 9). On one hand, ABM needs to link with theoretical research. The processing of theoretical research exists within the subjective consciousness of researchers, and research conclusions are influenced by researchers’ knowledge structures, research preferences, and research capabilities. The integration of ABM and theory has two directions. The first is “ABM-guided theoretical research”, where theory achieves processual and dynamic features through ABM, gaining confirmation, falsification, and development. The second is “theory-guided ABM research”. Bold hypotheses in theory can guide ABM modeling, while ABM has advantages in “careful verification”. On the other hand, ABM also needs to link with quantitative research (Zhou Wen, 2022), which also has two directions. The first is “ABM-guided quantitative research”. Quantitative research primarily uses static cross-sectional data (Bian Yanjie, Xiao Yang, 2014), and the interpretation of data is subjective, lacking support from dynamic process data; ABM can enhance visibility and process-oriented research. Quantitative research has long been troubled by repeatability issues (Wang Yang, Xiao Kun, 2020); ABM achieves repeatable verification, aiding in resolving this issue. Traditional data analysis can only reveal statistical causal relationships, while ABM can uncover causal mechanisms in behavioral terms and verify their effectiveness, robustness, and generalizability. The second direction for linking ABM and quantitative research is “quantitative-guided ABM research”. Empirical data can provide parameters, calibration, and verification for ABM (Zhou Wen, 2022), avoiding subjective issues in agent model settings, thus enhancing ABM’s robustness in factual reproduction and counterfactual inference.
ABM also has several limitations. First, it is challenging to ensure complete objectivity and completeness in setting agent attributes, and there is an issue of oversimplification in operational rules. Second, the verification methods of ABM are relatively singular; besides fitting real results, more methods need to be developed (He Zenghong, 2019). Third, the tension between the effectiveness and generalizability of ABM has yet to be resolved; the more effective ABM is for specific cases, the less its generalizability and universality. Fourth, current ABM primarily targets local spatial ranges and cannot yet face large-scale social simulations of real social scenarios. The shortcomings of ABM can be gradually resolved through interactions with theory and quantitative research. Domestic scholars have begun to integrate qualitative and quantitative methods for mixed research (Xu Jianping et al., 2019). Looking ahead, ABM should merge and interact with various methods to construct a new ecology of computational social science research methods.

Click the “Read Original” button below to download the full text