Recently, the fourth “Youth Scientist 50² Forum” was held at Southern University of Science and Technology, where Shen Xiangyang, an academician of the American National Academy of Engineering, gave a keynote speech titled “How Should We Think About Large Models in the Era of General Artificial Intelligence?” and provided his 10 insights on large models.
Here are the specific contents of his 10 insights:
1. Computing Power is a Barrier: The requirements for computing power for large models have been tremendous over the past 10 years. Today, to create an AI large model, it’s all about the cards; without cards, there’s no affection.
2. Data About Data: If GPT-5 comes out, it may reach a data volume of 200T. However, there aren’t that many good data sources on the internet; after cleaning, about 20T may be about the limit. Therefore, to create GPT-5 in the future, we need more multimodal data in addition to existing data, even artificially synthesized data.
3. The Next Chapter of Large Models: There is a lot of research to be done on multimodal systems. I believe a very important direction is the unification of multimodal understanding and generation.
4. Paradigm Shift in AI: After o1 came out, the approach shifted from the pre-training concept of GPT to today’s autonomous learning path, which involves reinforcement learning in the reasoning step, a self-learning process that resembles human problem-solving and analysis, and requires a lot of computing power.
5. Large Models Sweep Across Industries: In the wave of large model construction in China, more and more industry-specific large models are emerging. This trend is certain; the proportion of general large models will decrease in the future.
6. AI Agent, From Vision to Implementation: The super application has always been there; this super application is a super assistant, a super agent.
7. Open Source vs. Closed Source: I believe Meta’s Llama is not traditional open source; it only opens up a model without providing the original code and data. Therefore, when using open-source systems, we must be determined to truly understand the closed-source work of large models.
8. Focus on AI Governance: The impact of AI on various industries and society as a whole is significant, and we must face it together.
9. Rethink Human-Machine Relationships: Only by truly clarifying human-computer interaction can we become leaders with real commercial value in every generation of high-tech companies. It’s too early to say that OpenAI plus Microsoft represents this era; they are leading, but there is still much room for imagination in the future.
10. The Essence of Intelligence: Although large models have brought many shocks, we still lack a theoretical understanding of large models and deep learning. Discussions about the emergence of artificial intelligence are superficial, and we haven’t clarified them.
The “Youth Scientist 50² Forum” is an academic annual meeting organized by the New Cornerstone Science Foundation, Southern University of Science and Technology, and Tencent’s Sustainable Social Value Department. The New Cornerstone Science Foundation, funded by Tencent with 10 billion RMB over 10 years, is currently one of the largest public scientific foundations in China, embodying Tencent’s commitment to promoting technology for good and long-term investment in scientific funding.
The “Youth Scientist 50² Forum” serves as an interdisciplinary academic exchange platform for the winners of the “Scientific Exploration Award.” Established in 2018, the “Scientific Exploration Award” is a public award funded by the New Cornerstone Science Foundation and led by scientists, and is one of the highest monetary awards for young scientific talent in China. Each awardee shares their BIG IDEA and latest explorations at least once during the five years of funding. “50²” signifies that the 50 young scientists selected each year for the “Scientific Exploration Award” will have a significant impact on scientific and technological breakthroughs over the next 50 years.
Here is the full text of Shen Xiangyang’s speech at this forum:
I am very pleased to have the opportunity to share some recent learnings and insights in artificial intelligence with you all today in Shenzhen.
Following the topic of artificial intelligence presented by Mr. Yao Qizhi, I would like to report on some of the things we are currently doing in the era of large models, especially from the perspective of technological integration and industrial transition.
In fact, it is not just the importance of technological development in the era of artificial intelligence; the entire history of human development is a history of technological development. Without technology, there would be no GDP growth. We need not look back to the invention of the wheel or the discovery of fire—let’s look at the past 100 years for many remarkable breakthroughs in physics, and the past 70 years for breakthroughs in artificial intelligence and computer science, where we can see many opportunities for development.
Today, we are discussing artificial intelligence and large models. Over the past few years, everyone has been shocked step by step by new experiences in artificial intelligence. Even though I have been in the field of artificial intelligence for my entire life, I could hardly have imagined such a situation just a few years ago.
I want to discuss three examples: the first is text-to-text generation, the second is text-to-image generation, and the third is text-to-video generation. As mentioned earlier, ChatGPT is an artificial intelligence system that exists not only internationally but also domestically. For instance, before I came here to give a speech, I asked ChatGPT what topic I should discuss at the Tencent Youth Scientist 50² Forum, given my background. You might find it amusing, but I found it to be very helpful.
ChatGPT is quite familiar to everyone. Two years ago, OpenAI released a text-to-image generation system, which creates an image based on a given text. Seven months ago, it released Sora, which generates a 60-second video based on a given text, such as a video of walking through the streets of Tokyo. This is all very shocking. (Due to time constraints, I won’t play the video.)
Let me discuss the text-to-image generation example. I work in computer graphics and consider myself to have a good sense of what makes a photo good or bad. Two years ago, this photo emerged, which became the first AI-generated photo in human history to be featured on the cover of Cosmopolitan magazine. A digital artist in San Francisco used OpenAI’s system and provided a description, resulting in this outcome. The description was: “In the vast starry sky, an astronaut walks proudly on Mars, approaching a wide-angle lens.” I don’t have much artistic talent, but I was very shocked when I saw this image, and I believe you would agree with me that AI creating such an image is truly remarkable.
Today, with such remarkable technologies and products, we are also working hard on large models domestically, covering all aspects from technology to models to applications. As Mr. Yao mentioned, Tsinghua University has also done a lot of recent work. Therefore, I would like to share with you how we should think about large models in the era of general artificial intelligence. I want to discuss a few of my views.
First Insight: Computing Power is a Barrier
Today, general artificial intelligence, large models, and deep learning are fundamentally about the overall growth of computing power in artificial intelligence over recent years.
Over the past 10 years, the growth of computing power used in large models started with an annual increase of six to seven times, later exceeding four times a year. I have a question for everyone: if something grows four times a year, how much will it grow in 10 years? Think about it for a moment, and I’ll return to this question shortly.
As we all know, the biggest beneficiaries of this wave of artificial intelligence development have been NVIDIA. The company’s shipment volume has been increasing year by year, and its computing power has gradually strengthened, making it one of the three companies in the world with a market capitalization of over $3 trillion (alongside Microsoft and Apple). The key reason is the growing demand for computing power each year. In 2024, the number of NVIDIA chips purchased is still sharply increasing. For example, Elon Musk is currently building a cluster of 100,000 H100 cards, which is incredibly challenging to build a system with 10,000 cards, let alone 100,000 cards, which requires very high network standards.
When discussing computing power and large models, the most important thing is the scaling laws (scaling laws of computing power and data); the more computing power, the more intelligence grows. Currently, we have not yet reached the ceiling. Unfortunately, as the data volume increases, the growth of computing power is not linear; it resembles a quadratic growth.
Because as the model grows, to train the model, the amount of data must also increase significantly, making the growth appear more like a quadratic increase. Therefore, the requirements for computing power have been tremendous over the past 10 years. So I say, today, to create an AI large model, it’s all about the cards; without cards, there’s no affection.
I just asked everyone a question: if something grows four times a year, how much will it grow in 10 years? Those of us in computer science know about “Moore’s Law,” which states that computing power doubles approximately every 18 months. Intel has developed this way over the years. Why has NVIDIA now surpassed Intel? A key reason is the difference in growth speed. If it doubles every 18 months, it grows about 100 times in 10 years, which is remarkable; if it grows four times a year, it reaches 1,000,000 times in 10 years, which is astonishing. If you think about it this way, it’s understandable why NVIDIA’s market value has skyrocketed so quickly in the past 10 years.
Second Insight: Data About Data
Computing power, algorithms, and data are the three crucial factors for artificial intelligence. Earlier, I mentioned that we need a lot of data to train general artificial intelligence. When ChatGPT-3 was released, it was still in the paper-publishing stage, stating that it needed 2 trillion tokens of data; by the time GPT-4 came out, it was around 12T. With continuous training, GPT-4 is now estimated to have exceeded 20T. Those concerned with artificial intelligence have long awaited the release of GPT-5, but it has been delayed. I personally predict that if GPT-5 comes out, it may reach a data volume of 200T. Looking back, there aren’t that many good data sources on the internet; after cleaning, about 20T may be about the limit. Therefore, to create GPT-5 in the future, we need more multimodal data in addition to existing data, even artificially synthesized data.
An interesting point is that for the past 30 to 40 years, everyone has been sharing their information online. Previously, we thought we were working for search engines; now, more remarkably, our accumulation over these 30 to 40 years is for a moment like ChatGPT, which integrates everything together and learns this artificial intelligence model through powerful computing power, resulting in such an occurrence.
Third Insight: The Next Chapter of Large Models
Having reached this point, what should we do next? First, regarding language models. Represented by ChatGPT, its underlying technology is natural language processing. Today, we are working on multimodal models, represented by GPT-4, which incorporates many techniques from computer vision. Moving forward, we need to develop embodied intelligence. What is the purpose of embodied intelligence? In fact, we need to build a world model. Even for multimodal systems, the underlying physical model is absent, so we need to create such a world model. A world model means that you must not only read thousands of books but also travel thousands of miles, feeding more knowledge from the world back into your brain. Therefore, we should focus on robotics. I believe Shenzhen should be determined to develop robotics and embodied intelligence. Within robotics, there is a specific track called autonomous driving, which is a particular type of robot, only it drives along designated routes.
How should we proceed? There is much research work to be done on multimodal systems. I believe a very important direction is the unification of multimodal understanding and generation. Even if Sora is developed, it remains separate; multimodal generation and understanding have not been unified. There is a lot of scientific research work we can do in this regard.
For example, several of my students have founded a large model company called Jueyue Xingchen, and they excel in multimodal understanding. If you show AI an image, it can explain why the behavior depicted in the image is called “ineffective skill”. AI explains that the image seems to show a child rolling on the ground while their mother remains indifferent, engrossed in her phone and drinking, hence the child’s skill is dubbed ineffective. AI is now getting better at understanding images.
Fourth Insight: Paradigm Shift in AI
Two weeks ago, OpenAI released its latest model, o1. I previously mentioned that GPT has been developing, and after GPT-4, GPT-5 has yet to be released. People are wondering if merely increasing the parameters of large models has reached its peak. No one knows; it has not been released yet, and we haven’t developed any larger models domestically.
However, a new dimension has emerged, which is not about expanding pre-training but extending during reasoning. The approach has shifted from the original GPT concept to today’s autonomous learning path, which involves reinforcement learning during reasoning, a self-learning process.
Previously, we mainly did pre-training by predicting what the next word or token would be. Now, the new idea is to draft and test which path is correct, similar to how the human brain thinks, with a fast system and a slow system, akin to solving math problems by drafting and seeing which route is viable, creating a chain of thought, and optimizing opportunities during the thought process. So far, only OpenAI has released such a system, and I encourage everyone to look at some examples within it.
The most important aspect is that the entire process resembles how humans think and analyze problems—drafting, validating, correcting, and starting over. This thought space will be vast and requires a lot of computing power.
Fifth Insight: Large Models Sweep Across Industries
All companies must face the opportunities brought by large models, but not every company needs to create a general large model. If you don’t even have 10,000 cards, you won’t have the opportunity to create a general large model. To create a general large model, you need at least 10,000 cards.
For instance, when GPT-4 was released, its total training volume was 2×10^25 FLOPS. Such a massive training volume requires a year of running on 10,000 A100 cards to achieve. If this volume cannot be reached, there is no possibility of creating a true general large model. Once we have a general large model, we can build our industry-specific large models on that foundation, such as in finance or insurance, where perhaps 1,000 cards can perform very well with some fine-tuning. For a business, if you have your own data, internal data, and customer data, you can utilize dozens or hundreds of cards to create a very good model tailored to your enterprise. Thus, it is a layered build-up process.
Of course, there is also a very important dimension that I particularly like, which is the future of personal large models. Today, we are gradually accumulating data on PCs and smartphones, leading to a better understanding of us. In the future, I believe there will be a super-intelligent AI that helps you build a personal large model after collecting relevant data. This is a natural scenario on personal terminals, such as smartphones. On the PC side, companies like Microsoft and Lenovo are also promoting the concept of AI PCs, which presents such opportunities.
In the wave of large model construction in China, more and more industry-specific large models are emerging. For example, before large models can be launched in China, they require approval from the Cyberspace Administration. By the end of July this year, a total of 197 models had been approved, with 70% being industry-specific large models and 30% being general large models. This trend is certain; the proportion of general large models will continue to decrease in the future. For instance, we can build financial models on general large models, as a Shanghai-based company has done for its financial clients. For example, when NVIDIA’s financial report is released, it can immediately summarize its highlights and issues.
Sixth Insight: AI Agent, From Vision to Implementation
Today, we see what the largest super application of large models is and where the greatest opportunities lie. Many people are still trying to find a super application. In fact, the super application has always been there; it is a super assistant, a super agent.
In the past, I worked with Gates at Microsoft for many years, and we pondered this issue. What makes it difficult? The challenge lies in understanding a workflow when you need to do useful work. You ask a question, and it can break it down step by step. Today, there are already influential applications, such as customer service and personal assistants. However, many tasks are still impossible to accomplish. Why? Because creating a digital brain is necessary. The underlying large model is only the first step; its capabilities have not yet reached the point where it can help you complete these tasks step by step. To create such an agent that can perform tasks, it must understand what these problems are, and each part must have corresponding skills.
Today, many excellent examples have already been created using existing models. For instance, you can create an AI health consultant to discuss your understanding of cosmetics and recommend products. In the near future, we will see many applications in this area.
Seventh Insight: Open Source and Closed Source
Over the past few decades, the development of global technology, particularly in China, has hinged on two significant factors.
The first is the emergence of the internet, which allows you to find all papers and materials online.
The second is open source, which has dramatically narrowed the gap with leaders when developing applications. However, open sourcing is different from large models and database open sourcing. Although the capabilities of open source are now approaching those of closed source, many domestic companies are also working on open-source projects. Currently, Meta’s Llama 3.1 is claimed to be nearly on par with OpenAI. I do not share this belief; I think it is not traditional open source; it only releases a model without providing the original code and data. Therefore, when we use open-source systems, we must be determined to truly understand the closed-source work of large models.
Eighth Insight: Focus on AI Governance
The rapid development of AI has led to a global emphasis on AI safety. The impact of AI is substantial, and its influence on various industries and society as a whole is significant. The development of the world must be faced collectively.
Ninth Insight: Rethink Human-Machine Relationships
I have just introduced text-to-text, text-to-image, and text-to-video generation—how much of this is due to machine intelligence, and how much is the result of human-computer interaction that has shocked us?
About 10 years ago, New York Times columnist John Markoff wrote a book I greatly admire, “Machine of Loving Grace,” which summarizes two lines of technological development: one is artificial intelligence; the other is IA (Intelligent Augmentation), which enhances intelligence through human-computer interaction. With the advent of computers, they have aided humans in many tasks, chess being one example.
In fact, truly clarifying human-computer interaction is essential for every generation of high-tech companies to become leaders with real commercial value. Today, the interface of artificial intelligence is already very clear; it is the dialogue process, represented today by ChatGPT. However, to say that OpenAI plus Microsoft represents this era is still premature; they are leading, but there is still much room for imagination in the future.
Tenth Insight: The Essence of Intelligence
Today, although large models have brought many shocks, we lack theoretical understanding of large models and deep learning. Today, we would be thrilled to have any theory. Unlike physics, where there are beautiful laws describing everything from the vastness of the cosmos to the minutiae of quantum mechanics, today’s artificial intelligence lacks such theories; it lacks interpretability and robustness. The framework of deep learning does not reach true general artificial intelligence.
Discussions about the emergence of artificial intelligence are merely surface-level; we have not clarified them. Why does intelligence emerge when models reach a certain size? Why can a 70B model exhibit emergent intelligence? There is no clear reasoning behind this. Thus, we are working diligently to study these issues. Last summer, I organized a seminar at the Hong Kong University of Science and Technology titled “Mathematical Theory for Emergent Intelligence,” discussing the need to clarify the scientific and mathematical principles behind emergent intelligence. We need more explorers willing to engage in this challenging area, especially with the emergence of projects like Tencent’s “Scientific Exploration Award” and “New Cornerstone Researchers,” bringing in more young scientists who can contribute confidence and faith to tackle the difficult problems for future breakthroughs in artificial intelligence development.
Once again, congratulations to all the awardees and young scientists. The development of technology relies on generations of young people, especially in artificial intelligence. Thank you all once again.
For submissions, please click “Read the original article”

Responsible Editor: Duan Shaomin
Review: Li Guoqing
Source: Chief Digital Officer. If there are copyright issues, please contact us promptly. The copyright interpretation rights belong to the original author. This article is recommended reading by Intelligent Manufacturing IMS!
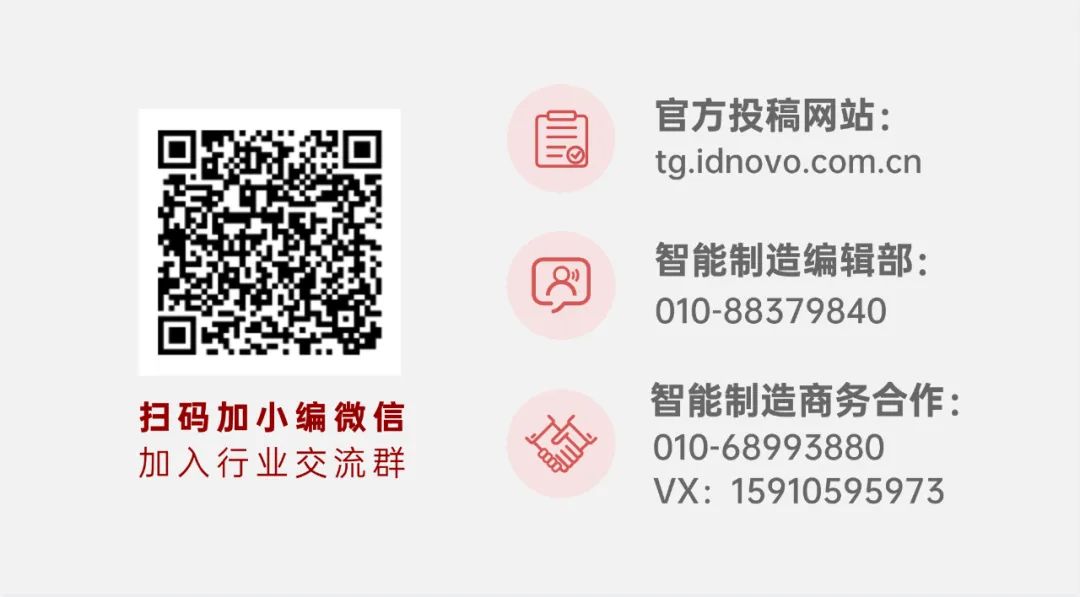
Pleasefollowthe video account
to learn more about intelligent manufacturing news