Originally from Robotics Network
In fact, artificial intelligence has existed in our lives for a long time. However, for many people, artificial intelligence is still a relatively “profound” technology, but no matter how profound the technology is, it starts from basic principles. In the field of artificial intelligence, there are 10 major algorithms that are simple in principle, discovered and applied a long time ago, and you may have learned them in high school; they are also very common in daily life.
▍Linear Regression
Linear Regression (Linear Regression) is probably the most popular machine learning algorithm. Linear regression seeks to find a line that fits the data points in a scatter plot as closely as possible. It attempts to represent the relationship between the independent variable (x value) and the dependent variable (y value) by fitting the line equation to the data. This line can then be used to predict future values!
The most commonly used technique for this algorithm is Least Squares. This method calculates the best fit line to minimize the vertical distance from each data point to the line. The total distance is the sum of the squares of the vertical distances (green line) from all data points. The idea is to fit the model by minimizing this squared error or distance.
For example, in simple linear regression, there is one independent variable (x-axis) and one dependent variable (y-axis).
For instance, predicting the increase in housing prices next year, or the sales of a new product next quarter. It doesn’t sound difficult, but the challenge of linear regression lies not in obtaining the predicted value, but in how to make it more precise. For that possibly very minute number, many engineers have exhausted their youth and hair.
▍Logistic Regression
Logistic Regression (Logistic regression) is similar to linear regression, but the result of logistic regression can only have two values. While linear regression predicts an open numerical value, logistic regression is more like a yes or no question.
The range of Y values in the logistic function is from 0 to 1, representing a probability. The logistic function usually has an S-shape, dividing the chart into two regions, making it suitable for classification tasks.
For example, the above logistic regression curve shows the probability of passing an exam in relation to study time, which can be used to predict whether one can pass the exam.
Logistic regression is often used by e-commerce or delivery platforms to predict user preferences for product categories.
▍Decision Trees
If linear and logistic regression complete tasks in one round, then Decision Trees (Decision Trees) are a multi-step process. They are used for both regression and classification tasks, but the scenarios are usually more complex and specific.
For a simple example, if a teacher is faced with a class of students, how can they determine who the good students are? Judging that a score of 90 is a good student seems too simplistic; one cannot rely solely on scores. For students with scores below 90, we can discuss several aspects such as homework, attendance, and questioning.
The above is an illustration of a decision tree, where each forked circle is called a node. At each node, we ask questions about the data based on the available features. The left and right branches represent possible answers. The final node (the leaf node) corresponds to a predicted value.
The importance of each feature is determined using a top-down approach. The higher the node, the more important its attribute. For example, in the above example, the teacher considers attendance more important than homework, so the attendance node is higher, and of course, the score node is higher.
▍Naive Bayes
Naive Bayes (Naive Bayes) is based on Bayes’ theorem, which relates two conditional probabilities. It measures the probability of each class, providing the conditional probability for each class given the value of x. This algorithm is used for classification problems, yielding a binary “yes/no” result. Take a look at the equation below.
The Naive Bayes classifier is a popular statistical technique, with classic applications in spam filtering.
Of course, I bet a hotpot meal that 80% of people didn’t understand the above statement. (The 80% figure is my guess, but experiential intuition is a kind of Bayesian calculation.)
To explain Bayes’ theorem in non-technical terms, it is the probability of B occurring given A, to deduce the probability of A occurring given B. For example, a kitten likes you, with a a% chance of rolling on its back in front of you; how likely is it that the kitten likes you if it rolls on its back?
Of course, doing such a problem is like shooting in the dark, so we need to introduce other data. For example, the kitten likes you with a b% chance of cuddling, and a c% chance of purring. So how do we know how likely the kitten likes us? We can calculate it through Bayes’ theorem from the probabilities of rolling over, cuddling, and purring.
▍Support Vector Machine
Support Vector Machine (Support Vector Machine, SVM) is a supervised algorithm used for classification problems. A support vector machine tries to draw two lines between data points, maximizing the margin between them. To do this, we plot the data items as points in an n-dimensional space, where n is the number of input features. Based on this, the support vector machine finds an optimal boundary, called a hyperplane, which best separates the possible outputs by class labels.
The distance between the hyperplane and the nearest class point is called the margin. The optimal hyperplane has the maximum margin, which classifies points so that the nearest data points to both classes are maximized.
Thus, the problem that the support vector machine aims to solve is how to segregate a bunch of data. Its main application scenarios include character recognition, facial recognition, text classification, and various recognition tasks.
▍K-Nearest Neighbors Algorithm (KNN)
K-Nearest Neighbors (K-Nearest Neighbors, KNN) is very simple. KNN classifies an object by searching for K most similar instances in the entire training set, i.e., K neighbors, and assigns a common output variable to all these K instances.
The choice of K is crucial: a smaller value may yield a lot of noise and inaccurate results, while a larger value is impractical. It is most commonly used for classification but can also be applied to regression problems.
The distance used to assess similarity between instances can be Euclidean distance, Manhattan distance, or Minkowski distance. Euclidean distance is the ordinary straight-line distance between two points. It is actually the square root of the sum of the squares of the differences of the point coordinates..
KNN Classification Example
KNN is theoretically simple and easy to implement, and can be used for text classification, pattern recognition, cluster analysis, etc.
▍K-Means
K-Means (K-means) is a clustering algorithm that categorizes a dataset. For example, this algorithm can be used to group users based on purchase history. It finds K clusters in the dataset. K-Means is used in unsupervised learning, so we only need to use training data X and the number of clusters K we want to identify.
The algorithm iteratively assigns each data point to one of the K groups based on the features of each data point. It selects K points for each K-cluster (called centroids). Based on similarity, new data points are added to the cluster with the nearest centroid. This process continues until the centroids stop changing.
In daily life, K-Means plays an important role in fraud detection and is widely used in the fields of automotive, health insurance, and insurance fraud detection.
▍Random Forest
Random Forest (Random Forest) is a very popular ensemble machine learning algorithm. The basic idea of this algorithm is that the opinions of many people are more accurate than those of individuals. In Random Forest, we use an ensemble of decision trees (see Decision Trees).
(a) During training, each decision tree is built based on bootstrap samples of the training set.
(b) During classification, the decision for the input instance is made based on majority voting.
Random Forest has broad application prospects, from marketing to healthcare insurance; it can be used for modeling marketing simulations, statistics on customer sources, retention, and attrition, as well as predicting disease risks and patient susceptibility.
▍Dimensionality Reduction
Due to the large amount of data we can capture today, machine learning problems have become more complex. This means training is extremely slow and finding a good solution is difficult. This problem is often referred to as the “Curse of Dimensionality“.
Dimensionality reduction (Dimensionality reduction) attempts to solve this problem by combining specific features into higher-level features without losing the most important information. Principal Component Analysis (PCA) is the most popular dimensionality reduction technique.
Principal Component Analysis reduces the dimensionality of the dataset by compressing it into low-dimensional lines or hyperplanes/subspaces, preserving the significant features of the original data as much as possible.
An example of achieving dimensionality reduction could be approximating all data points to a straight line.
Artificial Neural Networks
Artificial Neural Networks (Artificial Neural Networks, ANN) can handle large and complex machine learning tasks. A neural network is essentially a set of interconnected layers of nodes with weights, called neurons. Between the input layer and the output layer, we can insert multiple hidden layers. Artificial neural networks use two hidden layers. Additionally, deep learning needs to be addressed.
The operation of artificial neural networks is similar to the structure of the brain. A set of neurons is assigned a random weight to determine how the neurons process input data. The neural network learns the relationship between input and output by training on input data. During the training phase, the system can access the correct answers.
If the network cannot accurately recognize the input, the system will adjust the weights. After sufficient training, it will consistently recognize the correct patterns.
Each circular node represents an artificial neuron, and the arrows indicate the connections from the output of one artificial neuron to the input of another.
Image recognition is a famous application within neural networks.
Now, you have learned the basic introduction to the most popular artificial intelligence algorithms, and you have a certain understanding of their practical applications.
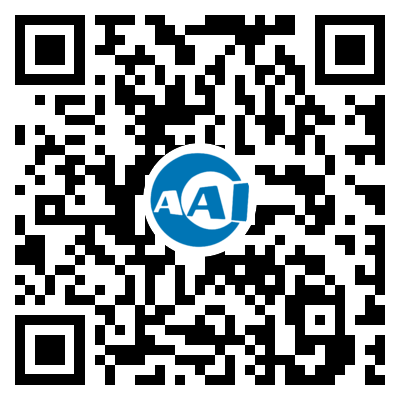
Scan to Join Us
Individual Membership: 1000 yuan/session (5 years)
Student Membership: 50 yuan/session (5 years)
Lifetime Membership: 2000 yuan