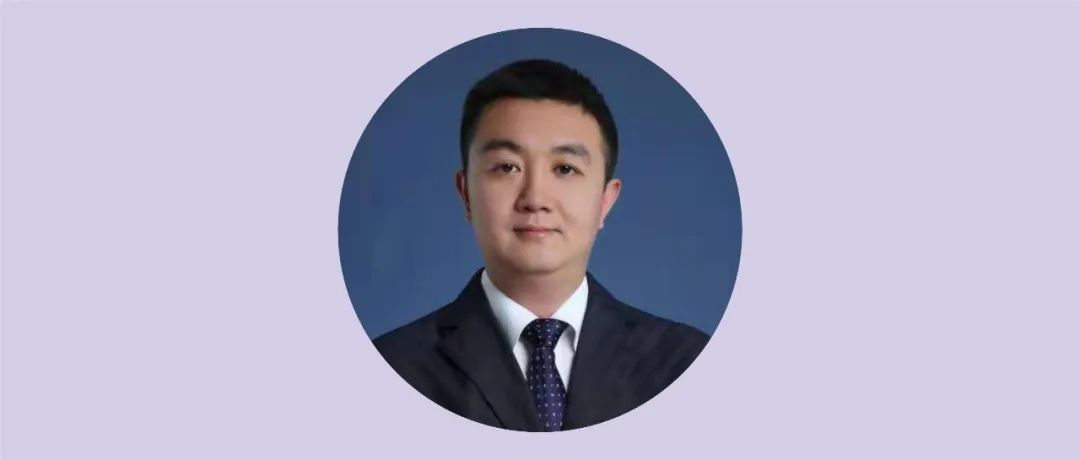

Editor | Wang Duoyu
Typesetting | Shui Chengwen
Deep Learning assisted functional protein design is a novel method that meets the growing demand for new biocatalysts in scientific and industrial production contexts, promoting the rapid discovery and modification of efficient and specialized proteins, and expanding the research boundaries of protein engineering. By harnessing the power of deep learning, designers can generate a variety of novel proteins tailored to specific criteria, such as higher stability, stronger binding affinity, and increased enzymatic activity. Furthermore, the diverse new protein sequences enrich the repertoire of research protein families, surpassing the limited natural sequences. This enhancement not only supplements the available resources for analyzing and understanding proteins but also provides a broader pool of protein templates aimed at enhancing functionality.
However, existing methods often require training a model with a massive number of parameters on large-scale datasets (making it difficult to generalize to specific proteins, such as those with few homologous sequences), and the generated proteins typically have relatively simple structures and functions (single-domain, single-function), with unsatisfactory experimental validation positive rates (the proportion of active proteins designed is low, and even fewer exceed the wild-type proteins).
On September 10, 2024, the team led by Hong Liang at the Shanghai Jiao Tong University Institute of Natural Sciences published a research paper titled: A conditional protein diffusion model generates artificial programmable endonuclease sequences with enhanced activity in the journal Cell Discovery.
This research designed a diffusion probability model framework—CPDiffusion, successfully designing and generating artificial programmable endonuclease sequences with enhanced activity.
This groundbreaking research demonstrates the powerful potential of deep learning in the field of protein engineering, bringing new application prospects to protein engineering, biotechnology, molecular diagnostics, and other fields. Compared to existing sequence design methods, this approach learns the implicit mapping rules between protein sequences, structures, and functions at an extremely low cost of model training and data, generating diverse protein sequences and achieving a very high success rate through wet lab validation. Ultimately, it achieved over a tenfold increase in DNA cleavage activity in two ultra-long, multi-domain complex functional proteins (KmAgo and PfAgo), significantly higher than any currently discovered wild-type protein activity at room temperature. Additionally, unlike traditional directed evolution methods, this approach can change hundreds of amino acids at once, making it possible to select entirely new evolutionary starting points for protein engineering, explore the evolutionary pathways of proteins in biological research, and break through patent barriers in biotechnology.
It is reported that this is the most complex and largest single protein generated by AI reported in the global literature to date, verified to have excellent activity through wet lab experiments.
This study designed a novel protein sequence design and screening process—CPDiffusion, which combines various generation conditions such as scaffold structure and active sites to generate diverse new sequences for specific functional proteins (Figure 1). The initialized model was trained on twenty thousand wild-type protein structures and sequences to learn the mapping relationships between protein sequences, structures, and functions. Meanwhile, to strengthen the model’s understanding of the features of the proteins to be generated, several hundred sequences and structures from other proteins in the same family were also included in the training set for joint training.
During the generation process, the original protein sequence and information are processed into amino acid-level graph representations with molecular biochemical and topological characteristics. In the forward diffusion process, each type of amino acid in the input protein is gradually destroyed over T steps by following a certain replacement probability matrix to achieve a uniform distribution; the reverse diffusion process starts from random sampling, where each amino acid node type is evenly distributed among 20 types of amino acids, followed by a stepwise denoising process. The denoising process is guided by conditions, such as the wild-type scaffold structure and secondary structure of the sequence to be generated, as well as the amino acid replacement matrix based on wild-type proteins (BLOSUM62). To ensure that the model learns the invariance implicit in the three-dimensional structure of the protein, the propagation function is fitted by an equivariant graph convolutional layer.
This diffusion probability model ultimately generates the joint probability distribution of each amino acid on the scaffold, and by sampling the learned distribution, corresponding protein sequences can be obtained. Subsequently, AlphaFold2 is used for structural prediction of the generated sequences, and after screening based on RMSD, pLDDT, etc., a batch of sequences is obtained, which are then synthesized, characterized, and evaluated in wet lab experiments to confirm their expression, activity, thermal stability, and other performances.
Figure 1: Schematic diagram of the CPDiffusion framework
To verify the generation effect of CPDiffusion, the research team specifically considered an important issue in biotechnology, which is generating prokaryotic endonucleases with high DNA cleavage activity and stability at room temperature (prokaryotic Argonaute, abbreviated as pAgo protein).
pAgo proteins are a class of endonucleases that play a key role in DNA interference in prokaryotes, with significant ability to target and cleave specific single-stranded DNA/RNA sequences, which have important applications in diagnostics, such as detecting and quantifying nucleic acid sequences associated with pathogens or cancer mutations, thus providing early disease detection and precise treatment. Furthermore, pAgo proteins possess high affinity for substrates and specific recognition of target sequences, making them important tools in imaging and gene editing. In isothermal nucleic acid detection and gene editing technologies, mesophilic pAgo proteins (e.g., KmAgo) are typically considered as candidate proteins. However, these proteins have relatively low DNA cleavage activity, limiting their potential applications. On the other hand, thermophilic pAgo proteins (e.g., PfAgo) exhibit significantly higher DNA cleavage activity but generally only function at elevated temperatures, losing activity as the temperature decreases, making them difficult to apply in detection and editing tasks at room temperature.
The above two proteins serve as representative wild-type proteins with high activity at mesophilic and hyperthermophilic conditions, both composed of nearly 800 amino acids and containing six domains. Using the CPDiffusion design and screening framework developed in this research, the team generated 27 new artificial KmAgos (Km-APs) and 15 artificial PfAgos (Pf-APs). Compared to the template WT, they share 50% to 70% similarity in sequence identity. Compared to other WT proteins in NCBI (excluding the template), the sequence identity of APs is less than 40%. Unlike classical rational design methods, the entire process of model training and inference requires almost no expert guidance to automatically identify highly conserved regions, thereby allowing for more changes in non-conserved regions while ensuring functionality, increasing the diversity of generated sequences (Figure 2).
Figure 2: CPDiffusion successfully learns the conserved features of protein sequences, surface properties, and other important characteristics
Through various experimental validations, the research team found that among the two groups of new sequences generated for KmAgo and PfAgo, over 90% of the new sequences exhibited DNA cleavage activity, with more than 70% of the activity enhanced compared to their wild-type baseline (Figure 3). Notably, the best-performing new KmAgo exhibited nine times the activity of wild-type KmAgo, while the best new PfAgo reduced the melting temperature of wild-type PfAgo from about 100°C to 50°C, achieving double the single-stranded DNA cleavage activity at 45°C compared to wild-type PfAgo at 95°C, and eleven times that of wild-type KmAgo at moderate temperatures. These significant results demonstrate the strong potential of CPDiffusion in automatically learning from wild-type functional proteins and designing protein sequences with highly complex biological functions to enhance functionality.
Figure 3: KmAgo working principle diagram and Km-APs expression, activity, thermal stability experimental results
Overall, CPDiffusion as a powerful new protein sequence design tool offers biologists and protein engineering designers new possibilities for designing more powerful proteins, studying the gradual evolution of protein functions, enriching existing protein databases, and more.
Dr. Zhou Bingxin, assistant researcher at the Shanghai Jiao Tong University Institute of Natural Sciences/Shanghai National Center for Applied Mathematics (Shanghai Jiao Tong University Branch), Dr. Zheng Lirong, postdoctoral researcher at the University of Michigan Neuroscience Institute/Cell and Developmental Biology Institute, and doctoral student Wu Banghao at the Shanghai Jiao Tong University School of Life Sciences and Technology are the co-first authors of the paper. Professor Hong Liang of the Shanghai Jiao Tong University Institute of Natural Sciences/School of Physics and Astronomy/Zhangjiang Advanced Research Institute/School of Pharmacy, Pietro Liò from the University of Cambridge, and Dr. Zheng Lirong from the University of Michigan are the co-corresponding authors of the paper.
Paper Link:
https://www.nature.com/articles/s41421-024-00728-2
Set Star to not miss exciting tweets
Welcome to share in Moments and WeChat groups
To promote the dissemination and exchange of cutting-edge research, we have formed several professional communication groups. Long press the QR code below to add the editor’s WeChat to join the group. Due to the high number of applications, please note your: School/Major/Name when adding WeChat. If you are a PI/Professor, please also indicate.
Click to View and Share Your Taste