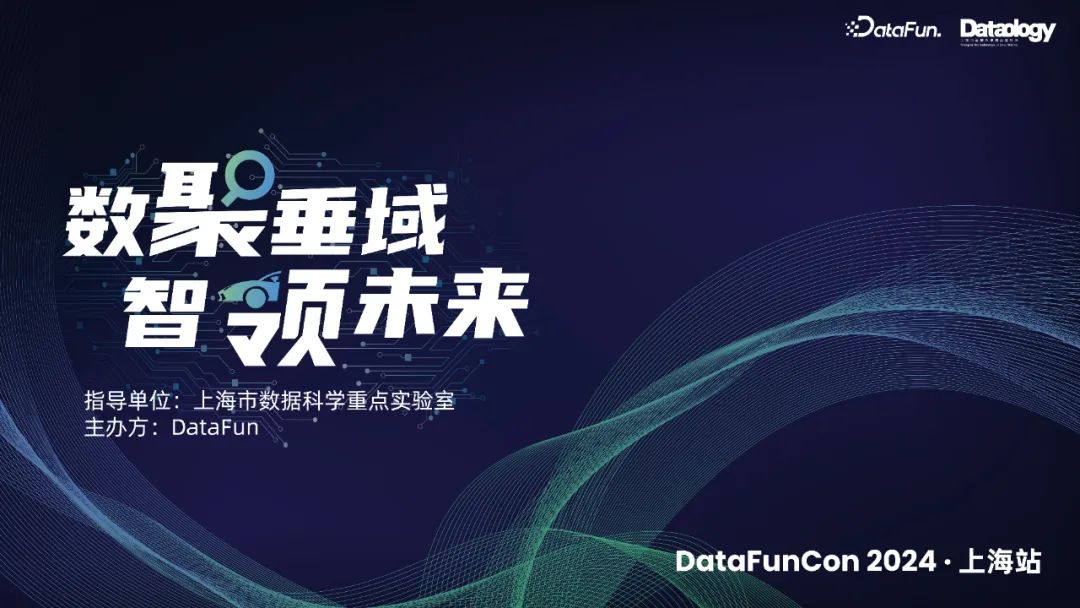
Wang Haofen, Tongji University, “Hundred Talents Program”, Distinguished Researcher, PhD Supervisor
Personal Introduction: Wang Haofen, distinguished researcher and PhD supervisor in the “Hundred Talents Program” at Tongji University. He has served as CTO in frontline artificial intelligence companies for a long time. He is one of the initiators of OpenKG, the world’s largest Chinese open knowledge graph alliance. He has participated in several national AI-related projects, published over 100 high-level papers in the AI field, with over 3100 citations and an H-index of 29. He built the world’s first interactive virtual idol—”Amber·Virtual Face”; the intelligent customer service robot he developed has served over 1 billion users. Currently, he holds various social positions including Deputy Director of the Terminology Committee of the Chinese Computer Society, Chair of SIGKG, Secretary-General of Shanghai, Secretary-General of the Natural Language Processing Committee, Director of the Chinese Association for Chinese Information Studies, Deputy Secretary-General of the Language and Knowledge Computing Committee, and Deputy Director of the Natural Language Processing Committee of Shanghai Computer Society.
Lecture Title: Recommendation Systems From RAG Perspective: Opportunities and Challenges
Lecture Outline: The recommendation system field currently faces many challenges, such as data long-tail distribution, personalized recommendations, multi-turn dialogue, and cold-start recommendations. The emergence of large language models has brought new opportunities for recommendation systems, and the integration of the two is gradually becoming the trend for the next generation of recommendation systems. However, simply using LLMs for recommendations still has limitations, such as insufficient use of personal information, limited context length, hallucinations, and an inability to learn from all historical interaction data. Therefore, introducing Retrieval-Augmented Generation (RAG) is particularly necessary, as it helps control hallucinations, reduce dependence on context, learn new knowledge, and can quickly collaborate with traditional recommendation systems. This report systematically reviews the limitations of current recommendation systems, the status of LLM-enhanced recommendation systems, the technical routes and paradigms of RAG, and its application in enhancing recommendation systems, while elaborating on the opportunities and development trends of recommendation systems from the RAG perspective.
Audience Benefits:
1. Challenges of recommendation systems and the current limitations of large models applied to recommendation systems
2. Development, key technologies, and paradigms of RAG
3. Opportunities and future development of RAG applied to recommendation systems
Scan to inquire and register
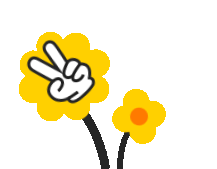