This article was published in “Modern Film Technology”, 2023, Issue 10.
Expert Review
Digital humans first appeared in the late 20th century. With the development and advancement of modern intelligent technology, digital human technology has driven rapid growth in industries such as healthcare, education, and entertainment, especially in film production. The application of digital humans not only brings more creativity and possibilities to films but also provides audiences with a realistic visual experience. In recent years, breakthroughs have been made in artificial intelligence-generated content (AIGC) technology. With the rapid development of multimodal AI large models, digital human creation has entered the AIGC era. This article, “Overview of Digital Humans in Film Empowered by AIGC,” introduces the application and technological evolution of two types of digital humans: real digital doubles and virtually created characters in film production, providing a detailed analysis of the current application status of digital humans in the film industry. It elaborates on how AIGC has significantly accelerated the production process of digital humans, reduced production costs, and improved production efficiency from the aspects of image generation, voice synthesis, and animation driving. Additionally, it discusses the development and application prospects of digital humans in film production from the perspective of mass generation and intelligent interaction. This article helps to understand the current application status of AIGC digital humans in film and television production, as well as their indispensable importance in the film industry.
ββ Wang Muwang
Senior Engineer
Deputy Director of the Transmission and Projection Technology Research Department, China Film Science and Technology Research Institute (Central Propaganda Department Film Technology Quality Inspection Institute)
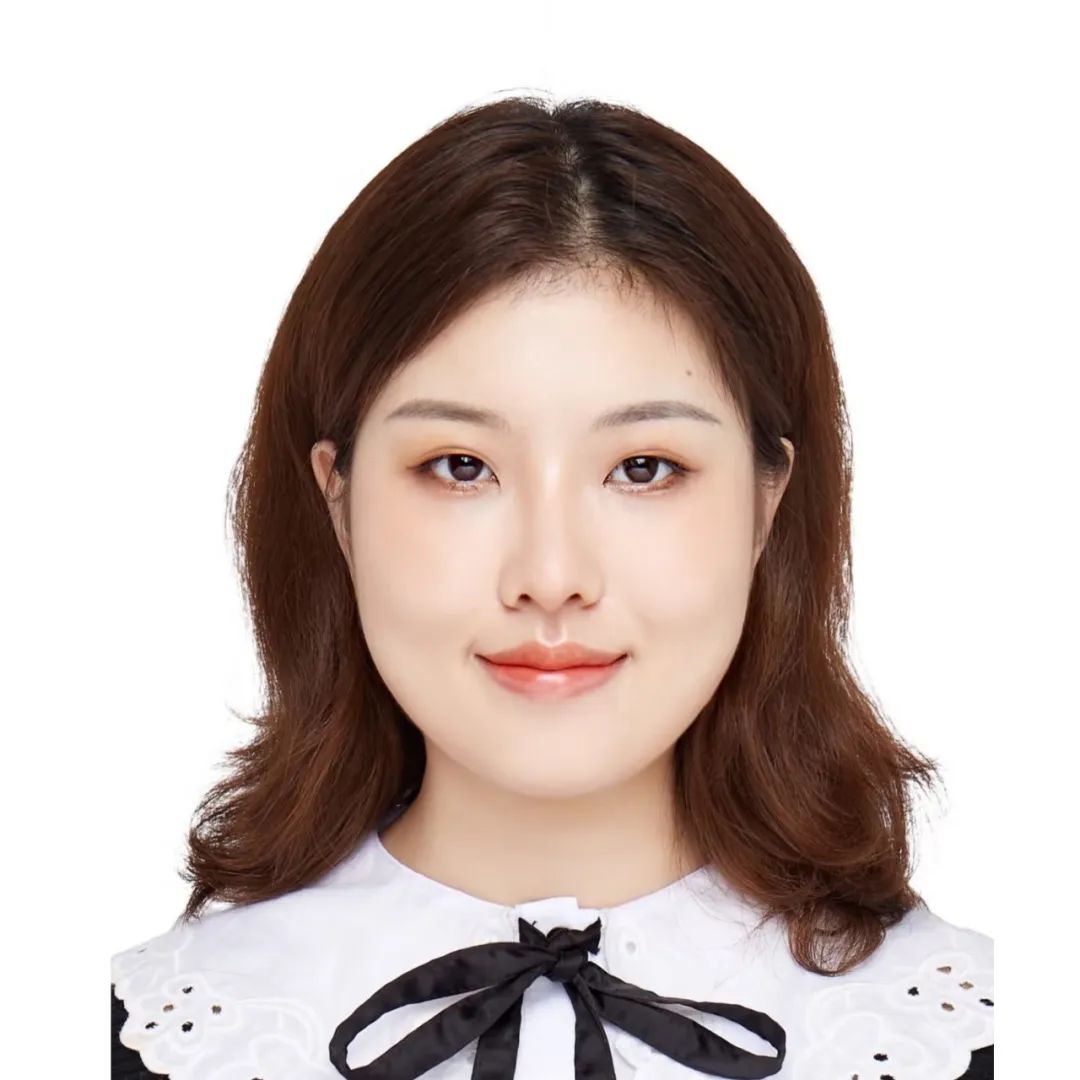
Master’s student at Tsinghua University Shenzhen International Graduate School, majoring in digital human visualization expression and interactive applications.
Master’s student at Tsinghua University Shenzhen International Graduate School, majoring in digital human motion generation and interactive applications.
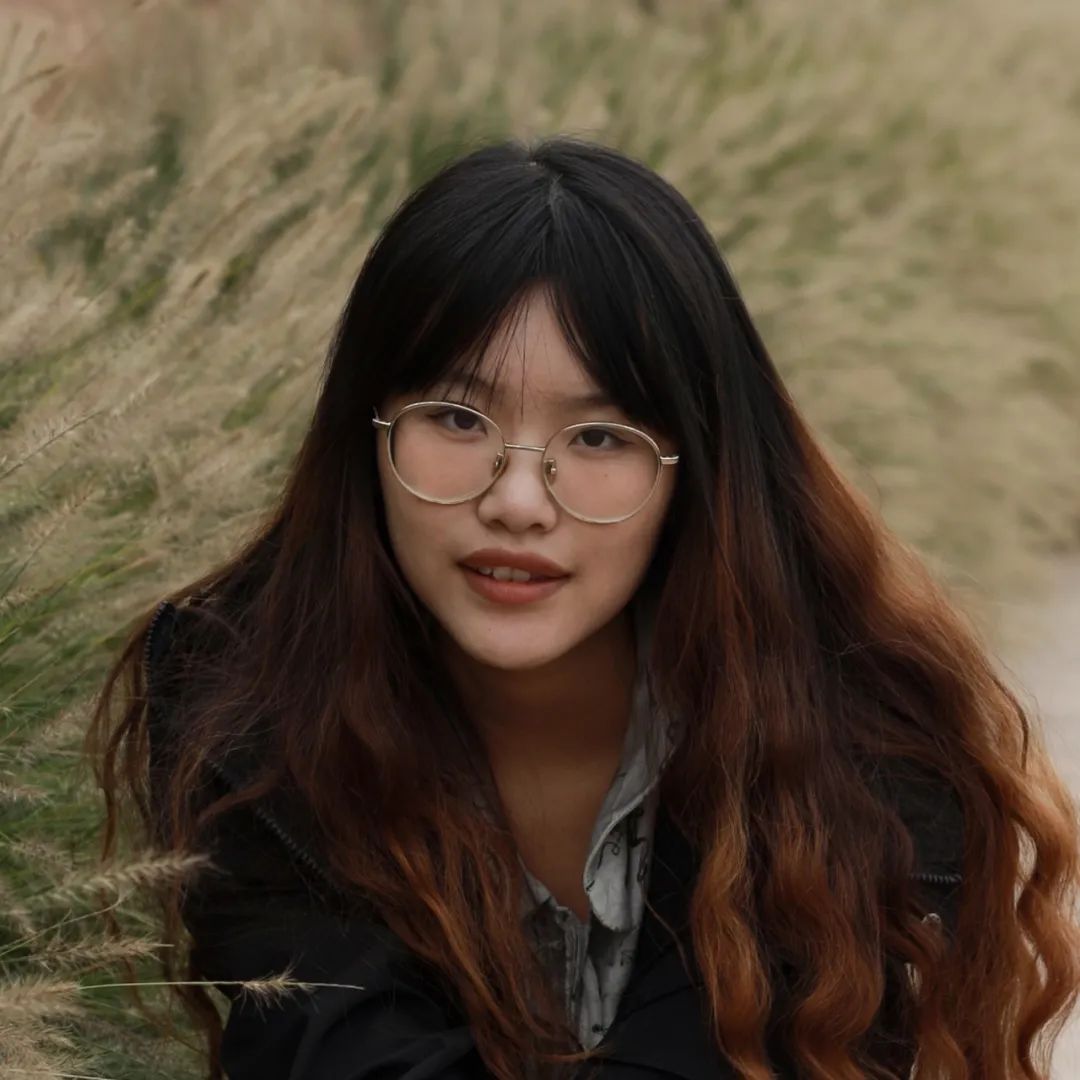
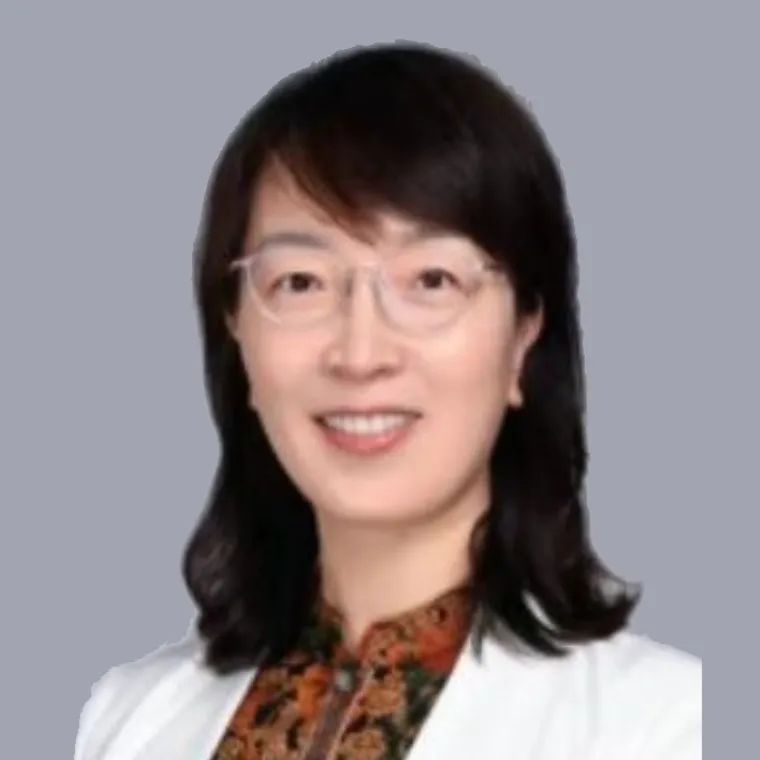
Professor at Tsinghua University, doctoral supervisor, major research directions include artificial intelligence, data mining, and pattern recognition.
Abstract
As an important component of digital film technology, digital humans not only provide filmmakers with a broader creative space but also allow audiences to experience more exciting and realistic visual effects and storylines, making it an important exploration direction for the high-quality development of the future film industry. With continuous breakthroughs in artificial intelligence (AI) technology in deep learning (DL), natural language processing (NLP), and computer vision (CV), more and more film and television companies have increased their technical research and development efforts on digital humans. This article briefly introduces the application status of digital humans in the film industry by analyzing two types of digital humans: real digital doubles and virtually created characters. It summarizes the optimization of digital human production efficiency empowered by artificial intelligence-generated content (AIGC) technology from three aspects: image generation, voice synthesis, and animation driving. It also explores the application prospects of digital humans in film production from the perspectives of mass generation and intelligent interaction, providing some insights into the application of digital humans in the film industry.
Keywords
Digital Humans; AIGC; Film Production; Deep Learning
1 Introduction
Digital humans are virtual entities that simulate and replicate human structure, form, and behavior through computer technology. With continuous progress and innovation in technology, film production teams can use digital human technology to present audiences with more realistic and stunning visual effects. The rapid development of this technology brings unlimited possibilities to the film industry, and many outstanding films have utilized digital human technology to bring historical figures, fictional characters, and magical creatures to life on screen. For example, James Cameron’s sci-fi epic “Avatar: The Way of Water” uses advanced digital human technology to merge humans with the fictional world of the Na’vi, providing audiences with a realistic visual experience. The Marvel superhero blockbuster “Avengers: Endgame” successfully utilized digital human technology to replicate and reshape many characters, allowing audiences to see younger versions of Tony Stark, Steve Rogers, and Thor Odinson, seamlessly integrating these digital human characters with the performances of real actors, adding emotional impact and dramatic tension to the film.
In 2012, deep learning (DL) technology entered the public eye, and the digital human industry gradually transitioned from costly and time-consuming animation production to artificial intelligence (AI) production, significantly reducing production costs and improving efficiency. Empowered by artificial intelligence-generated content (AIGC) technology, virtual digital humans, as an integration of multiple cross-domain technologies, not only significantly enhance the efficiency and quality of film production but also provide more possibilities for future application development.
2 Application of Digital Humans in the Film Industry
Real digital doubles and virtually created characters represent two stages in the development of digital humans. Real digital doubles use digital technology to replace real actors during scene shooting, while virtually created characters are entirely generated by computer programs. With technological advancements, the precision and technology of producing real digital doubles and virtually created characters continue to improve, providing filmmakers with broader creative spaces and making the performances of digital humans more realistic.
2.1 Real Digital Doubles
Real digital doubles are an early type of digital human that uses digital technology to create characters that replace real actors in films. Traditional film shooting often involves dangerous scenes such as fight sequences and explosions, leading to the use of digital doubles to reduce risk and costs. The earliest digital double can be traced back to the 1985 film “Young Sherlock Holmes,” where Industrial Light & Magic (ILM) created the first CG character in film history by creating a digital double of a knight for battle scenes. In 1997, the underwater scenes of the film “Titanic” first used completely computer-generated digital double actors, making underwater segments more realistic and stunning.
Constructing corresponding digital doubles by scanning real actors is a common practice in the film industry. Film production companies obtain high-fidelity three-dimensional reconstructions of actors’ images through light stage photography, digitizing the real actors’ images by simulating environmental light, skin tone reconstruction, and facial binding, and then combining the digital images with the actual performances of the actors to achieve the effect of shooting with digital doubles, thus avoiding many unavoidable shooting challenges such as time constraints, shooting difficulty, and film quality issues[1].
Once films fully entered the digital age, digital doubles transitioned to a stage involving 2D image face-swapping and 3D motion capture face-swapping. For example, during the shooting of “Furious 7,” lead actor Paul Walker tragically passed away in a serious car accident. To ensure the film’s smooth production, the crew chose to use 2D digital image face-swapping technology, completing the final shoot with the help of Paul Walker’s remaining footage and the performance of his brother as a stand-in. The application of 3D motion capture face-swapping technology is even more widespread, whether in scenes where characters encounter their clones in “Gemini Man” or in films like “Blade Runner 2049” and “Logan,” where characters portray different age versions of themselves, 3D motion capture face-swapping technology allows for more exquisite and realistic performances by real digital doubles, providing directors with more creative space.
As technology has developed, the production costs and effectiveness of real digital double technology have significantly improved. As early as 2008, a special effects company that created effects for the film “The Curious Case of Benjamin Button” had already used facial capture technology to combine the protagonist’s performance with a 3D digital model, allowing the protagonist to portray the stages of life from old age to youth, winning the 2009 Academy Award for Best Visual Effects. In the 2019 Oscar-winning film “Green Book,” the scenes of the protagonist playing the piano were also shot using a stand-in pianist and AI face-swapping technology for post-production synthesis.
On the technology optimization front, the threshold for AI deep synthesis technology is continuously lowering, and the iterative development of such technologies has provided solutions to the challenge of maintaining actors’ appearances across series films. In Marvel’s “Spider-Man: No Way Home,” AI face-swapping synthesis technology was used to make Doctor Octopus and the Green Goblin appear younger; in “The Irishman,” Robert De Niro, Al Pacino, and Joe Pesci, all in their seventies, achieved seamless de-aging to portray younger versions of themselves. Following the release of high-resolution versions of Deepfake tools in 2020[2], Disney recently released the first automated AI video face processing model suitable for real scenes, FRAN (Face Re-Aging Network)[3], which further enhances AI’s ability to change the visual effects of actors’ appearances. FRAN can use data information to predict the areas of real actors’ faces that will age and can add wrinkles and chins to existing video clips or remove wrinkles from characters’ faces in existing footage. The emergence of the FRAN algorithm has expanded the creative space for using digital doubles.
In terms of real-time feedback, the British generative AI company Metaphysic has proposed the Metaphysic Live product, which can create high-resolution, realistic face-swapping and de-aging effects in real-time based on actors’ live performances without further compositing or visual effects (VFX) work, and can stream AI-generated realistic content to real-world scenes at 30FPS. The film “Here” showcased ultra-realistic face-swapping and de-aging technology driven by AIGC, allowing directors, actors, and producers to view and adjust performances in real-time, significantly shortening the film production cycle; the same de-aging work took the production company two years for the 2019 film “Gemini Man.”
2.2 Virtually Created Characters
Virtually created characters are a mid-stage type of digital human, referring to digital humans that are entirely generated by computer programs without any physical form or real identity, existing only in the virtual space of computers. The development of CG technology has allowed digital humans to go beyond the biological models of real actors and to create characters and creatures that cannot be filmed in real life through artistic creation and computer synthesis.
The early virtually created characters can be traced back to James Cameron’s “The Abyss” in 1989, where alien intelligent beings controlled water columns that moved in front of the male and female protagonists, with the water columns reflecting their faces. In 2001, Peter Jackson’s “The Lord of the Rings: The Fellowship of the Ring” created a milestone virtual character, Gollum. Later, Weta Digital introduced virtual character images such as “King Kong” and “Caesar” from the “Planet of the Apes” series. In 2006, the special effects company Industrial Light & Magic created an octopus captain who could play the organ in “Pirates of the Caribbean: Dead Man’s Chest,” marking the full-scale application of virtually created characters in film production.
The development of motion capture technology has made virtually created characters appear more natural in film production. In 2001, the sci-fi film “Final Fantasy: The Spirits Within,” derived from a game, became a benchmark work in motion capture history. Not only was the female lead Aki’s entire animation driven by motion capture technology, but it also allowed her to have hair that flowed and rotated naturally, marking the first time a virtually created character achieved realistic hair movement in the CG world, with dynamic lighting effects applied. In 2004, Robert Zemeckis, who directed “Forrest Gump,” filmed Hollywood’s first full-motion CG movie, “The Polar Express,” where Oscar-winning actor Tom Hanks played six virtual digital characters, with all digital animations achieved through motion capture technology.
With the support of AI technology, virtually created characters have seen rapid improvements in both production precision and effect presentation. The protagonist Alita in the 2019 film “Alita: Battle Angel” is the first ultra-high precision character entirely created using digital human technology. Engineers developed a new pore growth technology using AI algorithms to achieve the natural hair effect on Alita’s face. In terms of driving, “Alita: Battle Angel” upgraded motion capture to “performance capture,” allowing Weta Studio to redirect the performance of the actress “Rosa Salazar – CG Rosa Salazar – Alita,” enhancing Alita’s facial expression tracking to realistically express the emotions and performances of the actress. Similarly, in “Avengers: Infinity War,” Disney used AI algorithms to perform high-resolution scans of actors’ faces and automatically mapped facial images onto Thanos’s body, allowing Thanos to exhibit realistic anthropomorphic performances. In “Avatar: The Way of Water,” Weta Studio developed a new APFS system (Anatomically Plausible Facial System) to enhance the quality of performances for virtually created characters. This system assists staff in drawing facial animations while collecting a large amount of facial scan data for subsequent neural network model training, learning the potential muscle behaviors of actors through AI deep learning models and algorithms to quickly assist in generating facial animations for the Na’vi, while correcting anatomical deviations in teeth and skull structure.
3 AIGC Empowering the Production and Application of Digital Humans in Film
3.1 AIGC Assisting Digital Human Production
High-cost investment and long production cycles have always been two major challenges faced by digital humans in film production. Traditional digital human production processes rely almost entirely on manual implementation, from character modeling to animation production to voice synthesis, with each step requiring significant time and manpower investment. However, with the continuous innovation of generative algorithms, pre-trained models, and multimodal technologies, the development of AIGC has significantly accelerated the production process of digital humans and greatly reduced production costs, significantly enhancing production efficiency.
The digital human production process can be mainly divided into three sections: image generation, voice synthesis, and animation driving. With the support of AIGC technology, image generation can utilize algorithm models to quickly generate high-precision digital human images, voice synthesis can generate realistic voice expressions using voice conversion technologies, and animation driving can utilize deep learning models to drive the expressions and movements of digital humans.
(1) Intelligent Generation of Digital Human Images
The traditional process of generating realistic digital humans relies on designers manually creating three-dimensional models of human bodies using 3D modeling software such as Maya, 3ds Max, and CAD. However, due to the high learning costs of these software and their low modeling efficiency, it is challenging to quickly and batch-generate digital human images.
With the acceleration and iteration of AI generation algorithms, two main model generation methods have emerged for generating realistic virtual human images: the first is image and video-based generation, which has initially achieved productization and can reach the precision level of next-generation game characters. Users can upload photos or videos to generate realistic digital humans. Representative applications of this generation method include NVIDIA’s Omniverse Avatar and Unreal Engine’s MetaHuman Creator, allowing users to quickly generate corresponding digital human images through customization. The second method is based on parameterized models for digital human generation, a research hotspot in academia in recent years. This method decouples the originally complex digital human space through parameterized models, abstracting digital humans into a limited number of parameters under certain constraints, which has also become a research hotspot in academia recently.
Image generation of digital humans based on images and videos already has a relatively mature production process. Taking MetaHuman Creator as an example, it is a cloud-based online editor that allows users to select elements from a mixed sample library or perform custom modeling to achieve the desired results. The process of generating realistic digital humans from images begins with photographing real people, importing multiple facial photographs into software like RealityCapture to generate high-quality meshes and textures. Next, the mesh is imported into Unreal Engine for facial marker frame tracking and identity parsing, submitting the model’s mesh to the MetaHuman backend to quickly generate the corresponding character model. Additionally, users can continue to edit details such as skin, eyes, clothing, hairstyle, and body proportions in MetaHuman Creator.
Parameterized model-based digital human generation is also a research hotspot for AIGC in the field of digital human modeling. This method learns the commonalities of human structure through a large database of real human scans to construct a unified parameterized model, decoupling the originally complex human mesh space into limited parameter expressions. These parameters cover information such as height, body type, muscle definition, and facial features, and adjusting these parameter values can deform and customize the human model. The linear blend model SMPL[5] was realized in 2015 to represent and change human posture and shape with a small number of parameters. SMPL-X[6] introduced parameterized representations for faces and gestures based on this, and such parameterized models are widely used in human reconstruction tasks like ICON[7]. In addition to real humans, the RaBit[8] model explored parameterized models for cartoon characters, allowing for the personalization of 3D models of cartoon people, bears, rabbits, etc., by changing parameters, with its proposed SVR method capable of reconstructing cartoon models with the same appearance and posture from a single cartoon image.
(2) Intelligent Synthesis of Digital Human Voices
High naturalness and personalized voice synthesis are also important modules in digital human production. Compared to other sections of digital human production, voice AI synthesis technology has entered a mature stage and is widely used in broadcasting, television, and online audio-visual fields. Voice synthesis technology is one of the important branches in the AIGC field, capable of quickly converting text into audio, enabling computers to automatically generate high-quality voice audio in real-time.
In 2016, Google proposed WaveNet[9], which used dilated causal convolutions to solve the long-range temporal dependencies of speech, allowing the model to complete high-quality voice synthesis in a short time. In 2017, based on WaveNet, Google proposed the first end-to-end TTS voice synthesis model, Tacotron[10], releasing Tacotron 2 in 2018. Tacotron 2 consists of a spectrogram prediction network and a vocoder, where the spectrogram prediction network maps the input character sequence to a sequence of mel spectrogram frames, and the vocoder restores the predicted mel spectrogram frames back to waveforms[11]. In 2019, Zhejiang University collaborated with Microsoft to propose the FastSpeech model[12], a non-autoregressive model that has a faster generation speed and better voice quality compared to previous autoregressive TTS models. A year later, FastSpeech 2 was released, which can directly generate predicted audio waveforms from text, achieving a training speed three times that of FastSpeech[13]. In recent years, voice synthesis tasks have begun to shift towards expressive voice synthesis (Expressive TTS), which focuses more on how to synthesize the style, prosody, and emotion of speech compared to TTS. Because this type of training requires less annotated data, these methods often use unsupervised learning to decouple emotional and prosodic features from reference audio and then combine these features with text vectors to achieve controllable styles[14][15].
(3) Intelligent Driving of Digital Human Animation
In addition to appearance representation, the naturalness of body movements and the flexibility of facial expressions are crucial for the realism of virtual humans. Unlike the generation of virtual human images, animating digital humans requires not only smoothness and realism but also certain requirements for real-time interactive feedback. This kind of animation production is complex, with just a few minutes of animation requiring an experienced animator to spend hours. However, with the development and application of AIGC technology, AI algorithms have been iteratively updated to generate movements and expressions that comply with human biomechanics.
Real human-driven animation relies on motion capture technology. Motion capture technology can be divided into inertial motion capture, optical motion capture, and video-based motion capture. The industry typically uses optical motion capture, which utilizes arrays of cameras and sensors to record and track human movement data, but this method can be costly, deterring many. With the development of AIGC technology, low-cost video-based motion capture technologies have matured. Zhang proposed a real-time facial capture system that can use a fixed digital human head combined with any perspective of facial video input to achieve facial video outputs down to micro-expression levels[16]. The emergence of applications like DeepMotion, which are based on video for full-body motion capture, has significantly lowered the threshold for full-body motion capture. Users upload videos, and AI algorithms calculate the global positions and joint rotation information of the human body in the video, resulting in a corresponding skeletal animation sequence.
As motion capture technology and video content data have become more abundant, accumulating motion data has become easier, providing a substantial data foundation for AI algorithm-driven digital human animation. Currently, AI algorithm-driven digital humans mainly generate animations through text, music, and video in a cross-modal manner, classified into three directions: lip animation generation, facial animation generation, and body motion generation.
Lip animation can be classified into text-driven and voice-driven based on different inputs. Regardless of whether it is text features or voice features, there is a one-to-one simple mapping relationship between them and lip movements under specified language constraints, making it easy to learn this mapping relationship from data. Currently, such models have been widely applied in the industry.
Facial animation generation primarily involves the vector representation of Blendshapes corresponding to 3D models. So far, both domestic and international tech companies have made progress in the intelligent synthesis of digital human facial animations. For instance, the Craytalk technology developed by Reallusion, which generates facial expressions from voice, has been successfully commercialized in animation production. Domestic companies like Sogou and Xiangxin Technology have also implemented some projects.
Motion generation mainly refers to skeletal animations driven by sequences of actions or cross-modal information such as text, music, and video. The mapping relationship between this input and skeletal animations is many-to-many, meaning that the same input could correspond to various physical actions in real space. This presents two major challenges for 3D avatar animations: personalized motion generation and controllable motion generation. For example, in text-driven scenarios, users generate body movements based on a given text script, requiring feature extraction from the input text, and then using those extracted features to guide the motion generation module. The Human Motion Diffusion Model[17] utilizes CLIP to extract text description features, combining them with diffusion models to generate action sequences, while Action-GPT[18] adopts a self-regressive approach based on the GPT-3 architecture to generate action sequences, guiding the decoder to produce actions through highly detailed action descriptions. These models have achieved good short-term action generation results, but challenges like action freezing and blurriness occur when generating long-term action sequences, indicating that practical applications are still some distance away.
3.2 Application Prospects
With the support of AIGC technology, the mass generation and intelligent interaction of digital humans provide more possibilities for their application development in film production.
As the digital human production process is optimized and strengthened, the production of such digital assets will inevitably become lower in cost and more efficient[19]. Replacing real background actors with generated virtual digital humans is a feasible development direction for digital humans in film production. Firstly, compared to real actors, generated virtual digital humans possess high flexibility and controllability, allowing for real-time adjustments and control based on directorial needs. They can quickly switch between different scenes and roles without worrying about time constraints or contract issues. Production teams can adjust the number, appearance, and movements of virtual digital humans as needed to achieve better visual effects. Secondly, virtual digital humans perfectly solve human resource management issues related to real actors, reducing management and coordination work, and providing additional confidentiality advantages to avoid potential information leaks.
At the same time, increasingly intelligent “individualized digital humans” have also emerged as a new development stage for digital humans in film production following real digital doubles and virtually created characters. Individualized digital humans refer to those with complete personalities and consciousness, possessing the ability to think and act autonomously. They have human-like intelligence and consciousness, capable of independent thinking, learning, and action, equipped with agency (Agent) based on advanced AI technologies such as deep learning (DL), natural language processing (NLP), and neural networks[20]. In the increasingly interactive “engine films” and the continuous deepening of traditional film technologies, the application attempts of computer-driven digital humans have become a reality.
The early concept of individualized digital humans mainly existed in metaverse films, reflecting directors’ imaginations about the future relationship between artificial intelligence technology and humanity. Films like “Blade Runner,” “Terminator,” and “I, Robot” feature digital humans with independent consciousness. With continuous breakthroughs in fields such as natural language processing (NLP) and multimodal transformation, the intelligence of digital humans has significantly improved, and individualized digital humans no longer exist merely as concepts in film content but appear as real figures in the real world.
For instance, the sci-fi film “b,” produced by Bondit Media Capital, which was nominated for an Oscar for “Loving Vincent: The Mystery of the Starry Night,” features the AI robot Erica as the lead. The development team trained Erica for acting in the film. Erica plays an AI robot in the film, breaking the traditional mode of filming robots with special effects, garnering attention and discussion from audiences both domestically and internationally, becoming the first film starring an artificial intelligence. In 2022, a virtual digital human actor named Chun Cao was officially launched by Beijing Weiling Times Technology Co., Ltd. Supported by AI algorithms, Chun Cao can respond promptly to human commands and continuously learn during interactions with humans. As its CEO Song Zhen mentioned in an interview, “In addition to the game ‘Chun Cao Legend,’ directors can also interact with this actor directly and let her perform live.”
Thus, AIGC technology brings more possibilities for the application of digital humans in film production, including the mass generation of virtual digital humans replacing background actors and the interactive application of intelligent individualized digital humans. The advancements in these digital human technologies provide new opportunities for film creation and offer more creativity and potential for the future development of the film industry.
4 Conclusion
Digital humans, as key elements in film production, have become an indispensable part of the film narrative process. This study mainly introduces the current application status and future development trends of digital humans in the film industry, summarizing existing AIGC technologies in image generation, voice synthesis, and animation driving to provide some references for optimizing the production efficiency of digital humans in the film industry. Additionally, in terms of mass generation and intelligent interaction, it offers some insights into the innovative transformative applications of digital humans in the film industry.
As AIGC technology continues to develop and support, the application of digital humans will transcend their past roles limited to special effects, expanding into broader fields such as autonomous plot development and real-time emotional feedback. In the future, the further development of digital humans will bring more creativity and technological breakthroughs to film production, enriching the cinematic experience and leading the film industry into a new era of brilliance.
References
(Scroll down to read)
[1] Ma Xuyi, Li Xuesong. Exploration of Digital Double Workflow[J]. Modern Film Technology, 2021(9): 17-22.
[2] Naruniec J, Helminger L, Schroers C, et al. High-resolution neural face swapping for visual effects[C]//Computer Graphics Forum. 2020, 39(4): 173-184.
[3] Zoss G, Chandran P, Sifakis E, et al. Production-Ready Face Re-Aging for Visual Effects[J]. ACM Transactions on Graphics, 2022, 41(6): 1-12.
[4] Zhang Xue. The successful application of Weta Digital’s intelligent facial animation system in the film “Avatar: The Way of Water”[J]. Modern Film Technology, 2023(5): 63-64.
[5] Loper M, Mahmood N, Romero J, et al. SMPL: A skinned multi-person linear model[M]//Seminal Graphics Papers: Pushing the Boundaries, Volume 2. 2023: 851-866.
[6] Pavlakos G, Choutas V, Ghorbani N, et al. Expressive body capture: 3d hands, face, and body from a single image[C]//Proceedings of the IEEE/CVF conference on computer vision and pattern recognition. 2019: 10975-10985.
[7] Xiu Y, Yang J, Tzionas D, et al. Icon: Implicit clothed humans obtained from normals[C]//2022 IEEE/CVF Conference on Computer Vision and Pattern Recognition (CVPR). IEEE, 2022: 13286-13296.
[8] Luo Z, Cai S, Dong J, et al. RaBit: Parametric Modeling of 3D Biped Cartoon Characters with a Topological-consistent Dataset[C]//Proceedings of the IEEE/CVF Conference on Computer Vision and Pattern Recognition. 2023: 12825-12835.
[9] Oord A van den, Dieleman S, Zen H, et al. WaveNet: A Generative Model for Raw Audio[EB/OL]. (2016-09-12). https://arxiv.org/abs/1609.03499.
[10] Wang Y, Skerry-Ryan R J, Stanton D, et al. Tacotron: Towards End-to-End Speech Synthesis[EB/OL]. (2017-05-29). https://arxiv.org/abs/1703.10135.
[11] Shen J, Pang R, Weiss R J, et al. Natural tts synthesis by conditioning wavenet on mel spectrogram predictions[C]//2018 IEEE international conference on acoustics, speech and signal processing (ICASSP). IEEE, 2018: 4779-4783.
[12] Ren Y, Ruan Y, Tan X, et al. Fastspeech: Fast, robust and controllable text to speech[J]. Advances in neural information processing systems, 2019, 32.
[13] Ren Y, Hu C, Tan X, et al. FastSpeech 2: Fast and High-Quality End-to-End Text to Speech[EB/OL]. (2020-06-08)[2023-06-05]. https://arxiv.org/abs/2006.04558v8.
[14] Hu T Y, Shrivastava A, Tuzel O, et al. Unsupervised style and content separation by minimizing mutual information for speech synthesis[C]//ICASSP 2020-2020 IEEE International Conference on Acoustics, Speech and Signal Processing (ICASSP). IEEE, 2020: 3267-3271.
[15] BIAN Y, CHEN C, KANG Y, et al. Multi-reference Tacotron by Intercross Training for Style Disentangling, Transfer and Control in Speech Synthesis[EB/OL]. (2019-04-04).https://arxiv.org/abs/1904.02373.
[16] Zhang L, Zeng C, Zhang Q, et al. Video-driven neural physically-based facial asset for production[J]. ACM Transactions on Graphics (TOG), 2022, 41(6): 1-16.
[17] Tevet G, Raab S, Gordon B, et al. Human Motion Diffusion Model[EB/OL]. (2022-09-29)[2023-06-05].https://arxiv.org/abs/2209.14916.
[18] Kalakonda S S, Maheshwari S, Sarvadevabhatla R K. Action-GPT: Leveraging Large-scale Language Models for Improved and Generalized Zero Shot Action Generation[EB/OL]. (2022-11-29)[2023-06-05].https://arxiv.org/abs/2211.15603.
[19] Achenbach J, Waltemate T, Latoschik M E, et al. Fast generation of realistic virtual humans[C]//Proceedings of the 23rd ACM Symposium on Virtual Reality Software and Technology. Gothenburg Sweden: ACM, 2017: 1-10.
[20] Song Lei Yu. From “Doubles” to “Individualization” – The Type and Aesthetic Shift of Digital Humans in Metaverse Films[J]. Contemporary Film, 2023(2): 151-157.
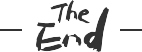
Supervisory Unit: National Film Administration
Organizing Unit: Film Technology Quality Inspection Institute
Publication Number: CN11-5336/TB
Standard International Serial Number: ISSN 1673-3215
Submission Email: [email protected]
Official Website: www.crifst.ac.cn
Advertising Cooperation: 010-63245082
Journal Distribution: 010-63245081