Li Zi from A Fei Si Quantum Bit | WeChat Official Account QbitAI
”Master, can you help me Photoshop my face onto the model in the street photo? Oh right, not just that, it has to look like the model…”
Extracting an element from one photo and seamlessly blending it into another seems like a daunting task. After all, it can easily result in a bizarre collage effect, whether done manually or through AI calculations.
And if “the other image” refers to a human painting, it becomes even more challenging.
However, researchers from Cornell University and the masters at A Dou Bi have produced an algorithm that can integrate various objects into paintings without any noticeable Photoshop traces.
A large number of artists’ hard work, even the artists themselves, have fallen victim to it:
△ What do you want a bicycle for?
△ Mona Lisa Bean
△ Picaboo
△ Bought you a biao
△ Fan · Cai Kangyong · Gao
What kind of algorithm is so insane?
Remember this name: Deep Painterly Harmonization.
In the eyes of the researchers at Cornell and the Photoshop masters of A Dou Bi, existing global style transfer algorithms are too weak.
They can indeed handle the style transfer of an entire photograph. Placing a painting and the photo it influences together might not reveal significant issues.
△ Introducing, this is my new gear
But to seamlessly blend Captain America’s shield into the work of Italian painter Onofrio Bramante, even slight differences become very apparent. The performance of global style transfer is also relatively modest (as shown on the left).
Whether it’s removing boundary lines, matching colors, or refining textures, it’s challenging to make the pasted part retain the original style of the painting.
We Are Different
Thus, this group of masters felt they needed to build a neural network for local style transfer.
The big strategy is to transfer the statistical features of the painting-related parts (neural response) to the corresponding positions of the foreign objects — the key lies in the selection of what should be transferred.
△ Gatys has a geometric cat
The team built a two-pass algorithm based on the style transfer techniques published by the Gatys team, and additionally introduced some style reconstruction losses to optimize the results.
Come, let’s take a step-by-step look at the algorithm details.
First Step (First Pass): Coarse Image Coordination (Single Scale)
Roughly adjust the color and texture of the foreign elements to correspond with semantically similar parts in the painting. For each layer of the neural network, find the nearest neighboring neural patches and match them with the neural response of the pasted part.
△ Benedict Cumberbatch Lisa, enchanting eyes
Take a step back, the sea is vast and fish leap. There’s no need to be too entangled with image quality at this stage, as sacrificing quality to some extent allows the team to design a powerful algorithm adaptable to various painting styles.
Using the Gram matrix to calculate style reconstruction losses can optimize the roughly coordinated version.
△ The consequences of not considering style loss
What we obtain here is an intermediate product, but the style is already very similar to the original painting.
Second Step (Second Pass): High-Quality Refinement (Multi-Scale)
With the foundation of the first step, it is only natural to raise strict quality requirements for the image at this point.
In this step, we first need to concentrate fire on an intermediate layer responsible for capturing texture attributes, generating a correspondence map to eliminate spatial outliers.
△ You sink into my yellow canvas
Then, upsample the correspondence map with spatial consistency and enter the more refined layers of the neural network. This ensures that for each output position, all neural responses across scales come from the same position in the painting.
As a result, the image can have a more coherent texture and appear much more natural.
Post-Processing
After the Two-Pass algorithm, the image quality is nearly impeccable at a medium scale; however, there may still be inaccuracies at finer scales. In other words, it can be viewed from a distance but not up close (fill in the blank: 10 points).
Thus, post-processing also requires two steps:
Chromatic Noise Reduction
The phenomenon of high-frequency distortion mainly affects the chromaticity channel, with little impact on brightness.
Upon discovering this characteristic, the team converts the image into the CIELAB color space; then, using the Guided Filter, they filter the ab chromaticity channel with the brightness channel as a guide.
△ Work or life, that is the question
This method effectively improves the situation of high-frequency color distortion, but it may expose larger loopholes. Then…
Patch Synthesis
This leads to the second step, ensuring that each output patch appears in the image. Using PatchMatch, each patch is matched with a similar patch. Then, the average value of all overlapping patches is taken to reconstruct the output, ensuring no new content emerges in the image.
However, the side effect here is that it softens details, so we need to use the Guided Filter to separate the image into a Base Layer and a Detail Layer to weaken the softening effect.
△ The painting style tortures me thousands of times, I choose to dog lead
Local Transfer Effectiveness
Experimental results show that transferring a set of regional feature statistics is much more effective than transferring many independent location feature statistics. The correspondence map generated by the neural network has been very helpful.
The local style transfer algorithm ensures spatial consistency and cross-scale consistency in a statistical sense. Achieving seamless puzzle quality, these two “consistencies” are remarkable.
△ The eightfold life of the Golden Arches
In the future, puzzle enthusiasts may probably abandon global style transfer algorithms. Looking at the local, your imagination can flourish brutally.
However, compared to the barely noticeable invasion, I still prefer this 360-degree all-dead-angle mesmerizing Photoshop.
△ The omnipotent copy, the omnipotent paste
For more thrilling papers, visit:
http://www.cs.cornell.edu/~fujun/files/painting-arxiv18/deeppainting.pdf
The authors of this paper include: Fujun Luan, Sylvain Paris, Eli Shechtman, Kavita Bala, among others. They have also open-sourced the code, where you can see more shocking examples:
https://github.com/luanfujun/deep-painterly-harmonization
By the way, the first author, Fujun Luan, graduated from Tsinghua University in 2015 and is currently pursuing a PhD at Cornell University. He has interned at Facebook, Face++, Adobe, and other companies.
The artistic photo boy has been exploring the mysteries of vision.
For instance, the Deep Photo Style Transfer, once hailed as the “next generation PS,” is also a research achievement of Fujun Luan. Deep Photo can achieve pixel-level style transfer.
It’s also very cool.
One More Thing
If you are temporarily unable to visit GitHub, we have also organized a small AI master Photoshop exhibition. In the Quantum Bit WeChat official account (ID: QbitAI) dialogue interface, reply with “master” to start the tour.
— The End —
Sincerely Recruiting
Quantum Bit is recruiting editors/reporters, located in Zhongguancun, Beijing. We look forward to talented and passionate students joining us! For details, please reply with “Recruitment” in the Quantum Bit WeChat official account (QbitAI) dialogue interface.
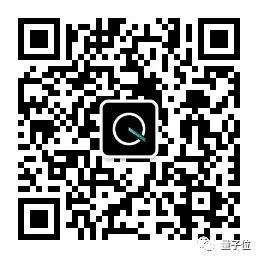
Quantum Bit QbitAI · Headline Author
վ’ᴗ’ ի Tracking new developments in AI technology and products