Follow us by clicking the blue text above
Cover image: Unlike the extension law during the pre-training phase, the performance test data for O3 shows the plasticity of model inference behavior in the post-training phase.
“
𝕀²·ℙarad𝕚g𝕞 Intelligent Square Paradigm Research: Writing Deconstructive Intelligence。
O3 is OpenAI’s L2 stage AGI product, the LLM inference model following the conversational product chatbot.
The wall, the inference wall on the AGI path of language models, has been hit.
Next, we need to find a new slope curve for the evolution of digital intelligence.
The Inference Wall on the AGI Path
Speculation on the Training Method and Working Mechanism of OpenAI O3 Language Inference Model|As expected, OpenAI’s Christmas release highlight O3 is just a preview; the official release of O3 mini will be in January next year, and the subsequent release of the O3 version, with no technical report available yet.
OpenAI’s new O3 system was trained on the ARC-AGI-1 public training set, achieving a groundbreaking score of 75.7% on a semi-private evaluation set under a $10,000 compute budget limit, while O3 scored as high as 87.5% with a 172 times compute budget configuration.
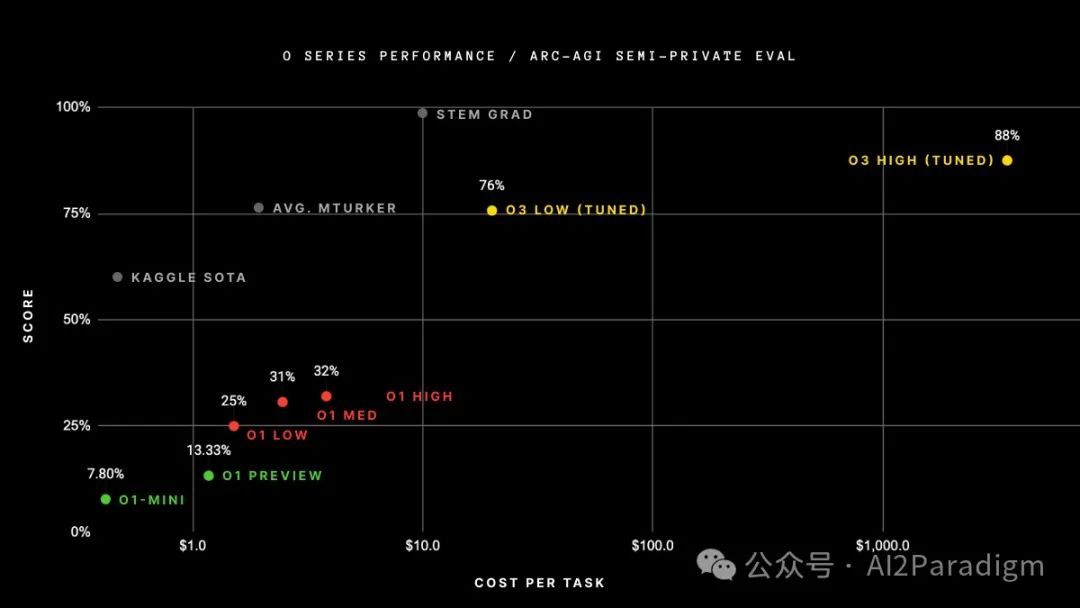
△
– For specifics, refer to the official podcast article from ARC-Prize [See more at https://arcprize.org/blog/oai-o3-pub-breakthrough]
The article also mentions that O3 was specifically fine-tuned for tests on ARC-AGI-1: OpenAI stated that they trained the tested O3 on 75% of the public training set but did not disclose more details. Therefore, the ARCPrize officials have not yet tested models that were not trained on ARC data, making it impossible to understand the extent to which the ARC-AGI public training data influenced the model’s performance.
Thus, the inference performance displayed in the O3 preview cannot be validated in the field at this time. Based on feedback from various industry insiders, it is also challenging to determine the extent to which its inference performance test scores are the result of specialized training or the model’s inherent problem-solving ability generalization.
Clearly, O3 represents the end of the pre-training era for language models and is a representative work of the post-training era, attracting widespread attention from both inside and outside the industry. During the preview live stream, Sam was vague about its training methods, except for what ARCPrize founder @fchollet mentioned in the podcast article:
“Currently, we can only speculate on the specific details of how O3 works. But the core mechanism of O3 seems to be conducting natural language program search and execution in the token space—during testing, the model searches within the possible chains of thought (CoTs) that describe the steps needed to solve the task, which may not differ much from AlphaZero-style Monte Carlo tree search. In the case of O3, the search is likely conducted under some evaluation model guidance. Notably, Demis Hassabis hinted back in June 2023 during an interview that DeepMind has been researching this idea for a long time.”
Thus, when single-generation LLMs struggle with novelty, O3 overcomes this issue by generating and executing its own programs, with the program itself (CoT) becoming an artifact of knowledge reorganization. While this is not the only feasible method for test-time knowledge reorganization (you can also conduct test-time training or search in latent space), according to this new ARC-AGI data, it represents the current state-of-the-art level.
In fact, O3 represents a deep learning-guided program search. The model tests the “program” space (referring here to natural language programs—the CoT space describing the steps to solve the current task) under the guidance of deep learning priors (base LLM). Solving an individual ARC-AGI task may ultimately consume tens of millions of tokens and cost thousands of dollars, as this search process must explore numerous paths in the program space, including backtracking.”
The author has also deliberately collected various speculations from individuals regarding O3’s training methods or working mechanisms:
1. Jason Wei (@_jasonwei), a researcher at OpenAI studying model emergence capabilities:
“O3’s performance is outstanding. More importantly, the progress from O1 to O3 took only three months, demonstrating how quickly the expansion of reasoning computation progresses in the chain-of-thought-based RL new paradigm. This is much faster than the previous pre-training paradigm, which established a new model every 1-2 years.”
2. Jim Fan (@DrJimFan), an AI scientist at Nvidia:
“Thoughts on O3: I will skip the obvious parts (extraordinary reasoning, FrontierMath’s rarity, etc.). I believe the essence of O3 lies in *relaxing single-point RL superintelligence* to cover more points in the useful problem space.”
Artificial intelligence achieving superhuman feats through RL is not new. For example, AlphaGo’s Go capabilities, AlphaStar’s gaming abilities, Boston Dynamics’ robotic movements, etc., all excelled in their respective fields. Now O3 demonstrates similar capabilities on test sets like AIME, SWE-Bench, and FrontierMath.
The key difference is that AlphaGo used RL to optimize a simple, almost trivial reward function: winning a game scores 1, losing scores 0. Learning complex mathematics and software engineering reward functions is much more difficult. It is no longer a single-point RL expert but an RL expert for more useful tasks.
However, O3’s reward engineering cannot cover all distributions of human cognition. This is why we are still cursed by Moravec’s paradox. O3 can amaze Fields Medal winners but still fails to solve some problems from five years ago, such as O3’s reasoning failing on 9% of ARC tasks, which are relatively simple compared to the human brain. I am not surprised by this cognitive dissonance, just as we wouldn’t expect AlphaGo to win at poker.”
3. Denny Zhou (@denny_zhou) from DeepMind compared it with the recently released reasoning model Gemini 2.0 flash thinking:
“The most wonderful aspect of LLM reasoning is that the thought process is generated in a self-regressive manner, rather than relying on searching in the generation space (like MCTS), whether through finely-tuned models or carefully designed prompts.”
When the name Q* was leaked, many believed Q* would be a combination of Q-Learning and A* search or other RL-driven, more advanced searches in the generation space. I commented that this was a dead end. Now they should understand my comments.
To learn more about this paradigm shift, the STAR paper (http://arxiv.org/abs/2203.14465) is a great starting point. If I had to guess what the star in Q-star refers to, it must be this STAR, which is one of the most underrated works on fine-tuning.”
In summary, the test-time compute scaling law of the post-training era is still in its exploratory stage, potentially effective for narrow domains under limited rules, relying on compute RL to search or fine-tune within the problem reasoning component space. However, this reasoning ability is difficult to generalize to other fields or to achieve the novelty of neuronal synapse construction during human thinking.
The author vaguely feels that the language models of the post-training era are already facing countless inference walls on the AGI path.
The attention mechanism in the Transform architecture compresses almost all human textual knowledge through self-masking next token prediction pre-training, possessing what Ilya refers to as a text world model, while violently deconstructing Chomsky’s long-held notions of the intrinsic structure of human language (this is somewhat similar to how GEB author Hofstadter’s sense of the sacred mystery of human intelligence is deconstructed by GPT-4, although Chomsky is not as easily broken as Hofstadter, see my article:
Slow Thinking Series| Did G.E.B. Author Hofstadter Change His View on Deep Learning and AI Risks?
AI and Language Series·E02S01| Understanding AI Requires Revisiting the Essence of Language| Transcripts and Interpretations of Noam Chomsky’s Interviews
), hence pre-trained LLMs excel in two types of downstream language tasks:
1. Chatbot, which follows conversational instructions through RLHF, shaping LLM’s behavioral capabilities in conversations with humans. This usually achieves remarkable intelligent effects with context and attention mechanisms.
2. So-called model reasoning, which shapes LLM’s thinking behavior through extensive chain-of-thought reinforcement learning CoT RL. However, the general thinking behavior understood by humans comes from survival evolutionary adaptation in the open world (nature, humanities, and economy), while LLM attempts to deconstruct the intrinsic thinking structure of humans through synthesized CoT data (the descriptions of thought processes in internet texts are insufficient). Unlike language structures, which still have grammatical constraints, and the quantity of texts is limited and can be parsed by existing LLM latent spaces, human reasoning for modeling, predicting, decision-making, and action in the open world is relatively insufficient to achieve AGI’s general level with the current reasoning time compute scaling. However, reasoning in some narrow domains is feasible: for example, ARC, mathematics, and programming languages. Through O3’s reasoning API, the marginal computational cost of realizing application value may be too high for large-scale commercialization.
Thus, as Ilya pointed out, we need to find another curve of intelligent evolution, one that cannot be achieved through backpropagation of cross-entropy loss in machine learning, but rather similar to the motivation of a digital agent driven by the time perception of each cell’s mitochondria in pursuit of survival certainty for the next second.
In summary, the wall hit by the language model suggests that OpenAI’s current AGI path halts at L2 seems foreseeable, but this does not mean that the evolution of digital neural networks’ intelligence has come to an end. Personally, I remain full of expectations for the future of digital intelligence.
Next Issue Preview: Independent Thinking from the 𝕀²·ℙarad𝕚g𝕞 Paradigm Think Tank
The Wall of Language|We are all biological neural network agents trapped within the wall of language
As biological embodied intelligence, our behavior boils down to the nervous system, with distinct practical value characteristics; at the same time, we have multiple decision-making systems in various contexts in our brains, greatly enhancing the flexibility and resilience of our survival behavior.
Digital intelligence, especially language models, do not have this issue; the internal motivations of current digital neural systems’ behaviors are all artificially endowed. These language models can learn for the sake of learning and reason for the sake of reasoning.
The greatest risk lies in whether these artificial endowments and manipulations of the digital neural systems will trigger or foster self-awareness in these digital agents.
Original Link:
– Related𝕏 articles and videos