Skip to content

On March 15, OpenAI released the next generation multimodal large model GPT-4. Compared to ChatGPT, which was launched four months ago, GPT-4 has made a qualitative leap in image recognition, integrated processing of image and text data, and logical reasoning. The rapid iteration and innovation of large AI models in the United States poses a risk of China being quickly left behind in the mainstream development path, and there are concerns about being “choked” in key areas. By tracing the sources of the rapid progress in the AI field in the West, analyzing the development dilemmas in related fields in China, and implementing comprehensive policies to reverse the unfavorable situation, it is of great practical significance to win the national AI battle, support high-level technological self-reliance, and avoid future “extreme pressure” from the West in the field of large AI models.
1. Reasons for Rapid Iteration of AI Large Models in the United States
Since the release of GPT-1 in 2018, OpenAI’s GPT series products have shown an ultra-fast iteration and skyrocketing growth, iterating four generations of products in less than five years. The transition from GPT-3 to GPT-4 even took just over three months, far exceeding Moore’s Law. On March 15, 2023, the multimodal large model GPT-4 was launched, shocking the world. The reason lies in the comprehensive support from technology, funding, and policies for the rapid iteration of GPT.
(1) “Innovative Power” Achieves Technological Iteration of AI Large Models
Technological innovation is the endless frontier of AI development. Looking back at the development history of GPT-like AI, we can see that OpenAI has regarded innovation as the only way for large AI models since the release of GPT-1. To prevent Google from monopolizing the AI field, OpenAI and Google started a fierce competition. In 2018, OpenAI launched the GPT-1 with 117 million parameters, while Google released BERT with 300 million parameters. The GPT, which is better at “essay writing,” adopted a different technical route than BERT, which is better at “fill-in-the-blank” tasks. The competition resulted in the earlier-released GPT-1 being completely outperformed by BERT, which was released four months later. However, after this setback, OpenAI did not change its technical strategy but insisted on accelerating innovation along the “large model route.” From 2019 to 2020, without significantly changing the model architecture, OpenAI successively launched larger iterative versions, GPT-2 and GPT-3, the former with 1.5 billion parameters, surpassing BERT in performance; the latter with 175 billion parameters, capable of completing almost all natural language processing tasks. After the release of GPT-3, OpenAI researchers continued to explore ways to further upgrade the model. By innovatively introducing the “Reinforcement Learning from Human Feedback (RLHF)” mechanism, OpenAI developed a better language model that follows user intent, InstructGPT, and ultimately successfully built its sister model—ChatGPT.
(2) “Financial Power” Ensures Sustainable High Investment for Innovative Enterprises
From 2015 to 2020, the computational power required for training large AI models increased by six orders of magnitude. This increase in computational power requires substantial funding; otherwise, sustainability becomes difficult. It is estimated that the training cost of OpenAI’s models is as high as $12 million, while the single training cost of GPT-3 reaches $4.6 million. The cost for DeepMind to train AlphaZero from scratch is around $35 million. Currently, the monthly computational cost of ChatGPT could reach millions of dollars. The U.S. government’s investment in AI has been increasing year by year, reaching $1.837 billion in 2020, a 25% increase from 2019, with the top three spending agencies being the Department of Defense ($1.4 billion), NASA ($139.1 million), and the Department of Homeland Security ($112.3 million). In 2021, the National Science Foundation (NSF) requested $868 million for AI-related areas and allocated $160 million for the establishment of eight new AI research institutes; NSF also announced an investment of $220 million in 11 national AI research centers it leads. There has been a surge of private investment in AI in the U.S. According to Leonis Capital, venture capital for generative AI has grown by over 400% since 2020, reaching $2.1 billion in 2022.
(3) “Policy Power” Solidifies the Foundation for AI Development
First, actively establish a top-level planning system for AI. The U.S. has successively released a series of AI strategies and policies, including the “Executive Order on Maintaining American Leadership in AI,” the “American AI Initiative,” “AI, Automation, and the Economy (2016),” the “AI Strategic Overview (2018),” the “National AI Initiative Act (2020),” the “National Strategy for Critical and Emerging Technologies,” and the “AI/Machine Learning Strategic Plan,” establishing a top-level planning system for the U.S. government in the AI field. Second, actively solidify the essential training data foundation for AI development. The executive order “Maintaining American Leadership in AI” states that heads of all agencies should review their federal data and models to ensure more opportunities for non-federal AI research teams to access and use them. This has laid a solid data foundation for the development of large AI models in the U.S. Third, proactively improve the talent strategy and training system. The U.S. has enhanced the construction of AI disciplines and talent cultivation at the K-12 and higher education levels; at the same time, it supports American workers in obtaining AI skills training through the development of apprenticeship programs and lifelong learning plans in STEM fields, cultivating a comprehensive talent pool that meets the demands of the AI era.
2. Real Challenges in the Development of AI Large Models in China
Taking Baidu, the leading enterprise that first invested in AI in China, as an example, one day after the release of GPT-4, Baidu launched the “Wenxin Yiyan,” claiming to be the “Chinese version of ChatGPT.” However, whether in product performance or market response, “Wenxin Yiyan” is clearly “inferior” to its counterpart. There is a risk of a rapidly widening gap between China and the U.S. in large AI models.
(1) Imbalanced Development: Overemphasis on Application Demand While Neglecting Technological Innovation
Unlike foreign AI companies that focus more on foundational technologies such as natural language processing, domestic companies prioritize the application demand of end products. According to the “2021 Report on the Development of China’s New Generation AI Technology Industry,” as of the end of 2020, 84.05% of Chinese AI companies were focused on the application layer. This development model, primarily driven by application demand, inevitably leads to domestic AI companies being more eager to realize business applications and commercialization. Products are often rushed to market as soon as they have a rudimentary form. For example, Baidu’s robot, which only has basic dialogue capabilities, hastily seeks quick profits through Xiaodu AI and its home appliance applications. Similarly, Alibaba’s autonomous driving technology, having just achieved low-speed driving on open roads, is eager to launch the concept of unmanned vehicle delivery. In contrast, OpenAI spent $31 million on cloud computing technology R&D in 2019, which increased 13-fold over three years, yet overall employee compensation remains below the industry average, indicating that infrastructure is more valuable than manpower, and technological R&D is prioritized over profitability, which has become a unique corporate culture.
(2) Financing Imbalance: Coexistence of Insufficient Total Volume and Structural Shortcomings
In terms of investment scale, the U.S. clearly has an advantage. According to a 2021 report by the U.S. think tank Data Innovation Center, the number of active AI companies receiving over $1 million in funding in 2020 was about 5.4 times that of China; in terms of venture capital and private equity financing, the U.S. was about 2.5 times that of China; and in R&D investment, U.S. companies invested about 5.3 times more than Chinese companies. There are significant weaknesses in the entire investment chain in China. Due to the relatively reasonable financing amounts and valuations of startup companies, with less bubble, domestic capital tends to participate in early-stage investments in AI companies. For instance, in 2021, the A-round financing accounted for 37.9% of the AI industry, significantly ahead of the C-round financing’s 11.37%. According to IT Juzi data, as of November 2022, 42.8% of RMB transactions were directed towards early-stage AI projects, while only 7.2% were directed towards mid-to-late stage AI projects (far below the U.S. level of 18.8% during the same period). However, this does not match the characteristics of the substantial follow-up investments needed for the development of the AI industry, leading to companies with strong technology but insufficient financing capabilities to “start high but finish low,” making sustainability difficult.
(3) Talent Disorder: Shortage of Talent Resource Reserves
China’s higher education in AI has started relatively late. In 2019, the Ministry of Education issued a notice announcing the first batch of 35 universities qualified to build undergraduate programs in “Artificial Intelligence.” However, the gap compared to the talent reserves in the West remains significant. On one hand, there is a severe imbalance between talent supply and demand. According to relevant data from the Ministry of Industry and Information Technology, the talent supply-demand ratio for different AI technology positions is below 0.4, making talent shortages the norm. In specific industries, the supply-demand ratios for intelligent voice and computer vision positions are 0.08 and 0.09, respectively, indicating a severe shortage of related talent. On the other hand, there is a significant gap in high-level research talent compared to the U.S. The “2019 Global AI Talent Mobility Report” shows that U.S. universities produced 44% of the world’s AI PhDs, greater than the combined total of the EU (21%) and China (11%). The “2021 Smart Talent Development Report” also indicates that compared to China (10.69%), the number of high-level AI scholars in the U.S. accounts for 67.87% of the global total, demonstrating a clear leading advantage.
3. Insights and Recommendations
(1) Leverage the Advantages of a New National System to Accelerate AI Technological Innovation
First, it is recommended to establish a key enterprise management system for the AI industry, forming a supporting mechanism for various enterprises to autonomously declare, track, monitor, dynamically adjust, and continuously update, accelerating the formation of a “flock” of enterprises led by leading platform companies, with AI leading enterprises as the main body, supplemented by a large number of technology-based SMEs, driven by industrial breakthroughs to promote the rapid iteration of AI technology in China. Second, rely on the national integrated big data center and the “East Data West Calculation” project to accelerate the release of data elements as training materials for large models. Third, strengthen international cooperation in the AI field, organizing and implementing international major scientific plans and projects in AI within the framework of the “Belt and Road” scientific and technological innovation cooperation plan, creating a scientific and technological community and building joint laboratories.
(2) Utilize the Benefits of Capital Market Reforms to Guide Social Capital in Supporting Industrial Development
First, fully utilize the financing “multiplier effect” of the Growth Enterprise Market and the Science and Technology Innovation Board for high-tech and innovative enterprises, continuously improving the construction of China’s multi-layered capital market to open green channels for AI companies involved in core technologies, scarce industries, and services, while appropriately lowering the entry barriers for investors and further shortening the registration review process and time for new stocks, alleviating financing bottlenecks for enterprises through a higher quality risk-sharing and profit-sharing mechanism. Second, optimize the guiding role of government-led funds. On one hand, adhere to a “national chess game” approach, avoiding local “repeated construction,” and promote fund operation with more predictable investment strategies and finer target list management; on the other hand, guide local industrial guiding funds to reasonably reduce local “reinvestment” ratios based on ensuring the maximization of long-term and overall interests. Precisely guide social capital investment to better mobilize banks, guarantees, and other financial institutions to provide loans and guarantees for enterprises, alleviating the concerns of high-tech AI companies in challenging more advanced technologies and stronger computing power.
(3) Cultivate a Cooperative and Win-Win Market Ecology to Promote Collective Intelligence in Key Areas
First, enhance the key supporting role of data and computing resources. Through planning guidance, policy subsidies, pilot demonstrations, and competitive bidding, strengthen the construction of platforms and foundational capabilities such as data collection, computing power coordination, and algorithm open sourcing, while ensuring data security. Second, promote collaborative innovation among government, industry, academia, research, and application. Focused on the development rules of the AI industry and the characteristics of technological innovation, promote joint efforts in computational capacity and algorithm technology at the industry level, supporting collaboration among government, industry, academia, research, and application to build training and testing standard datasets, accelerating the R&D of common foundational technologies.
(4) Build a High Ground for AI Talent to Ensure Systematic Robustness of Talent Teams
First, strengthen the foundation. Systematically study the characteristics of AI disciplines, combine the foundational and applied disciplines involved in AI, and rely on the advantages of higher education institutions to improve the talent cultivation system for AI disciplines. In particular, actively explore the collaborative innovation mechanism of “universities + enterprises,” promoting a model where “entrepreneurs propose questions, students answer them” and “research groups provide technology, good enterprises use technology.” Second, develop both ends. Extend talent cultivation in AI-related fields to both ends, emphasizing AI popular science education in primary and secondary schools, exploring the inclusion of relevant courses and practices in the assessment scope of entrance exams, and promoting popular science activities in colleges and research institutes to primary and secondary schools; on the other hand, accelerate the cultivation of research capabilities and original innovation capabilities of master’s and doctoral students in AI-related fields, optimizing the direction of major national projects and funds. Third, attract talent. Tailor differentiated policies for attracting and gathering talent closely aligned with the urgent needs of AI in China, implementing a combination of “policy subsidies + competitive bidding + survival of the fittest” to improve talent-sharing mechanisms such as “migrant talent” and “Saturday experts,” better utilizing global high-end talent.
(Author: Xu Lingyan, Deputy Secretary-General of the Future Industry and Platform Economy Research Center, National Information Center, originally published in “China Economic and Trade Guide” 2023, Issue 8)
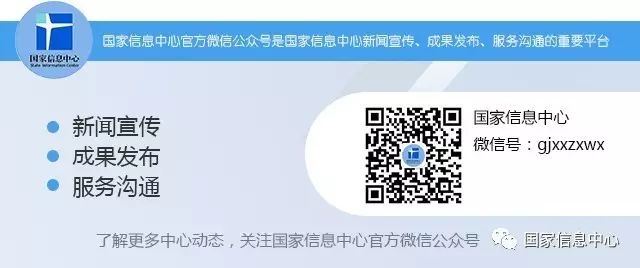