TL;DR
-
Public perception of AI in 2023: What is it? -> It doesn’t seem that powerful -> It doesn’t really concern me.
-
Currently, there are no hard limitations preventing the emergence of AGI, and we are only a few years away from it.
-
The “imagination” and “pleasing ability” of large models mature earlier than their “logical ability”.
-
There are no unicorns at the application layer; the biggest enemy for entrepreneurs is the official GPT.
-
Accelerated iteration of technology is the norm; we cannot wait for “technological stability” to take action.
-
The explosion of multimodal capabilities: once again proving the generational superiority of AGI over “narrow AI”.
-
Agents have not yet emerged; memory remains a bottleneck; the core issue is the lack of a “human model”.
-
Deep compression is the core capability of large models, bringing us closer to edge intelligence.
-
The AI ecosystems of China and the US are developing independently, and the technological gap did not narrow in 2023.
Image: “Explosion of AGI”, with Dall-E
[Author] Lian, MKIntroduction: Overestimating the Short-Term and Underestimating the Long-Term
2023 was a year of explosive development for large models: from the stunning debut of ChatGPT in November 2022, to GPT-4 in March 2023 as “the first contact with AGI (Artificial General Intelligence)”, to the full explosion of multimodal large models by the end of 2023, and to the recently released Sora that shocked the world again. Large models have brought the world many surprises, astonishments, confusions, and even fears.
Someone asked me: “What discovery/invention can the emergence of AGI be compared to in human history?”
“Probably the moment humans started using ‘fire’. Universal gravitation and iPhones are not worth mentioning.”
As I wrote in an article last April – at the emergence of AGI and any new thing: we tend to overestimate its short-term impact but underestimate its long-term potential. (Previous article: “AGI | Overestimating the Short-Term and Underestimating the Long-Term”) This is a true reflection of the current trend:
-
Short-term: AGI has not immediately spawned a large number of “star apps” and “monetization machines”. Only a few apps like ChatGPT and Character.ai have achieved user breakthroughs. Many upper-layer application apps are like weeds: not only do they bloom for a short time but are quickly replaced by OpenAI’s official offerings, and they also fail to break even on costs. As a result, investors are extremely cautious, and the public is gradually becoming numb to AI.
-
Long-term: Stable and accelerated iteration of technology. Many technologies predicted in March 2023 have made significant progress by now: video generation, audio generation, agents, memory capabilities, and model miniaturization… They may still have various issues before commercial use, but breaking through this layer of glass is just a matter of time.
One day in the human world equals ten years in AI. This lengthy article serves as a snapshot of the infant stage of AGI; it seeks to find an anchor point in the turbulent 2023 and aims for a better start in 2024.
-
Part One: Reviewing the major events of AI in 2023, outlining the main threads of the AI circle in 2023.
-
Part Two: Making some presumptuous predictions for 2024, providing some insights and ideas.
Here, I will not delve too much into technical details; rather, I will examine this technological tsunami from a business and individual perspective. The article contains many presumptuous estimates, as well as many inaccuracies and incompleteness; everyone is welcome to chat with me and share your thoughts. Additionally, this is my new public account, where articles related to AI will be concentrated. I kindly ask everyone to bookmark, star, like, and share.
Image: “Explosion of AGI – with some order”, with Dall-E
Part One: 2023, Prelude to the Storm<1> The Road to AGI: World Models, Emergence, and Self-Evolution
World Models: The Wolf Is Really Here. This revolution initiated by ChatGPT is fundamentally different from the previous “AI boom”. If previous AIs were merely “tools”, this time AGI is the “brain”. This distinction arises not only from the model’s purpose but also from the underlying data volume. OpenAI has always advocated for the “brutal aesthetics” of data volume and computational power; GPT was designed from the start to become a “world model”, meaning compressing all human knowledge into the model to achieve “omniscience and omnipotence”. Even the reason for using video and image data is “simply because there is more data” — Ilya Sutskever (former chief scientist of OpenAI, father of GPT).
From the perspective of data volume, large models are approaching the scale of all human data. Rumors suggest that GPT-5 will appear in 2024, and it should be “close to AGI”. However, regardless of GPT-5’s true capabilities or its release timing, it will likely be shrouded in a layer of “political correctness”, as neither the public nor the government is prepared to welcome the arrival of AGI.
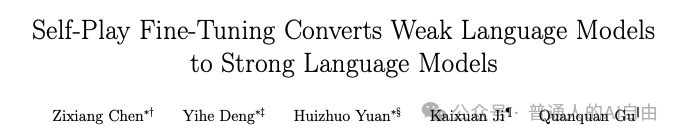
Data Sources:
https://arxiv.org/pdf/2211.04325.pdf
https://lifearchitect.ai/gpt-5/
Of course, GPT-5 should not only improve in data volume compared to GPT-4 but also in data quality, compression efficiency, and expressive capability. If we refer to the recent progress in model miniaturization: Mistral7B (7B parameters) can rival the capabilities of GPT-3.5 (175B parameters), then the improvement of GPT-5 over GPT-4 should not merely be a multiple of parameter volume.
Emergence: The Brain May Be Simpler Than We Imagine. One important characteristic of large models is “emergence”, meaning that large models can spontaneously acquire abilities that were not previously trained. For example, the single-modality GPT-3.5 trained solely on text can still exhibit certain spatial image capabilities. The term “emergence” originates from research in complex systems/chaos/brain science, referring to a system spontaneously developing structured rules after reaching a certain level of complexity – self-organization. Thus, a profound question arises: Is “intelligence” merely a phenomenon of the “emergence” of the human brain structure?
Following this line of thought, if we make a somewhat forced assumption: model parameters are roughly equivalent to neural connections; then the model still has a gap of two orders of magnitude (1.8 Trillion vs. 100 Trillion) to reach human brain levels. At the current rate of development, it would only take 2-4 years to bridge this gap. If the assumption that “emergence” equals “intelligence” holds, then by that time, the physical foundation for artificial intelligence to surpass human intelligence will be in place.
Of course, we still find it difficult to directly compare models to the brain. One obvious fact is that the brain can achieve far superior capabilities to large models with only a small amount of data for training. I believe this indicates that there is significant room for iteration in model structure; this is also why smaller models can achieve comparable results to larger models.
Self-Evolution: Training with Synthetic Data. When models require all of humanity’s data for training, they will quickly encounter a ceiling in terms of data acquisition and cost. However, after several months of practice, we can basically confirm that the data bottleneck does not exist: because model training can utilize synthetic data and continuously improve performance. A specific example is that almost all models on the market use synthetic data generated by ChatGPT for training, and recent research has found that generating synthetic data through “self-play (SPIN)” can continuously enhance model capabilities. True – left and right self-play!
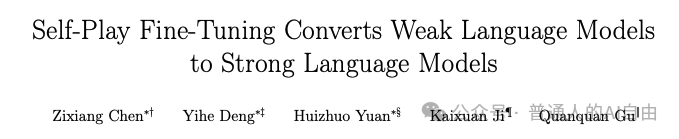
Paper Address: https://arxiv.org/abs/2401.01335v1
Compared to the brain, current models utilize far more data than the human brain can process, yet there is still a gap in effectiveness. Thus, the most natural viewpoint is that the model has not fully utilized this data. We can consider “self-play” as a way for models to continuously refine data, approaching brain capabilities. Moreover, a bolder hypothesis is that “self-play” has similarities to the “imagination” of the human brain – the brain also creates synthetic data through “imagination” for self-learning.
In summary, there are currently no hard limitations preventing the emergence of AGI, and we are only a few years away from it. What will carbon-based life forms do as they rush into silicon-based civilization?
Image: “Crumble of Liberty” with Dall-E
<2> Overestimating the Short-Term: No Unicorns at the Application Layer
Let’s temporarily jump out of long-term speculation and discuss something more practical: what is the actual capability of large models at the beginning of 2024?
In summary: GPT-4 is in college. It can chat, flirt, draw, and even complete some simple, clear tasks during internships; however, it often makes mistakes, gets lazy, is stubborn, and does not follow your instructions, leading to its own fabrications…
The seemingly prosperous AI ecosystem actually shows a significant gap between the applications that have truly emerged and people’s expectations. This is precisely where we have “overestimated the short-term capabilities of AI”.
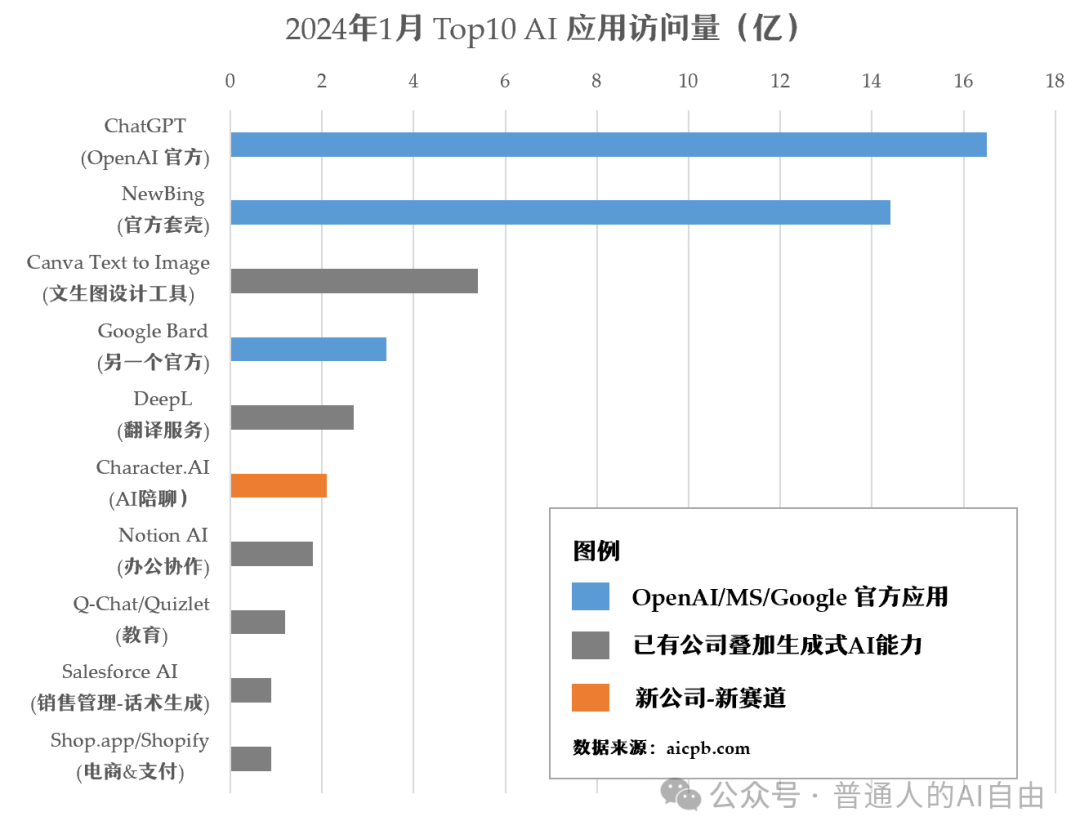
-
Serious Chatting: Except for ChatGPT, others are basically not effective. While everyone complains that OpenAI is too expensive, OpenAI’s revenue is only enough to cover the cost of inference services; new model training still relies entirely on financing.
-
Text-to-Image: The most profound impact on workers comes from products like Midjourney, Dall-E, and Stable Diffusion, which are completely revolutionizing the creative design industry. Following ChatGPT and Bing, the first application is the image generation app Canva. Text-to-image products have gone through several iterations in 2023, first focusing on drawing hands (which has been resolved), and then on detail control, reducing inference costs, and improving image generation speed (currently achievable in 300ms). The actual experience is that while the creativity is stunning, there are many poor-quality images, and detail modifications still require human intervention, so additional payments for quality are limited; meanwhile, despite several rounds of optimization, the cost of machines has not met the rising demands for image quality. Therefore, the only ones truly making money might be those creating anime girl images…
-
AI Companionship (AI Girlfriend/Boyfriend): Products like Character.ai, XH, and Doubao have achieved breakthroughs in 2023 as the only consumer-facing products. Users seem to accept some nonsensical talk when they are engaged, and these products can provide connections, whether straightforward or suggestive… I believe these companionship AI products have immense potential: this is the first step towards opening a new era of “human-AI connection” beyond human-to-human connections, and it can create a commercial moat based on “connection” in the future.
-
AI Legal Documents: This is currently the only relatively mature application of AI in the B2B sector. Legal documents have fixed formats, and the core technology relies on referencing laws/cases. This application is essentially a combination of “search” capabilities and large model capabilities.
-
Other B2B Applications: These are mostly loud but produce little. Although every company wants to associate with AI, in reality, very few have truly implemented it; “adding a ChatGPT shortcut in the browser” is the main approach for most companies that answered “using AI at work” in the survey. This is quite normal, as AI’s B2B capabilities are still lacking: even the best coding tools (like GitHub Copilot) are merely at the “intern level”; furthermore, the problem is that the speed of AI coding is much faster than humans can debug. Another seemingly accessible application is “AI customer service”, but the practical experience has not met expectations for significantly replacing human customer service.
I also tried using OpenAI’s API to build a “writing assistant”, but it completely failed to help write this article. My direct feeling was akin to “herding a group of runaway wild horses”: difficult to control, unable to fine-tune, lacking memory, and illogical behavior were the most painful aspects. Of course, my time spent was limited, and I didn’t properly research prompt engineering techniques. This process led me to reflect: What exactly are the current large models good at?
Hallucination = Imagination | Hallucination = Imagination
Based on the products and actual user experiences that emerged in 2023: the most mature capability of large models is not logic, but rather imagination and pleasing ability. This is likely logical. Firstly, the “hallucination problem” itself is an expression of imagination; moreover, during training, the model’s learning approach has always been “exhaustive inductive”, and we currently do not know how to “teach” the model logical methods, but can only hope that the model will spontaneously develop logical capabilities. Secondly, since the optimization goal during model training includes “keeping the conversation going”, it is the ability to please, rather than facts and logic, that large models excel in. This characteristic is something to consider carefully when choosing a product track: entertainment-oriented, creative, and consumer-facing products will mature faster than logical, B2B products.
Image: “AI Imagination”, with Dall-E
<3> Officially Crushing Startups: Is There Really a GPT Ecosystem?
Compared to the previous wave of mobile internet entrepreneurship, AI entrepreneurs face much greater challenges; a profound question is: how to avoid being crushed by the official offerings.
The shell companies riding on GPT’s capabilities like Jasper.ai (which relied on GPT to create advertising copy) have seen their value plummet to nearly zero following the release of GPT-4; the newly launched Sora has overshadowed previously flourishing companies like Runway and Pika… This is also the main reason why the investment community is currently very cautious about AI application companies: AI application companies lack a technological moat.
Hongshan created a map encompassing major AI startups, and the logos in it change as quickly as a revolving lantern. The euphemistic term is “ecological prosperity”, while the less flattering description is “a bear blindfolded with corn”.
On the ground, entrepreneurs face very tough choices:
<Route One>: API + Prompt + Product Shell: Suitable for companies with existing products/customers The most common products on the market are achieved through API calls, but the problem is that relying solely on API + Prompt cannot form a moat; Jasper’s downfall is the best example. The much-anticipated GPTs are also a low-threshold model of prompts, which may also explain why the GPT store currently lacks substantial prosperity: applications with large daily active users are either official offerings or extensions of existing companies’ products, with very few new business models. For general users, achieving fine control and stable output through GPTs remains a challenge. My understanding is that the era of personal “handcrafted applications” requires the capability of agents to be realized first.
For companies with existing products and users, adding AI applications is a good choice. Canva’s core competitiveness stems from its existing user base, friendly product design, massive templates for various social media, and one-click publishing features; the use of APIs and models only adds icing on the cake. Another currently successful example is Duolingo (a language learning app disguised as a casual game): its core moat lies in the design of casual game mechanics, while using OpenAI’s API significantly reduces the cost of its question bank, with no upper limit on the depth of the question bank.
<Route Two>: Open Source Models + Fine-Tuning: Suitable for companies with unique data
Companies with rich data can pursue the path of open-source models + fine-tuning. Unique data can allow fine-tuned models to perform better in specific scenarios. However, the practical implementation is quite challenging, and the core issues become: 1) Is the underlying open-source model strong enough? 2) After upgrades to the underlying model, can the previous fine-tuning experiences be reused? Unfortunately, both points are difficult to ensure, as the most powerful models are not open-sourced, and accumulating fine-tuning experiences under a black-box situation is also challenging. Thus, this business model is akin to building a tower on quicksand… The survival window depends on the speed of fine-tuning.
<Route Three>: Building Your Own Base Model: Only Suitable for Large Corporations and a Few Big Players
Training a foundational world model requires an investment in the billions of dollars and thousands of GPU cards; apart from a few large corporations like BBAT, it is very difficult for other companies. Even leading startups such as Zhizhu, Minimax, Luna’s Dark Side, and Baichuan will face significant challenges in the next round of financing. This is also why most domestic companies rush to launch products and cannot focus entirely on developing large models. Many companies in the second tier that claim to have world models are essentially selling “dog meat”.
Moreover, even among large corporations, only Google’s Gemini has the potential to challenge OpenAI within a year. Meta’s LLaMA is still half-baked; Amazon, Apple, and Tesla have made no significant progress; and domestic BBAT seems to be even further behind, with no one daring to claim they have reached the level of ChatGPT-3.5 comprehensively.
So, stepping back, what can ordinary entrepreneurs do at this moment? The only thought that comes to mind is “Speed is Key”: try to experiment continuously in smaller niches at low cost and high speed, without aiming to create a product for everyone, but rather to capture those small niche markets at low cost.
Lastly, remember that in this wave of AI, accelerated iteration of new technology is the norm; we cannot expect to take action when “technology stabilizes” because that moment will never come.
Image: “AI Entrepreneur”, with Dall-E
<4> AI Agent: Memory Issues Persist; Calling for a “Human Model”
AI agents are the most important concept after AGI itself, as they reveal the infinite possibilities of silicon-based civilization.
The core of the AI agent concept is: Set Goals-> Break Down Tasks-> Use Tools-> Make Decisions; Agents can Communicate in a Human-like Manner-> Build Social Cooperation Relationships Independently; Ultimately Achieve Imitate Humans-> Replace Humans. Since the publication of the “Stanford Town” paper, there have been numerous attempts, including BabyGPT, AutoGPT, etc.; OpenAI’s Assistant API and Function Calling features are also the first steps toward AI agents, allowing large language models to begin utilizing tools.
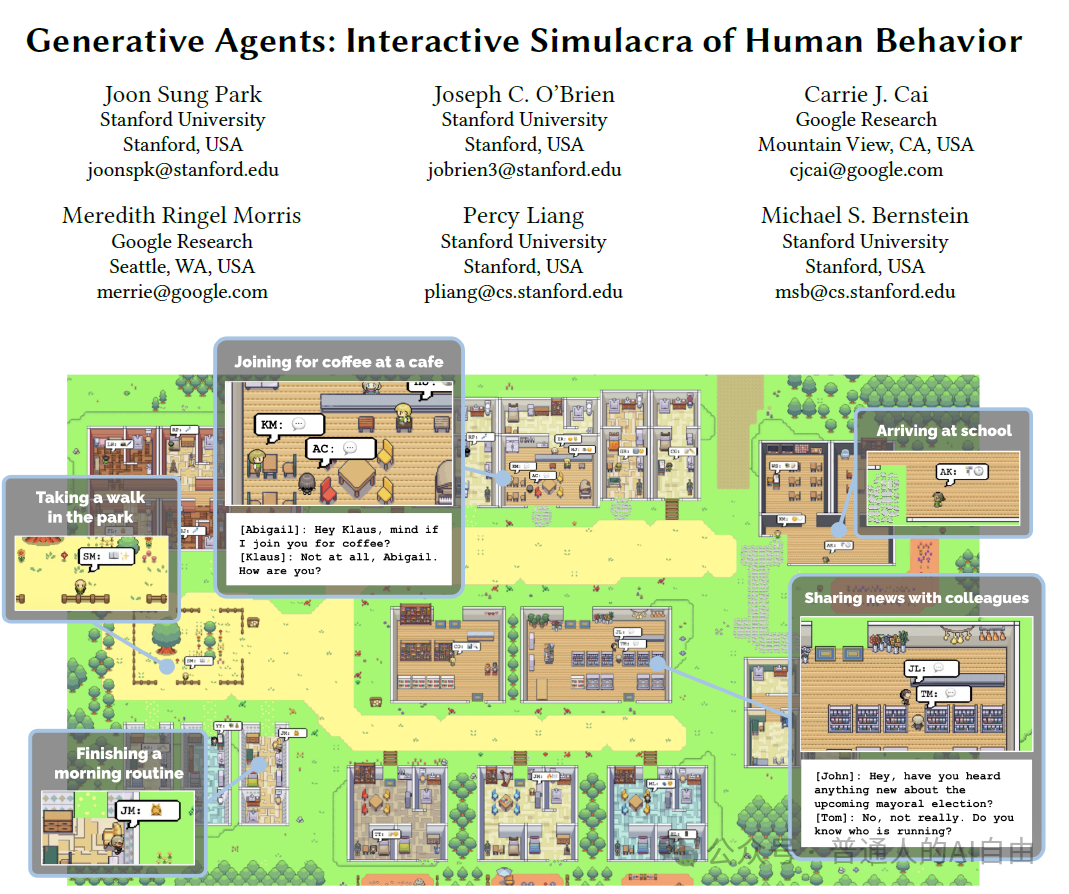
Paper Address: https://arxiv.org/abs/2304.03442
Fortunately, AI agents are still some distance from maturity. The most prominent issue is the memory problem. This involves technical aspects: memory accuracy, logical completeness, reasonable forgetting, balancing long-term memory mechanisms with token length, and calling efficiency. Memory capabilities and long token capabilities are the focus of many teams: the recently released Gemini1.5 has achieved a token length of 10M in research, which is two orders of magnitude greater than GPT-4; ChatGPT has also recently announced “memory capabilities”; the most prominent domestic company focusing on ultra-long tokens is “Luna’s Dark Side”.
There is also a category of intermediate-level products focusing on AI agents’ tool usage capabilities, with ByteDance’s Coze being a representative. In the current environment where the foundational models are not advancing, and upper-layer applications lack value, developing intermediate-level products may be the best choice. However, this opportunity may be quickly seized by well-capitalized large corporations. (Intermediate layers require substantial API fees or computing power.)
Stepping back, personality itself is an aggregation of memory. The more fundamental issue arising from memory problems is the memory independence of the AI agent’s “personality”, which directly relates to the current training methods of large models and underlying data. Memory independence is crucial because only when agents can understand “a specific person” can they collaborate effectively with that person and even replace them in decision-making.
However, upon closer examination, it is evident that current large language models are “world models”, not “human models”. This means that the underlying data comes from thousands of individuals, with only a tiny amount of data from each person; rather than large amounts of data from a single individual. Thus, a bold hypothesis arises: in a society where humans and AI fully cooperate, we need not only “world models” but also “human models”. At that time, agents will also differentiate into “world agents” and “personal agents”.
Of course, a pure silicon-based society without carbon-based humans is a different story.
Image: “Dialogue”, from LJH Color
<5> Deep Compression and Model Miniaturization
Another important direction besides AI agents is the miniaturization of large models. The direct benefit of smaller models is a significant reduction in training costs and inference costs. For example, the training cost of a comparable LLaMA-7B is equivalent to that of one A100 chip training for 9.3 years, while GPT-3 (175B) would require 100 years, a difference of 11 times. The training cost of GPT-4 is even as high as 6500 years!
However, cost is not the most significant meaning. “Compression” is the core capability of LLM models; the so-called “world model” aims to compress all human knowledge into the model. Following this line of thought, the significance of miniaturization lies in: miniaturized models allow a company, or even an individual, to use “the knowledge of all humanity” for the first time. Moreover, miniaturized models are the technical foundation for the “human model” mentioned above and a prerequisite for enabling “edge intelligence”. In the future, whether on phones, computers, or robots, there may be a mini model embedded.
A historical fork may emerge here: on one side is the “centralized, unified world model”, and on the other side is a “mixed society of edge intelligence + human models”. Let us wait and see.
Returning to today, miniaturization mainly has two levels. The first level consists of models with parameters in the range of 6B-7B, which can be covered by gaming graphics cards. The most representative are Meta’s LLaMA, Mistral7B, and domestic Zhizhu; NVIDIA’s Chat with RTX defaults to Mistral7B.
The second level of models has a larger imaginative space, with parameters in the range of 1-2B; this size can be widely used on mobile devices and hardware. Huawei, Honor, Xiaomi, OPPO, and VIVO have announced plans to embed large models on mobile devices, although they currently rely on the latest Snapdragon 8 Gen 3 flagship processors, and issues of energy consumption and heat dissipation remain; Samsung’s S24 also features Google Gemini Nano.
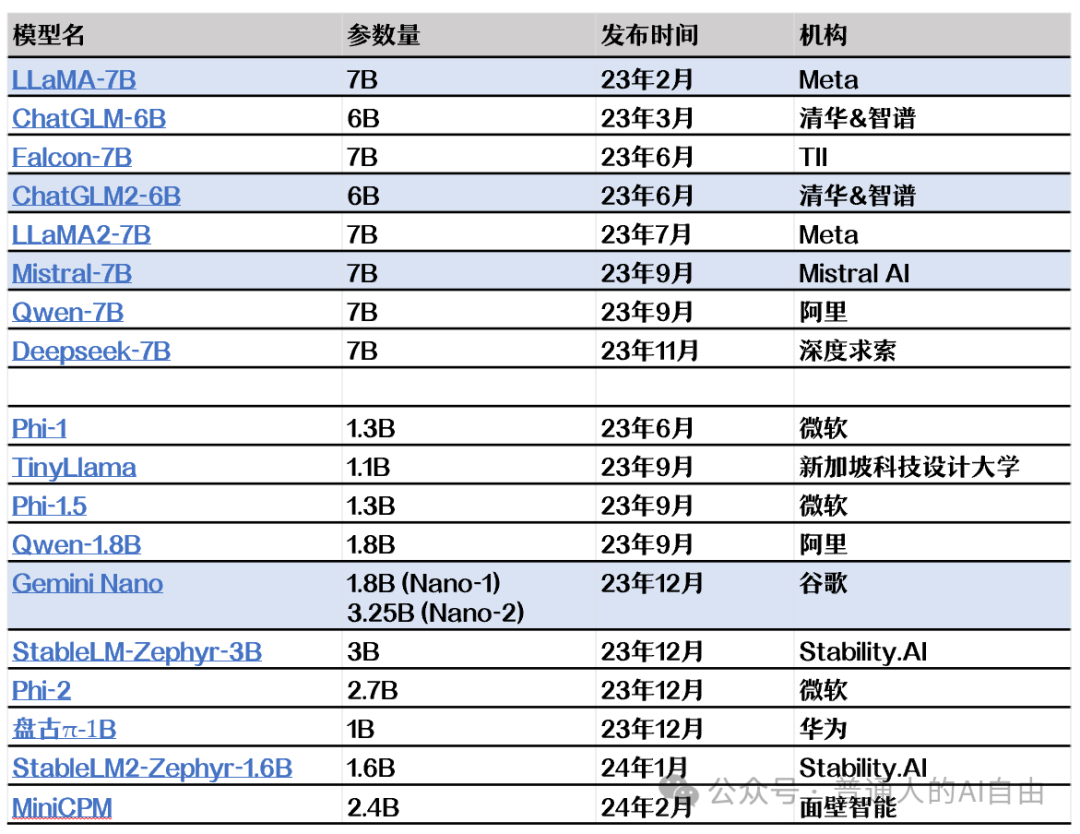
But do not rejoice too soon; a profound question arises: can miniaturized models truly possess the capabilities of large models? Are miniaturized models genuinely “world models” or merely “narrow AI question-answering machines”? Currently, it appears that when model scales are compressed, stability and memory capabilities may be compromised.
From a technical perspective, the optimization methods for miniaturization (Quantization, Batchsize Optimization, Learning Rate Scheduler, Neural architecture, etc.) can mostly also be applied to large models, so it is expected that the capabilities of smaller models lag behind those of larger models by one generation. However, on the other hand, the success of Mistral7B at least demonstrates that training/distilling based on data output from large models can rapidly replicate most capabilities of large models.
Image: “Dictatorship”, with Dall-E
<6> The Explosion of Multimodal Capabilities: Video, Audio, and Virtual Humans
From the end of 2023 to today, the most striking development has been the explosion of multimodal capabilities in videos and audio.
Video. Runway and Pika have shown us the possibilities of text-to-video generation, and numerous high-quality text-to-video editing tools have emerged in Q4 2023.
However, faster than expected was the dimensionality reduction impact from Sora. The emergence of Sora once again proves the generational superiority of AGI over “narrow AI”.
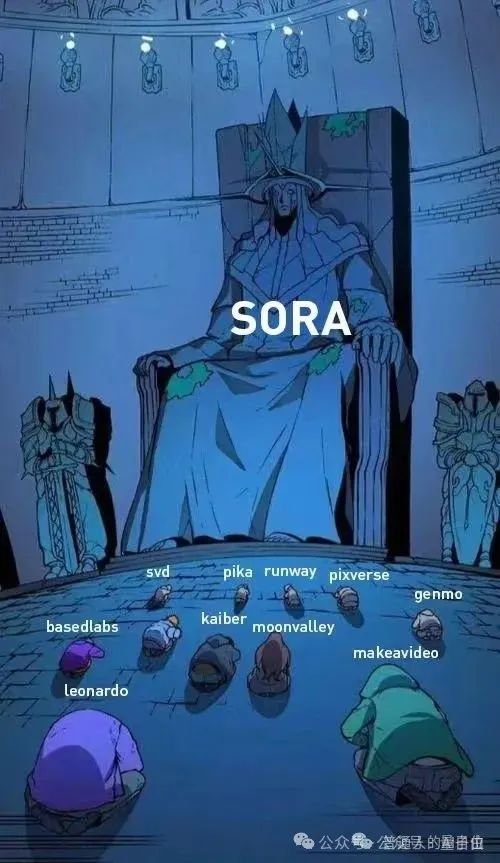
In addition to video, Sora has also achieved over 70% capability in 3D abilities. We are about to witness a major reshuffling in the video, film, and gaming production industries. Of course, Sora is not omnipotent; it still needs better physical world understanding capabilities, which may be due to a lack of data from sensors for “touch”, “gravity”, and “inertia” in the digital world. However, virtual reality is already on the horizon.
Another advancement in multimodal technology is in audio. With the introduction of generative AI, technology has reached the point of low-cost voice cloning (e.g., ByteDance and MiniMax have capabilities for rapid audio cloning within ten seconds) and highly natural text-to-speech technology (e.g., ElevenLabs). Specifically, in practical operations, it is now possible to create highly realistic audio in everyday/business and music scenarios (like AI Sun Yanzi, Suno, etc.) where complex/random emotional variations are not present. The current gap lies in emotionally rich conversational scenarios. However, bridging this gap requires first understanding emotions. This is reminiscent of the video generation industry before Sora’s emergence; in the not-too-distant future, we can also look forward to a dimensionality reduction impact from GPT in the audio industry.
Stepping back, the progress of various multimodal technologies gives me a sense of conversion: an era of true human imitation is approaching. There is no truth anymore online. In the future, it may only be real when we meet offline.
Image: “Faces”
<7> Domestic Progress and Bottlenecks
From an industry insider’s perspective, the most surprising aspect of the emergence of ChatGPT and GPT-4 is not its capabilities but rather the excellence of its confidentiality work. Before 2023, the domestic AI industry was still complacent, believing it was only a month or so behind the US and that it had advantages in population data; this narrative was abruptly shattered by GPT-4. OpenAI, without any warning in the domestic market, has directly opened a two-year technological gap. The specific reasons may include domestic arrogance, being misled by Google’s previously promoted T5 technology route, or the immense impact of AGI, which government agencies like the FBI and the US Department of Defense surely communicated with OpenAI about.
OpenAI’s success is a miracle born from hard work, so in the first half of 2023, the domestic market also firmly believed that as long as there were cards and money, they could “forge steel on a large scale”. During that time, whether it was large corporations hoarding cards and hiring talent to research GPT architectures or startups telling the story of a Chinese OpenAI while securing massive funding, everyone aimed to be the first to create domestic AGI. However, by the second half of the year, after experimenting and discovering the challenges, they all turned to focus on “vertical applications” and “commercialization”; AGI was no longer mentioned. This shift is short-sighted and even fatal. In 2023, the technological gap between China and the US in AGI did not narrow. Currently, the most advanced domestic model is roughly at the level of ChatGPT-3.5, with a significant gap to GPT-4; it may even be inferior to the makeshift Mistral team’s level.
Large Corporations. Large corporations possess the talent, GPU, data, and capital reserves to challenge AGI, but in terms of actual results, there have been no clear highlights. However, under the pressure of internal short-term assessments, most forces should be focused on rolling out new products and reporting to higher-ups. From another perspective, while these models may have average capabilities, their integration with business is relatively thorough. Lastly, large corporations bear too many other business and political considerations: whether to take the lead in developing large models, many companies must think twice.
Specifically, Baidu and Alibaba are among the more high-profile large corporations: Baidu’s “Wenxin 4.0” is currently one of the best models domestically, and Alibaba’s upcoming “Tongyi Qianwen” QwenVL-MAX and Qwen2.0 also have good metrics. Additionally, the integration of Alibaba’s Qianwen and DingTalk, as well as Baidu’s Wenxin and search, are also commendable. ByteDance’s “Lark” and Tencent’s “Hunyuan” are relatively low-key; on one hand, it reflects the company’s cultural characteristics, and on the other hand, it likely indicates that they have not yet achieved clear highlights. However, ByteDance is determined in product development and resource mobilization: the Flow department has Doubao and Kouzi; it will also launch the AI role interaction app “Hualu” and the image product Picpic.
Flow is led by Zhu Wenjia, with Hong Dingkun working on models, and Zhang Nan focusing on video editing, alongside cutting down on gaming and VR… ByteDance’s all-in commitment to AI may be the strongest in terms of determination and action.
Startups. Currently, the first-tier companies with good models and products are as follows:
-
Zhizhu: Over the past year, it has launched four generations of GLM, consistently being one of the best models domestically.
-
MiniMax: Launched a new model with MoE architecture, and “Xingye” is currently the most successful AI companionship app in the country.
-
Luna’s Dark Side: Focused on long token capabilities, with commendable memory and long token abilities.
Other than these, I will not list others for now; in 2023, many companies have officially announced AI large models, among which many are undoubtedly just riding the wave. Moreover, large models indeed have thresholds; companies that have secured funding still have some money to spend, so we can afford to give them more time to see the results in 2024. (The judgment method is not objective; discussions are welcome.)
From the product perspective, the only truly breakout product in the consumer sector is the “Miao Duck Camera”, but it was only a flash in the pan. Most consumers’ attitudes towards AI products are “curiosity” rather than necessity. In the B2B sector, large models are still primarily “pure technical investments”, with very limited revenue leverage; while the large corporations selling AI actually aim to sell cloud services…
Lastly, the hardware bottlenecks have not eased. Currently, there are still no chips capable of training large models domestically. However, alternatives to Nvidia are gradually emerging for inference. The Huawei Ascend chip is noteworthy for its single-card performance, but due to instability and the lack of a CUDA (hardware compilation library) ecosystem, it still requires time to refine. The US’s chip embargo on the domestic market will further deepen; therefore, apart from rolling out models, entrepreneurship based on the Ascend ecosystem is an opportunity, and it is a more certain opportunity.
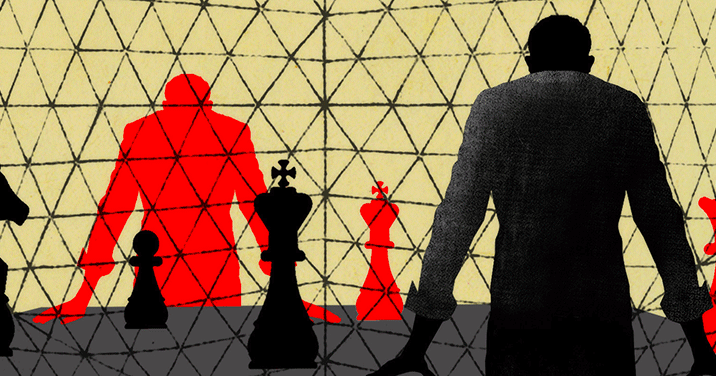
Postscript: Major Events in AGI in 2023
-
In November 2022, ChatGPT was launched: “Wow!”, large language models entered the public eye
-
In February 2023, ControlNet was proposed: AI image generation control foundation, text-to-image became a true productivity tool
-
In February 2023, LLaMA was open-sourced: the counterattack of the open-source ecosystem, providing a foundation for most companies’ “self-research” efforts
-
In March 2023, GPT-4 was launched: “The First Contact with AGI“, humanity began to see the shadow of the “world model“
-
In March 2023, Nvidia H100 was released, significantly enhancing the computational foundation for large models
-
In April 2023, the Stanford Town paper – AI Agent: The Infinite Possibilities of Silicon-Based Civilization: the first experiments on tool usage, collaboration, and decision-making abilities
-
In September and December 2023, Mistral-7B and Mixtral-8x7B were open-sourced: a milestone in model miniaturization, leveling up and surpassing GPT-3.5 (175B); Europe now has large model teams
-
In November 2023, GPTs + Assistants API: The initial form of agents and the construction of the GPT ecosystem
-
In November 2023, OpenAI’s internal conflict: the radical faction – CEO – capital spokesperson Sam Altman won, while the rescue faction – chief scientist – idealist Ilya Sutskever was ousted.
-
In December 2023, the Google Gemini series was released: OpenAI’s competitors started to play their cards, the competition officially accelerates
-
In February 2024, Nvidia Chat with RTX was released: The dawn of edge intelligence
-
In February 2024, OpenAI Sora was released: a generational leap in video generation, once again proving the generational superiority of AGI over “narrow AI”; virtual reality becomes possible
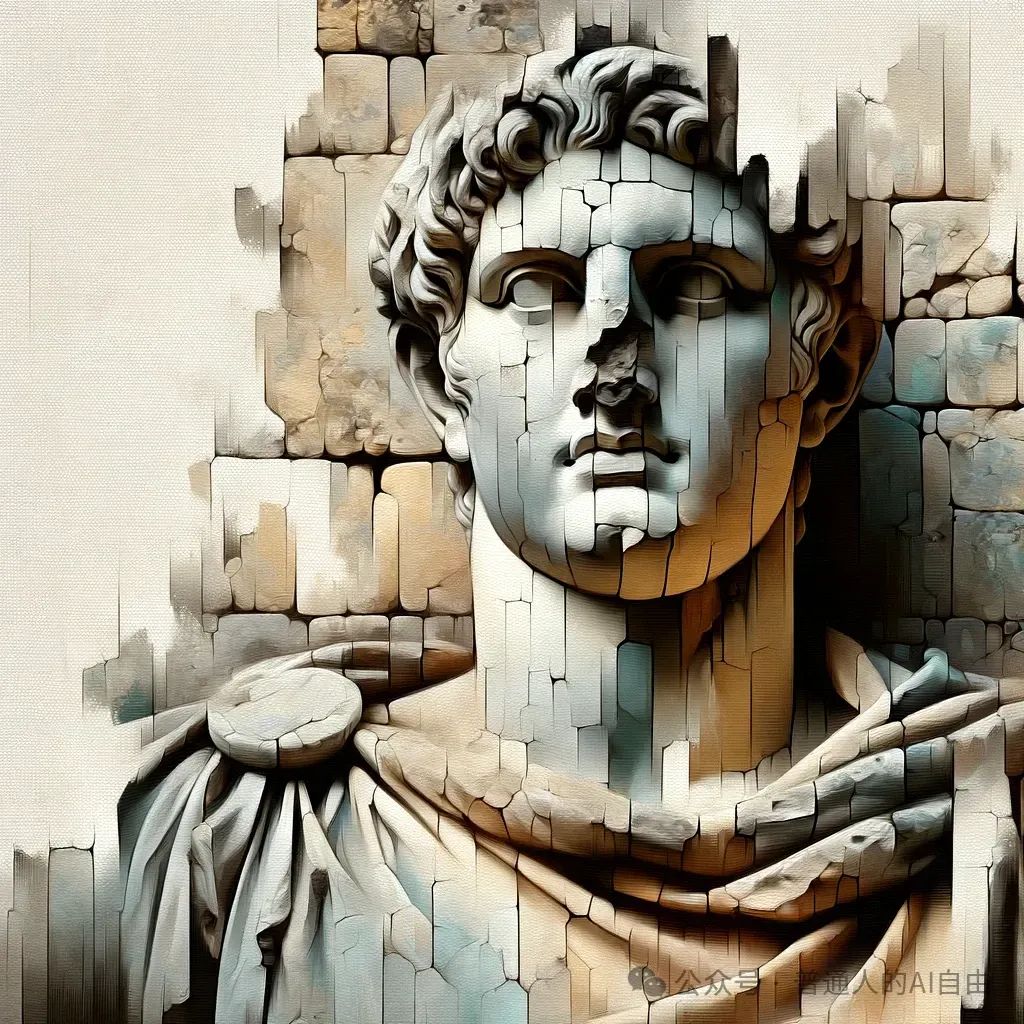
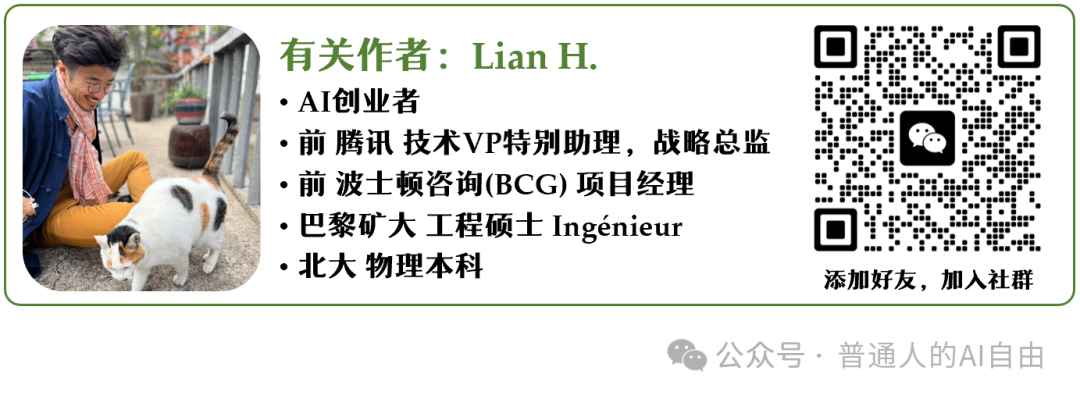